Case Study: Can a Virus Cause Diabetes?
Part a: thirsty child.
Rianne was a healthy 8-year-old who enjoyed playing soccer and going to dance class with her older sister. Her family live in a rural town in central Illinois where the skyline consists of green cornfields and grain silos. She had just started the third grade when her mother noticed that she was losing weight. Rianne’s pants were too loose and she seemed tired all the time. At first, she wasn’t too worried, perhaps it was just a change to the new school and a new routine. Rianne seemed to be eating and drinking normally. In fact, Rianne was drinking a lot of water. One night, her mother work up to find Rianne awake at three am, drinking a glass of water. At breakfast, she drank another bottle of water and then said, “I’m just so thirsty all the time.”
Her mother told her to go to school and scheduled a doctor’s appointment, just in case something was wrong. Rianne still seemed tired and thin, and now she might even be dehydrated . At the doctor’s office, Doctor Rashet looked over Rianne’s history. “I see you just had your Meningitis vaccine at the end of last year, and you are due for a tetanus shot. Also, your sister had hand foot and mouth disease a few weeks ago, did anyone else in your family have that?”
Rianne made a face and held out her hands to examine the palms. “I don’t think so, but Dad definitely got it. It was funny.” Doctor Rashet nodded and took out what looked like a small phone. The doctor explained: "This is a glucose meter, want to check something in your blood, so I need to prick your finger."
Rianne was wary about the device. "What's glucose?" The doctor explained that it's a fancy name for sugar.
Reluctantly , Rianne held out her hand and the doctor used a lancet to prick the tip of her finger. A little drop of blood was placed on the device and then a digital readout was displayed. The number on the display said 260 mg/dL. "Your blood sugar is really high. It could mean you have diabetes, or it could mean that you just ate a donut. I think we’re going to need to do further tests".
Rianne didn’t really know what that meant, but she did not like the worried look on her mother’s face. By the end of the day, they had a definite diagnosis, Rianne had type 1 diabetes and would need to take insulin injections.
Relieved to have a diagnosis but concerned about her daughter’s health, Rianne’s mother spent time looking on the internet to find out more about the disease. She didn’t understand how Rianne got this disease, or even if diabetes was hereditary ; no one in her family has diabetes. Were her other children at risk too?
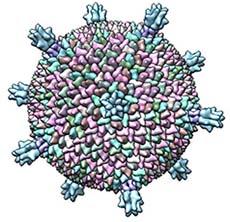
1. What were Rianne’s symptoms?
2. Why was the reading on the glucose meter concerning? What would be a normal level of blood glucose?
3. For each underlined word in the passage, write a short annotation for what the word means.
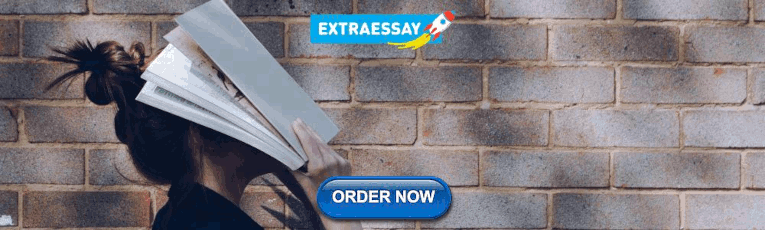
Part B: What is Type 1 Diabetes?
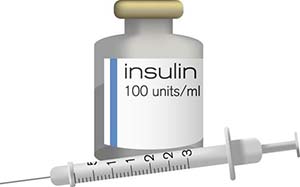
Type 1 diabetes is characterized by abnormally high blood sugar levels. Specialized cells in the pancreas called beta cells are responsible for producing insulin, which controls the uptake of sugar from the blood into the cells. Without this conversion, cells are deprived of the energy from glucose. Without treatment, the individual will lose weight and suffer damage to the brain and other tissues. Treatment involves injecting insulin daily so that cells can absorb glucose and the patient must constantly monitor their blood sugar levels.
Insulin dependent diabetes mellitis (IDDM) is considered an autoimmune disorder. Autoimmune disorders occur when the immune system attacks the body’s own cells and organs. Beta cells of the pancreas are damaged by the immune system and no longer function to create insulin. The reasons for the immune system malfunction are not fully understood and may be the result of genetic differences or exposure to something in the environment, or a combination of those two things.
There is no known “diabetes” gene, but those with certain genetic sequences may be more likely to develop the disease. Scientists have recently noted a connection between diabetes and the exposure to a virus.
Coxsackieviruses are nonenveloped viruses with linear single-stranded RNA and are divided into group A and group B. Group A coxsackievirus tend to infect the skin and can cause hand foot and mouth disease. Group B tend to infect the heart and liver. As with many viral infections, symptoms may not appear right away or may never appear, the infection silent and mostly unnoticed.
Some physicians have noted that children who get infected with this virus sometimes develop type 1 diabetes. Is there a causal relationship?
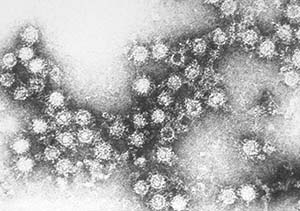
4. Consider what you know about cells. What structure of the beta cells of the pancreas are most likely affected by the immune system? Provide reasoning for your choice.
5. Develop a hypothesis related to diabetes and virus exposure. Remember this should be a testable statement.
6. Consider ways you could test this hypothesis. For ethical reasons, you cannot deliberately infect children with the virus. Describe your research method.
Part B – Collecting Data
Given that it would be unethical to purposely infect children with a virus that might cause a life-threatening illness, researchers have developed a way the hypothesis. They look at the risk of developing the disease if you have been exposed to the factor in question. For example, doctors looked at smokers and nonsmokers and found the risk for developing lung cancer was much higher in smokers. In this case, the factor they are investigating is the exposure to the Coxsackievirus B and whether that increases the risk of diabetes. There are two methods for conducting this type of study:
- Case Control Studies examine a series of patients who have a disease and a control group that does not have the disease. Researchers compare the proportion of each group with their exposure to the factor in question.
- Cohort Studies classify subjects based on the presence or absence of exposure to the factor and then follow the subject for a period of time to determine if they develop the disease in question.
The raw data tables obtained from a physician records include a variety of information. Some may not be that useful for the case. Examine the raw data on the next page. *Note: The presence of Coxsackie B antibodies indicates that the patient was exposed to the virus at some point.
7. Scan the data, why do you think sibling information is included?
8. Why do you think that onset of IDDM is included? What is the average age of onset?
9. Based on the data table, would you characterize this as a case study or a cohort study? Why?
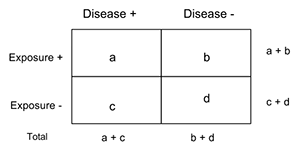
10. To analyze data, a two-by-two table is used to compare the exposure to the factor and the presence of the disease. Create this table to organize data. .
11. The odds ratio is the ratio of odds of exposure among cases to the odds of exposure among controls. For example, an odds ratio of 3.4 would indicate that those individuals with exposure to the factor in this study were 3.4 times more likely to get the disease than individuals without exposure. Calculate the odds ratio for your data. Calculate the odds ratio (OR). OR = (ad) / (bc)
12. Conclusions: Use the CER method (claim-evidence-reasoning) to state a claim regarding the question of whether virus exposure can result in the onset of juvenile diabetes.
|
|
|
|
|
1 | + | - | - | |
2 | + | + | + | 11 |
3 | - | + | - | |
4 | + | + | + | 9 |
5 | - | + | - | |
6 | - | + | + | 8 |
7 | - | - | - | |
8 | + | + | - | |
9 | - | + | + | 10 |
10 | - | + | + | 7 |
11 | + | + | + | 12 |
12 | - | - | - | |
13 | + | + | + | 8 |
14 | - | + | + | 9 |
15 | - | - | + | 8 |
16 | - | - | - | |
17 | - | + | + | 11 |
18 | + | + | - | |
19 | - | + | + | 10 |
20 | - | + | - | |
21 | + | - | - | |
22 | + | + | + | 9 |
23 | - | - | - | |
24 | - | + | + | 8 |
25 | + | + | + | 11 |
26 | - | + | - | |
27 | + | - | - | |
28 | - | - | - | |
29 | - | + | + | 7 |
30 | + | + | + | 12 |
31 | - | + | + | 8 |
32 | + | - | - | |
33 | - | - | - | |
34 | + | + | + | 10 |
35 | - | + | - | |
36 | - | + | + | 9 |
Component | Level 3 | Level 2 | Level 1 | Level 0 |
Claim | Claim answers the question, is accurate, and is complete. Completely describes the trend in the relationship between two variables. | Claim does answer the question but it is inaccurate or incomplete. | Claim does not answer the question. | Does not make a claim. |
Evidence | Provides appropriate and sufficient evidence to support the claim. Summarizes data. | Provides appropriate, but insufficient evidence to support the claim. May include some inappropriate evidence. | Evidence does not support the claim; only provides inappropriate evidence. | Does not provide evidence. |
Scientific | Includes logic statements that link the claim, evidence and science concepts (including words such as ‘because…’ ‘therefore…’) that clearly demonstrates logical reasoning. | Attempts to include a logic statement that links the evidence to the claim but does not adequately link the evidence to the claim. | Restates evidence or claim and does not include a logic statement that links the evidence to the claim. | Does not include scientific reasoning. |
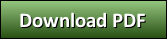
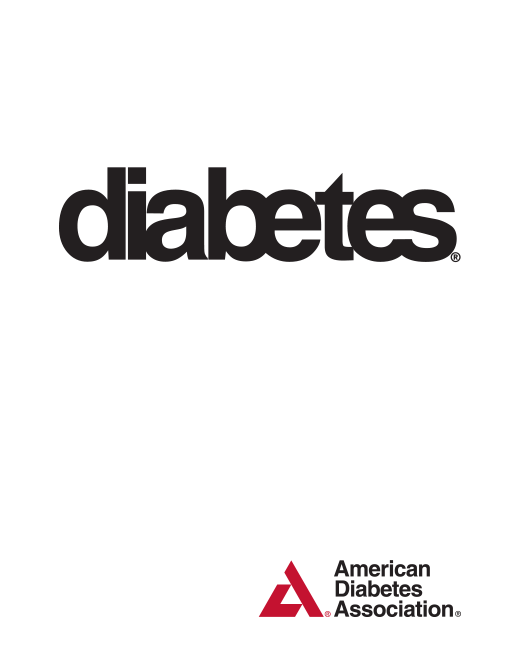
- Next Article
INSIGHT FROM EPIDEMIOLOGY AND CLINICAL INVESTIGATIONS
Insight from experimental work, clinical implications: do viral infections induce or prevent type 1 diabetes, article information, viral trigger for type 1 diabetes : pros and cons.
- Split-Screen
- Article contents
- Figures & tables
- Supplementary Data
- Peer Review
- Open the PDF for in another window
- Cite Icon Cite
- Get Permissions
Christophe M. Filippi , Matthias G. von Herrath; Viral Trigger for Type 1 Diabetes : Pros and Cons . Diabetes 1 November 2008; 57 (11): 2863–2871. https://doi.org/10.2337/db07-1023
Download citation file:
- Ris (Zotero)
- Reference Manager
The most popular hypothesis circulating within and beyond the scientific community is that viral infections enhance or elicit autoimmune disorders such as type 1 diabetes. Indeed, viruses can injure β-cells and have been isolated in pancreatic tissues from diabetic patients. However, accumulating evidence suggests that the opposite scenario, which is prevention or amelioration of type 1 diabetes, might be at least as common an outcome of viral infection. Here, we discuss epidemiological and experimental evidence for the main mechanisms accounting for the role of viruses in type 1 diabetes to better understand the complex relationship between viral infections and autoimmune diabetes.
The influence of the environment.
Type 1 diabetes is a genetic autoimmune disorder caused by autoreactive CD4 + and CD8 + T-cells that recognize pancreatic antigens such as insulin or GAD and subsequently destroy insulin-producing β-cells. The subject of very active research is the question of how endogenous β-cell antigens become immunogenic. Infiltration of the islets of Langerhans, where β-cells reside, by activated autoreactive T-cells is considered to be the major driving force in type 1 diabetes progression. The islet infiltrate in humans consists primarily of CD8 + T-cells and B-cells, followed by macrophages and dendritic cells of different subtypes ( 1 ). Interestingly, significantly fewer T-cells are found in human islets compared with islets from nonobese diabetic (NOD) mice. The reduced numbers of T-cells, and in this way a limited autoreactive component in human islets, leads one to consider whether other contributing factors may be involved in disease development. Otherwise, sufficient insulitic infiltrate to destroy islet β-cells might not be easily maintained in humans. Further supporting a role for nongenetic factors in the control of type 1 diabetes is the observation that disease concordance among monozygotic twins is below 50% ( 2 ). Migrant studies also suggest the involvement of an environmental factor in type 1 diabetes, since disease incidence in migrating populations appears to conform to the incidence of the region to which there is migration ( 3 ). There is an ever-increasing body of literature suggesting that the significant environmental component to type 1 diabetes development and progression is a viral infection. However, this has not been clearly demonstrated. In fact, viral infections appear to have both detrimental and protective effects on type 1 diabetes development, which might be contingent upon the nature of the virus, but also the immune status of the host and thus the timing of infection.
Certain viruses might promote autoimmunity.
A significant number of viruses have been associated with type 1 diabetes, including enteroviruses such as Coxsackievirus B (CVB) ( 4 ), but also rotavirus ( 5 , 6 ), mumps virus ( 7 ), and cytomegalovirus ( 8 ). Rubella virus has been suggested to cause type 1 diabetes, but so far only congenital rubella syndrome has conclusively been associated with the disease ( 9 – 11 ). The prime viral candidates for causing type 1 diabetes in humans are enteroviruses. Enterovirus infections are more frequent in siblings developing type 1 diabetes compared with nondiabetic siblings, and enterovirus antibodies are elevated in pregnant mothers whose children later develop type 1 diabetes ( 12 ). Interestingly, studies in the Finnish population demonstrated that appearance of autoantibodies in genetically susceptible children paralleled the seasonal pattern of enterovirus infections ( 13 ). More specifically, a temporal association has been observed between the appearance of the first autoantibodies and signs of enterovirus infection both among siblings of affected children and among children with increased HLA-conferred diabetes susceptibility ( 14 ).
CVB4 is the most common enteroviral strain found in pre-diabetic and diabetic individuals. CVB RNA has been detected in blood from patients at the onset or during the course of type 1 diabetes ( 15 , 16 ). Furthermore, cellular immune responses to CVB antigens were found to be enhanced in type 1 diabetic patients after the onset of the disease ( 17 ). One CVB4 strain was isolated from the pancreas of a deceased diabetic child, passaged through murine β-cells, and found to induce diabetes after inoculation in mice ( 18 ). Recently, Dotta et al. ( 19 ) also detected CVB4 in pancreatic tissue specimens from three of six type 1 diabetic patients. Elshebani et al. ( 20 ) recently found that enterovirus isolates obtained from newly diagnosed type 1 diabetic patients could infect and induce destruction of human islet cells in vitro. Recently, Oikarinen et al. ( 21 ) have isolated enteroviruses from intestinal biopsy samples in 75% of type 1 diabetes cases versus 10% of control patients, possibly reflecting persistent enterovirus infection of gut mucosa in type 1 diabetic patients. In sum, isolation of enteroviral antigens from diabetic individuals, particularly after recent onset, is becoming a fairly reproducible finding, supporting a role for these viruses in disease development. However, it is still unclear whether this phenomenon is indeed a common etiology for the majority of patients diagnosed with type 1 diabetes, or whether it can be found only in a particular subpopulation of individuals with perhaps higher genetic susceptibility to infection.
The molecular means by which enteroviruses could enhance type 1 diabetes is a topic of significant inquiry. Virus infections activate strong immune responses. CVB4 infection of islet cells was indeed reported to induce strong inflammation mediated by natural killer (NK) cells within the islets ( 19 ). If the virus can, in addition, promote direct cytolysis of β-cells, autoantigens are introduced in a context of heightened immune response and inflammation. This might be the case after direct infection of β-cells by the virus. Enteroviruses might target β-cells via surface molecules such as the poliovirus receptor and integrin α v β 3 . Both of these molecules are expressed on human β-cells and can act as enterovirus receptors in established cell lines ( 22 ). Infections by viruses that target β-cells and promote strong inflammation within the islets may thus represent the initial step in the induction of autoimmunity. However, studies on human pancreata or cultured islets have shown that there are considerable variations in the adverse effects of enteroviruses on β-cells, not only between various viral serotypes, but also between strains of the same serotype ( 23 – 25 ). While the mechanism by which viruses might induce autoimmunity is not understood, viral infections might be capable of “unmasking” β-cells for recognition by CD8 + T-cells by promoting interferon production and upregulation of major histocompatibility complex (MHC) class I molecules on β-cells. These events combined may be sufficient to condition the pancreatic islets for autoimmune attack. In this respect, Foulis et al. ( 26 ) described MHC class I upregulation and interferon induction in noninfiltrated islets obtained from presumably pre-diabetic individuals. Although this may possibly be a postmortem artifact, such findings should prompt larger-scale investigations, for example, on freshly obtained tissues via nPOD (Network for Pancreatic Organ Donors with Diabetes; www.jdrfnpod.org ). Access to fresh pre-diabetic pancreata is needed to conduct valid viral studies.
Viruses may be wrongly accused.
The possibility that enteroviruses promote autoimmunity suggests that vaccination, which will impair viral infection of the islets, may provide protection against type 1 diabetes. Accordingly, an earlier report suggested that lower type 1 diabetes incidence in Estonia compared with Finland may be associated with polio vaccination schedule, resulting in stronger immunity to diabetogenic enterovirus infections ( 27 ). Similarly, while a significant increase in the number of type 1 diabetes cases was observed in Finland 2–4 years after a mumps epidemic ( 7 ), the incidence of type 1 diabetes reached a plateau 6 years after introduction of the mumps-measles-rubella vaccine ( 28 ). However, there is significant epidemiological data contradicting the involvement of viruses as causative agents in type 1 diabetes. There exists a geographical north-south gradient indicative of an inverse correlation between “hygiene” and incidence of autoimmune disease (as well as allergy). Countries such as Finland versus Venezuela/China, or wider regions such as Northern versus Southern Europe (with the exception of Sardinia), represent areas where socioeconomics correlate closely with type 1 diabetes prevalence. Reduced type 1 diabetes incidence is observed in countries of lower socioeconomic status, which is associated with a higher rate of infection. This phenomenon may also be related to the use of particular vaccine strategies in countries exhibiting different sanitary standards. It is also interesting to note that many type 1 diabetic patients are first born of large families, possibly indicative of lower exposure to infections. In addition, while congenital infections have been proposed to account for type 1 diabetes development in the offspring, the use of antimicrobials by mothers before pregnancy and subsequently by the child was suggested to be associated with higher risk for type 1 diabetes ( 29 ). Increased diabetes incidence in the Western world may thus be reflective of the “ultra-clean living” phenomenon. In the “hygiene hypothesis,” reduced rates of infection contribute to increased type 1 diabetes incidence, not supporting a disease-inductive role for viruses. Alternatively, it has been argued that reduced frequencies of infection may result in increased susceptibility to the effect of diabetogenic viruses ( 30 , 31 ). Regardless, although there is significant evidence for viral penetration of pancreatic tissue from type 1 diabetic patients, exposure to viruses does not appear to be necessarily causative of type 1 diabetes and may in fact be beneficial in some cases. This could be an indication that the immune system can be educated to better deal with inflammatory disorders by being frequently exposed to inflammatory events over life.
While epidemiological studies have shed important insight into the association between viral infections and autoimmune diabetes in humans, a significant body of evidence is derived from investigations using animal models for type 1 diabetes. Notably, NOD mice are susceptible to spontaneous type 1 diabetes that develops slowly over several weeks and mimics most aspects of human type 1 diabetes ( 32 ). In NOD mice, nondestructive insulitis develops in the pancreas during the pre-diabetic phase and, although this period is variable, most mice go on to develop T-cell–mediated destruction of β-cells leading to overt diabetes. NOD mice thus constitute a critical tool to address how exposure to viral infection during the pre-diabetic phase will affect subsequent disease development. In humans, the prime candidates for infectious causes of type 1 diabetes are enteroviruses such as CVB. In mice, and most notably in NOD mice, CVB also appears associated with the development of autoimmunity. Early studies have shown that infection of normal mice with CVB4 causes a diabetic state associated with low insulin levels consistent with islet cell destruction ( 33 ). CVB4 has since been shown to be tightly associated with the initiation of type 1 diabetes in the NOD mouse. However, the influence of the virus appears to be contingent upon the precise point in time at which infection occurs ( 34 ). The B3 strain of CVB3, in contrast, mediates significant protection against type 1 diabetes development in NOD mice regardless of the time of infection ( 35 ). However, as discussed below, CVB3 and CVB4 differ regarding tropism for pancreatic tissue, which may account for the differential effect of these two stains in autoimmune diabetes. Importantly in that respect, there might be fundamental difference between rodents and humans regarding tropism of enteroviruses for pancreatic β-cells. One should thus be cautious when extrapolating rodent data to human type 1 diabetes in the context of enterovirus infections. Interestingly, other viruses that are also thought to be associated with the pathogenesis of type 1 diabetes in animals have been shown to mediate protective effects in some instances. For example, the D variant of encephalomyocarditis virus (EMC-DV) was found to induce β-cell destruction and subsequent type 1 diabetes in the mouse, but this was found to occur in a T-cell–independent fashion. EMC-DV was also reported to diminish autoimmunity in diabetes-prone NOD mice ( 36 – 38 ). In the BioBreeding diabetes-resistant (BB-DR) rat, however, it was shown that infection with Kilham's rat virus induces autoimmune diabetes ( 39 ). But while the use of animals has clearly established that particular viruses are capable of inducing autoimmune diabetes, it has also evidenced that these viruses can play a preventive role in the development of the disease. Two different, yet non–mutually exclusive, mechanisms have been proposed to account for the causative role of viruses in type 1 diabetes. Detailed insight into these mechanisms could provide better understanding of the dual roles played by viral infections in autoimmune diabetes.
Molecular mimicry might enhance but not initiate autoimmunity.
