Bibliographic Research: Definition, Types, Techniques
The Bibliographic research or documentary consists in the revision of existing bibliographical material with respect to the subject to be studied. It is one of the main steps for any investigation and includes the selection of information sources.
It is considered an essential step because it includes a set of phases that encompass observation, inquiry, interpretation, reflection and analysis to obtain the necessary bases for the development of any study.

- 1 Definition
- 2 characteristics
- 3.1 Argumentative or exploratory type
- 3.2 Informative or of the expository type
- 4.1 Relevance
- 4.2 Exhaustive
- 4.3 Present
- 5.1 Accumulate references
- 5.2 Select references
- 5.3 Incorporate elements in the work plan
- 5.6 Confront and verify
- 5.7 Correct and make the final revisions
- 6.1 Primary
- 6.2 Secondary
- 6.3 Tertiary
- 7 Examples of bibliographical references
- 8 Importance
- 9 References
Different authors have been in charge of conceptualizing bibliographic research. Next, the definitions made by three prominent authors will be described:
- Guillermina Baena, graduated in Information Sciences (1985):"Documentary research is a technique that consists of the selection and collection of information through reading, critique of documents and bibliographic materials, libraries, newspaper archives and educational centers. documentation and information."
- Laura Cázares, researcher at the Autonomous Metropolitan University of Mexico (2000):"(....) Depends primarily on the information collected or consulted in documents that can be used as a source or reference at any time or place."
- Manual of the Universidad Pedagógica Experimental Libertador (UPEL -2005):"Integration, organization and evaluation of existing theoretical and empirical information on a problem".
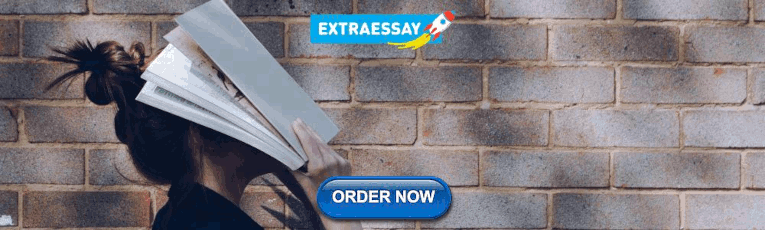
characteristics
- There is a review of documents to know the state of the subject or object that is being investigated.
- Presents a process that consists in the collection, selection, analysis and presentation of the results.
- Involves complex cognitive processes, such as analysis, synthesis and deduction.
- It is done in an orderly manner and with precise objectives.
- Its purpose is the construction of knowledge.
- It supports the research that is being carried out, at the same time that it allows to avoid carrying out studies already explored.
Types of bibliographic research
In general terms, there are two types of bibliographic or documentary research:
Argumentative or exploratory type
The main objective of the researcher is to take a position on a certain topic to test whether that element to study is correct or incorrect. Consider causes, consequences and possible solutions that will lead to a conclusion more critical type.
Informative or of the expository type
Unlike the previous one, it does not seek to object to a topic but to recreate the theoretical context of the investigation. For this it uses reliable sources, and the selection and analysis of the material in question.
Criteria for the selection of material
It should be noted that it is vital for the researcher to rely on his capacity for analysis and synthesis of ideas to present a fluid and coherent work. During the bibliographic research process it is necessary to consider a series of criteria for the selection of documentary material:
It refers to the fact that the sources must be consonant with the object of study, as well as their objectives, in order to base the investigation.
All sources must be necessary, sufficient and possible, without excluding any that may also represent an important contribution. They must correspond to the objectives set.
Recent research or studies to support the research will be taken into account.
It is important to point out that before carrying out the review of documentary and bibliographic material, it is vital to be clear about the following:
- Determine the subject to be studied, which must be combined with the possibilities of the researcher, framed in a prudential time with future projection and with a connection to his area of study.
- After this, make a work plan that will serve as a guide for the correct selection of bibliography.
The process of collecting data, information and documents is complex and requires a series of steps for the correct handling of information:
Accumulate references
The references include any type of written or audiovisual document that will be essential to support the investigation.
Select references
The material that respects the quality and current standards will be chosen.
Incorporate elements in the work plan
It deals with the organization of the chosen documents in alphabetical or chronological order.
It refers to the emptying of the basic information of the collected material, where the appointment to be used, the summary and the comment made by the researcher will be collected.
Placement of specific data.
Confront and verify
The aim is to determine if, indeed, the hypothesis raised by the author is valid, based on the information collected.
Correct and make the final revisions
It refers to the latest arrangements made to the form and background of the investigation.
Types of documents
To simplify the search and make it easier, three types of documents are classified:
They transmit direct information. For example, original articles and doctoral theses.
They make reference to the primary documents and extract the author and the type of publication. For example, catalogs and databases.
They synthesize the information found in the primary and secondary documents to answer questions and specific questions.
Likewise, another type of document classification can be included:
- Books and monographs: manuals, texts, minutes, anthologies.
- Periodicals: magazines, newspapers, advances.
- Reference publications: indexes, databases, bibliographies.
- Technical publications: standards, patents, technical catalogs.
- Reference material: encyclopedias, dictionaries, atlas.
Examples of bibliographical references
In bibliographic research it is necessary to respect the rules related to the citation of texts. To have a better reference in this regard, here are some examples:
-"Pinillos, José Luis (1975). Principles of Psychology. Madrid: Alliance."
-"Taylor, S. and Bogdan, R. (1992). Introduction to qualitative research methods. Barcelona: Paidós."
- When it is a chapter of a book:"Martí, Eduardo (1999). Metacognition and learning strategies. In: J Pozo and C. Monereo (Coords.). The strategic learning. (111-121). Madrid: Classroom XXI-Santillana".
- Scientific journal article:"García Jiménez, E. (1998). A practical theory about evaluation. Journal of Education, 287, 233-253."
- Article signed in a newspaper:"Debesa, Fabián (200, March 12). Careers and their entry strategies. Clarín, Education Section, p.12".
- Any field of study needs constant study and research.
- It is estimated that, thanks to documentary and bibliographic research, it is possible to achieve a good educational training at all levels.
- The progress of scientific studies needs documentation.
- To start any type of study it is necessary to review previous material to carry out the investigation.
- What is the bibliographic review? (s.f.). In Philosophia, Scientia et Praxis. Retrieved: March 1, 2018. In Philosophia, Scientia et Praxis de filoncien.blogspot.pe.
- About bibliographic and documentary research. (2013). In Thesis Guide. Retrieved: March 1, 2018. In Thesis Guide of guiadetesis.wordpress.com.
- Córdoba González, Saray. (s.f.). The bibliographic research . In Ucrindex. Retrieved: 01 d March 2018. In Ucrindex of ucrindex.ucr.ac.cr.
- Definitions Documentary Investigation. (s.f.). In Scribd. Retrieved: March 1, 2018. On Scribd from es.scribd.com.
- Bibliographic research. (s.f.). In Monographs. Retrieved: March 1, 2018. Monographs of monographs.com.
- Mora De Labastida, Natalia. (s.f.). The bibliographical investigation. Main and secondary ideas . In Fido. Retrieved: March 1, 2018. In Fido from fido.palermo.edu.
- Types of research. (s.f.). In research thesis. Retrieved: March 1, 2018. In research thesis of tesisdeinvestig.blogspot.pe.
Recent Posts

Writing your Dissertation / Thesis
- Getting started
- Dissertations and theses
- Bibliographic research and literature review
- Citations and bibliography
- Copyright and plagiarism
- Ask a Librarian
Bibliographic research
The search and collection of information from published sources (books, journals, newspapers, etc.) nowadays may include other types of documents, such as websites, reports from bibliographic databases, etc.
Searching for bibliographic sources relevant to your project is an integral and unavoidable part of the thesis work.
To find out how to conduct your bibliographic research, we suggest you consult the Bibliographic Research Guide .
For a start, you can consult the Library books on academic writing (how to write assignments, presentations, theses ...):
Literature review
Literature review is the analysis of the academic literature (articles, books, dissertations, theses, etc.) that you have identified when performing your search on the topic.
A review of the relevant literature for the topic selected is a key element of any academic project (dissertation or PhD thesis, writing an article for an academic journal…) for several reasons:
• it provides you with the conceptual context for your research
• it allows you to acquire, deepen and organize knowledge in the chosen research area
• helps you define or better focus your research objectives
Furthermore, its objectives are:
- describing the state-of-the-art on the given subject (what is the knowledge achieved so far in the research area in which your project fits?)
- identifying strengths and weaknesses, potential gaps in the current knowledge, unexplored empirical issues, or issues that need to be updated
- understanding how the research question is positioned within the field (to what extent does your work provide an original contribution to the research context?)
Want to learn more about the literature review? Explore the Project Planner on SAGE Research Methods .
If you notice that a significant book or resource is not included in the Library collections, please let us know : the Library will consider acquiring it!
- << Previous: Dissertations and theses
- Next: Citations and bibliography >>
- Last Updated: Jul 29, 2024 10:15 AM
- URL: https://unibocconi.libguides.com/dissertation

ENGL 5374: Methods of Bibliographic and Research Analysis
Methods of bibliographic and research analysis.
- Course Reserves
Textbooks for this Course
MLA Resources
A collection of links to help you with MLA.
- Purdue OWL MLA Style Guide Purdue OWL guide to MLA style.
- KnightCite A citation maker where you enter the information.
- MLA Style @ MLA The main page for MLA Style on the MLA homepage.
- Citing Government Information Sources Using MLA Style MLA help for citing legal documents and other types of government reports.
- Purdue OWL YouTube Tutorials A website with video tutorials for citing, formating, and writing.
- Purdue OWL Citation Style Comparison Chart A chart that outlines the similarities and differences between APA, MLA, and CML.
MLA Games and Tests
A collection of games and tests online to help you assess your knowledge of citing and plagiarism.
- APA and MLA Citation Game @ University of Washington A game from the University of Washington TRIO Training.
- Avoiding Plagiarism @ University of Arizona Libraries This tutorial shows how accidental plagiarism can occur and how to avoid it.
- Goblin Threat @ Lycoming College An game from Lycoming College about avoiding plagiarism.
- MLA Master Blaster @ Williams College A citation game from the Williams College Libraries.
- MLA Tutorial @ University of Southern Mississippi An interactive tutorial with a pre-test and post-test from the University of Southern Mississippi Libraries.
Google Scholar

This guide will help you find resources to research in a variety of English and humanities disiplines.
Recommended Databases
Search in library databases to find scholarly journal articles on your research topic.
Keywords: The right search term is important. Think about the words an expert might use to describe the concepts you are looking for.
Peer Reviewed: Look for search options that let you limit your search to articles that have been reviewed by experts.
Recent: Try to choose articles written in the last ten years to insure that your aren't retrieving outdated research.
Hover over the "i" icon to find out more about each database.
Use the Google Scholar link on the left-hand of this page to see how many times an article has been cited. Google Scholar indexes both traditional library databases as well as Open Access scholarly content.
- MLA Directory of Periodicals Indexes critical materials on literature, criticism, drama, languages, linguistics, and folklore. Provides access to citations from over 3,500 journals, series, books, essay collections, working papers, proceedings, dissertations, and bibliographies. Coverage is international. The MLA Directory of Periodicals contains all information available on the journals and series on the bibliography's Master List of Periodicals.
- MLA International Bibliography Indexes critical materials on literature, criticism, drama, languages, linguistics, and folklore. Provides access to citations from over 3,500 journals, series, books, essay collections, working papers, proceedings, dissertations, and bibliographies. Coverage is international. The MLA Directory of Periodicals contains all information available on the journals and series on the bibliography's Master List of Periodicals.
Serves as the central resource for researchers at all levels. Covering more than 160 subjects areas, ProQuest Central is the largest aggregated database of periodical content. This award-winning online reference resource features a highly-respected, diversified mix of content including scholarly journals, trade publications, magazines, books, newspapers, reports and videos.
Use Full Text Finder to find a journal in our library databases:
- Go the the library homepage .
- Click on Journal s .
- Type in the name of the journal (not the title of the article).
- Click "search" or hit "enter."
- In the result list, click on "Full Text Access."
- Click on the link with the best date ranges for your needs.
- If the journal is not available in full text, request it through interlibrary loan.
To check if a journal is peer reviewed, use the submission guidelines on the journal's webpage.
Or click on the journal titles:
- American Literature
- Cultural Anthropology
- Cultural Studies
- Cultural Studies/Critical Methodologies
- ELH: English Literary History
- Modern Fiction Studies
- New Literary History
- Nineteenth-Century Literature
- Shakespeare Quarterly
- War, Literature and the Arts
- War and Society
Reliable Websites for Literature
- 18thConnect Eighteenth-Century Scholarship Online, this is a free collection of peer-reviewed digital-objects from across the internet.
- Internet Archive A free online archive of video, audio, text, and web documents. Includes the Wayback Machine, an archive of websites over time.
- Library of Congress Digital Collections Digitized materials from collections at the Library of Congress.
- Making of America Primary sources from American social history.
- NINES Nineteenth-Century Scholarship Online, this is a free collection of peer-reviewed digital-objects from across the internet.
Reference Librarian

Live Research Help
Composition.
A collection of links to help you with composing your writing.
- Purdue OWL Writing Writing help from OWL at Purdue.
- Elements of Style by Strunk and White The original version of this classic writing guide.
- Texas A&M University Writing Lab in College Station The writing lab at TAMU in College Station.
- Central Texas University Writing Center For writers of all ability levels and all stages of the writing process! Thanks for choosing the University Writing Center (UWC) for help with your overall writing process and various writing assignments/tasks!
A collection of links to help you with grammar.
- Purdue OWL Grammar More than just citing help--Purdue OWL also provides a usage guide.
- Grammar Girl A collection of short explanations and tutorials about grammar and writing style.
- Dr. Grammar A resource from the University of Northern Illinois.
Open Source Academic Journals and Preprints
These search engines retrieve open source academic journals.
- DOAJ: Directory of Open Access Journals
- OAIster: Find the Pearls A free catalog that contains digital resources from open archive collections Represents multidisciplinary resources from more than 1,100 contributors worldwide. Records contain a digital object link allowing users access to the object in a single click. Subjects included: Digitized books and articles, Born-digital texts, Audio files, Images, Movies, Datasets, Theses, Technical reports, Research papers, Image collections.
- OpenDOAR: Directory of Open Access Repositories A vetted directory of open access repositories.
- Registry of Open Access Repositories A registry that lists all of the digital repositories online with open access content policies.
- Next: Course Reserves >>
- Last Updated: Aug 5, 2024 8:47 AM
- URL: https://tamuct.libguides.com/c.php?g=904005
- Background Information
- Find Articles
- Get the Full Text of a Journal Article
- Why Can't I Find That Article?
Library Research Methods
- Evaluating Websites
- Citing Sources
- Productivity Tools for Scholars
(Adapted from Thomas Mann, Library Research Models )
Keyword searches . Search relevant keywords in catalogs, indexes, search engines, and full-text resources. Useful both to narrow a search to the specific subject heading and to find sources not captured under a relevant subject heading. To search a database effectively, start with a Keyword search, find relevant records, and then find relevant Subject Headings. In search engines, include many keywords to narrow the search and carefully evaluate what you find.
Subject searches . Subject Headings (sometimes called Descriptors) are specific terms or phrases used consistently by online or print indexes to describe what a book or journal article is about. This is true of the library’s Catalog as well as many other library databases .