One of the two mechanisms by which viruses may be capable of initiating type 1 diabetes is termed molecular mimicry. If the T-cell receptors expressed by particular autoreactive T-cells enable these cells to recognize viral antigens (or vice versa), both autoreactive and antiviral T-cells have the potential to become activated as a consequence of presentation of viral antigens by antigen-presenting cells (APCs). Activation of autoreactive T-cells upon CVB4 infection was proposed to occur by molecular mimicry, since the P2-C protein sequence of the virus partially resembles that of human GAD, a protein expressed in the islets as well as other nervous tissues ( 40 ). T-cells from patients at risk for type 1 diabetes were found to recognize a GAD determinant that shares significant sequence similarity with the P2-C protein of CVB4, and patients whose T-cells responded to this particular GAD determinant were found to also respond to a Coxsackie viral peptide ( 41 ). However, antibodies present in GAD-positive sera from patients with type 1 diabetes were not found to cross-react with P2-C ( 42 ). Cross-reactivity between GAD and P2-C was further assessed using the NOD mouse model for type 1 diabetes, where it was determined that the common region of these two proteins is immunodominant and presented to cross-reactive T-cells only in the context of a NOD diabetes susceptibility MHC allele ( 43 ). Cross-reactivity between P2-C and GAD was thus proposed to account for the capacity of CVB4 to induce type 1 diabetes in genetically predisposed humans. However, Horwitz et al. ( 44 ) found that congenic B10.H2 g7 mice, which carry the NOD MHC allele but lack other type 1 diabetes susceptibility factors, do not develop diabetes upon CVB4 infection. Moreover, infection of BDC2.5 transgenic mice, which express a T-cell receptor specific for an islet antigen that does not cross-react with the CVB4 P2-C protein was able to induce type 1 diabetes in the majority of these otherwise non–diabetes-prone mice. Therefore, cross-reactivity between P2-C and GAD may not by itself account for initiation of type 1 diabetes but might act as an essential enhancer of disease once autoimmune attack of β-cells has been initiated.
The rat insulin promoter (RIP)–lymphocytic choriomeningitis virus (LCMV) system is a mouse model in which diabetes is initiated by viral infection ( 45 – 47 ). In this model, RIP-LCMV mice transgenically express the glycoprotein or nucleoprotein of LCMV as a target antigen in their islets under the control of the RIP. Infection of these mice with LCMV breaks peripheral responsiveness to glycoprotein/nucleoprotein, leading to attack of β-cells by T-cells and eventual development of type 1 diabetes. Importantly, this model suggests that viral infection is able to induce autoimmunity only if homology between viral and β-cell antigens is 100%, since a single amino acid change flanking a cytotoxic T-lymphocyte (CTL) epitope was found to interfere with the development of type 1 diabetes ( 48 ). These data further support the hypothesis that molecular mimicry alone might not be capable of inducing type 1 diabetes but rather be an essential precipitator once autoimmunity has been initiated. Indeed, we previously reported that an LCMV mimic ligand can accelerate preexisting autoimmunity by inducing autoreactive T-cells to proliferate and localize in the islets, yet does not generate sufficient autoreactive T-cells to initiate disease in naive mice ( 49 ). We propose that viral infections alone will not initiate autoimmunity but rather act to provide a “fertile field” for further expansion of activated autoreactive T-cells, leading to autoimmune disease ( 50 ).
Bystander mechanisms induce APC activation and initiate autoimmunity.
While molecular mimicry may enhance autoimmune responses, the central role in induction of autoimmunity by viruses might be played by the proinflammatory/inflammatory mediators produced upon infection. Hence, bystander activation of autoreactive T-cells could occur during a viral infection with heterologous antigenic specificity and result in autoimmune disease. This might be the consequence of inflammation inducing tissue damage and release of sequestered islet antigens, leading to enhanced autoantigen presentation by APCs. Accordingly, CVB4-induced type 1 diabetes was found to be associated with initial phagocytosis of CVB4-infected β-cells by macrophages, leading to increased presentation of islet antigens, which promoted type 1 diabetes ( 51 ). Limited injury to β-cells using the islet-damaging agent streptozotocin was found to induce type 1 diabetes in BDC2.5 mice similar to CVB4 infection, as a consequence of release of β-cell antigens followed by their presentation by macrophages ( 52 ). Of note, injection of insulin along with poly-IC (polyinosinic-polycytidylic acid), which mimics double-stranded viral RNA, was found to induce anti-islet autoimmunity ( 53 ). Thus, virally induced release of β-cell antigen under inflammatory conditions might promote type 1 diabetes through activation of APCs such as macrophages, which have been shown to play a crucial role in the development of spontaneous diabetes ( 54 , 55 ). In particular, APCs themselves can release inflammatory and proinflammatory mediators as a result of viral infection. Likewise in the RIP-LCMV model, in the absence of infection, the glycoprotein/nucleoprotein protein is not expressed by costimulation-competent APCs, and the autoimmune process is only initiated when sufficient numbers of activated autoreactive T-cells are generated, after activation of APCs in the pancreas ( 56 , 57 ). Interestingly, activated T-cells from BDC2.5 mice are unable to induce diabetes in the mouse in the absence of CVB4 infection, which supports the possibility that bystander activation of APCs through virally mediated inflammation is necessary for efficient activation of autoreactive T-cells ( 44 ). Accordingly, we reported that type 1 diabetes develops in the absence of infection in the RIP-LCMV system when APCs are rendered costimulation-competent through transgenic expression of the B7–1 (CD80) costimulatory molecule ( 58 ). Importantly, in the RIP-LCMV system, APCs presenting self-antigens not only mediate priming of autoreactive T-cells, but also help maintain a peripheral immune response in the pancreatic islets ( 59 ). Thus, antigen presentation appears to play a crucial role not only in inducing but also sustaining the diabetogenic response.
Viral infection and local β-cell injury.
Initial damage to β-cells and uptake of autoantigen by APCs appear crucial in the initiation of autoimmunity upon viral infection. If a particular virus is highly lytic for β-cells, insulin deficiency and type 1 diabetes will result when more than 90% of β-cells are destroyed ( 60 , 61 ). This scenario can notably be observed after high-dose infection with EMC-DV, in which case type 1 diabetes is nonautoimmune in nature ( 62 , 63 ). However, limited injury to β-cells, caused by the virus or antiviral mechanisms, can lead to initial release of sequestered self-antigens and ultimately their presentation by APCs, which in turn promote further β-cell damage by activating autoreactive T-cells. Inflammatory cytokines produced during viral infection may play a crucial role in initial destruction of β-cells. Using the RIP-LCMV model, we found that upon viral infection, systemic production of interferon (IFN)-γ can directly cause β-cell destruction in the islets ( 64 ). Furthermore, inflammatory cytokines such as type I and II interferons may indirectly contribute to β-cell death by inducing upregulation of MHC class I by these cells, thereby “unmasking” them for recognition by autoreactive T-cells. It was reported that immunization with an LCMV-derived peptide does not induce type 1 diabetes in RIP-LCMV mice in the absence of IFN-α production and MHC class I upregulation in the islets ( 65 ). Furthermore, our work has shown that upregulation of MHC class II and activation of APCs within the pancreatic islets is required for β-cell destruction by activated autoreactive T-cells ( 56 ). Thus, cytokines produced upon infection by viruses exhibiting pancreatic tropism might be capable of preconditioning the islets for initial autoimmune attack of β-cells, similar to the possible scenario in humans.
Studies in the mouse further indicate that activation of Toll-like receptor (TLR) signaling might play a crucial part in the process. TLR ligation was shown to induce type 1 diabetes by increasing IFN-α and MHC class I expression in the islets of RIP-LCMV mice immunized with an LCMV-derived peptide ( 65 ). Similarly, in the BB-DR rat, initiation of autoimmune diabetes by Kilham's rat virus was found to be enhanced through activation of TLR signaling and induction of inflammatory cytokine production by APCs ( 66 , 67 ). More recently, Kim et al. ( 68 ) reported that activation of the TLR2 signaling pathway by secondary apoptotic β-cells might participate in the initiation of type 1 diabetes by inducing tumor necrosis factor-α (TNF-α) production by macrophages. However, TLR2 signaling was also recently suggested to enhance immune regulation ( 69 ), and it was previously reported that limited apoptosis of β-cells decreases diabetes incidence in NOD mice ( 70 ). In addition, as discussed below, TNF-α may have a protective function in type 1 diabetes depending on the time of action. Yet, regardless of the underlying mechanism, the observation by Kim et al. suggests that the mode by which initial injury to β-cells occurs is a crucial determinant in the induction of autoimmunity. Therefore, direct viral tropism for β-cells could play a major role in the capacity of viruses to mediate type 1 diabetes. Of note, CVB4 has a direct tropism for β-cells and exhibits a differential effect in type 1 diabetes depending on the time of infection, whereas CVB3 infects the acinar cells of the exocrine pancreas and prevents disease regardless of the time of infection ( 34 , 35 ). Although CVB4 infection of β-cells may not directly cause their demise ( 51 ), CVB3 and CVB4 do not mediate similar injury to these cells, which may account for their differential role in type 1 diabetes ( 68 ). Modulation of autoimmune diabetes by viruses may thus depend in part on their capacity to influence the mode and extent of β-cell death, which both appear as crucial factors influencing the course of the disease ( 71 ).
The importance of timing.
Another major component determining virally mediated modulation of autoimmunity appears to be the time at which infection occurs during the pre-diabetic phase. Whereas type 1 diabetes is enhanced in 8-week-old NOD mice infected with CVB4, infection of younger mice has no effect on disease outcome ( 34 ). This suggests that the status of autoimmune progression is a crucial determinant in the diabetogenic potency of the virus. Importantly, just like viral infections, inflammatory cytokines appear to play a dual role in autoimmune diabetes. Previous work has shown that expression or neutralization of cytokines commonly produced during viral infections has opposing effects on type 1 diabetes outcome depending on the time of expression. For instance, we found that early neutralization of TNF-α abrogates type 1 diabetes in RIP-LCMV mice, while at later time points, this cytokine appears to play a beneficial role by diminishing the number and activity of autoreactive T-cells ( 72 , 73 ). Thus, the capacity of particular viral infections to modulate autoimmunity at a certain point in time might be the direct consequence of their ability to promote inflammation during the pre-diabetic process beyond a particular autoimmune threshold. Accordingly, it was reported that enhancement of diabetes by CVB4 infection occurs only after a critical mass of activated autoreactive T-cells has accumulated in the islets ( 74 ). However, CVB3 was reported to prevent disease in both young and older mice ( 35 ). This suggests that while timing is important, the state of advancement of autoimmunity at the time of infection is not the sole explanation for the dual role of viruses in type 1 diabetes.
Impairment of autoimmunity may occur through bystander effects.
APC activation and associated inflammation, whether or not induced by viral infection, may not always have detrimental consequences in autoimmune diabetes. As discussed above, epidemiological studies provide evidence that infectious events occurring during early childhood might have the ability to prevent or delay type 1 diabetes development ( 75 ). The ability of viral infections to abrogate autoimmune diabetes was also reported in different animal models using not only CVB3 ( 35 ), but also LCMV ( 46 , 76 , 77 ), EMC-DV ( 38 ), mouse hepatitis virus ( 78 ), and lactate dehydrogenase virus ( 79 ). Interestingly, both acute and persistent viral infections appear capable of modulating the immune system in a diabetes-preventive fashion. While the mechanisms accounting for the beneficial effect of viruses on the immune system are poorly understood and may vary from one individual to the next (or one mouse model to the next), a feature common to viral infections is their paradoxical capacity to induce inflammation. This is also the case for a number of nonviral infections, vaccines, or treatments reported to protect NOD mice against diabetes ( 80 – 89 ). In fact, in some cases, type 1 diabetes can be inhibited by direct treatment of pre-diabetic mice with proinflammatory or inflammatory cytokines such as type I interferons, IFN-γ, or interleukin-2 ( 81 , 90 – 93 ), or by inducing the production of similar factors via stimulation of innate immunity ( 81 , 82 , 85 , 94 ). In the RIP-LCMV system, although viral infection represents the event initiating autoimmunity and diabetes, we have shown that inflammation mediated upon viral challenge can prevent type 1 diabetes. Our results indicate that secondary infection of RIP-nucleoprotein mice with a different strain of LCMV during the pre-diabetic phase completely abrogates diabetes development ( 95 ). This phenomenon is dependent on IFN-γ and TNF-α production and results from the recruitment of activated T-cells away from the islet infiltrate toward the pancreatic draining lymph node, as a consequence of selective expression of the chemokine IP-10 (CXCL10). In these studies, the LCMV strain used to prevent diabetes shares a homologous nucleoprotein sequence with that used to initiate diabetes, and thus the two infections activate comparable T-cell responses. Therefore, inflammatory cytokines and chemokines produced during viral infection might play a crucial part in controlling the location of virally activated autoreactive T-cells and their subsequent capacity to infiltrate pancreatic islets.
Antiviral T-cell memory and autoimmune diabetes.
Previous reports suggest that part of the T-cells activated during viral infection can cross-react with new infectious agents or allo-antigens and modulate immunity to unrelated antigens ( 96 – 98 ). Consequently, such heterologous immunity might result in the accumulation of memory T-cells of specificity unrelated to the original viral agent ( 99 ). It is thus possible that the memory T-cell pool generated during life comprises autoreactive/cross-reactive T-cells induced nonspecifically as a consequence of cumulative or chronic viral infections. Since stimulated memory T-cells respond to antigen more rapidly and efficiently than naive cells, repeated or sustained antiviral immunity during life may eventually favor autoimmunity. While in most cases, this phenomenon will not result in autoimmune disease, it might be a prerequisite for overt diabetes in genetically predisposed individuals. As discussed above, in different mouse models, initiation of diabetes by viral infection requires a critical mass of autoreactive T-cells along with activated APCs ( 34 , 56 – 58 , 100 ), and it is possible that, in humans, such a mass is provided progressively over life by repeated or sustained viral infections. In other words, autoimmunity might not be induced de novo at type 1 diabetes onset, and the autoreactive T-cell pool is likely comprised of cells that have already responded to antigenic stimulation during viral infection in the past. On the other hand, previous work suggests that repeated or sustained encounters with viral antigen during chronic infection is associated with protection against type 1 diabetes ( 46 , 77 ). This may be due in part to exhaustion of T-cell immunity, which is commonly found in chronic viral infection and was notably reported in protracted LCMV infection ( 101 ). Alternatively, or in addition, diabetes abrogation during chronic viral infection may be the consequence of virally induced regulatory mechanisms suppressing antiviral immunity and possibly autoimmunity as well ( 102 , 103 ). In this respect, a number of chronic as well as acute infections have been reported to induce the activation of regulatory T-cells (Tregs), in particular naturally occurring Tregs (CD4 + CD25 + Tregs), which are known to play a crucial role in the control of autoimmunity ( 104 – 108 ). Thus, repeated exposure to viral antigen during life may not necessarily be pathogenic in autoimmunity. Nonspecific activation of autoreactive T-cells as a consequence of repeated or protracted viral infections may even be beneficial in some cases. Notably, CD4 + CD25 + Tregs, which are selected in the thymus and thought to react to self-antigens in the periphery ( 109 , 110 ), can in essence be considered “autoreactive” and may thus be beneficially activated in heterologous immunity. In fact, we found that CD4 + CD25 + Tregs are modulated during viral infection and become capable of halting the course of type 1 diabetes (C.M.F., unpublished data). In addition, our results suggest that resemblance between viral and β-cell antigens can, in some cases, promote activation of diabetes-preventive CD4 + CD25 + Tregs.
A number of epidemiological studies support the hypothesis that viral infections play a causative role in type 1 diabetes. However, systematic review of control studies published between 1966 and 2002 has shown no convincing evidence for or against an association between type 1 diabetes and the prime candidate for infectious cause, CVB ( 111 ). In animal models for type 1 diabetes, solid evidence supporting an inductive role for viruses is faced with just as solid evidence supporting a protective effect of viral infections. Based on mouse studies alone, there is no doubt that association between viruses and type 1 diabetes is extremely complex: while belonging to the same enteroviral group, CVB3 and CVB4 have opposing effects on type 1 diabetes in the same mouse model; LCMV initiates diabetes in the RIP-LCMV model but prevents disease in the NOD model; and to make matters more complicated, CVB4 and LCMV are capable of both inducing and preventing diabetes in the same mouse model depending on the time of infection. Thus, the reason for current failure to associate a particular virus with induction of autoimmune diabetes likely is that such an association might be impossible to make. Certain viruses might be capable of inducing diabetes and others of preventing diabetes, and type 1 diabetes inducers might be capable of preventing disease under certain conditions. This will depend of course on the nature of the considered virus (resemblance with β-cell antigens; tropism for β-cells; induction of a chronic infection), but also on the state of advancement of autoimmunity at the time of infection (generation of sufficient numbers of autoreactive T-cells; nature of the cytokine milieu systemically and in the islets). A given viral infection could thus be an essential disease precipitator once required predisposing events have occurred, but could on the other hand disrupt accumulation of these events.
Most important is the indication from animal studies that modulation of autoimmunity during viral infection does not depend merely on inherent properties of the virus, but also significantly on intrinsic factors of the host. The close interplay between the two will dictate whether enhancement or abrogation of autoimmune diabetes occurs. While molecular mimicry might activate autoreactive T-cells, it could also segregate these cells away from the islets and/or induce the activation of protective Tregs. While inflammatory cytokines might promote bystander activation of APCs and autoreactive T-cells, infection could occur at a time where inflammation will induce the relocation or demise of these cells. Whereas β-cell lysis and presentation of islet antigen might promote activation of autoreactive T-cells, it could also suppress the function of these cells by promoting Treg activity. Whereas repeated/sustained infections might lead to the accumulation of autoreactive T-cells within the memory pool, they could also induce suppressor mechanisms that will hinder autoimmunity. These possibilities are illustrated in Fig. 1 .
Based on current evidence, it thus appears impossible to assess the capacity of viruses to modulate type 1 diabetes without knowledge of the state of advancement of autoimmunity and infection history of affected individuals. This is no easy task, but tremendous effort is currently being made in the U.S. and Europe to closely monitor exposure to infections in individuals at risk for type 1 diabetes. In particular, the TEDDY (The Environmental Determinants of Diabetes in the Young) study is currently assessing the influence of environmental factors, among which are viral infections, on the development of autoimmune diabetes. In this study, blood from children with increased genetic risk for type 1 diabetes is assessed for viral exposure every 3 months for the first 4 years of life, and then every 6 months until the age of 15 years. Stool samples are also assessed for viral exposure at monthly intervals for the first 4 years of life and then biannually until the age of 15. Importantly, as the period of time between a particular viral infection and the initiation of autoimmunity appears variable, occurrence of a possibly critical infectious event might be extremely hard to detect. It thus appears crucial that children with increased genetic risk for type 1 diabetes are monitored not only at regular intervals, but also whenever they are undergoing a viral infection. Closer monitoring of individuals with high risk for type 1 diabetes should give us more convincing evidence for the contribution of an infectious agent to progression toward autoimmunity. In addition, newly obtained mechanisms from experimental investigations will be useful for the development of novel immunotherapy for type 1 diabetes.
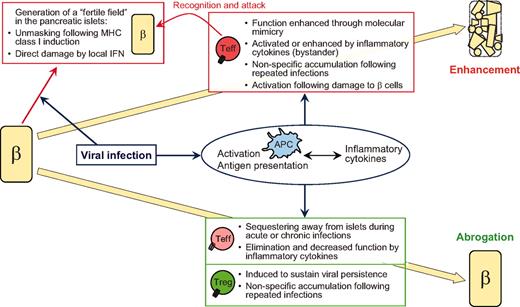
Interplay between virus- and host-intrinsic properties dictates whether enhancement or abrogation of type 1 diabetes occurs. β = β cells. Teff, effector (autoreactive) T-cells.
Readers may use this article as long as the work is properly cited, the use is educational and not for profit, and the work is not altered. See http://creativecommons.org/licenses/by-nc-nd/3.0/ for details.
The authors acknowledge support from the Brehm Coalition.
Email alerts
- Online ISSN 1939-327X
- Print ISSN 0012-1797
- Diabetes Care
- Clinical Diabetes
- Diabetes Spectrum
- Standards of Medical Care in Diabetes
- Scientific Sessions Abstracts
- BMJ Open Diabetes Research & Care
- ShopDiabetes.org
- ADA Professional Books
Clinical Compendia
- Clinical Compendia Home
- Latest News
- DiabetesPro SmartBrief
- Special Collections
- DiabetesPro®
- Diabetes Food Hub™
- Insulin Affordability
- Know Diabetes By Heart™
- About the ADA
- Journal Policies
- For Reviewers
- Advertising in ADA Journals
- Reprints and Permission for Reuse
- Copyright Notice/Public Access Policy
- ADA Professional Membership
- ADA Member Directory
- Diabetes.org
- X (Twitter)
- Cookie Policy
- Accessibility
- Terms & Conditions
- Get Adobe Acrobat Reader
- © Copyright American Diabetes Association
This Feature Is Available To Subscribers Only
Sign In or Create an Account
- U.S. Department of Health & Human Services

- Virtual Tour
- Staff Directory
- En Español
You are here
How covid-19 can lead to diabetes, nih blog post date, connect with us.
- More Social Media from NIH
- Alzheimer's disease & dementia
- Arthritis & Rheumatism
- Attention deficit disorders
- Autism spectrum disorders
- Biomedical technology
- Diseases, Conditions, Syndromes
- Endocrinology & Metabolism
- Gastroenterology
- Gerontology & Geriatrics
- Health informatics
- Inflammatory disorders
- Medical economics
- Medical research
- Medications
- Neuroscience
- Obstetrics & gynaecology
- Oncology & Cancer
- Ophthalmology
- Overweight & Obesity
- Parkinson's & Movement disorders
- Psychology & Psychiatry
- Radiology & Imaging
- Sleep disorders
- Sports medicine & Kinesiology
- Vaccination
- Breast cancer
- Cardiovascular disease
- Chronic obstructive pulmonary disease
- Colon cancer
- Coronary artery disease
- Heart attack
- Heart disease
- High blood pressure
- Kidney disease
- Lung cancer
- Multiple sclerosis
- Myocardial infarction
- Ovarian cancer
- Post traumatic stress disorder
- Rheumatoid arthritis
- Schizophrenia
- Skin cancer
- Type 2 diabetes
- Full List »
share this!
October 20, 2020
Research team describes how a virus can cause diabetes
by The Spanish National Cancer Research Centre
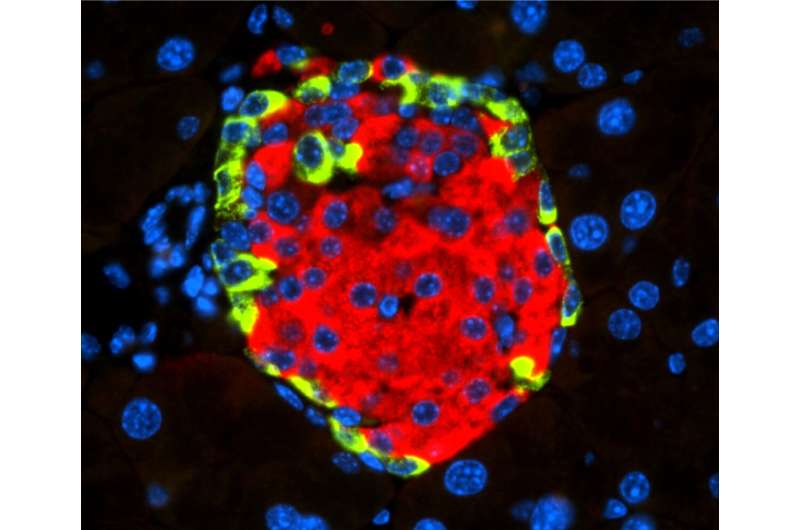
It has recently been described that infection by some enteroviruses—a genus of viruses that commonly cause diseases of varying severity—could potentially trigger diabetes, although its direct effect 'in vivo' as well as its mechanism of action at the molecular level were unknown. Now, a team of researchers from the Growth Factors, Nutrients and Cancer Group, led by Nabil Djouder at the Spanish National Cancer Research Centre (CNIO), demonstrates for the first time in Cell Reports Medicine how the enterovirus coxsackievirus type B4 (CVB4) could induce diabetes. These findings can be a fundamental step to open the way to the search of new therapeutic strategies.
The researchers also point out that the finding could be of relevance for the COVID-19 pandemic, since clinical information indicates a possible relationship between SARS-CoV-2 viral infection and diabetes . Djouder and his team suggest that since receptor of SARS-CoV-2 is expressed in the endocrine pancreas, it could operate and lead to diabetes in a similar way that CVB4 does, independently of immune reactions.
Molecular mechanisms of failure in insulin production
Coxsackieviruses belong to the family of Enterovirus , which also includes poliovirus and echovirus, and which can cause from mild flu-like illnesses to more serious illnesses such as myocarditis, pericarditis, meningitis, or pancreatitis. It was suspected that these viruses can cause diabetes in humans, but molecular mechanisms were unknown.
With the aim of finding and describing these mechanisms, the CNIO researchers worked with animal models engrafted with human pancreatic cells infected by CVB4, as well as with human and mouse insulin-producing cells, also infected with this virus.
What they observed was that CVB4 infection induces deregulation of URI, a protein that regulates the normal functions of numerous cellular activities. "In this case, URI downregulation triggers a cascade of molecular events leading to modification of the genome via hypermethylation and silencing of Pdx1. This is a gene critical for the identity and the function of beta cells present in the endocrine pancreas, at the so-called Islets of Langerhans, and responsible for the production and secretion of insulin, a hormone that decreases blood glucose levels ," explains Nabil Djouder, lead author of the work published in Cell Reports Medicine . "PDX1 silencing causes the loss of the identity and function of the beta cells, which become more like alpha cells, in charge of increasing blood glucose levels, and hence leading to hyperglycemia and subsequent diabetes, independently of any immune reactions."
The researchers demonstrated their findings by using various genetically engineered mouse models and genomic studies. They show that loss of URI in mouse pancreata alters beta cell identity and function, leading to diabetes. Furthermore, they observed that diabetic mice that overexpress URI in beta cells are more tolerant to glucose. Finally, they demonstrated in several pancreata from diabetic patients that expression of URI, PDX1 and viral particles correlates in beta cells, highlighting a causal link between enterovirus infection and diabetes in humans.
The CNIO results could help advance knowledge about the pathological effects of the virus causing the current pandemic: "Similarly to our investigations on enteroviruses, some recent clinical observations have associated SARS-CoV-2, the virus responsible for COVID-19, to diabetes in infected patients," explains Djouder. "Since the receptor of SARS-Co-V2 is present in beta cells , it would be interesting to study if this virus also alters URI function and silences the expression of PDX1 to affect beta-cell function, promoting diabetes."
The researchers also suggest that, following these results, a possible prevention and therapeutic strategy would be to use, in combination with anti-viral therapies, inhibitors against DNA methylase transferase, a protein responsible for the hypermethylation of the genome and silencing of Pdx1. Indeed, Djouder's team demonstrated that this class of inhibitors reinstated PDX1 expression and glucose tolerance in diabetic mice. Several of these inhibitors have already been licensed for clinical use in cancer treatments, which could speed up their application in these cases.
Explore further
Feedback to editors