Look for recent, scholarly books and articles. Within catalogs and databases, sort by the most recent date and look for books from scholarly presses and articles from scholarly journals. The more recent the source, the more up-to-date the references and citations.
Citation searches in scholarly sources . Track down references, footnotes, endnotes, citations, etc. within relevant readings. Search for specific books or journals in the library’s Catalog . This technique helps you become part of the scholarly conversation on a particular topic.
Searches through published bibliographies (including sets of footnotes in relevant subject documents). Published bibliographies on particular subjects (Shakespeare, alcoholism, etc.) often list sources missed through other kinds of searches. BIBLIOGRAPHY is a subject heading in the Catalog , so a Guided Search with BIBLIOGRAPHY as a Subject and your topic as a keyword will help you find these.
Searches through people sources (whether by verbal contact, e-mail, etc.). People are often more willing to help than you might think. The people to start with are often professors with relevant knowledge or librarians.
Systematic browsing, especially of full-text sources arranged in predictable subject groupings . Libraries organize books by subject, with similar books shelved together. Browsing the stacks is a good way to find similar books; however, in large libraries, some books are not in the main stacks (e.g., they might be checked out or in ReCAP), so use the catalog as well.
The advantages of trying all these research methods are that:
Each of these ways of searching is applicable in any subject area
None of them is confined exclusively to English-language sources
Each has both strengths and weaknesses, advantages and disadvantages
The weaknesses within any one method are balanced by the strengths of the others
The strength of each is precisely that it is capable of turning up information or knowledge records that cannot be found efficiently—or often even at all—by any of the others
How to Gut a (Scholarly) Book in 5 Almost-easy Steps
Evaluating sources.
From Wayne C. Booth et al., The Craft of Research , 4th ed., pp.76-79
5.4 EVALUATING SOURCES FOR RELEVANCE AND RELIABILITY When you start looking for sources, you’ll find more than you can use, so you must quickly evaluate their usefulness; use two criteria: relevance and reliability.
5.4.1 Evaluating Sources for Relevance
If your source is a book, do this:
- Skim its index for your key words, then skim the pages on which those words occur.
- Skim the first and last paragraphs in chapters that use a lot of your key words.
- Skim prologues, introductions, summary chapters, and so on.
- Skim the last chapter, especially the >rst and last two or three pages.
- If the source is a collection of articles, skim the editor’s introduction.
- Check the bibliography for titles relevant to your topic.
If your source is an article, do this:
- Read the abstract, if it has one.
- Skim the introduction and conclusion, or if they are not marked by headings, skim the first six or seven paragraphs and the last four or five.
- Skim for section headings, and read the first and last paragraphs of those sections.
If your source is online, do this:
- If it looks like a printed article, follow the steps for a journal article.
- Skim sections labeled “introduction,” “overview,” “summary,” or the like. If there are none, look for a link labeled “About the Site” or something similar.
- If the site has a link labeled “Site Map” or “Index,” check it for your key words and skim the referenced pages.
- If the site has a “search” resource, type in your key words.
This kind of speedy reading can guide your own writing and revision. If you do not structure your report so your readers can skim it quickly and see the outlines of your argument, your report has a problem, an issue we discuss in chapters 12 and 14.
5.4.2 Evaluating Sources for Reliability You can’t judge a source until you read it, but there are signs of its reliability:
1. Is the source published or posted online by a reputable press? Most university presses are reliable, especially if you recognize the name of the university. Some commercial presses are reliable in some fields, such as Norton in literature, Ablex in sciences, or West in law. Be skeptical of a commercial book that makes sensational claims, even if its author has a PhD after his name. Be especially careful about sources on hotly contested social issues such as stem-cell research, gun control, and global warming. Many books and articles are published by individuals or organizations driven by ideology. Libraries often include them for the sake of coverage, but don’t assume they are reliable.
2. Was the book or article peer-reviewed? Most reputable presses and journals ask experts to review a book or article before it is published; it is called “peer review.” Many essay collections, however, are reviewed only by the named editor(s). Few commercial magazines use peer review. If a publication hasn’t been peer-reviewed, be suspicious.
3. Is the author a reputable scholar? This is hard to answer if you are new to a field. Most publications cite an author’s academic credentials; you can find more with a search engine. Most established scholars are reliable, but be cautious if the topic is a contested social issue such as gun control or abortion. Even reputable scholars can have axes to grind, especially if their research is financially supported by a special interest group. Go online to check out anyone an author thanks for support, including foundations that supported her work.
4. If the source is available only online, is it sponsored by a reputable organization? A Web site is only as reliable as its sponsor. You can usually trust one sponsored and maintained by a reputable organization. But if the site has not been updated recently, it may have been abandoned and is no longer endorsed by its sponsor. Some sites supported by individuals are reliable; most are not. Do a Web search for the name of the sponsor to find out more about it.
5. Is the source current? You must use up-to-date sources, but what counts as current depends on the field. In computer science, a journal article can be out-of-date in months; in the social sciences, ten years pushes the limit. Publications have a longer life in the humanities: in philosophy, primary sources are current for centuries, secondary ones for decades. In general, a source that sets out a major position or theory that other researchers accept will stay current longer than those that respond to or develop it. Assume that most textbooks are not current (except, of course, this one).
If you don’t know how to gauge currency in your field, look at the dates of articles in the works cited of a new book or article: you can cite works as old as the older ones in that list (but perhaps not as old as the oldest). Try to find a standard edition of primary works such as novels, plays, letters, and so on (it is usually not the most recent). Be sure that you consult the most recent edition of a secondary or tertiary source (researchers often change their views, even rejecting ones they espoused in earlier editions).
6. If the source is a book, does it have a notes and a bibliography? If not, be suspicious, because you have no way to follow up on anything the source claims.
7. If the source is a Web site, does it include bibliographical data? You cannot know how to judge the reliability of a site that does not indicate who sponsors and maintains it, who wrote what’s posted there, and when it was posted or last updated.
8. If the source is a Web site, does it approach its topic judiciously? Your readers are unlikely to trust a site that engages in heated advocacy, attacks those who disagree, makes wild claims, uses abusive language, or makes errors of spelling, punctuation, and grammar.
The following criteria are particularly important for advanced students:
9. If the source is a book, has it been well reviewed? Many fields have indexes to published reviews that tell you how others evaluate a source.
10. Has the source been frequently cited by others? You can roughly estimate how influential a source is by how often others cite it. To determine that, consult a citation index.
- << Previous: Why Can't I Find That Article?
- Next: Evaluating Sources >>
- Last Updated: Jul 31, 2024 9:15 AM
- URL: https://libguides.princeton.edu/philosophy
Educational resources and simple solutions for your research journey
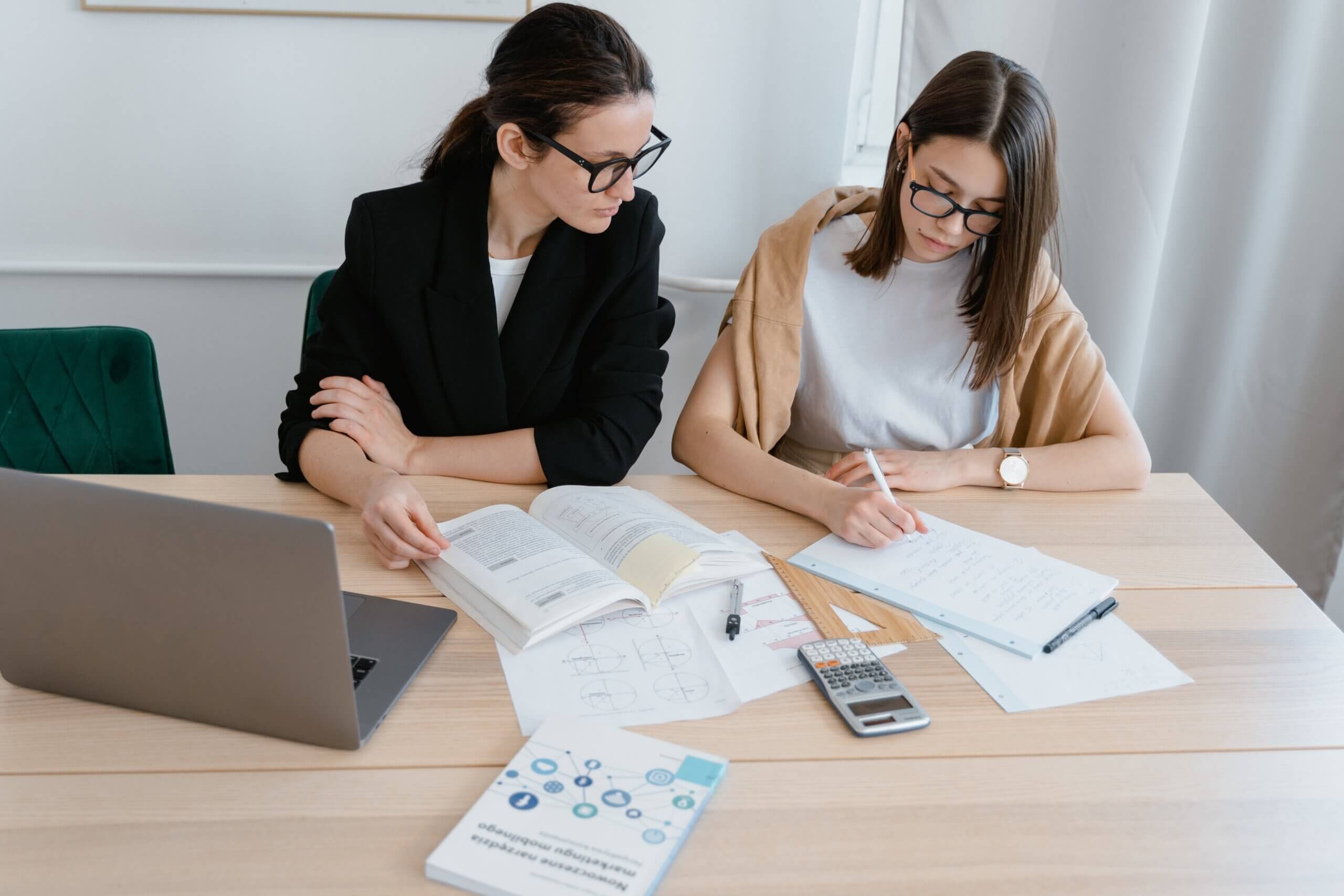
A Beginner’s Guide to Citations, References and Bibliography in Research Papers
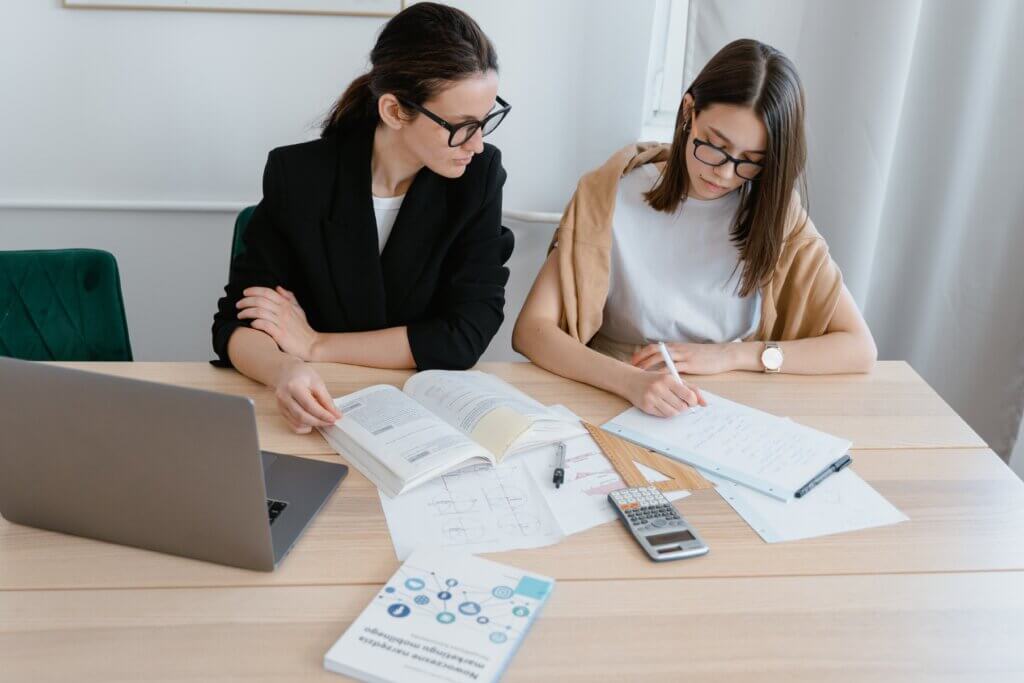
As an academician, terms such as citations, references and bibliography might be a part of almost every work-related conversation in your daily life. However, many researchers, especially during the early stages of their academic career, may find it hard to differentiate between citations, references and bibliography in research papers and often find it confusing to implement their usage. If you are amongst them, this article will provide you with some respite. Let us start by first understanding the individual terms better.
Citation in research papers: A citation appears in the main text of the paper. It is a way of giving credit to the information that you have specifically mentioned in your research paper by leading the reader to the original source of information. You will need to use citation in research papers whenever you are using information to elaborate a particular concept in the paper, either in the introduction or discussion sections or as a way to support your research findings in the results section.
Reference in research papers: A reference is a detailed description of the source of information that you want to give credit to via a citation. The references in research papers are usually in the form of a list at the end of the paper. The essential difference between citations and references is that citations lead a reader to the source of information, while references provide the reader with detailed information regarding that particular source.
Bibliography in research papers:
A bibliography in research paper is a list of sources that appears at the end of a research paper or an article, and contains information that may or may not be directly mentioned in the research paper. The difference between reference and bibliography in research is that an individual source in the list of references can be linked to an in-text citation, while an individual source in the bibliography may not necessarily be linked to an in-text citation.
It’s understandable how these terms may often be used interchangeably as they are serve the same purpose – namely to give intellectual and creative credit to an original idea that is elaborated in depth in a research paper. One of the easiest ways to understand when to use an in-text citation in research papers, is to check whether the information is an ongoing work of research or if it has been proven to be a ‘fact’ through reproducibility. If the information is a proven fact, you need not specifically add the original source to the list of references but can instead choose to mention it in your bibliography. For instance, if you use a statement such as “The effects of global warming and climate changes on the deterioration of environment have been described in depth”, you need not use an in-text citation, but can choose to mention key sources in the bibliography section. An example of a citation in a research paper would be if you intend to elaborate on the impact of climate change in a particular population and/or a specific geographical location. In this case, you will need to add an in-text citation and mention the correct source in the list of references.

Citations | References | Bibliography | |
Purpose | To lead a reader toward a source of information included in the text | To elaborate on of a particular source of information cited in the research paper | To provide a list of all relevant sources of information on the research topic
|
Placement | In the main text | At the end of the text; necessarily linked to an in-text citation | At the end of the text; not necessarily linked to an in-text citation
|
Information | Minimal; denoting only the essential components of the source, such as numbering, names of the first and last authors, etc.
| Descriptive; gives complete details about a particular source that can be used to find and read the original paper if needed | Descriptive; gives all the information regarding a particular source for those who want to refer to it |
Now that you have understood the basic similarities and differences in these terms, you should also know that every journal follows a particular style and format for these elements. So when working out how to write citations and add references in research papers, be mindful of using the preferred style of your target journal before you submit your research document.