Hippocampal study unveils distinct protein machineries for calcium channel clustering and vesicle priming
2 hours ago

Wearable brain imaging device shines a light on how babies respond in real-world situations
13 hours ago

Weight loss drug liraglutide is safe and effective in children under 12, study finds
14 hours ago

New study shows that chronic neurodegeneration can be prevented after traumatic brain injury
15 hours ago

Diabetes drug helps the immune system recognize reservoirs of HIV, study discovers
16 hours ago

Why a promising breast cancer drug doesn't work—and how to improve it

New precision tools make quick work of tumor dissection

Finding the right pathway to reduce fat accumulation in the liver

Variety is the spice of learning, memory study suggests

Can chatbots help with genetic testing for cancer risk?
Related stories.

New research challenges the most common model for diabetes research
Sep 30, 2020
COVID-19 and type 2 diabetes: do shared pathways have therapeutic implications?
Sep 23, 2020

A protein in the pancreas protects it from stress induced by a high-fat diet
Sep 16, 2020

A surprising protein player in diabetes
Aug 28, 2020

Gene therapy restores normal blood glucose levels in mice with type 1 diabetes
Jan 4, 2018

Researchers discover process that may explain how type 2 diabetes develops
Dec 20, 2019
Recommended for you

Novel class of once-weekly insulin as effective as daily injections for patients with type 2 diabetes, new study finds
20 hours ago

Low-carb/high-fat diets for weight loss may actually boost risk of type 2 diabetes
Sep 5, 2024

Insulin and metformin combo aids diabetic foot ulcer healing, new study finds
Sep 4, 2024

Beta cells alone can regulate blood sugar levels, study finds
Sep 3, 2024

No link found between popular diabetes medication and suicide

Mankai plant found to reduce post-meal sugar levels in diabetics
Aug 29, 2024
Let us know if there is a problem with our content
Use this form if you have come across a typo, inaccuracy or would like to send an edit request for the content on this page. For general inquiries, please use our contact form . For general feedback, use the public comments section below (please adhere to guidelines ).
Please select the most appropriate category to facilitate processing of your request
Thank you for taking time to provide your feedback to the editors.
Your feedback is important to us. However, we do not guarantee individual replies due to the high volume of messages.
E-mail the story
Your email address is used only to let the recipient know who sent the email. Neither your address nor the recipient's address will be used for any other purpose. The information you enter will appear in your e-mail message and is not retained by Medical Xpress in any form.
Newsletter sign up
Get weekly and/or daily updates delivered to your inbox. You can unsubscribe at any time and we'll never share your details to third parties.
More information Privacy policy
Donate and enjoy an ad-free experience
We keep our content available to everyone. Consider supporting Science X's mission by getting a premium account.
E-mail newsletter
Talk to us about diabetes
0345 123 2399
customer support
Exploring research: Can coronavirus cause diabetes, or make it worse?
There are some very early clues to suggest that coronavirus might be triggering type 1 or type 2 diabetes in some people, or making the condition worse for others. We take a look at the evidence and explain why it’s too early to know for sure yet.

Written by Faye Riley PhD, our Senior Research Communications Officer. This article is part of our series on the key things to know about coronavirus and diabetes research .
New cases of diabetes
At the start of the coronavirus pandemic, doctors started to raise concerns around new cases of both type 1 and type 2 diabetes in people who had caught the virus.
Type 1 diabetes
Since then, small studies have suggested that rates of new type 1 diabetes diagnoses in children are higher in 2020 compared to average rates in previous years.
The causes of type 1 diabetes are complex, and scientists think that there are a variety of environmental and genetic reasons that could explain why the condition develops.
Viruses could be one of these reasons, but the evidence around this is mixed and we just don’t know for sure yet. And as the virus that causes the Covid-19 infection is so new, there’s a lot we still need to learn about how it interacts with our immune system and its longer-term effects.
Type 2 diabetes
Cases of new type 2 diabetes diagnoses have also been reported in people who have had coronavirus. One possible reason for this could be to do with ACE-2. This is the protein on the surface of cells that coronavirus uses to enter and infect them.
ACE-2 is found in the pancreas and in other organs and tissues that play an important role in how our body processes glucose in our blood.
When coronavirus ‘locks on’ to ACE-2, our cells might not be able to do their normal job and help us release insulin and process glucose. It is possible that this could bring already existing type 2 diabetes to light, or perhaps even cause a new type of diabetes to develop.
It’s also possible that blood glucose (sugar) levels in some people with coronavirus rise due to the stress the body is under when trying to fight the infection, or because of some of the treatments used to treat it.
This could play a role in new cases of diabetes developing. But we don’t yet know if, or when, high blood sugar levels in people with coronavirus return to normal after they have fully recovered.
Finding answers
The evidence to suggest coronavirus could trigger type 1, type 2 - or even a new type of diabetes - is growing, but we need to keep in mind that everything we know so far has come from only a handful of observational studies.
This is when researchers look at groups of people and collect information about them, such as who does and who doesn’t develop diabetes. But from these types of studies we can’t be sure if coronavirus is directly causing any new cases of diabetes, or whether there are other factors that could explain the link.
Although it still remains unclear what’s behind any apparent rise in cases, scientists are working hard to find answers.
An international group of top diabetes researchers are building a database of new cases of diabetes in people with coronavirus, called the CoviDiab registry . This will give them the information they need to carry out more thorough studies and discover more.
Coronavirus and how type 2 diabetes progresses
We’ve also heard reports that coronavirus could make type 2 diabetes progress more quickly in people who already have it. This could also be down to the impact of the virus on ACE-2, affecting how our body makes insulin and processes glucose.
More research, including the PHOSP-COVID study, will help us to fully understand this better. The UK-wide study is following 10,000 people who were in hospital with coronavirus to monitor the long-term impact of the virus on their health. This study will include people with type 2 diabetes and will help us to understand how their condition has been affected.
More things to know about coronavirus research
We know that you may have more questions about coronavirus and diabetes, so we've taken a look at the research to bring you the key things to know. Find out more about:
- the risk of needing to go to hospital or dying if people with diabetes get coronavirus
Share this Page

© The British Diabetic Association operating as Diabetes UK, a charity registered in England and Wales (no. 215199) and in Scotland (no. SC039136). A company limited by guarantee registered in England and Wales with (no.00339181) and registered office at Wells Lawrence House, 126 Back Church Lane London E1 1FH
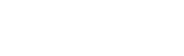
- Featured News
- Artificial Intelligence
- Bioprocessing
- Drug Discovery
- Genome Editing
- Infectious Diseases
- Translational Medicine
- Browse Issues
- Learning Labs
- eBooks/Perspectives
- GEN Biotechnology
- Re:Gen Open
- New Products
- Conference Calendar
- Get GEN Magazine
- Get GEN eNewsletters

How a Virus Can Trigger Diabetes
Islet of Langerhans with beta cell-secreting insulin (in red) and alpha cell-secreting glucagon (in green). [CNIO]
According to the World Health Organization, around 1.6 million people worldwide died due to diabetes in 2016. It is estimated that 425 million people are living with diabetes all over the world. By 2045, projections show this number rising to some 629 million diabetics globally. Type 1 diabetes is an autoimmune condition where the pancreas cannot produce insulin, whereas type 2 diabetes is the body’s resistance to insulin.
Previous studies have linked viruses to diabetes, and recent studies have suggested that enteroviruses could potentially trigger diabetes, although its direct effect in vivo as well as its mechanism of action at the molecular level were unknown. A recent mouse study by researchers at the Spanish National Cancer Research Centre (CNIO) reveals how the enterovirus coxsackievirus type B4 (CVB4) could induce diabetes.
Their findings, “ Coxsackievirus B Type 4 Infection in β Cells Downregulates the Chaperone Prefoldin URI to Induce a MODY4-like Diabetes via Pdx1 Silencing ,” is published in Cell Reports Medicine and led by Nabil Djouder, PhD, group leader at CNIO.
The researchers suggest their findings could be of relevance for the COVID-19 pandemic, since clinical information indicates a possible relationship between SARS-CoV-2 viral infection and diabetes. The researchers suggest that since the receptor of SARS-CoV-2 is expressed in the endocrine pancreas, it could operate and lead to diabetes in a similar way that CVB4 does, independently of immune reactions.
“Enteroviruses are suspected to contribute to insulin-producing β cell loss and hyperglycemia-induced diabetes. However, mechanisms are not fully defined. Here, we show that coxsackievirus B type 4 (CVB4) infection in human islet-engrafted mice and in rat insulinoma cells displays loss of unconventional prefoldin RPB5 interactor (URI) and PDX1, affecting β cell function and identity. Genetic URI ablation in the mouse pancreas causes PDX1 depletion in β cells,” noted the researchers.
The researchers observed animal models engrafted with human pancreatic cells infected by CVB4, as well as with human and mouse insulin-producing cells, also infected with this virus. They discovered that CVB4 infection induces deregulation of URI, a protein that regulates the normal functions of numerous cellular activities.
“In this case, URI downregulation triggers a cascade of molecular events leading to modification of the genome via hypermethylation and silencing of Pdx1. This is a gene critical for the identity and the function of beta cells present in the endocrine pancreas, at the so-called Islets of Langerhans, and responsible for the production and secretion of insulin, a hormone that decreases blood glucose levels,” stated Djouder. “PDX1 silencing causes the loss of the identity and function of the beta cells, which become more like alpha cells, in charge of increasing blood glucose levels, and hence leading to hyperglycemia and subsequent diabetes, independently of any immune reactions.”
The researchers also observed that diabetic mice that overexpress URI in beta cells are more tolerant to glucose. They demonstrated that expression of URI, PDX1, and viral particles correlates in beta cells, highlighting a causal link between enterovirus infection and diabetes in humans.
“Similarly to our investigations on enteroviruses, some recent clinical observations have associated SARS-CoV-2, the virus responsible for COVID-19, to diabetes in infected patients,” explained Djouder. “Since the receptor of SARS-Co-V2 is present in beta cells, it would be interesting to study if this virus also alters URI function and silences the expression of PDX1 to affect beta-cell function, promoting diabetes.”
These findings could be a critical step to a new wave of therapeutic strategies and may aid in understanding the relationship between SARS-CoV-2 and diabetes.
Related Content

StockWatch: Athira Pays Price as Alzheimer’s Candidate Fails Trial

Spinal Cord Injury Recovery in Mice Boosted by Immunotherapy

Animal Models Aren’t Human!

Lonza Completes Expansion of Microbial Manufacturing Facility
Eww that smell: key basis for bo production identified, the effect of ms scan speed on uplc peek separation and....
- Research article
- Open access
- Published: 31 August 2024
The relationship between COVID-19 and hyperglycemia: screening and monitoring hospitalized patients
- Faranak Salajegheh 1 , 2 ,
- Somayyeh Salajegheh 3 ,
- Mohsen Nakhaie ORCID: orcid.org/0000-0001-7605-2593 2 , 4 ,
- Niloofar Farsiu 4 ,
- Seyedeh Mahdieh Khoshnazar 4 ,
- Reza Sinaei 2 ,
- Mehrdad Farrokhnia 5 &
- Soheila Torabiyan 2
Clinical Diabetes and Endocrinology volume 10 , Article number: 29 ( 2024 ) Cite this article
Metrics details
Elevated blood glucose concentration, also known as hyperglycemia, has been identified as a significant factor influencing the prognosis of COVID-19, alongside the impact of the SARS-CoV-2 infection itself.
This research is a cross-sectional investigation that examined the relationship between COVID-19 and hyperglycemia in patients admitted to Afzalipour Hospital in Kerman, Iran, from July to September 2021. A standardized data sheet was used to capture demographic data (age, gender) and laboratory information (blood sugar, arterial blood oxygen saturation, and C-reactive protein (CRP)) upon admission.
The present research evaluated a total of 300 individuals diagnosed with COVID-19, with an average age of 50.19 ± 15.55 years. Among these patients, the majority were male, accounting for 51.67% of the total. Hyperglycemia was seen in 21.67% of patients, but less than 20% had new-onset diabetes. Individuals exhibiting hyperglycemia were typical of advanced age ( P < 0.001). Furthermore, there was a slight but statistically significant association between advanced age and elevated blood glucose concentration ( R = 0.254, P < 0.001). Gender had no significant impact on the occurrence of hyperglycemia ( P = 0.199). There was no significant association between CRP levels and blood glucose concentration ( P = 0.524) or the incidence of hyperglycemia ( P = 0.473). Although there was no significant disparity in blood oxygen saturation between individuals with or without hyperglycemia ( P = 0.06), higher blood glucose concentration was correlated with lower blood oxygen saturation ( R = -0.151, P < 0.001).
Considering the correlation between blood glucose concentration, advanced age, and disease severity, it is recommended to carefully screen and monitor all COVID-19 patients for hyperglycemia and new-onset diabetes. Effective management of these complications could enhance the control of patients’ overall prognosis and subsequent complications.
The COVID-19 outbreak in Wuhan originated on December 12, 2019, with patients displaying similar clinical signs, including fever, cough, shortness of breath, and distinctive pneumonia [ 1 ]. The first instance of this event outside of China was reported on January 13 in Thailand. As the disease progressively invaded various countries, the World Health Organization (WHO) declared a global health emergency on January 30, and COVID-19 was officially designated a pandemic on March 11, 2020 [ 2 ].
The symptoms of COVID-19 exhibit significant variability depending on the specific variants of SARS-CoV-2, the demographic characteristics of the patients, and the existence of underlying health issues [ 4 , 5 , 6 ]. Although many symptoms of this condition are associated with respiratory tract infections, such as fever and cough, there have also been reports of other systemic symptoms, including gastrointestinal and neurological manifestations [ 7 ]. Additional severe consequences, such as acute respiratory distress syndrome (ARDS) and acute cardiac damage, were seen in more susceptible cases [ 8 ]. Several lingering symptoms, including fatigue and shortness of breath, were noted in afflicted individuals until weeks after the infection [ 8 , 9 ].
Some studies have indicated that the viral load of SARS-CoV-2 is notably higher among individuals with diabetes [ 10 , 11 , 12 ]. Both pre-existing diabetes and new-onset hyperglycemia are identified as crucial factors influencing the prognosis of COVID-19 [ 10 , 11 , 13 ]. Importantly, several investigations have documented instances of COVID-19 individuals exhibiting elevated blood glucose concentrations, irrespective of a prior diabetes diagnosis [ 14 ].
Corticosteroid medications, inflammation, stress hormones, and antibiotic treatments are a few of the common causes of hospitalization-related insulin resistance (IR) [ 15 ]. Elevated cytokine levels in COVID-19 individuals may exacerbate IR, leading to elevated blood sugar levels and decreased insulin production. Furthermore, SARS-CoV-2 can infiltrate endocrine pancreatic cells, compromising beta cell insulin release and exacerbating pre-existing diabetes. The mentioned beta-cell dysfunctions may result in diabetic ketoacidosis, hyperglycemia, and potentially new diabetes cases [ 16 , 17 ].
Hyperglycemia during a COVID-19 infection, especially in severe cases, can be dangerous. For example, it can cause a sudden rise in inflammatory factors because of the higher blood glucose level and the activation of ACE2 receptors, which lets SARS-CoV-2 attach to these receptors [ 20 ]. The elevated interaction between aberrantly glycosylated ACE2 in uncontrolled hyperglycemia and the virus contributes to increased viral replication, potentially leading to greater disease severity [ 21 ].
Although several studies have examined the frequency of hyperglycemia in COVID-19 patients, especially those with pre-existing diabetes mellitus, there is still a critical need for research that explicitly targets people who do not have a previous diagnosis of diabetes. This research specifically targeted people who did not have pre-existing diabetes, a group that has not been extensively studied in the current literature. Our objective was to shed light on COVID-19-associated hyperglycemia by examining the prevalence and correlates of this subgroup’s hyperglycemia and new-onset diabetes. Additionally, our research was conducted at Afzalipour Hospital in Kerman, Iran, providing vital data from a region that may have distinctive patterns of illness occurrence and treatment. By collecting and analyzing the data thoroughly, we aimed to provide new insights that improved our comprehension of the intricate connection between COVID-19 and blood sugar problems. The results of the study will help develop more efficient approaches to patient treatment and care.
Study population
This cross-sectional retrospective study was focused on COVID-19 patients hospitalized at Afzalipour Hospital in Kerman, Iran, from July to September 2021, who were discharged in a healthy or stable condition. The patients’ data were obtained from hospital records, and the study population was selected according to the inclusion and exclusion criteria described later.
Inclusion and exclusion criteria
The inclusion criteria for the study included:
Positive RT-PCR test result for the SARS-CoV-2 virus or a chest computed tomography (CT) scan indicating evidence in favor of infection with this virus;
Patient’s full consciousness;
Age 20 ≤ and ≥ 50 years (excluding age ranges with a high risk of diabetes 1 and 2 development).
The exclusion criteria included:
Previous definite diabetes 1 or 2 (measured by fasting blood sugar (FBS) and HbA1c) or glucose tolerance disorders;
Pregnancy (due to the risk of gestational diabetes);
Use of corticosteroid drugs (which can lead to hyperglycemia);
Lack of patient file information;
Occurrence of death during hospitalization.
The methodology for enrolling the study population is summarized in the flowchart provided in Fig. 1 .
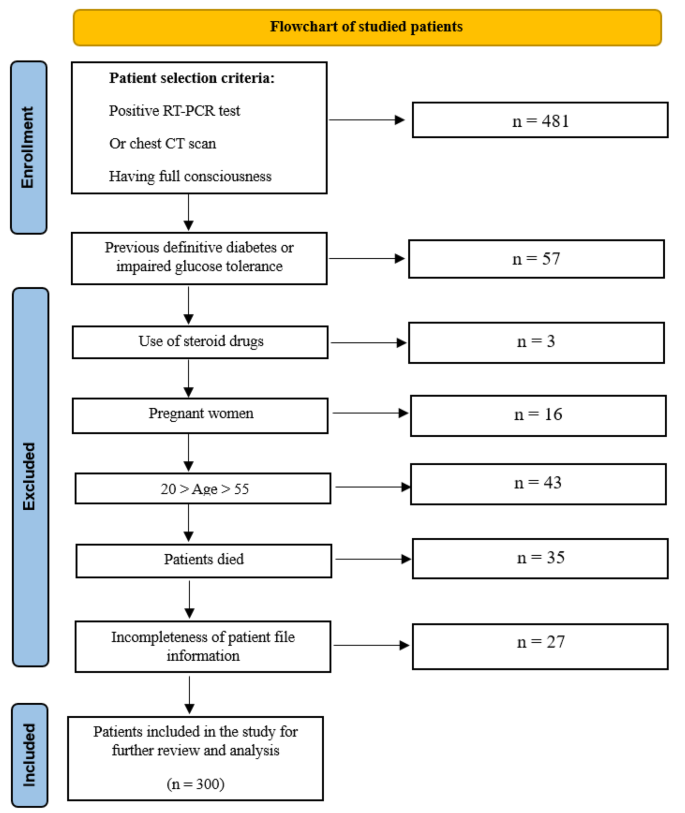
Flowchart for the method of enrolling the study population
Data collection
After the patients were selected, relevant information from the patients’ records was collected using a comprehensive data sheet. The collected data included demographic details such as age and gender, as well as laboratory information such as blood glucose concentration, arterial blood oxygen saturation (SpO2), and C-reactive protein (CRP) serum levels upon admission.
Specific criteria were established to identify hyperglycemia. Patients were considered to have hyperglycemia if they exhibited at least one of the following on the first day of admission:
Two-hour postprandial glucose (2hpp) ≥ 200 mg/dL.
FBS ≥ 126 mg/dL.
It should be noted that glucose tolerance disorders, dysglycemia that comprises both prediabetes and diabetes, including conditions of impaired fasting glucose (IFG), impaired glucose tolerance (IGT), and diabetes mellitus (DM), were also recognized with FBS between 110 and 125 mg/dL or 2hpp 140–199 mg/dL.
Statistical analysis
The collected data were analyzed using SPSS statistical software (version 27). Descriptive statistics, such as central tendency indices (mean and standard deviation), as well as frequency and percentage, were used to categorize and summarize the findings. The chi-squared test, student’s t-test, and Pearson correlation coefficient (R) were used to investigate the relationship between COVID-19 and demographic variables with hyperglycemia. Meanwhile, P.values less than 0.05 were considered significant.
Demographic and clinical information
The research included 300 patients diagnosed with COVID-19. The average age was 50.19 ± 15.55 years, with a majority of men (51.67%), as shown in Table 1 . Hyperglycemia was identified in 65 patients (21.67%), and new-onset diabetes was diagnosed in 17.67% of cases (Table 1 ). In addition, the average blood glucose concentration, blood oxygen saturation, and CRP levels were also measured among all patients (Table 1 ).
Relationship between hyperglycemia, new onset of diabetes, and gender
There was no significant difference in gender distribution between patients with or without hyperglycemia ( P = 0.199). However, patients with hyperglycemia had a significantly higher rate of new-onset diabetes diagnosis ( P < 0.001) (Table 2 ).
Relationship of hyperglycemia with quantitative parameters
Patients with hyperglycemia exhibited a higher age ( P < 0.001) and higher blood glucose concentration ( P < 0.001) compared to others. No significant differences were observed in blood oxygen saturation ( P = 0.06) and serum CRP ( P = 0.473) between the two groups (Table 3 ).
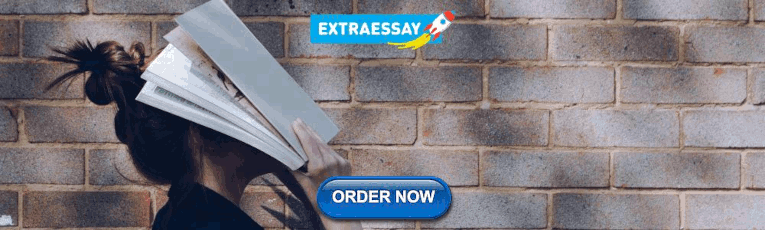
Correlation analysis
Examining correlations between quantitative variables revealed that CRP levels were not significantly related to blood glucose concentration ( P = 0.524). However, age showed a slight yet positive significant relationship with blood glucose concentration ( R = 0.254, P < 0.001), while blood oxygen saturation exhibited a slight yet negative significant relationship with blood glucose concentration ( R = -0.151, P < 0.001) (Table 4 ).
Multiple studies have shown that the presence of high levels of blood glucose concentration and hyperglycemia are not only influenced by the SARS-CoV-2 infection but also play a role in defining the severity and eventual outcome of COVID-19. Our study was designed to examine the links between COVID-19 and hyperglycemia, focusing on individuals without prior diabetes diagnosis. The objective was to determine whether COVID-19-induced hyperglycemia could contribute to the development of new-onset diabetes. This experiment focused on patients in Kerman, Iran, and aimed to determine whether regional disparities provide distinct results compared to other investigations.
The findings of our study revealed that over 20% of hospitalized COVID-19 patients experienced hyperglycemia, with less than 20% developing new-onset diabetes. In terms of demographic factors, advanced age correlated with both the presence of hyperglycemia and higher blood glucose concentration, while gender did not significantly influence the occurrence of hyperglycemia. Additionally, CRP levels did not impact blood glucose concentration or the occurrence of hyperglycemia. Although there was no significant difference in blood oxygen saturation between patients with or without hyperglycemia, lower blood oxygen saturation levels were associated with elevated blood glucose concentration.
In our study, the incidence of hyperglycemia and new-onset diabetes was found to be 21.67% and 17.67%, respectively. This rate of hyperglycemia occurrence in COVID-19 patients without a previous history of diabetes varied across similar studies, ranging from 4% to more than 20% [ 22 , 23 , 24 , 25 , 26 ]. Unfortunately, the patients in our study are on the higher end of the spectrum. It is difficult to accurately determine the occurrence of hyperglycemia and new-onset diabetes in COVID-19 patients since it is challenging to distinguish between cases where individuals had pre-existing high blood glucose concentrations that remained undetected before developing COVID-19 and cases in which hyperglycemia developed after the infection.
The present study emphasizes the need for further research to carefully assess COVID-19 patients for prior elevated blood glucose concentrations in order to acquire more dependable findings, with a particular focus on the occurrence of new-onset diabetes. It is essential to additionally take into account confounding variables, such as the treatment plans for COVID-19, particularly corticosteroids, and the influence of IR during severe diseases like sepsis on high blood glucose concentrations [ 18 , 19 , 27 ].
Our study revealed that aging is associated with a higher risk of hyperglycemia in patients with COVID-19, and there is a positive correlation between age and blood glucose concentrations. This finding aligns with the results of previous similar studies, highlighting that elderly patients are at a greater risk for hyperglycemia [ 28 , 29 , 30 ]. This association may be attributed to the weaker immune response and increased susceptibility to infections in older individuals, leading to more severe clinical symptoms following the SARS-CoV-2 infection [ 31 ].
Also, our study found that gender did not have a significant impact on the occurrence of hyperglycemia in patients with COVID-19. While this study did not specifically investigate the link between gender and the occurrence of hyperglycemia in patients with COVID-19, the absence of a significant relationship between the two parameters was expected. This is because previous research has shown that men are more likely to experience severe COVID-19 due to cultural, social, and behavioral factors that increase their exposure to the virus. Conversely, it has been shown that women often have a more robust immune response to illnesses and immunizations, leading to potentially severe consequences. Gender-related disparities may have mitigated the influence of gender on the prevalence of hyperglycemia in the setting of COVID-19 [ 32 ].
When comparing individuals with or without hyperglycemia, there was no significant difference in CRP levels or blood oxygen saturation, which are both indicators of disease severity and inflammation. However, lower blood oxygen levels are often correlated with higher blood glucose concentrations. The etiopathological role of inflammation in the development and progression of hyperglycemia and type 2 diabetes was previously recognized. Inflammatory cytokines can induce structural and functional abnormalities in endothelial cells, leading to impaired insulin secretion, damage to pancreatic beta-cells, and, ultimately, an increase in blood glucose concentration [ 33 , 34 , 35 , 36 ]. Hence, the presence of increased inflammation after acquiring SARS-CoV-2 and IR is to be anticipated. Nevertheless, our findings do not provide definitive proof of a direct correlation between hyperglycemia and these inflammatory markers. It is recommended that future studies investigate the incidence of mild COVID-19 cases (outpatient cases) and the likelihood of hyperglycemia to further elucidate the relationship between COVID-19, inflammation, and hyperglycemia.
In addition to infection, medications, and inflammation, stress can also contribute to hyperglycemia and lead to the development of new-onset diabetes. Future studies can explore the stress hyperglycemia ratio (SHR), which measures the ratio of blood glucose (mmol/L) per HbA1c (%) [ 27 ]. The evaluation of SHR can provide valuable information about the progression and severity of the disease. By incorporating the SHR into future research, our comprehension of stress-induced hyperglycemia in COVID-19 patients can be improved. This will provide a thorough evaluation of stress-induced hyperglycemia’s effect on the prognosis of the disease.
The current study has several limitations, including its retrospective nature, the examination of COVID-19 patients in a single medical facility, and a lack of investigation into the relationship between blood glucose concentration, hyperglycemia, and the course and prognosis of the disease. Furthermore, the research failed to do post-recovery and discharge follow-ups on blood glucose concentration, particularly in the long term. In addition, the study did not gather any data on the symptoms reported by the participants, which could have supplied useful information about how COVID-19 presents clinically in relation to hyperglycemia. Furthermore, the study did not distinguish between different variants of the SARS-CoV-2 virus, which could have given invaluable perspectives into the virus’s attributes and its potential effects on hyperglycemia. Future studies are recommended to overcome these limitations and provide a more comprehensive understanding of the topic. Despite these limitations, the study’s strengths lie in its substantial sample size and the examination of crucial factors influencing disease severity, as well as their interplay in the occurrence of hyperglycemia and blood glucose concentration.
In conclusion, given the significant occurrence of hyperglycemia and new-onset diabetes in COVID-19 patients, particularly among seniors, and the observed association between blood glucose concentration and increased disease severity, it is crucial to screen and closely monitor all COVID-19 patients for hyperglycemia and new-onset diabetes. This proactive approach is essential for controlling the relative prognosis of these patients and mitigating subsequent complications through appropriate management of this complication.
Data availability
The datasets of the present investigation can be obtained from the corresponding author following an adequate request.
Zhu N, et al. A novel coronavirus from patients with pneumonia in China, 2019. N Engl J Med. 2020;382(8):727–33.
Article CAS PubMed PubMed Central Google Scholar
Organization WH. WHO timeline-Covid-19. 2020, Accessed May , vol. 30, 2020.
Wang D et al. Clinical characteristics of 138 hospitalized patients with 2019 novel coronavirus–infected pneumonia in Wuhan, China, jama , vol. 323, no. 11, pp. 1061–1069, 2020.
Hu B, Guo H, Zhou P, Shi Z-L. Characteristics of SARS-CoV-2 and COVID-19. Nat Rev Microbiol, 19, 3, pp. 141–54, 2021/03/01 2021, https://doi.org/10.1038/s41579-020-00459-7
Whitaker M et al. Variant-specific symptoms of COVID-19 in a study of 1,542,510 adults in England, Nature Communications , vol. 13, no. 1, p. 6856, 2022/11/11 2022, https://doi.org/10.1038/s41467-022-34244-2
Clark A et al. Global, regional, and national estimates of the population at increased risk of severe COVID-19 due to underlying health conditions in 2020: a modelling study, (in eng), Lancet Glob Health , vol. 8, no. 8, pp. e1003-e1017, Aug 2020, https://doi.org/10.1016/s2214-109x(20)30264-3
Sardu C, Gambardella J, Morelli MB, Wang X, Marfella R, Santulli G. Hypertension, Thrombosis, Kidney Failure, and Diabetes: Is COVID-19 an Endothelial Disease? A Comprehensive Evaluation of Clinical and Basic Evidence, Journal of Clinical Medicine , vol. 9, no. 5, p. 1417, 2020. [Online]. Available: https://www.mdpi.com/2077-0383/9/5/1417
Marjenberg Z et al. Risk of long COVID main symptoms after SARS-CoV-2 infection: a systematic review and meta-analysis. Sci Rep, 13, 1, p. 15332, 2023/09/15 2023, https://doi.org/10.1038/s41598-023-42321-9
Trimarco V, et al. Endothelial dysfunction in long-COVID: new insights from the nationwide multicenter LINCOLN Study, (in eng). Pharmacol Res. Nov 2022;185:106486. https://doi.org/10.1016/j.phrs.2022.106486
Bode B, et al. Glycemic characteristics and clinical outcomes of COVID-19 patients hospitalized in the United States. J Diabetes Sci Technol. 2020;14(4):813–21.
Smith SM, et al. Impaired glucose metabolism in patients with diabetes, prediabetes, and obesity is associated with severe COVID-19. J Med Virol. 2021;93(1):409–15.
Article CAS PubMed Google Scholar
Mone P et al. Endothelial extracellular vesicles enriched in microRNA-34a Predict New-Onset diabetes in Coronavirus Disease 2019 (COVID-19) patients: Novel insights for Long COVID metabolic sequelae, (in eng). J Pharmacol Exp Ther, 389, 1, pp. 34–9, Mar 15 2024, https://doi.org/10.1124/jpet.122.001253
Izzo R et al. Incidence of type 2 diabetes before and during the COVID-19 pandemic in Naples, Italy: a longitudinal cohort study, (in eng), EClinicalMedicine , vol. 66, p. 102345, Dec 2023, https://doi.org/10.1016/j.eclinm.2023.102345
Ilias I et al. Glycemia, beta-cell function and sensitivity to insulin in mildly to critically ill Covid-19 patients, Medicina , vol. 57, no. 1, p. 68, 2021.
Zahedi M, Kordrostami S, Kalantarhormozi M, Bagheri M. A Review of Hyperglycemia in COVID-19, (in eng), Cureus , vol. 15, no. 4, p. e37487, Apr 2023, https://doi.org/10.7759/cureus.37487
Apicella M, Campopiano MC, Mantuano M, Mazoni L, Coppelli A, Del Prato S. COVID-19 in people with diabetes: understanding the reasons for worse outcomes, (in eng), Lancet Diabetes Endocrinol , vol. 8, no. 9, pp. 782–792, Sep 2020, https://doi.org/10.1016/s2213-8587(20)30238-2
Ceriello A, De Nigris V, Prattichizzo F. Why is hyperglycaemia worsening COVID-19 and its prognosis? (in eng). Diabetes Obes Metab. Oct 2020;22(10):1951–2. https://doi.org/10.1111/dom.14098
Ceriello A, De Nigris V, Prattichizzo F. Why is hyperglycaemia worsening COVID-19 and its prognosis? Diabetes, obesity & metabolism , vol. 22, no. 10, p. 1951, 2020.
Apicella M, Campopiano MC, Mantuano M, Mazoni L, Coppelli A, Del Prato S. COVID-19 in people with diabetes: understanding the reasons for worse outcomes. Lancet Diabetes Endocrinol. 2020;8(9):782–92.
Ceriello A, Zarich SW, Testa R. Lowering glucose to prevent adverse cardiovascular outcomes in a critical care setting. J Am Coll Cardiol, 53, no. 5S, pp. S9-S13, 2009.
Brufsky A. Hyperglycemia, hydroxychloroquine, and the COVID-19 pandemic. J Med Virol. 2020;92(7):770–5.
Coppelli A et al. Hyperglycemia at hospital admission is associated with severity of the prognosis in patients hospitalized for COVID-19: the Pisa COVID-19 study, Diabetes care , vol. 43, no. 10, pp. 2345–2348, 2020.
Fadini GP, et al. Newly-diagnosed diabetes and admission hyperglycemia predict COVID-19 severity by aggravating respiratory deterioration. Diabetes Res Clin Pract. 2020;168:108374.
Charoenngam N, Alexanian SM, Apovian CM, Holick MF. Association between hyperglycemia at hospital presentation and hospital outcomes in COVID-19 patients with and without type 2 diabetes: A retrospective cohort study of hospitalized inner-city COVID-19 patients, Nutrients , vol. 13, no. 7, p. 2199, 2021.
Le VT, Ha QH, Tran MT, Le NT, Le MK, Le TV. Hyperglycemia in Severe and Critical COVID-19 Patients: Risk Factors and Outcomes, Cureus , vol. 14, no. 8, 2022.
Nateghi S, et al. Moderately hyperglycemia as an independent prognostic factor for the worse outcome of COVID-19. Prim Care Diabetes. 2022;16(3):361–4.
Bar-Or D, et al. Stress hyperglycemia in critically ill patients: insight into possible molecular pathways. Front Med. 2019;6:54.
Article Google Scholar
Khalangot M, et al. Relationship between hyperglycemia, waist circumference, and the course of COVID-19: mortality risk assessment. Experimental Biology Med. 2022;247(3):200–6.
Article CAS Google Scholar
Gerganova A, Assyov Y, Kamenov Z. Stress hyperglycemia, diabetes mellitus and COVID-19 infection: risk factors, clinical outcomes and post-discharge implications. Front Clin Diabetes Healthc. 2022;3:826006.
Article PubMed PubMed Central Google Scholar
Zhou W, Ye S, Wang W, Li S, Hu Q. Clinical features of COVID-19 patients with diabetes and secondary hyperglycemia, Journal of diabetes research , vol. 2020, 2020.
Allard R, Leclerc P, Tremblay C, Tannenbaum T-N. Diabetes and the severity of pandemic influenza A (H1N1) infection, Diabetes care , vol. 33, no. 7, pp. 1491–1493, 2010.
Fink AL, Engle K, Ursin RL, Tang W-Y, Klein SL. Biological sex affects vaccine efficacy and protection against influenza in mice, Proceedings of the National Academy of Sciences , vol. 115, no. 49, pp. 12477–12482, 2018.
Prattichizzo F, et al. Prevalence of residual inflammatory risk and associated clinical variables in patients with type 2 diabetes. Diabetes Obes Metabolism. 2020;22(9):1696–700.
Bonnet F, Scheen A. Effects of SGLT2 inhibitors on systemic and tissue low-grade inflammation: the potential contribution to diabetes complications and cardiovascular disease. Diabetes Metab. 2018;44(6):457–64.
Hotamisligil GS. Inflammation, metaflammation and immunometabolic disorders, Nature , vol. 542, no. 7640, pp. 177–185, 2017.
Trimarco V, Izzo R, Lombardi A, Coppola A, Fiorentino G, Santulli G. Beneficial effects of L-Arginine in patients hospitalized for COVID-19: new insights from a randomized clinical trial, (in eng). Pharmacol Res. May 2023;191:106702. https://doi.org/10.1016/j.phrs.2023.106702
Download references
Acknowledgements
This study was performed as part of doctoral dissertation in general medicine by Somayyeh Salajegheh. We also thank individuals and organizations who participated in this research.
This study was funded by Kerman University of Medical Sciences, Kerman, Iran, (grant number 400000947).
Author information
Authors and affiliations.
Endocrinology and Metabolism Research Center, Kerman University of Medical Sciences, Kerman, Iran
Faranak Salajegheh
Clinical Research Development Unit, Afzalipour Hospital, Kerman University of Medical Sciences, Kerman, Iran
Faranak Salajegheh, Mohsen Nakhaie, Reza Sinaei & Soheila Torabiyan
Physiology Research Center, Institute of Neuropharmacology, Kerman University of Medical Sciences, Kerman, Iran
Somayyeh Salajegheh
Gastroenterology and Hepatology Research Center, Institute of Basic and Clinical Physiology Sciences, Kerman University of Medical Sciences, Kerman, Iran
Mohsen Nakhaie, Niloofar Farsiu & Seyedeh Mahdieh Khoshnazar
Research Center of Tropical and Infectious Diseases, Kerman University of Medical Sciences, Kerman, Iran
Mehrdad Farrokhnia
You can also search for this author in PubMed Google Scholar
Contributions
Study concept and design: FS and MF. Analysis and interpretation of data: MN and NF. Drafting of the manuscript: SS, RS and ST. Statistical analysis: SMK. Critical revision of the manuscript for important intellectual content: MN.
Corresponding author
Correspondence to Mohsen Nakhaie .
Ethics declarations
Ethics approval and consent to participate.
This research was approved by the Ethics Committee of Kerman University of Medical Sciences, Kerman, Iran (IR.KMU.AH.REC.1400.319).
Consent for publication
Not applicable.
Competing interests
The authors declare that they have no potential conflicts of interest.
Additional information
Publisher’s note.
Springer Nature remains neutral with regard to jurisdictional claims in published maps and institutional affiliations.
Rights and permissions
Open Access This article is licensed under a Creative Commons Attribution 4.0 International License, which permits use, sharing, adaptation, distribution and reproduction in any medium or format, as long as you give appropriate credit to the original author(s) and the source, provide a link to the Creative Commons licence, and indicate if changes were made. The images or other third party material in this article are included in the article’s Creative Commons licence, unless indicated otherwise in a credit line to the material. If material is not included in the article’s Creative Commons licence and your intended use is not permitted by statutory regulation or exceeds the permitted use, you will need to obtain permission directly from the copyright holder. To view a copy of this licence, visit http://creativecommons.org/licenses/by/4.0/ . The Creative Commons Public Domain Dedication waiver ( http://creativecommons.org/publicdomain/zero/1.0/ ) applies to the data made available in this article, unless otherwise stated in a credit line to the data.
Reprints and permissions
About this article
Cite this article.
Salajegheh, F., Salajegheh, S., Nakhaie, M. et al. The relationship between COVID-19 and hyperglycemia: screening and monitoring hospitalized patients. Clin Diabetes Endocrinol 10 , 29 (2024). https://doi.org/10.1186/s40842-024-00184-7
Download citation
Received : 12 March 2024
Accepted : 08 May 2024
Published : 31 August 2024
DOI : https://doi.org/10.1186/s40842-024-00184-7
Share this article
Anyone you share the following link with will be able to read this content:
Sorry, a shareable link is not currently available for this article.
Provided by the Springer Nature SharedIt content-sharing initiative
- Hyperglycemia
- New-onset diabetes
Clinical Diabetes and Endocrinology
ISSN: 2055-8260
- Submission enquiries: Access here and click Contact Us
- General enquiries: [email protected]