R Discovery is a literature search and research reading platform that accelerates your research discovery journey by keeping you updated on the latest, most relevant scholarly content. With 250M+ research articles sourced from trusted aggregators like CrossRef, Unpaywall, PubMed, PubMed Central, Open Alex and top publishing houses like Springer Nature, JAMA, IOP, Taylor & Francis, NEJM, BMJ, Karger, SAGE, Emerald Publishing and more, R Discovery puts a world of research at your fingertips.
Try R Discovery Prime FREE for 1 week or upgrade at just US$72 a year to access premium features that let you listen to research on the go, read in your language, collaborate with peers, auto sync with reference managers, and much more. Choose a simpler, smarter way to find and read research – Download the app and start your free 7-day trial today !
Related Posts

Research in Shorts: R Discovery’s New Feature Helps Academics Assess Relevant Papers in 2mins

How Does R Discovery’s Interplatform Capability Enhance Research Accessibility
How to Write a Bibliography for a Research Paper
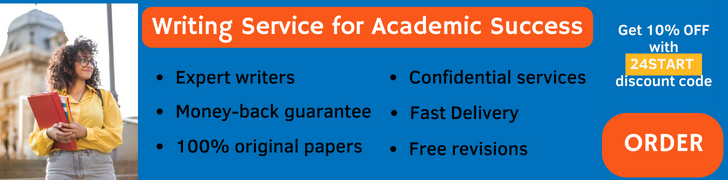
Do not try to “wow” your instructor with a long bibliography when your instructor requests only a works cited page. It is tempting, after doing a lot of work to research a paper, to try to include summaries on each source as you write your paper so that your instructor appreciates how much work you did. That is a trap you want to avoid. MLA style, the one that is most commonly followed in high schools and university writing courses, dictates that you include only the works you actually cited in your paper—not all those that you used.
Academic Writing, Editing, Proofreading, And Problem Solving Services
Get 10% off with 24start discount code, assembling bibliographies and works cited.
- If your assignment calls for a bibliography, list all the sources you consulted in your research.
- If your assignment calls for a works cited or references page, include only the sources you quote, summarize, paraphrase, or mention in your paper.
- If your works cited page includes a source that you did not cite in your paper, delete it.
- All in-text citations that you used at the end of quotations, summaries, and paraphrases to credit others for their ideas,words, and work must be accompanied by a cited reference in the bibliography or works cited. These references must include specific information about the source so that your readers can identify precisely where the information came from.The citation entries on a works cited page typically include the author’s name, the name of the article, the name of the publication, the name of the publisher (for books), where it was published (for books), and when it was published.
The good news is that you do not have to memorize all the many ways the works cited entries should be written. Numerous helpful style guides are available to show you the information that should be included, in what order it should appear, and how to format it. The format often differs according to the style guide you are using. The Modern Language Association (MLA) follows a particular style that is a bit different from APA (American Psychological Association) style, and both are somewhat different from the Chicago Manual of Style (CMS). Always ask your teacher which style you should use.
A bibliography usually appears at the end of a paper on its own separate page. All bibliography entries—books, periodicals, Web sites, and nontext sources such radio broadcasts—are listed together in alphabetical order. Books and articles are alphabetized by the author’s last name.
Most teachers suggest that you follow a standard style for listing different types of sources. If your teacher asks you to use a different form, however, follow his or her instructions. Take pride in your bibliography. It represents some of the most important work you’ve done for your research paper—and using proper form shows that you are a serious and careful researcher.
Bibliography Entry for a Book
A bibliography entry for a book begins with the author’s name, which is written in this order: last name, comma, first name, period. After the author’s name comes the title of the book. If you are handwriting your bibliography, underline each title. If you are working on a computer, put the book title in italicized type. Be sure to capitalize the words in the title correctly, exactly as they are written in the book itself. Following the title is the city where the book was published, followed by a colon, the name of the publisher, a comma, the date published, and a period. Here is an example:
Format : Author’s last name, first name. Book Title. Place of publication: publisher, date of publication.
- A book with one author : Hartz, Paula. Abortion: A Doctor’s Perspective, a Woman’s Dilemma . New York: Donald I. Fine, Inc., 1992.
- A book with two or more authors : Landis, Jean M. and Rita J. Simon. Intelligence: Nature or Nurture? New York: HarperCollins, 1998.
Bibliography Entry for a Periodical
A bibliography entry for a periodical differs slightly in form from a bibliography entry for a book. For a magazine article, start with the author’s last name first, followed by a comma, then the first name and a period. Next, write the title of the article in quotation marks, and include a period (or other closing punctuation) inside the closing quotation mark. The title of the magazine is next, underlined or in italic type, depending on whether you are handwriting or using a computer, followed by a period. The date and year, followed by a colon and the pages on which the article appeared, come last. Here is an example:
Format: Author’s last name, first name. “Title of the Article.” Magazine. Month and year of publication: page numbers.
- Article in a monthly magazine : Crowley, J.E.,T.E. Levitan and R.P. Quinn.“Seven Deadly Half-Truths About Women.” Psychology Today March 1978: 94–106.
- Article in a weekly magazine : Schwartz, Felice N.“Management,Women, and the New Facts of Life.” Newsweek 20 July 2006: 21–22.
- Signed newspaper article : Ferraro, Susan. “In-law and Order: Finding Relative Calm.” The Daily News 30 June 1998: 73.
- Unsigned newspaper article : “Beanie Babies May Be a Rotten Nest Egg.” Chicago Tribune 21 June 2004: 12.
Bibliography Entry for a Web Site
For sources such as Web sites include the information a reader needs to find the source or to know where and when you found it. Always begin with the last name of the author, broadcaster, person you interviewed, and so on. Here is an example of a bibliography for a Web site:
Format : Author.“Document Title.” Publication or Web site title. Date of publication. Date of access.
Example : Dodman, Dr. Nicholas. “Dog-Human Communication.” Pet Place . 10 November 2006. 23 January 2014 < http://www.petplace.com/dogs/dog-human-communication-2/page1.aspx >
After completing the bibliography you can breathe a huge sigh of relief and pat yourself on the back. You probably plan to turn in your work in printed or handwritten form, but you also may be making an oral presentation. However you plan to present your paper, do your best to show it in its best light. You’ve put a great deal of work and thought into this assignment, so you want your paper to look and sound its best. You’ve completed your research paper!
Back to How To Write A Research Paper .
ORDER HIGH QUALITY CUSTOM PAPER


Research Process: Bibliographic Information
- Selecting a Topic
- Background Information
- Narrowing the Topic
- Library Terms
- Generating Keywords
- Boolean Operators
- Search Engine Strategies
- Google Searching
- Basic Internet Terms
- Research & The Web
- Search Engines
- Evaluating Books
- Evaluating Articles
- Evaluating Websites
Bibliographic Information
- Off Campus Access
- Periodical Locator
What is a bibliography?
A bibliography is a list of works on a subject or by an author that were used or consulted to write a research paper, book or article. It can also be referred to as a list of works cited. It is usually found at the end of a book, article or research paper.
Gathering Information
Regardless of what citation style is being used, there are key pieces of information that need to be collected in order to create the citation.
For books and/or journals:
- Author name
- Title of publication
- Article title (if using a journal)
- Date of publication
- Place of publication
- Volume number of a journal, magazine or encyclopedia
- Page number(s)
For websites:
- Author and/or editor name
- Title of the website
- Company or organization that owns or posts to the website
- URL (website address)
- Date of access
This section provides two examples of the most common cited sources: a print book and an online journal retrieved from a research database.
Book - Print
For print books, bibliographic information can be found on the TITLE PAGE . This page has the complete title of the book, author(s) and publication information.
The publisher information will vary according to the publisher - sometimes this page will include the name of the publisher, the place of publication and the date.
For this example : Book title: HTML, XHTML, and CSS Bible Author: Steven M. Schafer Publisher: Wiley Publications, Inc.
If you cannot find the place or date of publication on the title page, refer to the COPYRIGHT PAGE for this information. The copyright page is the page behind the title page, usually written in a small font, it carries the copyright notice, edition information, publication information, printing history, cataloging data, and the ISBN number.
For this example : Place of publication: Indianapolis, IN Date of publication: 2010
Article - Academic OneFile Database
In the article view:
Bibliographic information can be found under the article title, at the top of the page. The information provided in this area is NOT formatted according to any style.
Citations can also be found at the bottom of the page; in an area titled SOURCE CITATION . The database does not specify which style is used in creating this citation, so be sure to double check it against the style rules for accuracy.
Article - ProQuest Database
Bibliographic information can be found under the article title, at the top of the page. The information provided in this area is NOT formatted according to any style.
Bibliographic information can also be found at the bottom of the page; in an area titled INDEXING . (Not all the information provided in this area is necessary for creating citations, refer to the rules of the style being used for what information is needed.)
Other databases have similar formats - look for bibliographic information under the article titles and below the article body, towards the bottom of the page.
- << Previous: Plagiarism
- Next: Research Databases >>
- Last Updated: Jun 26, 2024 2:47 PM
- URL: https://pgcc.libguides.com/researchprocess
Bibliographic analysis on research publications using authors, categorical labels and the citation network
- Published: 11 March 2016
- Volume 103 , pages 185–213, ( 2016 )
Cite this article
- Kar Wai Lim 1 &
- Wray Buntine 2
8921 Accesses
12 Citations
2 Altmetric
Explore all metrics
Bibliographic analysis considers the author’s research areas, the citation network and the paper content among other things. In this paper, we combine these three in a topic model that produces a bibliographic model of authors, topics and documents, using a non-parametric extension of a combination of the Poisson mixed-topic link model and the author-topic model. This gives rise to the Citation Network Topic Model (CNTM). We propose a novel and efficient inference algorithm for the CNTM to explore subsets of research publications from CiteSeer \(^{\mathrm{X}}\) . The publication datasets are organised into three corpora, totalling to about 168k publications with about 62k authors. The queried datasets are made available online. In three publicly available corpora in addition to the queried datasets, our proposed model demonstrates an improved performance in both model fitting and document clustering, compared to several baselines. Moreover, our model allows extraction of additional useful knowledge from the corpora, such as the visualisation of the author-topics network. Additionally, we propose a simple method to incorporate supervision into topic modelling to achieve further improvement on the clustering task.
Similar content being viewed by others
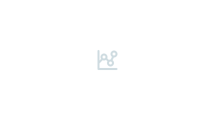
Topic discovery and evolution in scientific literature based on content and citations
Topic Modeling: Measuring Scholarly Impact Using a Topical Lens
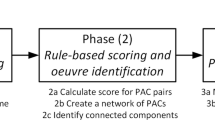
Collecting large-scale publication data at the level of individual researchers: a practical proposal for author name disambiguation
Explore related subjects.
- Artificial Intelligence
Avoid common mistakes on your manuscript.
1 Introduction
Models of bibliographic data need to consider many kinds of information. Articles are usually accompanied by metadata such as authors, publication data, categories and time. Cited papers can also be available. When authors’ topic preferences are modelled, we need to associate the document topic information somehow with the authors’. Jointly modelling text data with citation network information can be challenging for topic models, and the problem is confounded when also modelling author-topic relationships.
In this paper, we propose a topic model to jointly model authors’ topic preferences, text content Footnote 1 and the citation network. The model is a non-parametric extension of previous models discussed in Sect. 2 . Using simple assumptions and approximations, we derive a novel algorithm that allows the probability vectors in the model to be integrated out. This yields a Markov chain Monte Carlo (MCMC) inference via discrete sampling.
As an extension of our previous work (Lim and Buntine 2014 ), we propose a supervised approach to improve document clustering, by making use of categorical information that is available. Our method allows the level of supervision to be adjusted through a variable, giving us a model with no supervision, semi-supervised or fully supervised. Additionally, we present a more extensive qualitative analysis of the learned topic models, and display a visualisation snapshot of the learned author-topics network. We also perform additional diagnostic tests to assess our proposed topic model. For example, we study the convergence of the proposed learning algorithm and report on the computation complexity of the algorithm.
In the next section, we discuss the related work. Sects. 3 , 4 and 5 detail our topic model and its inference algorithm. We describe the datasets in Sect. 6 and report on experiments in Sect. 7 . Applying our model on research publication data, we demonstrate the model’s improved performance, on both model fitting and a clustering task, compared to several baselines. Additionally, in Sect. 8 , we qualitatively analyse the inference results produced by our model. We find that the learned topics have high comprehensibility. Additionally, we present a visualisation snapshot of the learned topic models. Finally, we perform diagnostic assessment of the topic model in Sect. 9 and conclude the paper in Sect. 10 .
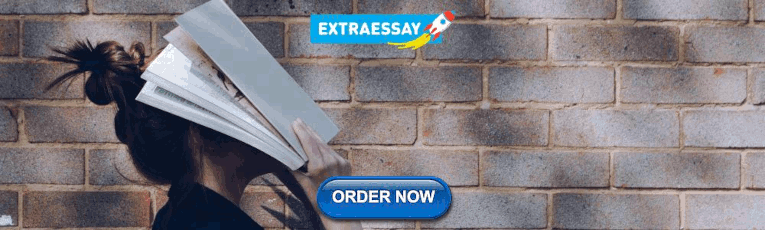
2 Related work
Latent Dirichlet Allocation (LDA) (Blei et al. 2003 ) is the simplest Bayesian topic model used in modelling text, which also allows easy learning of the model. Teh and Jordan ( 2010 ) proposed the Hierarchical Dirichlet process (HDP) LDA, which utilises the Dirichlet process (DP) as a non-parametric prior which allows a non-symmetric, arbitrary dimensional topic prior to be used. Furthermore, one can replace the Dirichlet prior on the word vectors with the Pitman–Yor Process (PYP, also known as the two-parameter Poisson Dirichlet process) (Teh 2006b ), which models the power-law of word frequency distributions in natural language (Goldwater et al. 2011 ), yielding significant improvement (Sato and Nakagawa 2010 ).
Variants of LDA allow incorporating more aspects of a particular task and here we consider authorship and citation information. The author-topic model (ATM) (Rosen-Zvi et al. 2004 ) uses the authorship information to restrict topic options based on author. Some recent work jointly models the document citation network and text content. This includes the relational topic model (Chang and Blei 2010 ), the Poisson mixed-topic link model (PMTLM) (Zhu et al. 2013 ) and Link-PLSA-LDA (Nallapati et al. 2008 ). An extensive review of these models can be found in Zhu et al. ( 2013 ). The Citation Author Topic (CAT) model (Tu et al. 2010 ) models the author-author network on publications based on citations using an extension of the ATM. Note that our work is different to CAT in that we model the author-document-citation network instead of author-author network.
The Topic-Link LDA (Liu et al. 2009 ) jointly models author and text by using the distance between the document and author topic vectors. Similarly the Twitter-Network topic model (Lim et al. 2013 ) models the author network Footnote 2 based on author topic distributions, but using a Gaussian process to model the network. Note that our work considers the author-document-citation of Liu et al. ( 2009 ). We use the PMTLM of Zhu et al. ( 2013 ) to model the network, which lets one integrate PYP hierarchies with the PMTLM using efficient MCMC sampling.
There is also existing work on analysing the degree of authors’ influence. On publication data, Kataria et al. ( 2011 ) and Mimno and McCallum ( 2007 ) analyse influential authors with topic models, while Weng et al. ( 2010 ), Tang et al. ( 2009 ), and Liu et al. ( 2010 ) use topic models to analyse users’ influence on social media.