An official website of the United States government
The .gov means it’s official. Federal government websites often end in .gov or .mil. Before sharing sensitive information, make sure you’re on a federal government site.
The site is secure. The https:// ensures that you are connecting to the official website and that any information you provide is encrypted and transmitted securely.
- Publications
- Account settings
Preview improvements coming to the PMC website in October 2024. Learn More or Try it out now .
- Advanced Search
- Journal List
- v.57(11); 2008 Nov

Viral Trigger for Type 1 Diabetes
Christophe m. filippi.
From the La Jolla Institute for Allergy and Immunology, La Jolla, California
Matthias G. von Herrath
The most popular hypothesis circulating within and beyond the scientific community is that viral infections enhance or elicit autoimmune disorders such as type 1 diabetes. Indeed, viruses can injure β-cells and have been isolated in pancreatic tissues from diabetic patients. However, accumulating evidence suggests that the opposite scenario, which is prevention or amelioration of type 1 diabetes, might be at least as common an outcome of viral infection. Here, we discuss epidemiological and experimental evidence for the main mechanisms accounting for the role of viruses in type 1 diabetes to better understand the complex relationship between viral infections and autoimmune diabetes.
INSIGHT FROM EPIDEMIOLOGY AND CLINICAL INVESTIGATIONS
The influence of the environment..
Type 1 diabetes is a genetic autoimmune disorder caused by autoreactive CD4 + and CD8 + T-cells that recognize pancreatic antigens such as insulin or GAD and subsequently destroy insulin-producing β-cells. The subject of very active research is the question of how endogenous β-cell antigens become immunogenic. Infiltration of the islets of Langerhans, where β-cells reside, by activated autoreactive T-cells is considered to be the major driving force in type 1 diabetes progression. The islet infiltrate in humans consists primarily of CD8 + T-cells and B-cells, followed by macrophages and dendritic cells of different subtypes ( 1 ). Interestingly, significantly fewer T-cells are found in human islets compared with islets from nonobese diabetic (NOD) mice. The reduced numbers of T-cells, and in this way a limited autoreactive component in human islets, leads one to consider whether other contributing factors may be involved in disease development. Otherwise, sufficient insulitic infiltrate to destroy islet β-cells might not be easily maintained in humans. Further supporting a role for nongenetic factors in the control of type 1 diabetes is the observation that disease concordance among monozygotic twins is below 50% ( 2 ). Migrant studies also suggest the involvement of an environmental factor in type 1 diabetes, since disease incidence in migrating populations appears to conform to the incidence of the region to which there is migration ( 3 ). There is an ever-increasing body of literature suggesting that the significant environmental component to type 1 diabetes development and progression is a viral infection. However, this has not been clearly demonstrated. In fact, viral infections appear to have both detrimental and protective effects on type 1 diabetes development, which might be contingent upon the nature of the virus, but also the immune status of the host and thus the timing of infection.
Certain viruses might promote autoimmunity.
A significant number of viruses have been associated with type 1 diabetes, including enteroviruses such as Coxsackievirus B (CVB) ( 4 ), but also rotavirus ( 5 , 6 ), mumps virus ( 7 ), and cytomegalovirus ( 8 ). Rubella virus has been suggested to cause type 1 diabetes, but so far only congenital rubella syndrome has conclusively been associated with the disease ( 9 – 11 ). The prime viral candidates for causing type 1 diabetes in humans are enteroviruses. Enterovirus infections are more frequent in siblings developing type 1 diabetes compared with nondiabetic siblings, and enterovirus antibodies are elevated in pregnant mothers whose children later develop type 1 diabetes ( 12 ). Interestingly, studies in the Finnish population demonstrated that appearance of autoantibodies in genetically susceptible children paralleled the seasonal pattern of enterovirus infections ( 13 ). More specifically, a temporal association has been observed between the appearance of the first autoantibodies and signs of enterovirus infection both among siblings of affected children and among children with increased HLA-conferred diabetes susceptibility ( 14 ).
CVB4 is the most common enteroviral strain found in pre-diabetic and diabetic individuals. CVB RNA has been detected in blood from patients at the onset or during the course of type 1 diabetes ( 15 , 16 ). Furthermore, cellular immune responses to CVB antigens were found to be enhanced in type 1 diabetic patients after the onset of the disease ( 17 ). One CVB4 strain was isolated from the pancreas of a deceased diabetic child, passaged through murine β-cells, and found to induce diabetes after inoculation in mice ( 18 ). Recently, Dotta et al. ( 19 ) also detected CVB4 in pancreatic tissue specimens from three of six type 1 diabetic patients. Elshebani et al. ( 20 ) recently found that enterovirus isolates obtained from newly diagnosed type 1 diabetic patients could infect and induce destruction of human islet cells in vitro. Recently, Oikarinen et al. ( 21 ) have isolated enteroviruses from intestinal biopsy samples in 75% of type 1 diabetes cases versus 10% of control patients, possibly reflecting persistent enterovirus infection of gut mucosa in type 1 diabetic patients. In sum, isolation of enteroviral antigens from diabetic individuals, particularly after recent onset, is becoming a fairly reproducible finding, supporting a role for these viruses in disease development. However, it is still unclear whether this phenomenon is indeed a common etiology for the majority of patients diagnosed with type 1 diabetes, or whether it can be found only in a particular subpopulation of individuals with perhaps higher genetic susceptibility to infection.
The molecular means by which enteroviruses could enhance type 1 diabetes is a topic of significant inquiry. Virus infections activate strong immune responses. CVB4 infection of islet cells was indeed reported to induce strong inflammation mediated by natural killer (NK) cells within the islets ( 19 ). If the virus can, in addition, promote direct cytolysis of β-cells, autoantigens are introduced in a context of heightened immune response and inflammation. This might be the case after direct infection of β-cells by the virus. Enteroviruses might target β-cells via surface molecules such as the poliovirus receptor and integrin α v β 3 . Both of these molecules are expressed on human β-cells and can act as enterovirus receptors in established cell lines ( 22 ). Infections by viruses that target β-cells and promote strong inflammation within the islets may thus represent the initial step in the induction of autoimmunity. However, studies on human pancreata or cultured islets have shown that there are considerable variations in the adverse effects of enteroviruses on β-cells, not only between various viral serotypes, but also between strains of the same serotype ( 23 – 25 ). While the mechanism by which viruses might induce autoimmunity is not understood, viral infections might be capable of “unmasking” β-cells for recognition by CD8 + T-cells by promoting interferon production and upregulation of major histocompatibility complex (MHC) class I molecules on β-cells. These events combined may be sufficient to condition the pancreatic islets for autoimmune attack. In this respect, Foulis et al. ( 26 ) described MHC class I upregulation and interferon induction in noninfiltrated islets obtained from presumably pre-diabetic individuals. Although this may possibly be a postmortem artifact, such findings should prompt larger-scale investigations, for example, on freshly obtained tissues via nPOD (Network for Pancreatic Organ Donors with Diabetes; www.jdrfnpod.org ). Access to fresh pre-diabetic pancreata is needed to conduct valid viral studies.
Viruses may be wrongly accused.
The possibility that enteroviruses promote autoimmunity suggests that vaccination, which will impair viral infection of the islets, may provide protection against type 1 diabetes. Accordingly, an earlier report suggested that lower type 1 diabetes incidence in Estonia compared with Finland may be associated with polio vaccination schedule, resulting in stronger immunity to diabetogenic enterovirus infections ( 27 ). Similarly, while a significant increase in the number of type 1 diabetes cases was observed in Finland 2–4 years after a mumps epidemic ( 7 ), the incidence of type 1 diabetes reached a plateau 6 years after introduction of the mumps-measles-rubella vaccine ( 28 ). However, there is significant epidemiological data contradicting the involvement of viruses as causative agents in type 1 diabetes. There exists a geographical north-south gradient indicative of an inverse correlation between “hygiene” and incidence of autoimmune disease (as well as allergy). Countries such as Finland versus Venezuela/China, or wider regions such as Northern versus Southern Europe (with the exception of Sardinia), represent areas where socioeconomics correlate closely with type 1 diabetes prevalence. Reduced type 1 diabetes incidence is observed in countries of lower socioeconomic status, which is associated with a higher rate of infection. This phenomenon may also be related to the use of particular vaccine strategies in countries exhibiting different sanitary standards. It is also interesting to note that many type 1 diabetic patients are first born of large families, possibly indicative of lower exposure to infections. In addition, while congenital infections have been proposed to account for type 1 diabetes development in the offspring, the use of antimicrobials by mothers before pregnancy and subsequently by the child was suggested to be associated with higher risk for type 1 diabetes ( 29 ). Increased diabetes incidence in the Western world may thus be reflective of the “ultra-clean living” phenomenon. In the “hygiene hypothesis,” reduced rates of infection contribute to increased type 1 diabetes incidence, not supporting a disease-inductive role for viruses. Alternatively, it has been argued that reduced frequencies of infection may result in increased susceptibility to the effect of diabetogenic viruses ( 30 , 31 ). Regardless, although there is significant evidence for viral penetration of pancreatic tissue from type 1 diabetic patients, exposure to viruses does not appear to be necessarily causative of type 1 diabetes and may in fact be beneficial in some cases. This could be an indication that the immune system can be educated to better deal with inflammatory disorders by being frequently exposed to inflammatory events over life.
INSIGHT FROM EXPERIMENTAL WORK
While epidemiological studies have shed important insight into the association between viral infections and autoimmune diabetes in humans, a significant body of evidence is derived from investigations using animal models for type 1 diabetes. Notably, NOD mice are susceptible to spontaneous type 1 diabetes that develops slowly over several weeks and mimics most aspects of human type 1 diabetes ( 32 ). In NOD mice, nondestructive insulitis develops in the pancreas during the pre-diabetic phase and, although this period is variable, most mice go on to develop T-cell–mediated destruction of β-cells leading to overt diabetes. NOD mice thus constitute a critical tool to address how exposure to viral infection during the pre-diabetic phase will affect subsequent disease development. In humans, the prime candidates for infectious causes of type 1 diabetes are enteroviruses such as CVB. In mice, and most notably in NOD mice, CVB also appears associated with the development of autoimmunity. Early studies have shown that infection of normal mice with CVB4 causes a diabetic state associated with low insulin levels consistent with islet cell destruction ( 33 ). CVB4 has since been shown to be tightly associated with the initiation of type 1 diabetes in the NOD mouse. However, the influence of the virus appears to be contingent upon the precise point in time at which infection occurs ( 34 ). The B3 strain of CVB3, in contrast, mediates significant protection against type 1 diabetes development in NOD mice regardless of the time of infection ( 35 ). However, as discussed below, CVB3 and CVB4 differ regarding tropism for pancreatic tissue, which may account for the differential effect of these two stains in autoimmune diabetes. Importantly in that respect, there might be fundamental difference between rodents and humans regarding tropism of enteroviruses for pancreatic β-cells. One should thus be cautious when extrapolating rodent data to human type 1 diabetes in the context of enterovirus infections. Interestingly, other viruses that are also thought to be associated with the pathogenesis of type 1 diabetes in animals have been shown to mediate protective effects in some instances. For example, the D variant of encephalomyocarditis virus (EMC-DV) was found to induce β-cell destruction and subsequent type 1 diabetes in the mouse, but this was found to occur in a T-cell–independent fashion. EMC-DV was also reported to diminish autoimmunity in diabetes-prone NOD mice ( 36 – 38 ). In the BioBreeding diabetes-resistant (BB-DR) rat, however, it was shown that infection with Kilham's rat virus induces autoimmune diabetes ( 39 ). But while the use of animals has clearly established that particular viruses are capable of inducing autoimmune diabetes, it has also evidenced that these viruses can play a preventive role in the development of the disease. Two different, yet non–mutually exclusive, mechanisms have been proposed to account for the causative role of viruses in type 1 diabetes. Detailed insight into these mechanisms could provide better understanding of the dual roles played by viral infections in autoimmune diabetes.
Molecular mimicry might enhance but not initiate autoimmunity.
One of the two mechanisms by which viruses may be capable of initiating type 1 diabetes is termed molecular mimicry. If the T-cell receptors expressed by particular autoreactive T-cells enable these cells to recognize viral antigens (or vice versa), both autoreactive and antiviral T-cells have the potential to become activated as a consequence of presentation of viral antigens by antigen-presenting cells (APCs). Activation of autoreactive T-cells upon CVB4 infection was proposed to occur by molecular mimicry, since the P2-C protein sequence of the virus partially resembles that of human GAD, a protein expressed in the islets as well as other nervous tissues ( 40 ). T-cells from patients at risk for type 1 diabetes were found to recognize a GAD determinant that shares significant sequence similarity with the P2-C protein of CVB4, and patients whose T-cells responded to this particular GAD determinant were found to also respond to a Coxsackie viral peptide ( 41 ). However, antibodies present in GAD-positive sera from patients with type 1 diabetes were not found to cross-react with P2-C ( 42 ). Cross-reactivity between GAD and P2-C was further assessed using the NOD mouse model for type 1 diabetes, where it was determined that the common region of these two proteins is immunodominant and presented to cross-reactive T-cells only in the context of a NOD diabetes susceptibility MHC allele ( 43 ). Cross-reactivity between P2-C and GAD was thus proposed to account for the capacity of CVB4 to induce type 1 diabetes in genetically predisposed humans. However, Horwitz et al. ( 44 ) found that congenic B10.H2 g7 mice, which carry the NOD MHC allele but lack other type 1 diabetes susceptibility factors, do not develop diabetes upon CVB4 infection. Moreover, infection of BDC2.5 transgenic mice, which express a T-cell receptor specific for an islet antigen that does not cross-react with the CVB4 P2-C protein was able to induce type 1 diabetes in the majority of these otherwise non–diabetes-prone mice. Therefore, cross-reactivity between P2-C and GAD may not by itself account for initiation of type 1 diabetes but might act as an essential enhancer of disease once autoimmune attack of β-cells has been initiated.
The rat insulin promoter (RIP)–lymphocytic choriomeningitis virus (LCMV) system is a mouse model in which diabetes is initiated by viral infection ( 45 – 47 ). In this model, RIP-LCMV mice transgenically express the glycoprotein or nucleoprotein of LCMV as a target antigen in their islets under the control of the RIP. Infection of these mice with LCMV breaks peripheral responsiveness to glycoprotein/nucleoprotein, leading to attack of β-cells by T-cells and eventual development of type 1 diabetes. Importantly, this model suggests that viral infection is able to induce autoimmunity only if homology between viral and β-cell antigens is 100%, since a single amino acid change flanking a cytotoxic T-lymphocyte (CTL) epitope was found to interfere with the development of type 1 diabetes ( 48 ). These data further support the hypothesis that molecular mimicry alone might not be capable of inducing type 1 diabetes but rather be an essential precipitator once autoimmunity has been initiated. Indeed, we previously reported that an LCMV mimic ligand can accelerate preexisting autoimmunity by inducing autoreactive T-cells to proliferate and localize in the islets, yet does not generate sufficient autoreactive T-cells to initiate disease in naive mice ( 49 ). We propose that viral infections alone will not initiate autoimmunity but rather act to provide a “fertile field” for further expansion of activated autoreactive T-cells, leading to autoimmune disease ( 50 ).
Bystander mechanisms induce APC activation and initiate autoimmunity.
While molecular mimicry may enhance autoimmune responses, the central role in induction of autoimmunity by viruses might be played by the proinflammatory/inflammatory mediators produced upon infection. Hence, bystander activation of autoreactive T-cells could occur during a viral infection with heterologous antigenic specificity and result in autoimmune disease. This might be the consequence of inflammation inducing tissue damage and release of sequestered islet antigens, leading to enhanced autoantigen presentation by APCs. Accordingly, CVB4-induced type 1 diabetes was found to be associated with initial phagocytosis of CVB4-infected β-cells by macrophages, leading to increased presentation of islet antigens, which promoted type 1 diabetes ( 51 ). Limited injury to β-cells using the islet-damaging agent streptozotocin was found to induce type 1 diabetes in BDC2.5 mice similar to CVB4 infection, as a consequence of release of β-cell antigens followed by their presentation by macrophages ( 52 ). Of note, injection of insulin along with poly-IC (polyinosinic-polycytidylic acid), which mimics double-stranded viral RNA, was found to induce anti-islet autoimmunity ( 53 ). Thus, virally induced release of β-cell antigen under inflammatory conditions might promote type 1 diabetes through activation of APCs such as macrophages, which have been shown to play a crucial role in the development of spontaneous diabetes ( 54 , 55 ). In particular, APCs themselves can release inflammatory and proinflammatory mediators as a result of viral infection. Likewise in the RIP-LCMV model, in the absence of infection, the glycoprotein/nucleoprotein protein is not expressed by costimulation-competent APCs, and the autoimmune process is only initiated when sufficient numbers of activated autoreactive T-cells are generated, after activation of APCs in the pancreas ( 56 , 57 ). Interestingly, activated T-cells from BDC2.5 mice are unable to induce diabetes in the mouse in the absence of CVB4 infection, which supports the possibility that bystander activation of APCs through virally mediated inflammation is necessary for efficient activation of autoreactive T-cells ( 44 ). Accordingly, we reported that type 1 diabetes develops in the absence of infection in the RIP-LCMV system when APCs are rendered costimulation-competent through transgenic expression of the B7–1 (CD80) costimulatory molecule ( 58 ). Importantly, in the RIP-LCMV system, APCs presenting self-antigens not only mediate priming of autoreactive T-cells, but also help maintain a peripheral immune response in the pancreatic islets ( 59 ). Thus, antigen presentation appears to play a crucial role not only in inducing but also sustaining the diabetogenic response.
Viral infection and local β-cell injury.
Initial damage to β-cells and uptake of autoantigen by APCs appear crucial in the initiation of autoimmunity upon viral infection. If a particular virus is highly lytic for β-cells, insulin deficiency and type 1 diabetes will result when more than 90% of β-cells are destroyed ( 60 , 61 ). This scenario can notably be observed after high-dose infection with EMC-DV, in which case type 1 diabetes is nonautoimmune in nature ( 62 , 63 ). However, limited injury to β-cells, caused by the virus or antiviral mechanisms, can lead to initial release of sequestered self-antigens and ultimately their presentation by APCs, which in turn promote further β-cell damage by activating autoreactive T-cells. Inflammatory cytokines produced during viral infection may play a crucial role in initial destruction of β-cells. Using the RIP-LCMV model, we found that upon viral infection, systemic production of interferon (IFN)-γ can directly cause β-cell destruction in the islets ( 64 ). Furthermore, inflammatory cytokines such as type I and II interferons may indirectly contribute to β-cell death by inducing upregulation of MHC class I by these cells, thereby “unmasking” them for recognition by autoreactive T-cells. It was reported that immunization with an LCMV-derived peptide does not induce type 1 diabetes in RIP-LCMV mice in the absence of IFN-α production and MHC class I upregulation in the islets ( 65 ). Furthermore, our work has shown that upregulation of MHC class II and activation of APCs within the pancreatic islets is required for β-cell destruction by activated autoreactive T-cells ( 56 ). Thus, cytokines produced upon infection by viruses exhibiting pancreatic tropism might be capable of preconditioning the islets for initial autoimmune attack of β-cells, similar to the possible scenario in humans.
Studies in the mouse further indicate that activation of Toll-like receptor (TLR) signaling might play a crucial part in the process. TLR ligation was shown to induce type 1 diabetes by increasing IFN-α and MHC class I expression in the islets of RIP-LCMV mice immunized with an LCMV-derived peptide ( 65 ). Similarly, in the BB-DR rat, initiation of autoimmune diabetes by Kilham's rat virus was found to be enhanced through activation of TLR signaling and induction of inflammatory cytokine production by APCs ( 66 , 67 ). More recently, Kim et al. ( 68 ) reported that activation of the TLR2 signaling pathway by secondary apoptotic β-cells might participate in the initiation of type 1 diabetes by inducing tumor necrosis factor-α (TNF-α) production by macrophages. However, TLR2 signaling was also recently suggested to enhance immune regulation ( 69 ), and it was previously reported that limited apoptosis of β-cells decreases diabetes incidence in NOD mice ( 70 ). In addition, as discussed below, TNF-α may have a protective function in type 1 diabetes depending on the time of action. Yet, regardless of the underlying mechanism, the observation by Kim et al. suggests that the mode by which initial injury to β-cells occurs is a crucial determinant in the induction of autoimmunity. Therefore, direct viral tropism for β-cells could play a major role in the capacity of viruses to mediate type 1 diabetes. Of note, CVB4 has a direct tropism for β-cells and exhibits a differential effect in type 1 diabetes depending on the time of infection, whereas CVB3 infects the acinar cells of the exocrine pancreas and prevents disease regardless of the time of infection ( 34 , 35 ). Although CVB4 infection of β-cells may not directly cause their demise ( 51 ), CVB3 and CVB4 do not mediate similar injury to these cells, which may account for their differential role in type 1 diabetes ( 68 ). Modulation of autoimmune diabetes by viruses may thus depend in part on their capacity to influence the mode and extent of β-cell death, which both appear as crucial factors influencing the course of the disease ( 71 ).
The importance of timing.
Another major component determining virally mediated modulation of autoimmunity appears to be the time at which infection occurs during the pre-diabetic phase. Whereas type 1 diabetes is enhanced in 8-week-old NOD mice infected with CVB4, infection of younger mice has no effect on disease outcome ( 34 ). This suggests that the status of autoimmune progression is a crucial determinant in the diabetogenic potency of the virus. Importantly, just like viral infections, inflammatory cytokines appear to play a dual role in autoimmune diabetes. Previous work has shown that expression or neutralization of cytokines commonly produced during viral infections has opposing effects on type 1 diabetes outcome depending on the time of expression. For instance, we found that early neutralization of TNF-α abrogates type 1 diabetes in RIP-LCMV mice, while at later time points, this cytokine appears to play a beneficial role by diminishing the number and activity of autoreactive T-cells ( 72 , 73 ). Thus, the capacity of particular viral infections to modulate autoimmunity at a certain point in time might be the direct consequence of their ability to promote inflammation during the pre-diabetic process beyond a particular autoimmune threshold. Accordingly, it was reported that enhancement of diabetes by CVB4 infection occurs only after a critical mass of activated autoreactive T-cells has accumulated in the islets ( 74 ). However, CVB3 was reported to prevent disease in both young and older mice ( 35 ). This suggests that while timing is important, the state of advancement of autoimmunity at the time of infection is not the sole explanation for the dual role of viruses in type 1 diabetes.
Impairment of autoimmunity may occur through bystander effects.
APC activation and associated inflammation, whether or not induced by viral infection, may not always have detrimental consequences in autoimmune diabetes. As discussed above, epidemiological studies provide evidence that infectious events occurring during early childhood might have the ability to prevent or delay type 1 diabetes development ( 75 ). The ability of viral infections to abrogate autoimmune diabetes was also reported in different animal models using not only CVB3 ( 35 ), but also LCMV ( 46 , 76 , 77 ), EMC-DV ( 38 ), mouse hepatitis virus ( 78 ), and lactate dehydrogenase virus ( 79 ). Interestingly, both acute and persistent viral infections appear capable of modulating the immune system in a diabetes-preventive fashion. While the mechanisms accounting for the beneficial effect of viruses on the immune system are poorly understood and may vary from one individual to the next (or one mouse model to the next), a feature common to viral infections is their paradoxical capacity to induce inflammation. This is also the case for a number of nonviral infections, vaccines, or treatments reported to protect NOD mice against diabetes ( 80 – 89 ). In fact, in some cases, type 1 diabetes can be inhibited by direct treatment of pre-diabetic mice with proinflammatory or inflammatory cytokines such as type I interferons, IFN-γ, or interleukin-2 ( 81 , 90 – 93 ), or by inducing the production of similar factors via stimulation of innate immunity ( 81 , 82 , 85 , 94 ). In the RIP-LCMV system, although viral infection represents the event initiating autoimmunity and diabetes, we have shown that inflammation mediated upon viral challenge can prevent type 1 diabetes. Our results indicate that secondary infection of RIP-nucleoprotein mice with a different strain of LCMV during the pre-diabetic phase completely abrogates diabetes development ( 95 ). This phenomenon is dependent on IFN-γ and TNF-α production and results from the recruitment of activated T-cells away from the islet infiltrate toward the pancreatic draining lymph node, as a consequence of selective expression of the chemokine IP-10 (CXCL10). In these studies, the LCMV strain used to prevent diabetes shares a homologous nucleoprotein sequence with that used to initiate diabetes, and thus the two infections activate comparable T-cell responses. Therefore, inflammatory cytokines and chemokines produced during viral infection might play a crucial part in controlling the location of virally activated autoreactive T-cells and their subsequent capacity to infiltrate pancreatic islets.
Antiviral T-cell memory and autoimmune diabetes.
Previous reports suggest that part of the T-cells activated during viral infection can cross-react with new infectious agents or allo-antigens and modulate immunity to unrelated antigens ( 96 – 98 ). Consequently, such heterologous immunity might result in the accumulation of memory T-cells of specificity unrelated to the original viral agent ( 99 ). It is thus possible that the memory T-cell pool generated during life comprises autoreactive/cross-reactive T-cells induced nonspecifically as a consequence of cumulative or chronic viral infections. Since stimulated memory T-cells respond to antigen more rapidly and efficiently than naive cells, repeated or sustained antiviral immunity during life may eventually favor autoimmunity. While in most cases, this phenomenon will not result in autoimmune disease, it might be a prerequisite for overt diabetes in genetically predisposed individuals. As discussed above, in different mouse models, initiation of diabetes by viral infection requires a critical mass of autoreactive T-cells along with activated APCs ( 34 , 56 – 58 , 100 ), and it is possible that, in humans, such a mass is provided progressively over life by repeated or sustained viral infections. In other words, autoimmunity might not be induced de novo at type 1 diabetes onset, and the autoreactive T-cell pool is likely comprised of cells that have already responded to antigenic stimulation during viral infection in the past. On the other hand, previous work suggests that repeated or sustained encounters with viral antigen during chronic infection is associated with protection against type 1 diabetes ( 46 , 77 ). This may be due in part to exhaustion of T-cell immunity, which is commonly found in chronic viral infection and was notably reported in protracted LCMV infection ( 101 ). Alternatively, or in addition, diabetes abrogation during chronic viral infection may be the consequence of virally induced regulatory mechanisms suppressing antiviral immunity and possibly autoimmunity as well ( 102 , 103 ). In this respect, a number of chronic as well as acute infections have been reported to induce the activation of regulatory T-cells (Tregs), in particular naturally occurring Tregs (CD4 + CD25 + Tregs), which are known to play a crucial role in the control of autoimmunity ( 104 – 108 ). Thus, repeated exposure to viral antigen during life may not necessarily be pathogenic in autoimmunity. Nonspecific activation of autoreactive T-cells as a consequence of repeated or protracted viral infections may even be beneficial in some cases. Notably, CD4 + CD25 + Tregs, which are selected in the thymus and thought to react to self-antigens in the periphery ( 109 , 110 ), can in essence be considered “autoreactive” and may thus be beneficially activated in heterologous immunity. In fact, we found that CD4 + CD25 + Tregs are modulated during viral infection and become capable of halting the course of type 1 diabetes (C.M.F., unpublished data). In addition, our results suggest that resemblance between viral and β-cell antigens can, in some cases, promote activation of diabetes-preventive CD4 + CD25 + Tregs.
CLINICAL IMPLICATIONS: DO VIRAL INFECTIONS INDUCE OR PREVENT TYPE 1 DIABETES?
A number of epidemiological studies support the hypothesis that viral infections play a causative role in type 1 diabetes. However, systematic review of control studies published between 1966 and 2002 has shown no convincing evidence for or against an association between type 1 diabetes and the prime candidate for infectious cause, CVB ( 111 ). In animal models for type 1 diabetes, solid evidence supporting an inductive role for viruses is faced with just as solid evidence supporting a protective effect of viral infections. Based on mouse studies alone, there is no doubt that association between viruses and type 1 diabetes is extremely complex: while belonging to the same enteroviral group, CVB3 and CVB4 have opposing effects on type 1 diabetes in the same mouse model; LCMV initiates diabetes in the RIP-LCMV model but prevents disease in the NOD model; and to make matters more complicated, CVB4 and LCMV are capable of both inducing and preventing diabetes in the same mouse model depending on the time of infection. Thus, the reason for current failure to associate a particular virus with induction of autoimmune diabetes likely is that such an association might be impossible to make. Certain viruses might be capable of inducing diabetes and others of preventing diabetes, and type 1 diabetes inducers might be capable of preventing disease under certain conditions. This will depend of course on the nature of the considered virus (resemblance with β-cell antigens; tropism for β-cells; induction of a chronic infection), but also on the state of advancement of autoimmunity at the time of infection (generation of sufficient numbers of autoreactive T-cells; nature of the cytokine milieu systemically and in the islets). A given viral infection could thus be an essential disease precipitator once required predisposing events have occurred, but could on the other hand disrupt accumulation of these events.
Most important is the indication from animal studies that modulation of autoimmunity during viral infection does not depend merely on inherent properties of the virus, but also significantly on intrinsic factors of the host. The close interplay between the two will dictate whether enhancement or abrogation of autoimmune diabetes occurs. While molecular mimicry might activate autoreactive T-cells, it could also segregate these cells away from the islets and/or induce the activation of protective Tregs. While inflammatory cytokines might promote bystander activation of APCs and autoreactive T-cells, infection could occur at a time where inflammation will induce the relocation or demise of these cells. Whereas β-cell lysis and presentation of islet antigen might promote activation of autoreactive T-cells, it could also suppress the function of these cells by promoting Treg activity. Whereas repeated/sustained infections might lead to the accumulation of autoreactive T-cells within the memory pool, they could also induce suppressor mechanisms that will hinder autoimmunity. These possibilities are illustrated in Fig. 1 .