3 Supervised Citation Network Topic Model
In our previous work (Lim and Buntine 2014 ), we proposed the Citation Network Topic Model (CNTM) that jointly models the text , authors , and the citation network of research publications (documents). The CNTM allows us to both model the authors and text better by exploiting the correlation between the authors and their research topics. However, the benefit of the above modelling is not realised when the author information is simply missing from the data. This could be due to error in data collection (e.g. metadata not properly formatted), or even simply that the author information is lost during preprocessing.
In this section, we propose an extension of the CNTM that remedies the above issue, by making use of additional metadata that is available. For example, the metadata could be the research areas or keywords associated with the publications, which are usually provided by the authors during the publication submission. However, this information might not always be reliable as it is not standardised across different publishers or conferences. In this paper, rather than using the mentioned metadata, we will instead incorporate the categorical labels that were previously used as ground truth for evaluation. As such, our extension gives rise to a supervised model, which we will call the Supervised Citation Network Topic Model (SCNTM).
We first describe the topic model part of SCNTM for which the citations are not considered, it will be used for comparison later in Sect. 7 . We then complete the SCNTM with the discussion on its network component. The full graphical model for SCNTM is displayed in Fig. 1 .
To clarify the notations used in this paper, variables that are without subscript represent a collection of variables of the same notation . For instance, \(w_d\) represents all the words in document d , that is, \(w_d = \{w_{d1}, \dots , w_{dN_d}\}\) where \(N_d\) is the number of words in document d ; and w represents all words in a corpus, \(w=\{w_1, \dots , w_D\}\) , where D is the number of documents.
Graphical model for SCNTM. The box on the top left with \(D^2\) entries is the citation network on documents represented as a Boolean matrix. The remainder is a non-parametric hierarchical PYP topic model where the labelled categories and authors are captured by the topic vectors \(\nu \) . The topic vectors \(\nu \) influence the D documents’ topic vectors \(\theta '\) and \(\theta \) based on the observed authors a or categories e . The latent topics and associated words are represented by the variables z and w . The K topics, shown in the top right , have bursty modelling following Buntine and Mishra ( 2014 )
3.1 Hierarchical Pitman–Yor topic model
The SCNTM uses both the Griffiths–Engen–McCloskey (GEM) distribution (Pitman 1996 ) and the Pitman–Yor process (PYP) (Teh 2006b ) to generate probability vectors. Both the GEM distribution and the PYP are parameterised by a discount parameter \(\alpha \) and a concentration parameter \(\beta \) . The PYP is additionally parameterised by a base distribution H , which is also the mean of the PYP when it can be represented by a probability vector. Note that the base distribution can also be a PYP. This gives rise to the hierarchical Pitman–Yor process (HPYP).
In modelling authorship, the SCNTM modifies the approach of the author-topic model (Rosen-Zvi et al. 2004 ) which assumes that the words in a publication are equally attributed to the different authors. This is not reflected in practice since publications are often written more by the first author, excepting when the order is alphabetical. Thus, we assume that the first author is dominant and attribute all the words in a publication to the first author. Although, we could model the contribution of each author on a publication by, say, using a Dirichlet distribution, we found that considering only the first author gives a simpler learning algorithm and cleaner results.
The generative process of the topic model component of the SCNTM is as follows. We first sample a root topic distribution \(\mu \) with a GEM distribution to act as a base distribution for the author-topic distributions \(\nu _a\) for each author a , and also for the category-topic distributions \(\nu _e\) for each category e :
Here, \(\mathcal {A}\) represents the set of all authors while \(\mathcal {E}\) denotes the set of all categorical labels in the text corpus. Note we have used the same symbol ( \(\nu \) ) for both the author-topic distributions and the category-topic distributions.
We introduce a parameter \(\eta \) called the author threshold which controls the level of supervision used by SCNTM. We say an author a is significant if the author has produced more than or equal to \(\eta \) publications, i.e.
Here, \(a_d\) represents the author for document d , and \(I(\triangle )\) is the indicator function that evaluates to 1 if \(\triangle \) is true, else 0.
Next, for each document d in a publication collection of size D , we sample the document-topic prior \(\theta '_d\) from \(\nu _{a_d}\) or \(\nu _{e_d}\) depending on whether the author \(a_d\) for the document is significant:
where \(e_d\) is the categorical label associated with document d . For the sake of notational simplicity, we introduce a variable b to capture both the author and the category. We let b takes the value of \(1, \dots , A\) for each author in \(\mathcal {A}\) , and let b takes the value of \((A+1), \dots , B\) for the categories in \(\mathcal {E}\) . Note that \(B = |\mathcal {A}| + |\mathcal {E}|\) . Thus, we can also write the distribution of \(\theta '_d\) as
where \(b = a_d\) if \(\mathrm {significance}(a_d) = 1\) , else \(b = e_d\) .
By modelling this way, we are able to handle missing authors and incorporate supervision into the SCNTM. For example, choosing \(\eta = 1\) allows us to make use of the categorical information for documents that have no valid author. Alternatively, we could select a higher \(\eta \) , this smooths out the document-topic distributions for documents that are written by authors who have authored only a small number of publications. This treatment leads to a better clustering result as these authors are usually not discriminative enough for prediction. On the extreme, we can set \(\eta = \infty \) to achieve full supervision. We note that the SCNTM reverts to the CNTM when \(\eta = 0\) , in this case the model is not supervised.
We then sample the document-topic distribution \(\theta _d\) given \(\theta '_d\) :
Note that instead of modelling a single document-topic distribution, we model a document-topic hierarchy with \(\theta '\) and \(\theta \) . The primed \(\theta '\) represents the topics of the document in the context of the citation network. The unprimed \(\theta \) represents the topics of the text, naturally related to \(\theta '\) but not the same. Such modelling gives citation information a higher impact to take into account the relatively low amount of citations compared to the text. The technical details on the effect of such modelling is presented in Sect. 9.2 .
For the vocabulary side, we generate a background word distribution \(\gamma \) given \(H^\gamma \) , a discrete uniform vector of length \(|\mathcal {V}|\) , i.e. \(H^\gamma = (\dots , \frac{1}{|\mathcal {V}|}, \dots )\) . \(\mathcal {V}\) is the set of distinct word tokens observed in a corpus. Then, we sample a topic-word distribution \(\phi _k\) for each topic k , with \(\gamma \) as the base distribution:
Modelling word burstiness (Buntine and Mishra 2014 ) is important since words in a document are likely to repeat in the document. The same applies to publication abstract, as shown in Sect. 6 . To address this property, we make the topics bursty so each document only focuses on a subset of words in the topic. This is achieved by defining the document-specific topic-word distribution \(\phi '_{dk}\) for each topic k in document d as:
Finally, for each word \(w_{dn}\) in document d , we sample the corresponding topic assignment \(z_{dn}\) from the document-topic distribution \(\theta _d\) ; while the word \(w_{dn}\) is sampled from the topic-word distribution \(\phi '_d\) given \(z_{dn}\) :
Note that w includes words from the publications’ title and abstract, but not the full article. This is because title and abstract provide a good summary of a publication’s topics and thus more suited for topic modelling, while the full article contains too much technical detail that might not be too relevant.
In the next section, we describe the modelling of the citation network accompanying a publication collections. This completes the SCNTM.
3.2 Citation Network Poisson Model
To model the citation network between publications, we assume that the citations are generated conditioned on the topic distributions \(\theta '\) of the publications. Our approach is motivated by the degree-corrected variant of PMTLM (Zhu et al. 2013 ). Denoting \(x_{ij}\) as the number of times document i citing document j , we model \(x_{ij}\) with a Poisson distribution with mean parameter \(\lambda _{ij}\) :
Here, \(\lambda _i^+\) is the propensity of document i to cite and \(\lambda _j^-\) represents the popularity of cited document j , while \(\lambda ^T_k\) scales the k -th topic, effectively penalising common topics and strengthen rare topics. Hence, a citation from document i to document j is more likely when these documents are having relevant topics. Due to the limitation of the data, the \(x_{ij}\) can only be 0 or 1, i.e. it is a Boolean variable. Nevertheless, the Poisson distribution is used instead of a Bernoulli distribution because it leads to dramatically reduced complexity in analysis (Zhu et al. 2013 ). Note that the Poisson distribution is similar to the Bernoulli distribution when the mean parameter is small. We present a list of variables associated with the SCNTM in Table 1 .
4 Model representation and posterior likelihood
Before presenting the posterior used to develop the MCMC sampler, we briefly review handling of the hierarchical PYP models in Sect. 4.1 . We cannot provide an adequately detailed review in this paper, thus we present the main ideas.
4.1 Modelling with hierarchical PYPs
The key to efficient sampling with PYPs is to marginalise out the probability vectors (e.g. topic distributions) in the model and record various associated counts instead, thus yielding a collapsed sampler. While a common approach here is to use the hierarchical Chinese Restaurant Process (CRP) of Teh and Jordan ( 2010 ), we use another representation that requires no dynamic memory and has better inference efficiency (Chen et al. 2011 ).
We denote \(f^*(\mathcal {N})\) as the marginalised likelihood associated with the probability vector \(\mathcal {N}\) . Since the vector is marginalised out, the marginalised likelihood is in terms of—using the CRP terminology—the customer counts \(c^\mathcal {N} = (\dots , c_k^\mathcal {N}, \dots )\) and the table counts \(t^\mathcal {N} = (\dots , t_k^\mathcal {N}, \dots )\) . The customer count \(c_k^\mathcal {N}\) corresponds to the number of data points (e.g. words) assigned to group k (e.g. topic) for variable \(\mathcal {N}\) . Here, the table counts \(t^\mathcal {N}\) represent the subset of \(c^\mathcal {N}\) that gets passed up the hierarchy (as customers for the parent probability vector of \(\mathcal {N}\) ). Thus \(t_k^\mathcal {N} \le c_k^\mathcal {N}\) , and \(t_k^\mathcal {N}=0\) if and only if \(c_k^\mathcal {N}=0\) since the counts are non-negative. We also denote \(C^\mathcal {N} = \sum _k c_k^\mathcal {N}\) as the total customer counts for node \(\mathcal {N}\) , and similarly, \({T}^\mathcal {N} = \sum _k t_k^\mathcal {N}\) is the total table counts. The marginalised likelihood \(f^*(\mathcal {N})\) , in terms of \(c^\mathcal {N}\) and \(t^\mathcal {N}\) , is given as
\(S^x_{y,\alpha }\) is the generalised Stirling number that is easily tabulated; both \((x)_C\) and \((x|y)_C\) denote the Pochhammer symbol (rising factorial), see Buntine and Hutter ( 2012 ) for details. Note the GEM distribution behaves like a PYP in which the table count \(t_k^\mathcal {N}\) is always 1 for non-zero \(c_k^\mathcal {N}\) .
The innovation of Chen et al. ( 2011 ) was to notice that sampling with Eq. 14 directly led to poor performance. The problem was that sampling an assignment to a latent variable, say moving a customer from group k to \(k'\) (so \(c_k^\mathcal {N}\) decreases by 1 and \(c_{k'}^\mathcal {N}\) increases by 1), the potential effect on \(t_k^\mathcal {N}\) and \(t_{k'}^\mathcal {N}\) could not immediately be measured. Whereas, the hierarchical CRP automatically included table configurations in its sampling process and thus included the influence of the hierarchy in the sampling. Thus sampling directly with Eq. 14 lead to comparatively poor mixing. As a solution, Chen et al. ( 2011 ) develop a collapsed version of the hierarchical CRP following the well known practice of Rao-Blackwellisation of sampling schemes (Casella and Robert 1996 ), which, while not being as fast per step, it has two distinct advantages, (1) it requires no dynamic memory and (2) the sampling has significantly lower variance so converges much faster. This has empirically been shown to lead to better mixing of the samplers (Chen et al. 2011 ) and has been confirmed on different complex topic models (Buntine and Mishra 2014 ).
The technique for collapsing the hierarchical CRP uses Eq. 14 but the counts ( \(c^\mathcal {N},t^\mathcal {N}\) ) are now derived variables. They are derived from Boolean variables associated with each data point. The technique comprises the following conceptual steps: (1) add Boolean indicators \(u_{dn}\) to the data \((z_{dn},w_{dn})\) from which the counts \(c^\mathcal {N}\) and \(t^\mathcal {N}\) can be derived, (2) modify the marginalised posterior accordingly, and (3) derive a sampler for the model.
4.1.1 Adding Boolean indicators
We first consider \(c_k^{\theta _d}\) , which has a “+1” contributed to for every \(z_{dn}=k\) in document d , hence \(c_k^{\theta _d}=\sum _n I(z_{dn}=k)\) . We now introduce a new Bernoulli indicator variable \(u^{\theta _d}_{dn}\) associated with \(z_{dn}\) , which is “on” (or 1) when the data \(z_{dn}\) also contributed a “+1” to \(t^{\theta _d}_k\) . Note that \(t_k^{\theta _d} \le c_k^{\theta _d}\) , so every data contributing a “+1” to \(c_k^{\theta _d}\) may or may not contribute a “+1” to \(t_k^{\theta _d}\) . The result is that one derives \(t_k^{\theta _d}=\sum _n I(z_{dn}=k) \, I(u^{\theta _d}_{dn}=1)\) .
Now consider the parent of \(\theta _d\) , which is \(\theta '_d\) . Its customer count is derived as \(c_k^{\theta '_d}=t_k^{\theta _d}\) . Its table count \(t_k^{\theta '_d}\) can now be treated similarly. Those data \(z_{dn}\) that contribute a “+1” to \(t_k^{\theta _d}\) (and thus \(c_k^{\theta '_d}\) ) have a new Bernoulli indicator variable \(u^{\theta '_d}_{dn}\) , which is used to derive \(t_k^{\theta '_d}=\sum _n I(z_{dn}=k) \, I(u^{\theta '_d}_{dn}=1)\) , similar as before. Note that if \(u^{\theta '_d}_{dn}=1\) then necessarily \(u^{\theta _d}_{dn}=1\) .
Similarly, one can define Boolean indicators for \(\mu , \nu _b, \phi ', \phi \) , and \(\gamma \) to have a full suite from which all the counts \(c^\mathcal {N}\) and \(t^\mathcal {N}\) are now derived. We denote \(u_{dn} = \{ u^{\theta _d}_{dn}, u^{\theta '_d}_{dn}, u^{\nu _b}_{dn}, u^{\mu }_{dn}, u^{\phi '_d}_{dn}, u^{\phi _d}_{dn}, u^\gamma _{dn} \}\) as the collection of the Boolean indicators for data ( \(z_{dn}, w_{dn}\) ).
4.1.2 Probability of Boolean indicators
By symmetry, if there are \(t_k^\mathcal {N}\) Boolean indicators “on” (out of \(c_k^\mathcal {N}\) ), we are indifferent as to which is on. Thus the indicator variable \(u^\mathcal {N}_{dn}\) is not stored, that is, we simply “forget” who contributed a table count and re-sample \(u^\mathcal {N}_{dn}\) as needed:
Moreover, this means that the marginalised likelihood \(f^*(\mathcal {N})\) of Eq. 14 is extended to include the probability of \(u^\mathcal {N}\) , which is written in terms of \(c^\mathcal {N}, t^\mathcal {N}\) and \(u^\mathcal {N}\) as:
4.2 Likelihood for the hierarchical PYP topic model
We use bold face capital letters to denote the set of all relevant lower case variables. For example, \(\mathbf {Z} = \{z_{11},\dots ,z_{DN_D}\}\) denotes the set of all topic assignments. Variables \(\mathbf {W}, \mathbf {T}, \mathbf {C}\) and \(\mathbf {U}\) are similarly defined, that is, they denote the set of all words, table counts, customer counts, and Boolean indicators respectively. Additionally, we denote \(\mathbf {\zeta }\) as the set of all hyperparameters (such as the \(\alpha \) ’s). With the probability vectors replaced by the counts, the likelihood of the topic model can be written—in terms of \(f(\cdot )\) as given in Eq. 16 —as \(p(\mathbf {Z}, \mathbf {W}, \mathbf {T}, \mathbf {C}, \mathbf {U} \,|\, \mathbf {\zeta }) \propto \)
Note that the last term in Eq. 17 corresponds to the parent probability vector of \(\gamma \) (see Sect. 3.1 ), and v indexes the unique word tokens in vocabulary set \(\mathcal {V}\) . Note that the extra terms for \(\mathbf {U}\) are simply derived using Eq. 16 and not stored in the model. So in the discussions below we will usually represent \(\mathbf {U}\) implicitly by \(\mathbf {T}\) and \(\mathbf {C}\) , and introduce the \(\mathbf {U}\) when explicitly needed.
Note that even though the probability vectors are integrated out and not explicitly stored, they can easily be estimated from the associated counts. The probability vector \(\mathcal {N}\) can be estimated from its posterior mean given the counts and parent probability vector \(\mathcal {P}\) :
4.3 Likelihood for the Citation Network Poisson Model
For the citation network, the Poisson likelihood for each \(x_{ij}\) is given as
Note that the term \(x_{ij}!\) is dropped in Eq. 19 due to the limitation of the data that \(x_{ij} \in \{0, 1\}\) , thus \(x_{ij}!\) is evaluated to 1. With conditional independence of \(x_{ij}\) , the joint likelihood for the whole citation network \(\mathbf {X} = \{x_{11}, \dots , x_{DD}\}\) can be written as \(p(\mathbf {X} \,|\, \lambda , \theta ') =\)
where \(g^+_i\) is the number of citations for publication \(i, g^+_i = \sum _j x_{ij}\) , and \(g^-_i\) is the number of times publication i being cited, \(g^-_i = \sum _j x_{ji}\) . We also make a simplifying assumption that \(x_{ii} = 1\) for all documents i , that is, all publications are treated as self-cited. This assumption is important since defining \(x_{ii}\) allows us to rewrite the joint likelihood into Eq. 20 , which leads to a cleaner learning algorithm that utilises an efficient caching. Note that if we do not define \(x_{ii}\) , we have to explicitly consider the case when \(i=j\) in Eq. 20 which results in messier summation and products.
Note the likelihood in Eq. 20 contains the document-topic distribution \(\theta '\) in vector form. This is problematic as performing inference with the likelihood requires the probability vectors \(\theta ', \nu \) and \(\mu \) to be stored explicitly (instead of counts as discussed in Sect. 4.1 ). To overcome this issue, we propose a novel representation that allows the probability vectors to remain integrated out. Such representation also leads to an efficient sampling algorithm for the citation network, as we will see in Sect. 5 .
We introduce an auxiliary variable \(y_{ij}\) , named the citing topic , to denote the topic that prompts publication i to cite publication j . To illustrate, for a biology publication that cites a machine learning publication for the learning technique, the citing topic would be ‘machine learning’ instead of ‘biology’. From Eq. 13 , we model the citing topic \(y_{ij}\) as jointly Poisson with \(x_{ij}\) :
Incorporating \(\mathbf {Y}\) , the set of all \(y_{ij}\) , we rewrite the citation network likelihood as \(p(\mathbf {X},\mathbf {Y}|\lambda , \theta ') \propto \)
where \(h_{ik}=\sum _j x_{ij}I(y_{ij}=k)+\sum _j x_{ji}I(y_{ji}=k)\) is the number of connections publication i made due to topic k .
To integrate out \(\theta '\) , we note the term \({\theta '_{ik}}^{h_{ik}}\) appears like a multinomial likelihood, so we absorb them into the likelihood for \(p(\mathbf {Z}, \mathbf {W}, \mathbf {T}, \mathbf {C}, \mathbf {U} \,|\, \mathbf {\zeta })\) where they correspond to additional counts for \(c^{\theta '_i}\) , with \(h_{ik}\) added to \(c^{\theta '_i}_k\) . To disambiguate the source of the counts, we will refer to these customer counts contributed by \(x_{ij}\) as network counts , and denote the augmented counts ( \(\mathbf {C}\) plus network counts) as \(\mathbf {C^+}\) . For the exponential term, we use the delta method (Oehlert 1992 ) to approximate \(\int q(\theta )\,\exp (-g(\theta ))\,\mathrm {d}\theta \approx \exp (-g({\hat{\theta }})) \int q(\theta )\,\mathrm {d}\theta \) , where \({\hat{\theta }}\) is the expected value according to a distribution proportional to \(q(\theta )\) . This approximation is reasonable as long as the terms in the exponential are small (see “Appendix 1”). The approximate full posterior of SCNTM can then be written as \(p(\mathbf {Z}, \mathbf {W}, \mathbf {T}, \mathbf {C^+}, \mathbf {U}, \mathbf {X}, \mathbf {Y} \,|\, \lambda ,\mathbf {\zeta }) \approx \)
where \(g_k^T = \frac{1}{2}\sum _i h_{ik}\) . We note that \(p(\mathbf {Z}, \mathbf {W}, \mathbf {T}, \mathbf {C^+}, \mathbf {U} \,|\, \mathbf {\zeta })\) is the same as Eq. 17 but now with \(\mathbf {C^+}\) instead of \(\mathbf {C}\) .
In the next section, we demonstrate that our model representation gives rise to an intuitive sampling algorithm for learning the model. We also show how the Poisson model integrates into the topic modelling framework.
5 Inference techniques
Here, we derive the Markov chain Monte Carlo (MCMC) algorithms for learning the SCNTM. We first describe the sampler for the topic model and then for the citation network. The full inference procedure is performed by alternating between the two samplers. Finally, we outline the hyperparameter samplers that are used to estimate the hyperparameters automatically.
5.1 Sampling for the hierarchical PYP topic model
To sample the words’ topic \(\mathbf {Z}\) and the associated counts \(\mathbf {T}\) and \(\mathbf {C}\) in the SCNTM, we design a Metropolis–Hastings (MH) algorithm based on the collapsed Gibbs sampler designed for the PYP (Chen et al. 2011 ). The concept of the MH sampler is analogous to LDA, which consists of (1) decrementing the counts associated with a word, (2) sampling the respective new topic assignment for the word, and (3) incrementing the associated counts. However, our sampler is more complicated than LDA. In particular, we have to consider the indicators \(u^\mathcal {N}_{dn}\) described in Sect. 4.1 operating on the hierarchy of PYPs. Our MH sampler consists of two steps. First we sample the latent topic \(z_{dn}\) associated with the word \(w_{dn}\) . We then sample the customer counts \(\mathbf {C}\) and table counts \(\mathbf {T}\) .
The sampler proceeds by considering the latent variables associated with a given word \(w_{dn}\) . First, we decrement the counts associated with the word \(w_{dn}\) and the latent topic \(z_{dn}\) . This is achieved by sampling the suite of indicators \(u_{dn}\) according to Eq. 15 and decrementing the relevant customer counts and table counts. For example, we decrement \(c^{\theta _d}_{z_{dn}}\) by 1 if \(u^{\theta _d}_{dn} = 1\) . After decrementing, we apply a Gibbs sampler to sample a new topic \(z_{dn}\) from its conditional posterior distribution, given as \(p(z^\mathrm{new}_{dn} \,|\, \mathbf {Z}^{-dn}, \mathbf {W}, \mathbf {T}^{-dn}, \mathbf {C^+}^{-dn}, \mathbf {U}^{-dn}, \mathbf {\zeta }) = \)
Note that the joint distribution in Eq. 24 can be written as the ratio of the likelihood for the topic model (Eq. 17 ):
Here, the superscript \(\mathcal {O}^{-dn}\) indicates that the topic \(z_{dn}\) , indicators and the associated counts for word \(w_{dn}\) are not observed in the respective sets, i.e. the state after decrement. Additionally, we use the superscripts \(\mathcal {O}^\mathrm{new}\) and \(\mathcal {O}^\mathrm{old}\) to denote the proposed sample and the old value respectively. The modularised likelihood of Eq. 17 allows the conditional posterior (Eq. 24 ) to be computed easily, since it simplifies to ratios of likelihood \(f(\cdot )\) , which simplifies further since the counts differ by at most 1 during sampling. For instance, the ratio of the Pochhammer symbols, \((x|y)_{C+1} / (x|y)_C\) , simplifies to \(x+Cy\) , while the ratio of Stirling numbers, such as \(S^{y+1}_{x+1, \alpha }/S^{y}_{x, \alpha }\) , can be computed quickly via caching (Buntine and Hutter 2012 ).
Next, we proceed to sample the relevant customer counts and table counts given the new \(z_{dn} = k\) . We propose an MH algorithm for this. We define the proposal distribution for the new customer counts and table counts as
Here, the potential sample space for \(\mathbf {T}^\mathrm{new}\) and \(\mathbf {C}^\mathrm{new}\) are restricted to just \(t_k + i\) and \(c_k + i\) where i is either 0 or 1. Doing so allows us to avoid considering the exponentially many possibilities of \(\mathbf {T}\) and \(\mathbf {C}\) . The acceptance probability associated with the newly sampled \(\mathbf {T}^\mathrm{new}\) and \(\mathbf {C}^\mathrm{new}\) is
Thus we always accept the proposed sample. Footnote 3 Note that since \(\mu \) is GEM distributed, incrementing \(t^\mu _k\) is equivalent to sampling a new topic, i.e. the number of topics increases by 1.
5.2 Sampling for the citation network
For the citation network, we propose another MH algorithm. The MH algorithm can be summarised in three steps: (1) estimate the document topic prior \(\theta '\) , (2) propose a new citing topic \(y_{ij}\) , and (3) accept or reject the proposed \(y_{ij}\) following an MH scheme. Note that the MH algorithm is similar to the sampler for the topic model, where we decrement the counts, sample a new state and update the counts. Since all probability vectors are represented as counts, we do not need to deal with their vector form. Additionally, our MH algorithm is intuitive and simple to implement. Like the words in a document, each citation is assigned a topic, hence the words and citations can be thought as voting to determine a documents’ topic.
We describe our MH algorithm for the citation network as follows. First, for each document d , we estimate the expected document-topic prior \({\hat{\theta }}'_d\) from Eq. 18 . Then, for each document pair ( i , j ) where \(x_{ij}=1\) , we decrement the network counts associated with \(x_{ij}\) , and re-sample \(y_{ij}\) with a proposal distribution derived from Eq. 21 :
which can be further simplified since the terms inside the exponential are very small, hence the exp term approximates to 1. We empirically inspected the exponential term and we found that almost all of them are between 0.99 and 1. This means the ratio of the exponentials is not significant for sampling new citing topic \(y_{ij}^\mathrm{new}\) . So we ignore the exponential term and let
We compute the acceptance probability A for the newly sampled \(y_{ij}^\mathrm{new}=y'\) , changed from \(y^\mathrm{old}_{ij}=y^*\) , and the successive change to the document-topic priors (from \({\hat{\theta }^{\prime }{^\mathrm{old}}}\) to \({\hat{\theta }}^{\prime }{^\mathrm{new}}\) ):
Note that we have abused the notations i and j in the above equation, where the i and j in the summation indexes all documents instead of pointing to particular document i and document j . We decided against introducing additional variables to make things less confusing.
Finally, if the sample is accepted, we update \(y_{ij}\) and the associated customer counts. Otherwise, we discard the sample and revert the changes.
5.3 Hyperparameter sampling
Hyperparameter sampling for the priors is important (Wallach et al. 2009 ). In our inference algorithm, we sample the concentration parameters \(\beta \) of all PYPs with an auxiliary variable sampler (Teh 2006a ), but leave the discount parameters \(\alpha \) fixed. We do not sample the \(\alpha \) due to the coupling of the parameter with the Stirling numbers cache.
Here we outline the procedure to sample the concentration parameter \(\beta ^\mathcal {N}\) of a PYP distributed variable \(\mathcal {N}\) , using an auxiliary variable sampler. Assuming each \(\beta ^\mathcal {N}\) has a Gamma distributed hyperprior with shape \(\tau _0\) and rate \(\tau _1\) , we first sample the auxiliary variables \(\xi \) and \(\psi _j\) for \(j \in \{0, T^\mathcal {N} -1 \}\) :
We then sample a new \(\beta '{^\mathcal {N}}\) from the following conditional posterior given the auxiliary variables:
In addition to the PYP hyperparameters, we also sample \(\lambda ^+, \lambda ^-\) and \(\lambda ^T\) with a Gibbs sampler. We let the hyperpriors for \(\lambda ^+, \lambda ^-\) and \(\lambda ^T\) to be Gamma distributed with shape \(\epsilon _0\) and rate \(\epsilon _1\) . With the conjugate Gamma prior, the posteriors for \(\lambda ^+_i, \lambda ^-_i\) and \(\lambda ^T_k\) are also Gamma distributed, so they can be sampled directly.
We apply vague priors to the hyperpriors by setting \(\tau _0 = \tau _1 = \epsilon _0 = \epsilon _1 = 1\) .
Before we proceed with the next section on the datasets used in the paper, we summarise the full inference algorithm for the SCNTM in Algorithm 1.
We perform our experiments on subsets of CiteSeer \(^{\mathrm{X}}\) data Footnote 4 which consists of scientific publications. Each publication from CiteSeer \(^{\mathrm{X}}\) is accompanied by title , abstract , keywords , authors , citations and other metadata. We prepare three publication datasets from CiteSeer \(^{\mathrm{X}}\) for evaluations. The first dataset corresponds to Machine Learning (ML) publications, which are queried from CiteSeer \(^{\mathrm{X}}\) using the keywords from Microsoft Academic Search. Footnote 5 The ML dataset contains 139,227 publications. Our second dataset corresponds to publications from ten distinct research areas. The query words for these ten disciplines are chosen such that the publications form distinct clusters. We name this dataset M10 (Multidisciplinary 10 classes), which is made of 10,310 publications. For the third dataset, we query publications from both arts and science disciplines. Arts publications are made of history and religion publications, while the science publications contain physics , chemistry and biology research. This dataset consists of 18,720 publications and is named Arts versus Science (AvS) in this paper. These queried datasets are made available online. Footnote 6
The keywords used to create the datasets are obtained from Microsoft Academic Search, and are listed in “Appendix 2”. For the clustering evaluation in Sect. 7.4 , we treat the query categories as the ground truth. However, publications that span multiple disciplines can be problematic for clustering evaluation, hence we simply remove the publications that satisfy the queries from more than one discipline. Nonetheless, the labels are inherently noisy. The metadata for the publications can also be noisy, for instance, the authors field may sometimes display publication’s keywords instead of the authors, publication title is sometimes an URL, and table of contents can be mistakenly parsed as the abstract. We discuss our treatments to these issues in Sect. 6.1 . We also note that non-English publications are discarded using langid.py (Lui and Baldwin 2012 ).
In addition to the manually queried datasets, we also make use of existing datasets from LINQS (Sen et al. 2008 ) Footnote 7 to facilitate comparison with existing work. In particular, we use their CiteSeer, Cora and PubMed datasets. Their CiteSeer data consists of Computer Science publications and hence we name the dataset CS to remove ambiguity. Although these datasets are small, they are fully labelled and thus useful for clustering evaluation. However, these three datasets do not come with additional metadata such as the authorship information. Note that the CS and Cora datasets are presented as Boolean matrices, i.e. the word counts information is lost and we assume that all words in a document occur only once. Additionally, the words have been converted to integer so they do not convey any semantics. Although this representation is less useful for topic modelling, we still use them for the sake of comparison. For the PubMed dataset, we recover the word counts from TF–IDF using a simple assumption (see “Appendix 3”). We present a summary of the datasets in Table 2 and their respective categorical labels in Table 3 .