Interplay between virus- and host-intrinsic properties dictates whether enhancement or abrogation of type 1 diabetes occurs. β = β cells. Teff, effector (autoreactive) T-cells.
Based on current evidence, it thus appears impossible to assess the capacity of viruses to modulate type 1 diabetes without knowledge of the state of advancement of autoimmunity and infection history of affected individuals. This is no easy task, but tremendous effort is currently being made in the U.S. and Europe to closely monitor exposure to infections in individuals at risk for type 1 diabetes. In particular, the TEDDY (The Environmental Determinants of Diabetes in the Young) study is currently assessing the influence of environmental factors, among which are viral infections, on the development of autoimmune diabetes. In this study, blood from children with increased genetic risk for type 1 diabetes is assessed for viral exposure every 3 months for the first 4 years of life, and then every 6 months until the age of 15 years. Stool samples are also assessed for viral exposure at monthly intervals for the first 4 years of life and then biannually until the age of 15. Importantly, as the period of time between a particular viral infection and the initiation of autoimmunity appears variable, occurrence of a possibly critical infectious event might be extremely hard to detect. It thus appears crucial that children with increased genetic risk for type 1 diabetes are monitored not only at regular intervals, but also whenever they are undergoing a viral infection. Closer monitoring of individuals with high risk for type 1 diabetes should give us more convincing evidence for the contribution of an infectious agent to progression toward autoimmunity. In addition, newly obtained mechanisms from experimental investigations will be useful for the development of novel immunotherapy for type 1 diabetes.
Acknowledgments
The authors acknowledge support from the Brehm Coalition.
Readers may use this article as long as the work is properly cited, the use is educational and not for profit, and the work is not altered. See http://creativecommons.org/licenses/by-nc-nd/3.0/ for details.
Thank you for visiting nature.com. You are using a browser version with limited support for CSS. To obtain the best experience, we recommend you use a more up to date browser (or turn off compatibility mode in Internet Explorer). In the meantime, to ensure continued support, we are displaying the site without styles and JavaScript.
- View all journals
- Explore content
- About the journal
- Publish with us
- Sign up for alerts
- Open access
- Published: 10 September 2024
Lethal Borna disease virus 1 infections of humans and animals – in-depth molecular epidemiology and phylogeography
- Arnt Ebinger 1 ,
- Pauline D. Santos ORCID: orcid.org/0000-0003-4324-5967 1 ,
- Florian Pfaff ORCID: orcid.org/0000-0003-0178-6183 1 ,
- Ralf Dürrwald ORCID: orcid.org/0000-0002-3432-0438 2 ,
- Jolanta Kolodziejek ORCID: orcid.org/0000-0001-5736-3644 3 ,
- Kore Schlottau ORCID: orcid.org/0000-0002-3999-0393 1 ,
- Viktoria Ruf 4 ,
- Friederike Liesche-Starnecker ORCID: orcid.org/0000-0003-1948-1580 5 , 6 ,
- Armin Ensser ORCID: orcid.org/0000-0001-8873-3863 7 ,
- Klaus Korn ORCID: orcid.org/0000-0003-1891-2107 7 ,
- Reiner Ulrich 8 ,
- Jenny Fürstenau ORCID: orcid.org/0000-0001-5349-1403 9 ,
- Kaspar Matiasek ORCID: orcid.org/0000-0001-5021-3280 10 ,
- Florian Hansmann ORCID: orcid.org/0000-0001-6064-7728 8 , 11 ,
- Torsten Seuberlich 12 ,
- Daniel Nobach ORCID: orcid.org/0000-0001-6279-7412 13 , 14 ,
- Matthias Müller 15 ,
- Antonie Neubauer-Juric ORCID: orcid.org/0000-0002-9867-1261 16 ,
- Marcel Suchowski 8 , 16 ,
- Markus Bauswein 17 ,
- Hans-Helmut Niller 18 ,
- Barbara Schmidt 17 ,
- Dennis Tappe 19 ,
- Daniel Cadar 19 ,
- Timo Homeier-Bachmann ORCID: orcid.org/0000-0002-8135-3814 20 ,
- Viola C. Haring ORCID: orcid.org/0009-0007-7595-8239 21 ,
- Kirsten Pörtner ORCID: orcid.org/0000-0001-8191-8056 22 ,
- Christina Frank ORCID: orcid.org/0000-0001-6776-8450 22 ,
- Lars Mundhenk ORCID: orcid.org/0000-0002-9033-9360 9 ,
- Bernd Hoffmann ORCID: orcid.org/0000-0001-5358-6445 1 ,
- Jochen Herms ORCID: orcid.org/0000-0002-6201-1042 4 ,
- Wolfgang Baumgärtner ORCID: orcid.org/0000-0001-8151-5644 11 ,
- Norbert Nowotny ORCID: orcid.org/0000-0002-3548-571X 3 , 23 ,
- Jürgen Schlegel 24 ,
- Rainer G. Ulrich ORCID: orcid.org/0000-0002-5620-1528 21 ,
- Martin Beer ORCID: orcid.org/0000-0002-0598-5254 1 &
- Dennis Rubbenstroth ORCID: orcid.org/0000-0002-8209-6274 1
Nature Communications volume 15 , Article number: 7908 ( 2024 ) Cite this article
1 Altmetric
Metrics details
- Viral epidemiology
- Viral reservoirs
- Viral transmission
Borna disease virus 1 (BoDV-1) is the causative agent of Borna disease, a fatal neurologic disorder of domestic mammals and humans, resulting from spill-over infection from its natural reservoir host, the bicolored white-toothed shrew ( Crocidura leucodon ). The known BoDV-1-endemic area is remarkably restricted to parts of Germany, Austria, Switzerland and Liechtenstein. To gain comprehensive data on its occurrence, we analysed diagnostic material from suspected BoDV-1-induced encephalitis cases based on clinical and/or histopathological diagnosis. BoDV-1 infection was confirmed by RT-qPCR in 207 domestic mammals, 28 humans and seven wild shrews. Thereby, this study markedly raises the number of published laboratory-confirmed human BoDV-1 infections and provides a first comprehensive summary. Generation of 136 new BoDV-1 genome sequences from animals and humans facilitated an in-depth phylogeographic analysis, allowing for the definition of risk areas for zoonotic BoDV-1 transmission and facilitating the assessment of geographical infection sources. Consistent with the low mobility of its reservoir host, BoDV-1 sequences showed a remarkable geographic association, with individual phylogenetic clades occupying distinct areas. The closest genetic relatives of most human-derived BoDV-1 sequences were located at distances of less than 40 km, indicating that spill-over transmission from the natural reservoir usually occurs in the patient´s home region.
Similar content being viewed by others
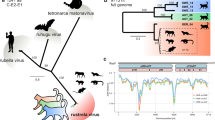
Mystery of fatal ‘staggering disease’ unravelled: novel rustrela virus causes severe meningoencephalomyelitis in domestic cats
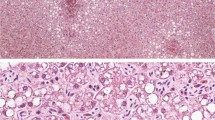
Multidecade Mortality and a Homolog of Hepatitis C Virus in Bald Eagles ( Haliaeetus leucocephalus ), the National Bird of the USA
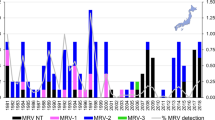
Novel human reovirus isolated from children and its long-term circulation with reassortments
Introduction.
Borna disease virus 1 (BoDV-1, species Orthobornavirus bornaense , family Bornaviridae ) is the causative agent of Borna disease, a severe and often fatal neurologic disease of various domestic mammals, particularly horses, sheep, and New World camelids 1 , 2 , 3 , 4 , 5 . Recently, the virus received increased attention following confirmation of fatal BoDV-1-induced encephalitis in humans 6 , 7 , 8 , 9 , 10 , 11 , 12 , 13 , 14 , 15 , 16 , 17 , 18 . In domestic mammals and humans, BoDV-1 establishes a persistent and strictly neurotropic infection mainly of the central nervous system, with so far no evidence of shedding of infectious virus. The infection usually results in non-purulent encephalomyelitis due to T lymphocyte-mediated immunopathology with a high case fatality rate 1 , 7 , 9 , 19 , 20 . The incubation period is presumably highly variable and assumed to range from several weeks to a few months in most cases 8 , 21 , 22 , which may at least partly be the result of different infectious doses or routes of infection 23 . Affected individuals usually develop fever accompanied by headaches in humans, followed by a broad range of behavioural and neurologic disorders, including apathy, compulsive movements, seizures, ataxia or blindness. In most cases, the disease progresses to coma and death within days to months after the onset of neurological symptoms 1 , 2 , 6 , 7 , 10 , 17 , 24 , 25 . Several compounds have been identified to possess antiviral activity against orthobornaviruses in cell culture but no therapeutic regime for animals or humans has been established yet 26 , 27 , 28 , 29 , though in some human cases intensive treatment attempts were made 15 . As licensed vaccines against BoDV-1 are likewise not available 30 , prophylactic measures are limited to reducing exposure to the BoDV-1 reservoir.
The known natural reservoir host of BoDV-1 is the bicoloured white-toothed shrew ( Crocidura leucodon ), in which BoDV-1 is not strictly neurotropic but also infects epithelial cells in various organs, thereby allowing for shedding of infectious virus via saliva, faeces, urine, and skin scales 4 , 31 , 32 , 33 , 34 . However, the routes of BoDV-1 transmission within shrew populations as well as for spill-over to domestic mammals and humans remain largely unknown 1 , 17 , 34 , 35 . The known distribution range of bicoloured white-toothed shrews covers large parts of the temperate zone of Europe and Western Asia, extending from the Atlantic ocean to the Caspian Sea 36 , 37 . Nevertheless, BoDV-1 appears to be prevalent only in comparably limited regions covering parts of Eastern and Southern Germany, Austria, Switzerland and the Principality of Liechtenstein, based on the occurrence of BoDV-1 infection in spill-over hosts 4 , 7 , 25 , 33 , 38 . Previous work had demonstrated BoDV-1 sequences to constitute four separate phylogenetic clusters (designated 1–4) with two subclusters (1A and 1B), which appear to be associated with different regions within the endemic area 4 , 7 , 8 , 11 , 12 , 25 , 33 , 35 , 38 , 39 , 40 , 41 .
The aim of this study was to provide an in-depth analysis of the molecular epidemiology and phylogeography of BoDV-1, in particular its spatial distribution in Germany and neighbouring countries, and to assess geographic risk areas for potential spill-over transmission to domestic animals and humans. As the available data on the occurrence of BoDV-1 in shrew populations are highly fragmentary and strongly dependent on the activities of a small number of research groups 4 , 25 , 31 , 32 , 33 , 34 , 42 , we collected material from archived and recent cases of Borna disease in domestic mammals, serving as indicators for the presence of the virus. Additional diagnostic samples were obtained from BoDV-1-infected human patients and from bicoloured white-toothed shrews collected in BoDV-1-endemic regions in Germany. BoDV-1 sequences were generated from this material and used for phylogeographic analysis including also sequence data derived from public databases.
RT-qPCR confirmation of BoDV-1 infections in domestic mammals, humans and shrews
Veterinary and human pathologists and diagnostic laboratories submitted fresh-frozen or formalin-fixed paraffin-embedded (FFPE) brain tissue or cerebrospinal fluid (CSF) from 231 suspected BoDV-1 infections in domestic mammals, 29 humans and seven BoDV-1-positive shrews (Table 1 ). The animals originated mainly from the known endemic regions of Germany (Bavaria, BY; Saxony-Anhalt, ST; Saxony, SN; Brandenburg, BB), Switzerland (Grisons, GR), the Principality of Liechtenstein and Austria (Vorarlberg, VA), with few exceptions originating from regions in Germany and Switzerland not previously known to be endemic for BoDV-1 (Fig. 1A ). Quantitative reverse transcription polymerase chain reaction (RT-qPCR) confirmed the BoDV-1 infection for 207 out of 231 domestic mammals (89.6%) and all analysed shrews. Of the 29 human cases analysed in this study, 28 (96.6%) could be confirmed by RT-qPCR. RT-qPCR as well as high-throughput sequencing (HTS) remained negative for FFPE brain sections from a previously published case from Lower Saxony (NI) in 1992 13 , possibly due to low RNA quality resulting from long-term storage of the material.
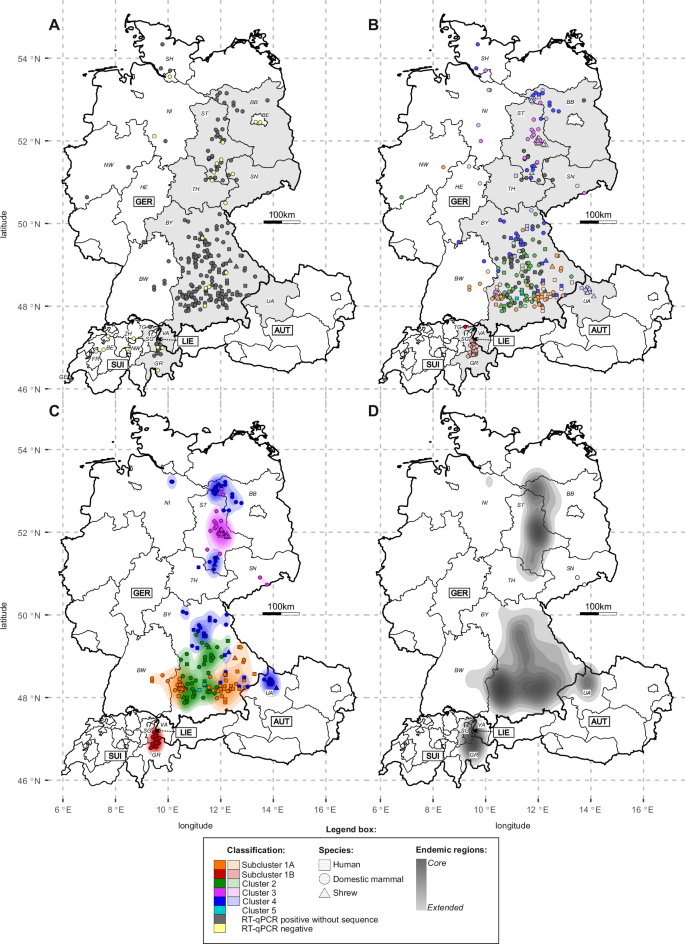
A Origin of suspected or confirmed cases of Borna disease from domestic mammals and humans and of BoDV-1-infected shrews submitted for analysis. Grey symbols represent cases confirmed by BoDV-1-specific RT-qPCR in this study. Yellow symbols represent cases without a positive RT-qPCR result. The federal states (Germany, Austria) and cantons (Switzerland) coloured in light grey represent the assumed endemic regions based on previously published work 4 , 7 , 25 , 33 , 38 , 55 . B Geographic locations of BoDV-1 sequences originating from this study (dark colours) or previously published cases (light colours). Colours represent phylogenetic BoDV-1 clusters and subclusters as determined in Figs. 2 and 3A and Supplementary Fig. 5 . Grey symbols represent cases confirmed by BoDV-1-specific RT-qPCR in this study without available sequence. C Visualisation of endemic regions of BoDV-1 clusters and subclusters by Kernel Density Estimation (KDE). The analysis is based on 214 BoDV-1 sequences with available location. Sequences classified as phylogenetic outliers (no additional sequence with at least 98.6% nucleotide sequence identity within a maximal distance of 37.9 km) were excluded from the analysis. D Cluster-independent BoDV-1 endemic region visualised by KDE. Only sequences meeting the criteria described for panel C ) were included. Germany (GER): BB Brandenburg, BE Berlin, BY Bavaria, BW Baden‐Wuerttemberg, HE Hesse, NI Lower Saxony, NW North Rhine-Westphalia, SH Schleswig-Holstein, SN Saxony, ST Saxony‐Anhalt, TH Thuringia; Switzerland (SUI): BE Bern, FR Fribourg, GE Geneva, GR Grisons, NW Nidwalden, SG St. Gall, TG Thurgau, ZH Zurich; Austria (AUT): UA Upper Austria, VA Vorarlberg; Liechtenstein (LIE).
Of the two BoDV-1-specific RT-qPCR assays used for screening, the matrix protein (M) gene-specific RT-qPCR BoDV-1 Mix-6 yielded significantly lower cycle of quantification (Cq) values for FFPE material than the phosphoprotein (P) gene-specific BoDV-1 Mix-1 ( P < 0.0001; paired Student´s t-test), whereas Mix-1 achieved significantly lower Cq values for fresh-frozen samples ( P < 0.0001; Supplementary Fig. 1 ). The apparently higher sensitivity of Mix-6 RT-qPCR for FFPE-derived RNA, as compared to Mix-1, is presumably due to its shorter amplicon (75 vs. 162 base pairs, bp), allowing for a more efficient detection of highly degraded RNA.
BoDV-1 infections in domestic mammals and humans
We then analysed the metadata available for the cases confirmed in this study in combination with previously published BoDV-1 infections of domestic mammals, humans and shrews, for which at least the BoDV-1 genome sequence spanning the nucleoprotein (N), accessory protein X (X) and P genes (1824 nucleotides [nt]) were available. These cases represented 55 domestic mammals, 16 humans, 36 shrews and 3 laboratory isolates originating from horses (Table 1 ). Samples from most of the 207 confirmed BoDV-1 infections in domestic mammals were collected between 2000 and 2023, but individual cases dated back as far as 1964 (Supplementary Fig. 2 A, B ). The seasonal pattern of Borna disease in domestic mammals was analysed for all RT-qPCR-confirmed cases together with all previously published cases included in this study with available information on month of death (Table 1 ). Death of BoDV-1-infected animals peaked in May and June, whereas the lowest numbers were observed during September to November (Supplementary Fig. 3A ).
This study raises the number of published laboratory-confirmed human BoDV-1 infections to 46 (Table 1 ), following the case definition of Eisermann et al. 11 , which requires direct virus detection from the patient. Twenty-eight cases were confirmed by RT-qPCR during this study (including previously published cases without BoDV-1 sequence; refs. 7 , 9 , 14 , 16 , 17 , 18 , 43 , 44 , 45 ). BoDV-1 sequences were available from public databases for further 16 previously published human cases (Table 1 ) 6 , 7 , 8 , 10 , 11 , 12 , 13 , 15 . Despite the lack of direct virus detection, the donor and the surviving liver recipient of a previously published solid organ transplant cluster were likewise regarded as confirmed cases due to their seroconversion and their unequivocal link to the confirmed BoDV-1 infections in both kidney recipients 8 .
The metadata assembled for all 46 patients (20 females and 26 males) revealed a diagnosis of fulminant encephalitis for 45 patients, with a fatal outcome in 44 of the encephalitic patients (97.8%). The only exceptions from these characteristics were the transplant donor and the liver recipient of the previously published solid organ transplant cluster. While the donor had died of an unknown cause without brain histopathology being performed, the liver recipient survived the acute encephalitis with severe sequelae 8 . The age of the patients ranged from 7 to 79 years (median 53.5 years; Supplementary Fig. 4A ). The first of these confirmed cases occurred in 1996 and had been diagnosed retrospectively 10 . Six patients died in 2016, representing the highest number of confirmed non-transplant-derived cases per year (Supplementary Fig. 2C ). The highest number of deaths was recorded in November (Supplementary Fig. 3B ), while the highest number of hospitalisations was reported in May (Supplementary Fig. 3C ). The median time from hospital admission to death was 29 days (range: 4 to 274 days; Supplementary Fig. 4B ).
Phylogenetic analysis identifies a novel BoDV-1 cluster 5
Sequencing of the BoDV-1 genome was attempted using HTS and/or Sanger sequencing for 157 cases, including 120 domestic mammals, all RT-qPCR-positive humans ( n = 28) and shrews ( n = 7) and the laboratory isolates H24 and DessauVac (Table 1 ). Overall, BoDV-1 sequences covering the complete coding region of the genome ( n = 54) or at least the N and X/P genes ( n = 82) were successfully generated from 136 individuals, including 102 domestic mammals, 25 humans, all seven shrews and both laboratory strains (Table 1 ). Sequencing attempts failed or yielded only short sequence fragments for 18 domestic mammals and three human cases, mainly due to low viral loads and/or insufficient RNA quality in FFPE and CSF samples.
Including the newly generated BoDV-1 sequences as well as those derived from public databases (Table 1 ), two maximum likelihood (ML) trees were constructed for 90 complete coding BoDV-1 genomes (Fig. 2 ) or 246 N-X/P sequences (Fig. 3A and Supplementary Fig. 5 ). Both trees supported the previously published clusters 1 to 4 and the subclusters 1 A and 1B with high statistical support (SH-aLRT ≥80% and ultrafast bootstrap ≥95%). The sequences from two human cases from BY analysed in this study did not fall into any of the previously described clusters but formed a separate cluster 5 basal to them (Figs. 2 and 3A and Supplementary Fig. 5 ).
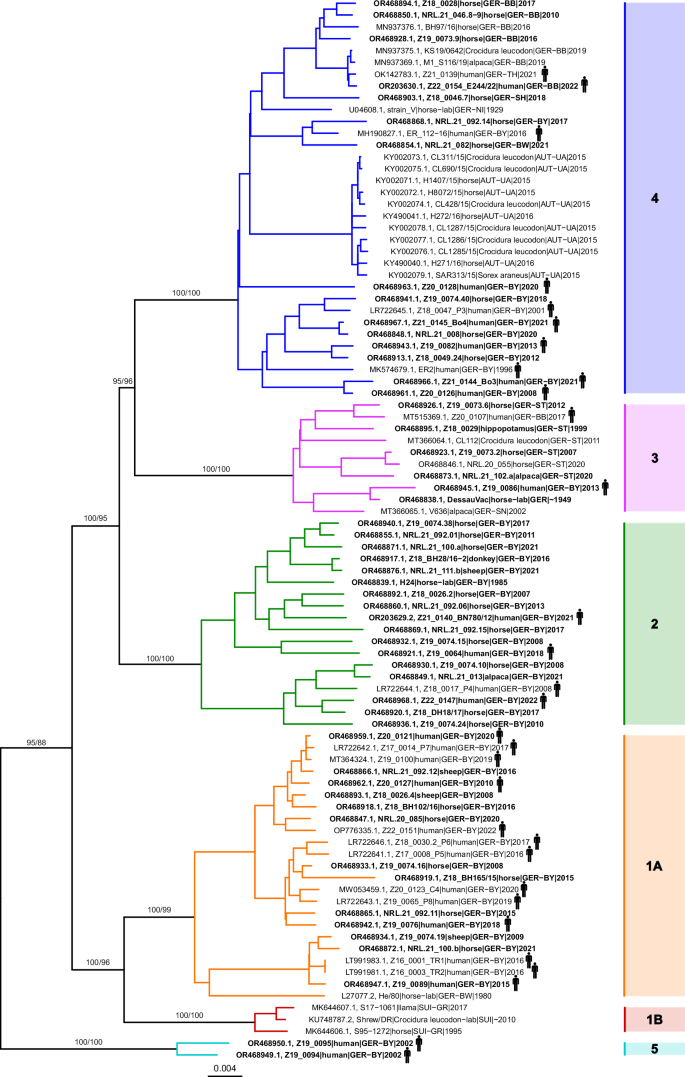
A maximum likelihood tree (model SYM + G4) was calculated for all 90 complete coding BoDV-1 sequences (genome positions 54 to 8822) of human and animal origin. Sequence BoDV-2 No/98 (AJ311524; not shown) was used to root the tree. Sequences generated during this study are depicted in bold. Statistical support is shown for major branches, using the format “SH-aLRT/ultrafast bootstrap”. Clusters 2 to 5 and subclusters 1A and 1B are indicated by coloured branches and bars. Germany (GER): BB Brandenburg, BY Bavaria, BW Baden‐Wuerttemberg, NI Lower Saxony, SH Schleswig-Holstein, SN Saxony, ST Saxony‐Anhalt; Switzerland (SUI): GR Grisons; Austria (AUT): UA Upper Austria. Phylogenetic tree in Newick format is provided in Supplementary Data 2 .
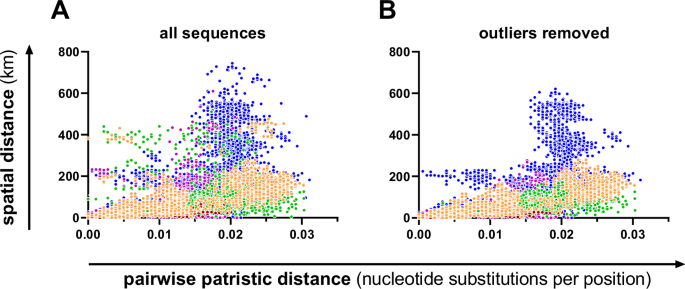
Pairwise patristic distances were inferred from the maximum likelihood (ML) tree of N-X/P nucleotide sequences (Fig. 4A and Supplementary Fig. 5 ) and plotted against pairwise spatial distances. A Results of all 238 N-X/P nucleotide sequences with available locations. B Results of 214 N-X/P sequences after removal of the 24 sequences that were categorised as phylogeographic outliers using the following criteria: existence of no other BoDV-1 N-X/P sequence with ≥98.6% nucleotide sequence identity within a distance of ≤37.9 km. Colours represent the phylogenetic clusters 2 to 5 and subclusters 1A and 1B (see Fig. 2 ). Only pairwise comparisons within clusters or subclusters are depicted in this figure. A detailed presentation of the analysis is shown in Supplementary Fig. 8 .
To provide a more objectifiable basis for BoDV-1 cluster designation, we performed further analyses based on pairwise nt sequence identities of complete coding genomes (Supplementary Fig. 6 ). Sequences belonging to the same cluster shared at least 96.0% pairwise nt sequence identity, whereas sequences of different clusters were only up to 95.7% identical. Cluster 1 sequences shared 96.0 to 96.4% nt sequence identity between subclusters 1A and 1B and at least 96.9% within each of the two subclusters, thus providing objectifiable demarcation criteria for cluster and subcluster assignment of complete coding genome sequences (Supplementary Fig. 6 ). In agreement with these values, the two sequences of the novel cluster 5 possessed 99.0% nt sequence identity with each other, but only 93.8 to 95.1% nt sequence identity with any other BoDV-1 sequence, supporting their affiliation to a separate cluster.
Temporal and spatial relationships and host-association within BoDV-1 clusters
In agreement with the well-known genetic stability of orthobornaviruses 38 , 40 , 41 , we observed pairs of nearly identical BoDV-1 sequences that were detected many years or even several decades apart from each other (Fig. 2 and Supplementary Fig. 5 ). This observation was further confirmed by linear regression analysis of the ML tree root-to-tip divergence of BoDV-1 sequences over the past 43 years, which demonstrated the year of sampling to have no significant effect on the genetic divergence of the phylogenetic clusters and subclusters ( R ² = 0.0057 to 0.0570; P > 0.05; Supplementary Fig. 7 ).
Furthermore, the phylogenetic analysis did not reveal host-specific clades. With the exception of the novel cluster 5, all clusters and subclusters were composed of closely related sequences derived from shrews and domestic mammals. Human sequences were identified in all clusters and subclusters except for subcluster 1B (Fig. 2 and Supplementary Fig. 5 ).
In contrast, the isolation by distance (IBD) analysis of 238 BoDV-1 N-X/P gene sequences with available location suggested a significant positive correlation between geographic and genetic distance (Mantel test p < 0.0001; Supplementary Fig. 8A ). This positive correlation was also found for the individual BoDV-1 clusters ( p ≤ 0.0028; Supplementary Fig. 8A ; summarised in Fig. 3A ).
Detailed spatial analysis of BoDV-1 phylogenetic clusters and subclusters
For a detailed analysis of the spatial distribution of BoDV-1, subtrees of individual clusters or subclusters were extracted from the BoDV-1 N-X/P ML tree (Fig. 4A and Supplementary Fig. 5 ) and all cases with available location were mapped geographically (Fig. 4 B– G ). To aid visualisation of relationships between phylogenetic analysis and geographic mapping, we indicated monophyletic subclades showing associations to particular geographic regions (Fig. 4 and Supplementary Fig. 5 ).
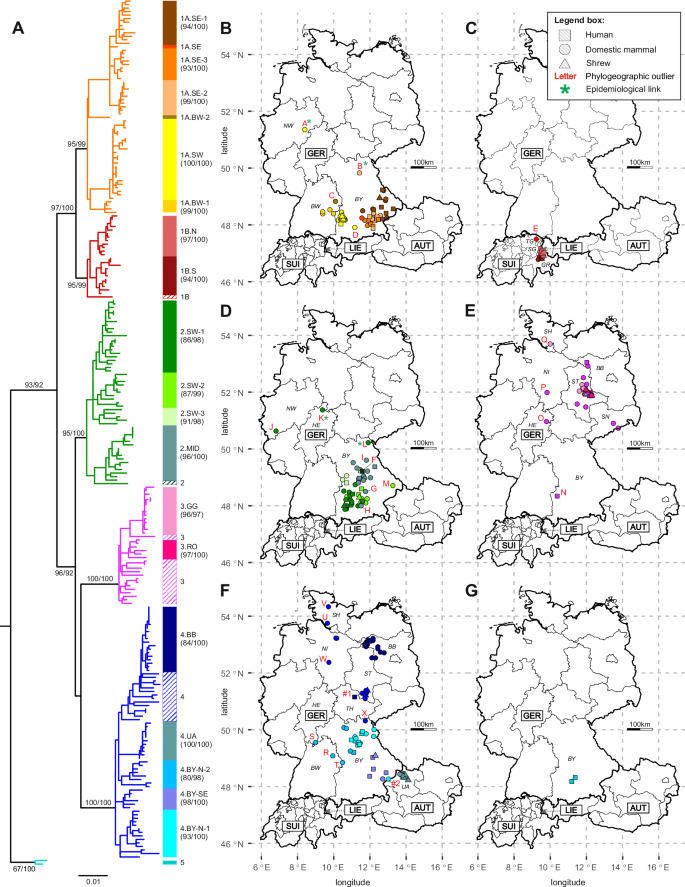
A A maximum likelihood (model GTR + F + I + G4) tree was calculated for 246 partial BoDV-1 sequences (1,824 nucleotides, nt) of human and animal origin that are covering the complete N, X and P genes (genome positions 54 to 1877). Sequence BoDV-2 No/98 (AJ311524; not displayed) was used to root the tree. Statistical support is shown for main branches (including clusters, subclusters, and subclades), using the format “SH-aLRT/ultrafast bootstrap”. Clusters 2 to 5 and subclusters 1A and 1B are indicated by coloured branches. Subclades are indicated by coloured bars and corresponding text labels, with statistical support of subclades shown in brackets. B – G Spatial distribution of subclusters 1 A ( B ) and 1B ( C ) and clusters 2 ( D ), 3 ( E ), 4 ( F ) and 5 ( G ). Colours of the symbols represent the phylogenetic subclades indicated in panel A). Human sequences are generally mapped no more precise than to the centre of the district of the patient´s residence. Red labels represent phylogeographic outliers (capital letters; Supplementary Table 3 ) or additional cases with potentially aberrant infection site (#1 and #2; Supplementary Table 4 ). Green asterisks indicate known epidemiologic links into the dispersal area of the respective subclade. Germany (GER): BB Brandenburg, BY Bavaria, BW Baden‐Wuerttemberg, HE Hesse, NI Lower Saxony, NW North Rhine-Westphalia, SH Schleswig-Holstein, SN Saxony, ST Saxony‐Anhalt, TH Thuringia; Switzerland (SUI): GR Grisons, SG St. Gall, TG Thurgau; Austria (AUT): UA Upper Austria; Liechtenstein (LIE). Subclade designations: GG Güterglück, MID Middle, N North, S South, RO Rosslau, SE Southeast, SW Southwest.
The spatial distribution of subcluster 1A covers parts of southeastern BY as well as an area from southwestern BY to eastern Baden-Wuerttemberg (BW; Fig. 4B and Supplementary Fig. 5A ). This bipartite distribution is also reflected by the phylogenetic pattern. The sequences from southeastern BY are located on a common branch that is subdivided into three phylogenetically and spatially distinguishable subclades (1A.SE-1, -2 and -3) covering distinct regions in southeastern BY with only minor overlaps among each other. The sequences from southwestern BY form a separate subclade (1A.SW). A further subclade is constituted by four sequences from BW (1A.BW-1), while the phylogenetic position of the fifth sequence from BW (1A.BW-2) is separated from all other sequences (Fig. 4A and Supplementary Fig. 5A ).
Subcluster 1B is restricted to a rather confined region in the Alpine Rhine valley in the Swiss cantons Grisons (GR) and St. Gall (SG), with a few cases detected across the border into Liechtenstein (Fig. 4C ). This subcluster can be subdivided into a southern and a northern subclade (1B.S and 1B.N, respectively; Fig. 4C and Supplementary Fig. 5B ). In this study, we were able to generate only one new sequence of subcluster 1B, which originated from the canton Thurgau (TG), a neighbouring canton of SG that has not been described as endemic for BoDV-1 so far. In agreement with its separate location, the phylogenetic position of this sequence is basal to subclades 1B.S and 1B.N (Fig. 4C and Supplementary Fig. 5B ).
Cluster 2 occupies major parts of central BY, mainly covering the region between the two parts of the dispersal area of subcluster 1A (Figs. 1 B and 4D ; and Supplementary Fig. 5C ). The cluster can be subdivided into three monophyletic subclades in the southwestern part of this area (2.SW-1, -2 and -3) and one that is mainly found more to the northeast in central BY (2.MID) with only a few overlapping cases (Fig. 4D and Supplementary Fig. 5C ).