6.1 Data noise removal
Here, we briefly discuss the steps taken to reduce the corrupted entries in the CiteSeer \(^{\mathrm{X}}\) datasets (ML, M10 and AvS). Note that the keywords field in the publications are often empty and are sometimes noisy, that is, they contain irrelevant information such as section heading and title, which makes the keywords unreliable source of information as categories. Instead, we simply treat the keywords as part of the abstracts. We also remove the URLs from the data since they do not provide any additional useful information.
Moreover, the author information is not consistently presented in CiteSeer \(^{\mathrm{X}}\) . Some of the authors are shown with full name, some with first name initialised, while some others are prefixed with title (Prof, Dr. etc. ). We thus standardise the author information by removing all title from the authors, initialising all first names and discarding the middle names. Although standardisation allows us to match up the authors, it does not solve the problem that different authors who have the same initial and last name are treated as a single author. For example, both Bruce Lee and Brett Lee are standardised to B. Lee. Note this corresponds to a whole research problem (Han et al. 2004 , 2005 ) and hence not addressed in this paper. Occasionally, institutions are mistakenly treated as authors in CiteSeer \(^{\mathrm{X}}\) data, example includes American Mathematical Society and Technische Universität München . In this case, we remove the invalid authors using a list of exclusion words. The list of exclusion words is presented in “Appendix 4”.
6.2 Text preprocessing
Here, we discuss the preprocessing pipeline adopted for the queried datasets (note LINQS data were already processed). First, since publication text contains many technical terms that are made of multiple words, we tokenise the text using phrases (or collocations) instead of unigram words. Thus, phrases like decision tree are treated as single token rather than two distinct words. Then, we use LingPipe (Carpenter 2004 ) Footnote 8 to extract the significant phrases from the respective datasets. We refer the readers to the online tutorial Footnote 9 for details. In this paper, we use the word words to mean both unigram words and phrases.
We then change all the words to lower case and filter out certain words. Words that are removed are stop words , common words and rare words. More specifically, we use the stop words list from MALLET (McCallum 2002 ). Footnote 10 We define common words as words that appear in more than 18 % of the publications, and rare words are words that occur less than 50 times in each dataset. Note that the thresholds are determined by inspecting the words removed. Finally, the tokenised words are stored as arrays of integers. We also split the datasets to 90 % training set for training the topic models, and 10 % test set for evaluations detailed in Sect. 7 .
7 Experiments and results
In this section, we describe experiments that compare the SCNTM against several baseline topic models. The baselines are HDP-LDA with burstiness (Buntine and Mishra 2014 ), a non-parametric extension of the ATM, the Poisson mixed-topic link model (PMTLM) (Zhu et al. 2013 ). We also display the results for the CNTM without the citation network for comparison purpose. We evaluate these models quantitatively with goodness-of-fit and clustering measures.
7.1 Experimental settings
In the following experiments, we initialise the concentration parameters \(\beta \) of all PYPs to 0.1, noting that the hyperparameters are updated automatically. We set the discount parameters \(\alpha \) to 0.7 for all PYPs corresponding to the “word” side of the SCNTM (i.e. \(\gamma , \phi , \phi '\) ). This is to induce power-law behaviour on the word distributions. We simply set the \(\alpha \) to 0.01 for all other PYPs.
Note that the number of topics grow with data in non-parametric topic modelling. To prevent the learned topics from being too fine-grained, we set a limit to the maximum number of topics that can be learned. In particular, we have the number of topics cap at 20 for the ML dataset, 50 for the M10 dataset and 30 for the AvS dataset. For all the topic models, our experiments find that the number of topics always converges to the cap. For CS, Cora and PubMed datasets, we fix the number of topics to 6, 7 and 3 respectively for comparison against the PMTLM.
When training the topic models, we run the inference algorithm for 2,000 iterations. For the SCNTM, the MH algorithm for the citation network is performed after the 1,000th iteration. This is so the topics can be learned from the collapsed Gibbs sampler first. This gives a faster learning algorithm and also allows us to assess the “value-added” by the citation network to topic modelling (see Sect. 9.1 ). We repeat each experiment five times to reduce the estimation error of the evaluation measures.
7.2 Estimating the test documents’ topic distributions
The topic distribution \(\theta '\) on the test documents is required to perform various evaluations on topic models. These topic distributions are unknown and hence need to be estimated. Standard practice uses the first half of the text in each test document to estimate \(\theta '\) , and uses the other half for evaluations. However, since abstracts are relatively shorter compared to articles, adopting such practice would mean there are too little text to be used for evaluations. Instead, we used only the words from the publication title to estimate \(\theta '\) , allowing more words for evaluation. Moreover, title is also a good indicator of topic so it is well suited to be used in estimating \(\theta '\) . The estimated \(\theta '\) will be used in perplexity and clustering evaluations below. We note that for the clustering task, both title and abstract text are used in estimating \(\theta '\) as there is no need to use the text for clustering evaluation.
We briefly describe how we estimate the topic distributions \(\theta '\) of the test documents. Denoting \(w_{dn}\) to represent the word at position n in a test document d , we independently estimate the topic assignment \(z_{dn}\) of word \(w_{dn}\) by sampling from its predictive posterior distribution given the learned topic distributions \(\nu \) and topic-word distributions \(\phi \) :
where \(b = a_d\) if \(\mathrm {significance}(a_d) = 1\) , else \(b = e_d\) . Note that the intermediate distributions \(\phi '\) are integrated out (see “Appendix 5”).
We then build the customer counts \(c^{\theta _d}\) from the sampled z (for simplicity, we set the corresponding table counts as half the customer counts). With these, we then estimate the document-topic distribution \(\theta '\) from Eq. 18 .
If citation network information is present, we refine the document-topic distribution \(\theta '_d\) using the linking topic \(y_{dj}\) for train document j where \(x_{dj} = 1\) . The linking topic \(y_{dj}\) is sampled from the estimated \(\theta '_d\) and is added to the customer counts \(c^{\theta '_d}\) , which further updates the document-topic distribution \(\theta '_d\) .
Doing the above gives a sample of the document-topic distribution \(\theta '^{(s)}_d\) . We adopt a Monte Carlo approach by generating \(R=500\) samples of \(\theta '^{(s)}_d\) , and calculate the Monte Carlo estimate of \(\theta '_d\) :
7.3 Goodness-of-fit test
Perplexity is a popular metric used to evaluate the goodness-of-fit of a topic model. Perplexity is negatively related to the likelihood of the observed words \(\mathbf {W}\) given the model, so the lower the better:
where \(p(w_{dn}|\theta '_d, \phi )\) is obtained by summing over all possible topics:
again noting that the distributions \(\phi '\) and \(\theta \) are integrated out (see the method in “Appendix 5”).
We can calculate the perplexity estimate for both the training data and test data. Note that the perplexity estimate is unbiased since the words used in estimating \(\theta \) are not used for evaluation. We present the perplexity result in Table 4 , showing the significantly (at 5 % significance level) better performance of SCNTM against the baselines on ML, M10 and AvS datasets. For these datasets, inclusion of citation information also provides additional improvement for model fitting, as shown in the comparison with CNTM without network component. For the CS, Cora and PubMed datasets, the non-parametric ATM was not performed due to the lack of authorship information. We note that the results for other \(\eta \) is not presented as they are significantly worse than \(\eta =0\) . This is because the models are more restrictive, causing the likelihood to be worse. We like to point out that when no author is observed, the CNTM is more akin to a variant of HDP-LDA which uses PYP instead of DP, this explains why the perplexity results are very similar.
7.4 Document clustering
Next, we evaluate the clustering ability of the topic models. Recall that topic models assign a topic to each word in a document, essentially performing a soft clustering in which the membership is given by the document-topic distribution \(\theta \) . For the following evaluation, we convert the soft clustering to hard clustering by choosing a topic that best represents the documents, hereafter called the dominant topic . The dominant topic corresponds to the topic that has the highest proportion in a topic distribution.
As mentioned in Sect. 6 , for M10 and AvS datasets, we assume their ground truth classes correspond to the query categories used in creating the datasets. The ground truth classes for CS, Cora and PubMed datasets are provided. We evaluate the clustering performance with purity and normalised mutual information (NMI) (Manning et al. 2008 ). Purity is a simple clustering measure which can be interpreted as the proportion of documents correctly clustered, while NMI is an information theoretic measures used for clustering comparison. For ground truth classes \(\mathcal {S} = \{s_1, \dots , s_J\}\) and obtained clusters \(\mathcal {R} = \{r_1, \dots , r_K\}\) , the purity and NMI are computed as
where \(I(\mathcal {S}; \mathcal {R})\) denotes the mutual information and \(H(\cdot )\) denotes the entropy:
The clustering results are presented in Table 5 . We can see that the SCNTM greatly outperforms the PMTLM in NMI evaluation. Note that for a fair comparison against PMTLM, the experiments on the CS, Cora and PubMed datasets are evaluated with a 10-fold cross validation. We find that incorporating supervision into the topic model leads to improvement on clustering task, as predicted. However, this is not the case for the PubMed dataset. We suspect this is because the publications in the PubMed dataset are highly related to one another so the category labels are less useful (see Table 3 ).
8 Qualitative analysis of learned topic models
We move on to perform qualitative analysis on the learned topic models in this section. More specifically, we inspect the learned topic-word distributions, as well as the topics associated with the authors. Additionally, we present a visualisation of the author-topic network learned by the SCNTM.
8.1 Topical summary of the datasets
By analysing the topic-word distribution \(\phi _k\) for each topic k , we obtain the topical summary of the datasets. This is achieved by querying the top words associated with each topic k from \(\phi _k\) , which are learned by the SCNTM. The top words give us an idea of what the topics are about. In Table 6 , we display some major topics extracted and the corresponding top words. We note that the topic labels are manually assigned based on the top words. For example, we find that the major topics associated with the ML dataset are various disciplines on machine learning such as reinforcement learning and data mining.
We did not display the topical summary for the CS, Cora and PubMed datasets. The reason being that the original word information is lost in the CS and Cora datasets since the words were converted into integers, which are not meaningful. While for the PubMed dataset, we find that the topics are too similar to each other and thus not interesting. This is mainly because the PubMed dataset focuses only on one particular topic, which is on Diabetes Mellitus.
8.2 Analysing authors’ research area
In SCNTM, we model the author-topic distribution \(\nu _i\) for each author i . This allows us to analyse the topical interest of each author in a collection of publications. Here, we focus on the M10 dataset since it covers a more diverse research areas. For each author i , we can determine their dominant topic k by looking for the largest topic in \(\nu _i\) . Knowing the dominant topic k of the authors, we can then extract the corresponding top words from the topic-word distribution \(\phi _k\) . In Table 7 , we display the dominant topic associated with several major authors and the corresponding top words. For instance, we can see that the author D. Aerts’s main research area is in quantum theory, while M. Baker focuses on financial markets. Again, we note that the topic labels are manually assigned to the authors based on the top words associated with their dominant topics.
8.3 Author-topics network visualisation
In addition to inspecting the topic and word distributions, we present a way to graphically visualise the author-topics network extracted by SCNTM, using Graphviz . Footnote 11 On the ML, M10 and AvS datasets, we analyse the influential authors and their connections with the various topics learned by SCNTM. The influential authors are determined based on a measure we call author influence, which is the sum of the \(\lambda ^-\) of all their publications, i.e. the influence of an author i is \(\sum _d \lambda ^-_d \, I(a_d = i)\) . Note that \(a_d\) denotes the author of document d , and \(I(\cdot )\) is the indicator function, as previously defined.
Snapshot of the author-topics network from the ML dataset. The pink rectangles represent the learned topics, their intensity ( pinkness ) corresponds to the topic proportion. The ellipses represent the authors, their size corresponds to the author’s influence in the corpus. The strength of the connections are given by the lines’ thickness (Color figure online)
Figure 2 shows a snapshot of the author-topics network of the ML dataset. The pink rectangles in the snapshot represent the topics learned by SCNTM, showing the top words of the associated topics. The colour intensity (pinkness) of the rectangle shows the relative weight of the topics in the corpus. Connected to the rectangles are ellipses representing the authors, their size is determined by their corresponding author influence in the corpus. For each author, the thickness of the line connecting to a topic shows the relative weight of the topic. Note that not all connections are shown, some of the weak connections are dropped to create a neater diagram. In Fig. 2 , we can see that Z. Ghahramani works mainly in the area of Bayesian inference, as illustrated by the strong connection to the topic with top words “bayesian, networks, inference, estimation, probabilistic”. While N. Friedman works in both Bayesian inference and machine learning classification, though with a greater proportion in Bayesian inference. Due to the large size of the plots, we present online Footnote 12 the full visualisation of the author-topics network learned from the CiteSeer \(^{\mathrm{X}}\) datasets.
9 Diagnostics
In this section, we perform some diagnostic tests for the SCNTM. We assess the convergence of the MCMC algorithm associated with SCNTM and inspect the counts associated with the PYP for the document-topic distributions. Finally, we also present a discussion on the running time of the SCNTM.
9.1 Convergence analysis
It is important to assess the convergence of an MCMC algorithm to make sure that the algorithm is not prematurely terminated. In Fig. 3 , we show the time series plot of the training word log likelihood \(\sum _{d,n} \log (p(w_{dn} \,|\, z_{dn}, \phi '))\) corresponds to the SCNTM trained with and without the network information. Recall that for SCNTM, the sampler for the topic model is first performed for 1,000 iterations before running the full MCMC algorithm. From Fig. 3 , we can clearly see that the sampler converges quickly. For SCNTM, it is interesting to see that the log likelihood improves significantly once the network information is used for training (red lines), suggesting that the citation information is useful. Additionally, we like to note that the acceptance rate of the MH algorithm for the citation network averages about 95 %, which is very high, suggesting that the proposed MH algorithm is effective.
(Coloured) Training word log likelihood versus iterations during training of the CNTM with and without the network component. The red lines show the log likelihoods of the SCNTM with the citation network while the blue lines represent the SCNTM without the citation network. The five runs are from five different folds of the Cora dataset (Color figure online)
9.2 Inspecting document-topic hierarchy
As previously mentioned, modelling the document-topic hierarchy allows us to balance the contribution of text information and citation information toward topic modelling. In this section, we inspect the customer and table counts associated with the document-topic distributions \(\theta '\) and \(\theta \) to give an insight on how the above modelling works. We first note that the number of words in a document tend to be higher than the number of citations.
We illustrate with an example from the ML dataset. We look at the 600th document, which contains 84 words but only 4 citations. The words are assigned to two topics and we have \(c_1^\theta = 53\) and \(c_2^\theta = 31\) . These customer counts are contributed to \(\theta '\) by way of the corresponding table counts \(t_1^\theta = 37\) and \(t_2^\theta = 20\) . The citations contribute counts directly to \(\theta '\) , in this case, three of the citations are assigned the first topic while another one is assigned to the second topic. The customer count for \(\theta '\) is the sum of the table counts from \(\theta \) and the counts from citations. Thus, \(c_1^{\theta '} = 37 + 3 = 40\) and \(c_2^{\theta '} = 20 + 1 = 21\) . Note that the counts from \(\theta '\) are used to determine the topic composition of the document. By modelling the document-topic hierarchy, we have effectively diluted the influence of text information. This is essential to counter the higher number of words compared to citations.
9.3 Computation complexity
Finally, we briefly discuss the computational complexity of the proposed MCMC algorithm for the SCNTM. Although we did not particularly optimise our implementation for algorithm speed, the algorithm is of linear time with the number of words, the number of citations and the number of topics. All implementations are written in Java .
We implemented a general sampling framework that works with arbitrary PYP network, this allows us to test various PYP topic models with ease and without spending too much time in coding. However, having a general framework for PYP topic models means it is harder to optimise the implementation, thus it performs slower than existing implementations (such as hca Footnote 13 ). Nevertheless, the running time is linear with the number of words in the corpus and the number of topics, and constant time with the number of citations.
A naïve implementation of the MH algorithm for the citation network would be of polynomial time, due to the calculation of the double summation in the posterior. However, with caching and reformulation of the double summation, we can evaluate the posterior in linear time. Our implementation of the MH algorithm is linear (in time) with the number of citations and the number of topics, and it is constant time with respect to the number of words. The MCMC algorithm is constant time with respect to the number of authors.
Table 8 shows the average time taken to perform the MCMC algorithm for 2000 iterations. All the experiments were performed with a machine having Intel(R) Core(TM) i7 CPU @ 3.20GHz (though only 1 processor was used) and 24 Gb RAM.
10 Conclusions
In this paper, we have proposed the Supervised Citation Network Topic Model (SCNTM) as an extension of our previous work (Lim and Buntine 2014 ) to jointly model research publications and their citation network. The SCNTM makes use of the author information as well as the categorical labels associated with each document for supervised learning. The SCNTM performs text modelling with a hierarchical PYP topic model and models the citations with the Poisson distribution given the learned topic distributions. We also proposed a novel learning algorithm for the SCNTM, which exploits the conjugacy of the Dirichlet distribution and the Multinomial distribution, allowing the sampling of the citation networks to be of similar form to the collapsed sampler of a topic model. As discussed, our learning algorithm is intuitive and easy to implement.
The SCNTM offers substantial performance improvement over previous work (Zhu et al. 2013 ). On three CiteSeer \(^{\mathrm{X}}\) datasets and three existing and publicly available datasets, we demonstrate the improvement of joint topic and network modelling in terms of model fitting and clustering evaluation. Additionally, incorporating supervision into the SCNTM provides further improvement on the clustering task. Analysing the learned topic models let us extract useful information on the corpora, for instance, we can inspect the learned topics associated with the documents and examine the research interest of the authors. We also visualise the author-topic network learned by the SCNTM, which allows us to have a quick look at the connection between the authors by way of their research areas.
Abstract and publication title.
The author network here corresponds to the Twitter follower network.
The algorithm is named MH algorithm instead of Gibbs sampling due to the fact that the sample space for the counts is restricted and thus we are not sampling from the posterior directly.
http://citeseerx.ist.psu.edu/ .
http://academic.research.microsoft.com/ .
http://karwai.weebly.com/publications.html .
http://linqs.cs.umd.edu/projects/projects/lbc/ .
http://alias-i.com/lingpipe/ .
http://alias-i.com/lingpipe/demos/tutorial/interestingPhrases/read-me.html .
http://mallet.cs.umass.edu/ .
http://www.graphviz.org/ .
https://drive.google.com/folderview?id=0B74l2KFRFZJmVXdmbkc3UlpUbzA (please download and view with a web browser for best quality).
http://mloss.org/software/view/527/ .
Note that there are multiple ways to define a TF–IDF in practice. The specific TF–IDF formula used by the PubMed dataset was determined via trial-and-error and elimination.
Blei, D., Ng, A., & Jordan, M. (2003). Latent Dirichlet Allocation. JMLR , 3 , 993–1022.
MATH Google Scholar
Buntine, W., & Hutter, M. (2012). A Bayesian view of the Poisson-Dirichlet process. ArXiv e-prints 1007.0296v2.
Buntine, W., & Mishra, S. (2014). Experiments with non-parametric topic models. In KDD (pp 881–890). ACM.
Carpenter, B. (2004). Phrasal queries with LingPipe and Lucene: Ad hoc genomics text retrieval. In TREC .
Casella, G., & Robert, C. P. (1996). Rao-Blackwellisation of sampling schemes. Biometrika , 83 (1), 81–94.
Article MathSciNet MATH Google Scholar
Chang, J., & Blei, D. (2010). Hierarchical relational models for document networks. The Annals of Applied Statistics , 4 (1), 124–150.
Chen, C., Du, L., & Buntine, W. (2011). Sampling table configurations for the hierarchical Poisson-Dirichlet process. In ECML (pp. 296–311). Springer.
Goldwater, S., Griffiths, T., & Johnson, M. (2011). Producing power-law distributions and damping word frequencies with two-stage language models. JMLR , 12 , 2335–2382.
MathSciNet MATH Google Scholar
Han, H., Giles, C. L., Zha, H., Li, C., & Tsioutsiouliklis, K. (2004). Two supervised learning approaches for name disambiguation in author citations. In JCDL (pp. 296–305). ACM.
Han, H., Zha, H., & Giles, C. L. (2005). Name disambiguation in author citations using a K-way spectral clustering method. In JCDL (pp. 334–343). ACM.
Kataria, S., Mitra, P., Caragea, C., & Giles, C. L. (2011). Context sensitive topic models for author influence in document networks. In IJCAI (pp. 2274–2280). AAAI Press.
Lim, K. W., & Buntine, W. (2014). Bibliographic analysis with the citation network topic model. In ACML (pp. 142–158).
Lim, K. W., Chen, C., & Buntine, W. (2013). Twitter-network topic model: A full Bayesian treatment for social network and text modeling. In NIPS Topic Model workshop .
Liu, L., Tang, J., Han, J., Jiang, M., & Yang, S. (2010). Mining topic-level influence in heterogeneous networks. In CIKM (pp. 199–208). ACM.
Liu, Y., Niculescu-Mizil, A., & Gryc, W. (2009). Topic-link LDA: Joint models of topic and author community. In ICML (pp. 665–672). ACM.
Lui, M., & Baldwin, T. (2012). langid.py: An off-the-shelf language identification tool. In ACL (pp. 25–30). ACL.
Manning, C., Raghavan, P., & Schütze, H. (2008). Introduction to information retrieval . Cambridge: Cambridge University Press.
Book MATH Google Scholar
McCallum, A. K. (2002). MALLET: A machine learning for language toolkit. http://www.cs.umass.edu/~mccallum/mallet .
Mimno, D., McCallum, A. (2007). Mining a digital library for influential authors. In JCDL (pp. 105–106). ACM.
Nallapati, R., Ahmed, A., Xing, E., & Cohen, W. (2008). Joint latent topic models for text and citations. In KDD (pp. 542–550). ACM.
Oehlert, G. W. (1992). A note on the delta method. The American Statistician , 46 (1), 27–29.
MathSciNet Google Scholar
Pitman, J. (1996). Some developments of the Blackwell–Macqueen urn scheme. Lecture Notes—Monograph Series (pp. 245–267).
Rosen-Zvi, M., Griffiths, T., Steyvers, M., & Smyth, P. (2004). The author-topic model for authors and documents. In UAI (pp. 487–494). AUAI Press.
Sato, I., & Nakagawa, H. (2010). Topic models with power-law using Pitman–Yor process. In KDD (pp. 673–682). ACM.
Sen, P., Namata, G., Bilgic, M., Getoor, L., Gallagher, B., & Eliassi-Rad, T. (2008). Collective classification in network data. AI Magazine , 29 (3), 93–106.
Google Scholar
Tang, J., Sun, J., Wang, C., & Yang, Z. (2009). Social influence analysis in large-scale networks. In KDD (pp. 807–816). ACM.
Teh, Y. W. (2006a). A Bayesian interpretation of interpolated Kneser–Ney. Tech. rep., School of Computing, National University of Singapore.
Teh, Y. W. (2006b). A hierarchical Bayesian language model based on Pitman–Yor processes. In ACL (pp 985–992). ACL.
Teh, Y. W., Jordan, M. (2010). Hierarchical Bayesian nonparametric models with applications. In N. L. Hjort, C. Holmes, P. Müller, & S. G. Walker (Eds.), Bayesian nonparametrics: Principles and practice (Chap. 5). Cambridge University Press.
Tu, Y., Johri, N., Roth, D., & Hockenmaier, J. (2010). Citation author topic model in expert search. In COLING (pp. 1265–1273). ACL.
Wallach, H., Mimno, D., & McCallum, A. (2009). Rethinking LDA: Why priors matter. In NIPS (pp. 1973–1981).
Weng, J., Lim, E. P., Jiang, J., & He, Q. (2010). TwitterRank: Finding topic-sensitive influential Twitterers. In WSDM (pp. 261–270). ACM.
Zhu, Y., Yan, X., Getoor, L., & Moore, C. (2013). Scalable text and link analysis with mixed-topic link models. In KDD (pp 473–481). ACM.
Download references
Acknowledgments
NICTA is funded by the Australian Government through the Department of Communications and the Australian Research Council through the ICT Centre of Excellence Program. The authors wish to thank CiteSeer \(^{\mathrm{X}}\) for providing the data.
Author information
Authors and affiliations.
The Australian National University (ANU) and NICTA, Canberra, Australia
Kar Wai Lim
Monash University, Clayton, Australia
Wray Buntine
You can also search for this author in PubMed Google Scholar
Corresponding author
Correspondence to Kar Wai Lim .
Additional information
Editors: Hang Li, Dinh Phung, Tru Cao, Tu-Bao Ho, and Zhi-Hua Zhou.
Appendix1: Delta method approximation
We employ the Delta Method to show that
where \({\hat{\theta }}\) is the expected value according to a distribution proportional to \(q(\theta )\) , more specifically, define \(p(\theta )\) as the probability density of \(\theta \) , we have
First we note that the Taylor expansion for a function \(h(\theta ) = \exp (-g(\theta ))\) at \({\hat{\theta }}\) is
where \(h^{(n)}(\hat{\theta })\) denotes the n -th derivative of \(h(\cdot )\) evaluated at \(\hat{\theta }\) :
Multiply Eq. 45 with \(q(\theta )\) and integrating gives
Since \(g(\hat{\theta })\) is small, the term \(\left( - g'(\hat{\theta }) \right) ^n\) becomes exponentially smaller as n increases. Here we let \(\left( - g'(\hat{\theta }) \right) ^n \approx 0\) for \(n \ge 2\) . Hence, continuing from Eq. 47 :
Appendix 2: Keywords for querying the CiteSeer \(^{\mathrm{X}}\) datasets
1. For ML dataset:
Machine Learning : Machine learning, neural network, pattern recognition, indexing term, support vector machine, learning algorithm, computer vision, face recognition, feature extraction, image processing, high dimensionality, image segmentation, pattern classification, real time, feature space, decision tree, principal component analysis, feature selection, backpropagation, edge detection, object recognition, maximum likelihood, statistical learning theory, supervised learning, reinforcement learning, radial basis function, support vector, em algorithm, self organization, image analysis, hidden markov model, artificial neural network, independent component analysis, genetic algorithm, statistical model, dimensional reduction, indexation, unsupervised learning, gradient descent, large scale, maximum likelihood estimate, statistical pattern recognition, cluster algorithm, markov random field, error rate, optimization problem, satisfiability, high dimensional data, mobile robot, nearest neighbour, image sequence, neural net, speech recognition, classification accuracy, diginal image processing, factor analysis, wavelet transform, local minima, probability distribution, back propagation, parameter estimation, probabilistic model, feature vector, face detection, objective function, signal processing, degree of freedom, scene analysis, efficient algorithm, computer simulation, facial expression, learning problem, machine vision, dynamic system, bayesian network, mutual information, missing value, image database, character recognition, dynamic program, finite mixture model, linear discriminate analysis, image retrieval, incomplete data, kernel method, image representation, computational complexity, texture feature, learning method, prior knowledge, expectation maximization, cost function, multi layer perceptron, iterated reweighted least square, data mining.
2. For M10 dataset:
Biology : Enzyme, gene expression, amino acid, Escherichia coli , transcription factor, nucleotides, dna sequence, Saccharomyces cerevisiae , plasma membrane, embryonics.
Computer Science : Neural network, genetic algorithm, machine learning, information retrieval, data mining, computer vision, artificial intelligent, optimization problem, support vector machine, feature selection.
Social Science : Developing country, higher education, decision making, health care, high school, social capital, social science, public health, public policy, social support.
Financial Economics : Stock returns, interest rate, stock market, stock price, exchange rate, asset prices, capital market, financial market, option pricing, cash flow.
Material Science : Microstructures, mechanical property, grain boundary, transmission electron microscopy, composite material, materials science, titanium, silica, differential scanning calorimetry, tensile properties.
Physics : Magnetic field, quantum mechanics, field theory, black hole, kinetics, string theory, elementary particles, quantum field theory, space time, star formation.
Petroleum Chemistry : Fly ash, diesel fuel, methane, methyl ester, diesel engine, natural gas, pulverized coal, crude oil, fluidized bed, activated carbon.
Industrial Engineering : Power system, construction industry, induction motor, power converter, control system, voltage source inverter, permanent magnet, digital signal processor, sensorless control, field oriented control.
Archaeology : Radiocarbon dating, iron age, bronze age, late pleistocene, middle stone age, upper paleolithic, ancient dna, early holocene, human evolution, late holocene.
Agriculture : Irrigation water, soil water, water stress, drip irrigation, grain yield, crop yield, growing season, soil profile, soil salinity, crop production.
3. For AvS dataset:
History : Nineteeth century, cold war, south africa, foreign policy, civil war, world war ii, latin america, western europe, vietnam, middle east.
Religion : Social support, foster care, child welfare, human nature, early intervention, gender difference, sexual abuse, young adult, self esteem, social services.
Physics : Magnetic field, quantum mechanics, string theory, field theory, numerical simulation, black hole, thermodynamics, phase transition, electric field, gauge theory.
Chemistry : Crystal structure, mass spectrometry, copper, aqueous solution, binding site, hydrogen bond, oxidant stress, free radical, liquid chromatography, organic compound.
Biology : Genetics, enzyme, gene expression, polymorphism, nucleotides, dna sequence, Saccharomyces cerevisiae , cell cycle, plasma membrane, embryonics.
Appendix 3: Recovering word counts from TF–IDF
The PubMed dataset (Sen et al. 2008 ) was preprocessed to TF–IDF (term frequency–inverse document frequency) format, i.e. the raw word count information is lost. Here, we describe how we recover the word count information, using a simple and reasonable assumption—that the least occurring words in a document only occur once.
We denote \(t_{dw}\) as the TF–IDF for word w in document \(d, f_{dw}\) as the corresponding term frequency (TF), and \(i_w\) as the inverse document frequency (IDF) for word w . Our aim is to recover the word counts \(c_{dw}\) given the TF–IDF. TF–IDF is computed Footnote 14 as
where \(I(\cdot )\) is the indicator function.
We note that \(I(c_{dw} > 0) = I(t_{dw} > 0)\) since the TF–IDF for a word w is positive if and only if the corresponding word count is positive. This allows us to compute the IDF \(i_w\) easily from Eq. 49 . We can then determine the TF:
Now we are left with computing \(c_{dw}\) given the \(f_{dw}\) , however, we can obtain infinitely many solutions since we can always multiply \(c_{dw}\) by a constant and get the same \(f_{dw}\) . Fortunately, since we are working with natural language, it is reasonable to assume that the least occurring words in a document only occur once, or mathematically,
Thus we can work out the normaliser \(\sum _w c_{dw}\) and recover the word counts for all words in all documents.
Appendix 4: Exclusion words to detect invalid authors
Below is a list of words we use to filter out invalid authors during preprocessing step:
Society, university, universität, universitat, author, advisor, acknowledgement, video, mathematik, abstract, industrial, review, example, department, information, enterprises, informatik, laboratory, introduction, encyclopedia, algorithm, section, available.
Appendix 5: Integrating out probability distributions
Here, we show how to integrate out probability distributions using the expectation of a PYP:
where \({\mathbb {E}}[\cdot ]\) denotes the expectation value. We note that the last step (Eq. 53 ) follows from the fact that the expected value of a PYP is the probability vector corresponding to the base distribution of the PYP (when the base distribution is a probability distribution). A similar approach can be taken to integrate out the \(\theta \) in Eq. 40 .
Rights and permissions
Reprints and permissions
About this article
Lim, K.W., Buntine, W. Bibliographic analysis on research publications using authors, categorical labels and the citation network. Mach Learn 103 , 185–213 (2016). https://doi.org/10.1007/s10994-016-5554-z
Download citation
Received : 24 March 2015
Accepted : 23 February 2016
Published : 11 March 2016
Issue Date : May 2016
DOI : https://doi.org/10.1007/s10994-016-5554-z
Share this article
Anyone you share the following link with will be able to read this content:
Sorry, a shareable link is not currently available for this article.
Provided by the Springer Nature SharedIt content-sharing initiative
- Bibliographic analysis
- Topic model
- Bayesian non-parametric
- Author-citation network
- Find a journal
- Publish with us
- Track your research
Academia.edu no longer supports Internet Explorer.
To browse Academia.edu and the wider internet faster and more securely, please take a few seconds to upgrade your browser .
Enter the email address you signed up with and we'll email you a reset link.
- We're Hiring!
- Help Center
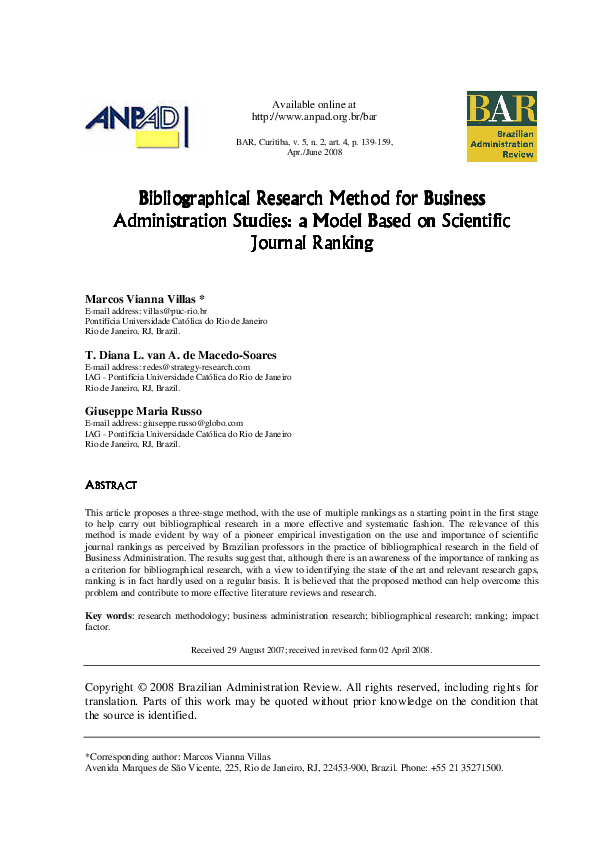
Bibliographical research method for business administration studies: a model based on scientific journal ranking

2008, BAR. Brazilian Administration …
Related Papers
Marcos Villas , Giuseppe Russo
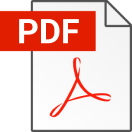
Scientometrics
Ronald Rousseau
Manuel Aníbal Silva Portugal Vasconcelos Ferreira
Resumo Publicar é essencial para os pesquisadores e as universidades, e publicar em periódicos internacionais com revisão pelos pares e ranqueados é, pelo menos, uma ambição crescente dos pesquisadores brasileiros em Administração. Os rankings de periódicos são relevantes para os pesquisadores, universidades, agências reguladoras e instituições financiadoras. Os rankings de periódicos são usados diretamente na avaliação da qualidade dos artigos científicos dos pesquisadores e, indiretamente, contribuem para a avaliação da atratividade de uma universidade, ou programa, para estudantes, professores, reguladores e financiadores. Apesar de amplamente usados e informalmente debatidos, poucos pesquisadores conhecem os rankings, suas implicações e o que realmente significam. Neste artigo discuto algumas facetas dos rankings de periódicos em Administração, incluindo a sua utilidade, apresento alguns rankings, e debato problemas no sistema. Se os rankings de periódicos cada vez mais orientam os esforços dos pesquisadores, é necessário um debate mais alargado que incida sobre seus sistemas, virtudes e disfunções. Este debate permitirá, por um lado, desmistificar e, por outro, esclarecer à comunidade e, especialmente, aos menos conhecedores da importância de avaliar os periódicos antes de submeter suas pesquisas. Palavras-chave: Ranking de periódicos. Periódicos. Avaliação pelos pares. Qualis. Abstract Publishing is crucial for researchers and universities, and publishing in international peer reviewed journals is becoming, at least, a growing ambition of many management scholars. Journal rankings are relevant for faculty, universities, and financial and regulatory agencies. Journal rankings are used directly in assessing the quality of researchers' scientific output and, indirectly, they influence the attractiveness of universities, or departments, to new students, professors, regulators and financiers. Albeit widely used and informally debated, few are aware of the journal rankings, implications and what they really mean. In this paper, I discuss some of the facets involving the existence of journal rankings in management, including their usefulness, present some rankings and debate problems in the system. If journal rankings increasingly guide scholars' research efforts, a broader debate is warranted on the systems, virtues and dysfunctions. This debate will contribute, on one hand, to demystify, and on the other to elucidate the community, especially those less knowledgeable of the importance of assessing journals' quality prior to submitting their research.
International Journal of Entrepreneurial Behaviour & Research
John Cotton
ABSTRACT Purpose ‐ Dozens of peer-reviewed, English language journals are currently published in our field. How ought we to evaluate them? This paper seeks to answer this question. Design/methodology approach ‐ The paper utilizes both relevant literature and data on entrepreneurship journals. The literature derives from both information science and other research areas that reflect on their journals. The data derives from six citation measures from Google Scholar, Scopus, and Web of Science. Findings ‐ The paper finds that there are 59 currently published English language, peer reviewed journals in entrepreneurship. Contestable judgments based on their impact measures suggest that one of these 59 could be considered as &amp;quot;A+&amp;quot;, four as &amp;quot;A&amp;quot;, five as &amp;quot;AB&amp;quot;, eight as &amp;quot;B&amp;quot;, four as &amp;quot;BC&amp;quot;, 23 as &amp;quot;C&amp;quot;, thirteen as &amp;quot;barely detectable&amp;quot;, and one as &amp;quot;insufficient data but promising&amp;quot;. Research limitations/implications ‐ Journal rankings affect the resources and prestige accorded to business schools, disciplines and subdisciplines, and individual scholars. However, the need to fit evaluations to school strategy implies that no rating system, ours included, is definitive. Multiple measures are needed, letter grades are misleading, and journal rankings should match the institution&amp;#39;s strategy and priorities in stakeholder service. A wider purpose of this study is to alert readers to the range of current methodologies and the limits of conventional rankings. Originality/value ‐ The conclusions presented in this paper appear innocuous, but standard practice is to use restrictive measures, to employ letter grades, and to prioritize only one stakeholder: scholars. These practices are poorly suited to the entrepreneurship field.
Milad Yadollahi
Journal of Informetrics
Alexander Serenko
Ignacio Roche
El presente artículo consta de dos partes. En la primera, se intenta identificar qué revistas académicas (tanto españolas, como extranjeras) son las más prestigiosas y relevantes en el área de márketing, desde la perspectiva española. Una vez obtenido el listado de revistas de referencia del área de márketing, la segunda fase de la investigación trata de obtener una ordenación de las revistas que los profesores de marketing consideran más importantes para publicar en esta área, según los resultados obtenidos a partir del ránking anterior, y utilizando una metodología no aplicada hasta ahora: ordenación de las preferencias mediante un análisis conjunto.
RAC Eletrônica
Luciano Rossoni
RAC-Eletrônica
Edson R. Guarido Filho , Luciano Rossoni
Edson R. Guarido Filho
Loading Preview
Sorry, preview is currently unavailable. You can download the paper by clicking the button above.
RELATED PAPERS
Communications of the Association for Information Systems
Paul Benjamin Lowry , Vernon Richardson
Journal of Leadership, Accountability, and Ethics
Reginald L . Bell
International Journal of Business Governance and Ethics
Nick Bontis
lethukuthula praysgod
Scandinavian Journal of Hospitality and Tourism
Prof. Dr. Anita Zehrer
Journal of The Ais
Aaron Curtis
BAR. Brazilian Administration Review
Reed Elliot Nelson
Journal of the Association for Information Systems
Paul Benjamin Lowry
Journal of Management Studies
Stuart Macdonald
Journal of Management
David Van Fleet , Abagail McWilliams
Esic Market
Entrepreneurship Theory and Practice
Clyde Holsapple , Brian Santos
Journal of Knowledge Management
Michael Cuellar
Journal of Operations Management
Aravind Chandrasekaran
Journal of Marketing
Hans Baumgartner
Revista de Administração …
Alexandre Graeml
Avaliação: Revista da Avaliação da Educação Superior (Campinas)
Isabel Pinho
Jeanette Lindau
Journal of Applied Economics
Richard Watson
Dr Muzammil Tahira
European Educational Research Journal
Stuart von Wolff
Journal of Business Communication
Academy of Management Annual Meeting
Ricky Griffin
Information Processing and Management
Antonis Sidiropoulos
MIS Quarterly
Dennis Galletta
British Journal of Management
Michael Rowlinson
RELATED TOPICS
- We're Hiring!
- Help Center
- Find new research papers in:
- Health Sciences
- Earth Sciences
- Cognitive Science
- Mathematics
- Computer Science
- Academia ©2024
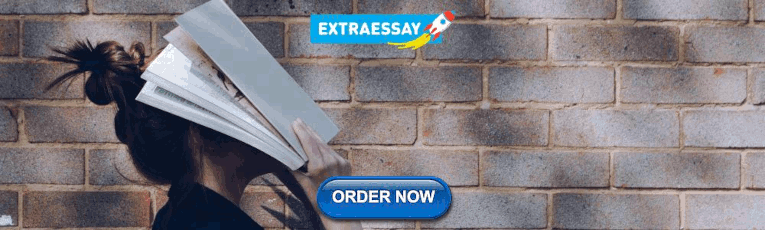
IMAGES
VIDEO
COMMENTS
The Bibliographic research or documentary consists in the revision of existing bibliographical material with respect to the subject to be studied. It is one of the main steps for any investigation and includes the selection of information sources. It is considered an essential step because it includes a set of phases that encompass observation ...
Student Research and Report Writing by Gabe T. Wang; Keumjae Park This is an invaluable, concise, all-in-one guide for carrying out student research and writing a paper, adaptable to course use and suitable for use by students independently, it successfully guides students along every step of the way. Allows students to better manage their research projects Exercises and worksheets break down ...
Sage Research Methods SAGE Research Methods supports beginning and advanced researchers in every step of a research project, from writing a research question, choosing a method, gathering and analyzing data, to writing up and publishing the findings. With information on the full range of qualitative, quantitative, and mixed methods for the ...
In other words: inform the reader that the analysis of bibliographic data with bibliometric methods provides important insights regarding your research goal. In general, bibliographic studies are particularly useful to describe the structure of a research field (see tip 2 above) and its development over time because they help to identify topic ...
The advantages of trying all these research methods are that: Each of these ways of searching is applicable in any subject area. None of them is confined exclusively to English-language sources. Each has both strengths and weaknesses, advantages and disadvantages. The weaknesses within any one method are balanced by the strengths of the others.
It's a bibliography of hundred books on Research Methodology. Entries made following standard bibliographical format with guide to users'. May be useful for research scholars. Content uploaded ...
The essential difference between citations and references is that citations lead a reader to the source of information, while references provide the reader with detailed information regarding that particular source. Bibliography in research papers: A bibliography in research paper is a list of sources that appears at the end of a research paper ...
Bibliography Entry for a Book. A bibliography entry for a book begins with the author's name, which is written in this order: last name, comma, first name, period. After the author's name comes the title of the book. If you are handwriting your bibliography, underline each title. If you are working on a computer, put the book title in ...
A bibliography is a list of works on a subject or by an author that were used or consulted to write a research paper, book or article. It can also be referred to as a list of works cited. It is usually found at the end of a book, article or research paper. Gathering Information. Regardless of what citation style is being used, there are key ...
Research is an endeavor to bring out the actual facts in a case by searching for them in places where they are thought to be found. There are several methods of research: the experimental method, for instance, the seminar method, or the bibliographical method. The experimental method is used when we bring out a new
Bibliographic analysis considers the author's research areas, the citation network and the paper content among other things. In this paper, we combine these three in a topic model that produces a bibliographic model of authors, topics and documents, using a non-parametric extension of a combination of the Poisson mixed-topic link model and the author-topic model. This gives rise to the ...
Proposal of a Bibliographical Research Method In terms of helping to render literature review processes more systematic, we propose a three-stage bibliographical research method that makes use of rankings and draws on Arenas et al. (2001). The proposed method adds effectiveness and rigor to the literature review process because it defines how ...
hensive bibliographical review (theor etical framework), which will be the base, the foundations of our research. This bibliographical review invol ves the analysis and e x-. planation of all the ...
Writing up research, or its oral presentation, is a 'site of contestation' (1), one which can be regarded as problem solving with its own subprocesses and mental events (2). Lewis-Beck, M.S., Bryman, A. & Liao, T.F. (eds) (2004) The Sage Encyclopaedia of Social Science Research Methods.
This article proposes a three-stage method, with the use of multiple rankings as a starting point in the first stage to help carry out bibliographical research in a more effective and systematic ...
A three-stage method, with the use of multiple rankings as a starting point in the first stage to help carry out bibliographical research in a more effective and systematic fashion, and contribute to more effective literature reviews and research. This article proposes a three-stage method, with the use of multiple rankings as a starting point in the first stage to help carry out ...
Proposal of a Bibliographical Research Method. In terms of helping to render literature review processes more systematic, we propose a three-stage bibliographical research method that makes use of rankings and draws on Arenas et al. (2001). The proposed method adds effectiveness and rigor to the literature review process because it defines how ...
research methods and pedagogical strategies are also provided. Librarians in search of a text that combines practical suggestions with ethical direction can find both in this book. - Christopher Hollister, March 2006 Published Review: Review of Foundations for research: Methods of inquiry in education and the social sciences,
The bibliographic review article is a methodology of observational research, retrospective, systematica ,oriented to the selection, analysis, interpretation and discussion of theoretical ...
The development of an adequate research method involved three steps: 1) a literature review; 2) a survey; and 3) the method proper which is described in Section Proposal of a Bibliographical Research Method. The literature review was carried out to identify the existing methods in different fields of knowledge.