Cluster 3 is situated mainly in eastern ST and in the South of SN, with single outliers in other federal states (Fig. 4E and Supplementary Fig. 5D ). We assigned two phylogenetically supported subclades with, however, strongly overlapping dispersal areas (3.GG and 3.RO, named after the locations of the majority of their mainly shrew-derived sequences, Güterglück and Rosslau, respectively). The remaining sequences could not be assigned to prominent phylogenetic subclades (Fig. 3E and Supplementary Fig. 5D ).
Cluster 4 is the most widely distributed cluster with a scattered geographic range covering parts of Upper Austria (UA), northern and southeastern BY, ST and BB. Individual additional cases were located in Schleswig-Holstein (SH), NI, Thuringia (TH), Hesse (HE) and BW (Fig. 4F and Supplementary Fig. 5E ). In agreement with this geographic pattern, sequences of cluster 4 form several subclades with apparent association with distinct regions in UA (4.UA), southeastern and northern BY (4.BY-SE, 4.BY-N-1 and -2) and BB (4.BB). No clear subclades could be defined for a large group of genetically rather diverse sequences from northern parts of Germany (Fig. 4F and Supplementary Fig. 5E ).
Both sequences of the newly identified cluster 5 originated from two neighbouring districts in a region in southern BY where usually sequences of cluster 2, subclades 2.SW-1 and -2, were found (Figs. 1 B and 4 D, G and Supplementary Fig. 5F ).
Definition of phylogeographic outliers
While phylogenetic grouping and geographic mapping were in good agreement with each other for the majority of BoDV-1 sequences, singular sequences appeared to be located distant from their phylogenetic relatives (Fig. 4 ). We sought for objective and reproducible criteria to define such sequences as phylogeographic outliers. Pairwise nt comparisons of all N-X/P-gene sequences with available location ( n = 238) showed that all sequences possessed one or more relatives with at least 98.6% nt sequence identity, with only one exception sharing only 98.1% nt sequence identity to its next relative (Supplementary Fig. 9A ; representing sequence OR468948, marked as case F in Fig. 4C , Supplementary Fig. 5B and Supplementary Table 3 ). The minimum distances of each case to all sequences with at least 98.6% nt sequence identity ranged from 0 to 366 km, with the 90th percentile at 37.9 km (Supplementary Fig. 9B ). Based on these observations, all cases without a sequence of at least 98.6% nt sequence identity within a maximum distance of 37.9 km were marked as outliers, corresponding to the approximately 10% sequences with the highest minimal spatial distance to any close relative. These criteria were met by 24 of the 238 N-X/P sequences (10.1%; Fig. 4 , Supplementary Fig. 5 and Supplementary Table 3 ). The same 24 cases were identified as phylogeographic outliers by a comparative approach using patristic distances instead of nt sequence identities (Supplementary Table 10 ). Removal of outlier sequences from the IBD analysis increased the correlation coefficient ( r ), which measures the strength and direction of the correlation between genetic and geographic distance, for all clusters with the exception of cluster 1B. This effect was most prominent for cluster 2 (Supplementary Fig. 8B ; summarised in Fig. 3B ).
Detailed characteristics of defined phylogeographic outliers
For four of these outliers (outliers A, B, K and L), the available records revealed potential epidemiological links to areas where the respective BoDV-1 variants were considered to be endemic (Supplementary Table 3 ). A horse (outlier A; accession OR468845) had developed Borna disease in 2019 in North Rhine-Westphalia (NW), which is not known to be endemic for BoDV-1. The animal had been purchased from an endemic region in southwestern BY approximately two months before its death. In line with this information, the BoDV-1 N-X/P sequence from this horse belonged to subclade 1A.SW and it was identical to a sequence from a sheep from southwestern BY in 2009 (OR468934; Fig. 4B , Supplementary Fig. 5A and Supplementary Table 3 ). An alpaca stallion from northern BY in 2022 (outlier B; OR468886) had been bought eight months before death from a region in southeastern BY, which is consistent with its BoDV-1 sequence belonging to subclade 1A.SE-2 (Fig. 4B , Supplementary Fig. 5A and Supplementary Table 3 ). The animal showed ataxia already on arrival at the new herd, which was assumed to be of orthopaedic cause. An additional alpaca stallion (outlier K; GQ861449) had developed disease in 2008 after having been transported from southwestern BY to the north of HE 2121 . The BoDV-1 sequence from this case belonged to subclade 2.SW-1, which is in congruence with an infection source in south-western BY (Fig. 4C Supplementary Fig. 5B and Supplementary Table 3 ). Outlier L (OR468852), a horse from northern BY in 2021, had been bought from a horse trader in BW two weeks before death. The horse was reported to have been bought by the trader several weeks before from a not further specified location in BY. The BoDV-1 sequence of subclade 2.SW-1 suggests an infection source in south-western BY (Fig. 4C ; Supplementary Fig. 5B ; Supplementary Table 3 ).
In contrast, no epidemiological link to a potentially aberrant location of infection could be identified for the majority of the 24 identified outliers. In some cases, the available information suggested that the animal had never been in a region considered endemic for the respective BoDV-1 variant (outliers J, P, U), but in most cases the available information was insufficient. In some cases, the accuracy of the location data was low, e.g. representing the owner´s address, which may be distant from the actual husbandry (Supplementary Table 3 ). The human case Z19_0093 from western BY in 2016 (outlier F; OR468948) was classified as an outlier, since its sequence possessed only up to 98.1% nt sequence identity to any other BoDV-1 sequence.
Two further RT-qPCR-confirmed BoDV-1 infections in horses were detected far from any known BoDV-1-endemic region. These cases had occurred in western Switzerland (canton Geneva [GE]) in 1988 and in eastern BB in 2006 (Fig. 1B ). However, sequencing attempts had failed due to poor RNA quality. Epidemiological data were not available for these cases.
Phylogeographic relationship of human- and animal-derived BoDV-1 sequences
Of the 43 non-transplant-derived human BoDV-1 infections confirmed prior to preparation of this manuscript, 40 were diagnosed in BY, two in BB and one in TH (Figs. 1 B and 4 ). The vast majority of their BoDV-1 sequences matched the phylogenetic subclades found in the respective patient´s region of residence (Fig. 4 ). Typically, sequences originating from animals or humans in close geographic proximity were representing the genetically closest relatives of human-derived sequences. For 90% of human-derived N-X/P sequences with available location ( n = 39), the distance to their phylogenetically closest relatives was less than 40 km, with a median distance of 15.6 km (Fig. 5 ). In several cases, identical animal-derived sequences or sequences sharing ≥99.9% nt sequence identity were found within <10 km distance to the residency of the respective human patient. For instance, the human BoDV-1 sequence Z21_0129 (OR468964; subclade 1A.SW) shared 99.9% nt sequence identity with the horse-derived sequence OR468847 (NRL.20_085) that originated from the same district in south-eastern BY (Supplementary Fig. 5A ). Similarly, human sequence Z19_0107 (MT515369; cluster 3) 12 was 99.9% identical to that of an alpaca (NRL.22_102; OR468885) from the same district in BB (Supplementary Fig. 5C ). The BoDV-1 N-X/P sequences from patients Z19_0100 (MT364324) 11 , 15 and Z20_0121 (OR468959) were completely identical among each other (99.9% at complete genome level) and 99.9% identical to the sequence of sheep NRL.21_092.12 (OR468866) from the same district in southeastern BY (Supplementary Fig. 5A ).
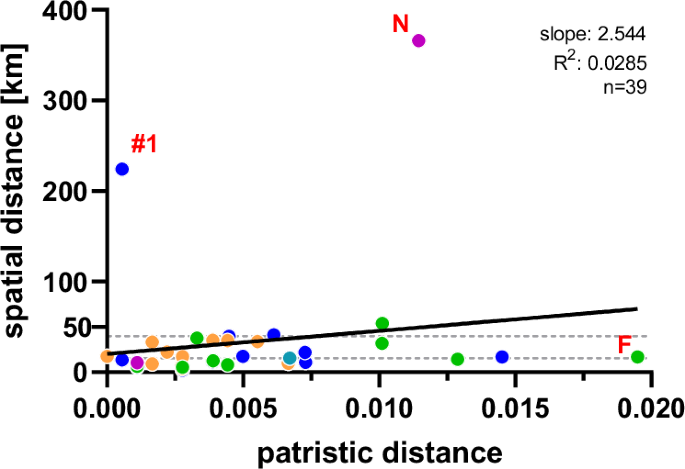
The minimal distance to the most closely related BoDV-1 nucleotide (nt) sequence was identified for all human BoDV-1 sequences with available geographic information ( n = 39) based on patristic distances calculated from the maximum likelihood (ML) tree of 246 N-X/P nt sequences (Supplementary Fig. 5 ). Sequences without available location as well as non-human sequences classified as phylogeographic outliers (Supplementary Table 3 ) were excluded from the analysis. For each human sequence, the minimal spatial distance was calculated to all sequences with a patristic distance of up to 1.2-fold the patristic distance to the phylogenetically closest relative. Colours of the dots represent phylogenetic clusters and subclusters as defined in Fig. 2 . Red capital letters indicate human cases identified as phylogeographic outliers (Supplementary Table 3 ). Sequence Z21_0139 (labelled as #1) is suspected to have an aberrant infection site due to its close genetic relation to animal sequences in more than 200 km distance (Supplementary Table 4 ). Broken horizontal lines represent the 90th percentile (39.8 km) and the median (15.6 km) of the presented dataset. The black line represents the linear regression of genetic and geographic distance. Slope and goodness of fit ( R 2 ) of the regression line are provided.
Besides outlier F mentioned above, only one additional human sequence was classified as an outlier. The BoDV-1 sequence of patient Z19_0086 (OR468945; outlier N; Supplementary Table 3 ) from southwestern BY belonged to cluster 3 and was, thus, genetically divergent from all other sequences originating from this area. Its sequence possessed 99.9% nt sequence identity to the nt sequence of the vaccine strain ‘DessauVac’ (Supplementary Fig. 5C ). The precise origin of this historic vaccine strain is unknown, but it is believed to have been isolated from a horse from ST or western SN around 1949 30 , 38 . The closest relative of sequence Z19_0086 with available location originated from an alpaca in SN (MT366065), about 370 km from the patient´s residency (Supplementary Table 3 and Fig. 5 ). Information on possible epidemiological links to ST or SN was not available for patient Z19_0086. Likewise, no information was available on potential contacts to the vaccine strain DessauVac, which had been used until 1992 in eastern parts of Germany (former German Democratic Republic), but never in BY. The sequence of case Z21_0139 from TH, 2021 (OK142783; marked as case #1) 13 , showed a high spatial distance to its most closely related BoDV-1 sequences. Its sequence belonged to subclade 4.BB and possessed up to 99.9% nt sequence identity to animal sequences from BB that are located more than 200 km from the patient´s home (Fig. 5 ), suggesting an aberrant infection site. However, since less closely related additional cluster 4 sequences (99.3% nt sequence identity) were found at a distance of roughly 25 km, the case did not match our criteria for a phylogeographic outlier (Supplementary Table 4 ).
Interestingly, both patients infected with BoDV-1 of cluster 5 had died after developing disease in 2002 and both lived in neighbouring districts in the vicinity of Munich (BY; Fig. 4G and Supplementary Fig. 5F ). Cluster 5 has not been detected in shrews or domestic mammals so far.
Determination of BoDV-1 endemic areas
To visualise the endemic areas of each BoDV-1 cluster or subcluster, we employed Kernel Density Estimation (KDE; Fig. 1C ). For this approach, all phylogeographic outliers defined above (Supplementary Table 3 ) were excluded from the dataset. The resulting KDE illustrated that the distribution of each BoDV-1 cluster or subcluster covered largely separate areas with little overlap (Fig. 1C ). In a second approach, we repeated the KDE using the combined dataset of all BoDV-1 clusters. This combined KDE revealed a tripartite endemic area (Fig. 1D ). The northernmost part ranges from northwestern BB to southern ST, possibly also including northern TH. The largest part covers most of BY and extends into BW and UA. The southernmost endemic area is found in the Alpine Rhine valley (Fig. 1D ). In addition, individual sequences not classified as outliers are found in NI south of Hamburg and in the Ore Mountains in SN, close to the Czech border (Fig. 1D ).
The aim of this study was to assemble the most comprehensive data on the molecular epidemiology and phylogeography of BoDV-1 allowing the identification of risk areas for the occurrence of spill-over transmission to domestic mammals and humans. Detection of BoDV-1 in shrews as the only known reservoir hosts would provide the most accurate information on its endemic presence. However, representative samples from shrews are not easily accessible. To date, these data are highly fragmented and biased due to active sampling at only a few locations 4 , 25 , 31 , 32 , 33 , 34 , 42 . We therefore adopted a passive surveillance approach, utilising Borna disease in domestic mammals as an indicator of endemic BoDV-1 infection in local shrew populations. This approach may provide a potentially less biased fundament for phylogeographic analyses, although some variability of the dissemination of susceptible domestic animal populations and of veterinary vigilance within and outside of known endemic areas cannot be excluded. Reliable information on the location of infected individuals, not only during onset of disease but even more importantly, at the potential time point of infection, is crucial for this study. However, due to the long and possibly highly variable incubation period, such information is not always available, particularly for cases from earlier decades. Despite our extensive efforts to fill these data gaps, there are still varying degrees of uncertainty that must be considered when interpreting the results of this study.
Our analyses supported the previously introduced phylogenetic clusters and subclusters 38 , 39 , 40 , for which we established objectifiable demarcation criteria, based on pairwise nt sequence identities of complete coding BoDV-1 genomes. The identification of a novel BoDV-1 cluster 5, represented by two human sequences from BY, indicates that the genetic variability of BoDV-1 may be higher than currently appreciated and that additional variants may exist within or outside the known endemic areas.
We found the BoDV-1 clusters and subclusters to be genetically remarkably stable over time and to be associated with spatial distribution rather than time of detection or host species, as demonstrated previously 7 , 25 , 38 , 40 . In our study, we were able to markedly increase the extent, reliability and resolution of the phylogeographic data, showing that BoDV-1 sequences of particular subclades are occupying circumscribed areas within the endemic regions. This geographically bound epidemiology further emphasises that BoDV-1 is tied to a reservoir with a strictly territorial behaviour and only very little mobility 38 , 40 . Bicoloured white-toothed shrews are known to occupy territories of 40 to 120 metres in diameter. They typically do not move voluntarily more than 800 m to 1 km from their territory, with a documented maximum of 2.5 km, thus allowing for only limited virus spread 36 . This spatial limitation also underlines the fact that spill-over hosts, such as domestic mammals and humans, which tend to be more mobile, serve as dead-end hosts for the virus. Otherwise, their contribution to virus spread would have resulted in a much wider distribution of confirmed cases with considerable spatial overlap of genetic variants. Thus, our results clearly support previous work 35 , 39 , 40 , 46 and refute former hypotheses of a possible worldwide spread of BoDV-1 among humans and non-reservoir animals 47 , 48 .
To indicate the regions in which BoDV-1 variants of particular clusters or subclusters are endemic, we defined criteria for those sequences that indicate the endemic presence of the virus with reasonable reliability. Due to the limitations described above, a single BoDV-1 detection in a domestic mammal or human spatially separated from its closest viral relative cannot be considered as an indicator of endemicity. It rather needs to be supported by additional genetically related sequences from the same region. We have tentatively defined the criteria for considering sequences as indicators of endemicity to be at least two sequences with at least 98.6% nt sequence identity within a 37.9 km diameter. Applying these criteria, the ~10% of the sequences with the highest spatial distance from their closest relatives are regarded as phylogeographic outliers and excluded from further analysis. We used N-X/P nt sequence identities rather than patristic distances to make the analysis more easily applicable to further cases diagnosed in the future. A comparative analysis confirmed that using patristic distances led to consistent results, demonstrating the robustness of our approach. The criteria defined in this study may be subject to further refinement as more extensive data will become available. Visualisation of the distribution of the BoDV-1 sequences after removal of the phylogeographic outliers confirmed and refined the previously assumed pattern of endemic areas 35 , but also extended it in certain regions, such as northern BY and eastern BW.
Geographic outliers do occasionally occur distant from the indicated distribution areas of their phylogenetic clades or even completely outside the defined endemic area of BoDV-1. Due to the limitations of the available metadata, it cannot be excluded that at least some of these cases may be the result of inaccurately reported locations. Others may be the result of travel within the incubation period, as previously described 21 , 22 . During the course of this study, at least three additional cases were identified in which animals were likely to have been moved to new locations during the incubation period, with BoDV-1 sequences suggesting sources of infection at the respective sites of origin. In one of these cases, the alpaca had been moved no less than eight months prior to death. However, the incubation period may have been shorter, as the animal was exhibiting a potentially BoDV-1-associated ataxia already at the time of transfer.
Such clear epidemiological links could not be established for the majority of phylogeographic outliers identified in this study. While no further information was available for most of these cases, some animals were reported to have never travelled to known endemic areas, or even to have remained in their holding of birth throughout their lives, thus suggesting the existence of so far unidentified infection sources in these regions. Whether or not BoDV-1 can be transmitted over long distances by passive vectors, such as import of contaminated feed, remains elusive. So far, no such cases have been documented and data on the environmental tenacity of the virus are sparse. Thus, at least some of the latter cases may actually indicate the endemic presence of the virus in local shrew populations, requiring confirmation by detection of additional genetically related viruses from their region. For instance, the phylogenetic analyses of the previously published human case Z19_0107 from BB had grouped the virus to cluster 3 that had not been detected in this region before 12 , suggesting a possibly aberrant infection source. The recent detection of a highly similar BoDV-1 sequence from an alpaca from the same district in BB now rather suggests endemicity of this BoDV-1 variant in this region and a peridomestic infection of both individuals, which is also in accordance with the patient´s epidemiological history during the last years before onset of the disease 12 .
Our study increases the number of published laboratory-confirmed human BoDV-1-infections to 46 and provides a first comprehensive summary of metadata for all published cases 6 , 7 , 8 , 9 , 10 , 11 , 12 , 13 , 14 , 15 , 16 , 17 , 18 . The cases covered all age groups and encephalitis was diagnosed in 45 of the 46 patients, 44 of whom had died as a result of the disease, resulting in a known case-fatality rate of 97.8%. As of now, the representativeness of these numbers for all incident cases is difficult to assess. Many cases in this study were diagnosed only by retrospective analysis of encephalitis cases. Given the only recently discovered human threat of the virus, it is likely that particularly in the past a considerable proportion of fatal BoDV-1 infections remained undetected 7 . This proportion may have been comparably higher for possible non-fatal infections due to a lower alertness of physicians to such cases and to the limited availability of diagnostic material for direct intra vitam detection of BoDV-1, which is hampered by the remarkable restriction of BoDV-1 to the central nervous system in erroneous spill-over hosts 1 , 7 , 9 , 20 , 25 , 35 , 49 . However, the estimated prevalence of bornavirus-reactive antibodies did not exceed 0.24% in recent serological surveys of healthy individuals or neuropsychiatric patients in known endemic areas 43 , 45 , 50 . Analysis of brain tissue from encephalitis cases of unknown origin failed to detect BoDV-1 in biopsy samples from 15 non-fatal cases, while BoDV-1 was detected in seven out of nine (78%) fatal cases from the same cohort 7 . Likewise, nation-wide screenings identified bornavirus-reactive antibodies in serological samples and/or BoDV-1 RNA in CSF only in patients suffering from severe or fatal encephalitis 11 , 43 . These findings support the assumption that non-fatal or even asymptomatic human BoDV-1 infections are indeed at least very rare.
The detailed phylogeographic network of animal-derived BoDV-1 sequences assembled in this study also allowed for a first phylogenetic assessment of potential geographic sources of human BoDV-1 infections. With the exception of three organ transplant-derived infections 8 , all known infections are assumed to have resulted from individual zoonotic spill-over events from the virus reservoir 6 , 7 , 9 , 10 , 11 , 12 , even though concrete transmission events could not be identified in a retrospective epidemiological analysis of 20 cases 17 . Almost all human BoDV-1 sequences clustered in accordance with the location of the patient´s residence. The median estimated distance to the closest phylogenetic relatives was 15.6 km, indicating that infection of most patients occurred close to home, which is in accordance with epidemiological work identifying rural residence on the fringe of the settlement as the major risk factor for BoDV-1 infection 17 . The actual distance to the source of infection is likely to be even lower in many cases, since inaccuracies of location data, as well as unavailability of further sequences representing genetically closer relatives are likely to lead to overestimation rather than underestimation.
The exact source and route of transmission remain elusive for almost all BoDV-1 infections in humans and domestic mammals. Due to its almost exclusively neurotropic nature in non-reservoir hosts 7 , 9 , 20 , 25 , 49 , transmission chains between spill-over hosts can be virtually excluded, with the exception of a so far singular iatrogenic transmission event by solid organ transplantation insert ref. 8 . This leaves infected shrews as the most likely infection source. So far, it remains unknown whether exposure to their excretions is sufficient for transmission or whether direct contact to an infected shrew or its carcass is required. In a previous study, household members of deceased patients could not recollect potential events of BoDV-1 exposure, indicating that these may be rather unremarkable 17 . Experimentally, BoDV-1 infection in animal models may be established via mucosal surfaces, mainly intranasally, or by subcutaneous injection, followed by axonal spread to the brain via nerves and olfactory bulb or spinal cord 23 , 51 , 52 , 53 , 54 , 54 . Overall, spill-over transmission of BoDV-1 to humans appears to be rather inefficient, as only isolated cases have been reported so far. Associated infections of potentially equally exposed individuals, such as family members or co-workers on agricultural farms, have not been detected, yet. While the disease usually affects only a single animal or a small number of individuals in herds of horses and sheep, higher incidences of BoDV-1 infections have been observed during outbreaks in New World camelid holdings, leading to mortality rates of up to 40% within a few months in affected herds 25 , 38 , 40 , 55 . Similarly, four out of eight alpacas of a herd from central BY analysed in our study succumbed to confirmed BoDV-1 infection within one year (cases 21_013, 21_149.a, 22_015.a and 22_015.b; Supplementary Fig. 5C ).
Previous studies have hypothesised a higher risk of BoDV-1 transmission to domestic mammals during winter, leading to disease outbreaks and eventually death during spring and early summer. Shrews entering animal stables in search of feed have been suggested to be responsible for this pattern 33 , 40 . In congruence with these assumptions, our assembled data suggested a peak of laboratory-confirmed fatal Borna disease in domestic mammals during May and June. In contrast, no such seasonality was observed for the time of death or first known hospitalisation of the comparably limited number of human BoDV-1 cases, similar to findings in a previous study of 20 human cases 17 . However, any conclusions regarding the time of infection are complicated by the unknown incubation period and the variable disease progression. Furthermore, the time from infection to death of human patients is affected by attempted treatments and life-sustaining measures, which further increase the variability. A larger dataset of human BoDV-1 infections may be required to demonstrate whether their temporal distribution actually differs from the occurrence of BoDV-1 infection in domestic mammals.
In summary, we performed a highly comprehensive phylogeographic analysis of the occurrence of the zoonotic pathogen BoDV-1 in Central Europe. The improved resolution of the phylogeographic data will provide a basis for assessing potential locations and sources of BoDV-1 infection in animals and humans. The visualisation of potential risk areas will allow for the implementation of prophylactic measures – mainly reducing the risk of exposure to the reservoir – in the affected regions. In such areas, BoDV-1 may be responsible for a considerable proportion of cases of severe human encephalitis that may have remained unresolved so far 7 . Increased awareness among veterinarians and physicians, together with the categorisation of BoDV-1 as a notifiable pathogen of humans and animals since 2020 11 , may lead to more extensive data collection, allowing for further refinement of phylogeographic analyses in the future.
Acquisition of sample material
Veterinary pathologists and federal and private veterinary diagnostic laboratories in Germany, Switzerland and Austria were informed about the study through presentations at scientific meetings, publications in national specialist journals, via mailing lists of expert societies and by direct contact. In total, 20 institutions provided fresh-frozen or FFPE brain tissue or CSF from 231 archived or recent suspected BoDV-1 infections in domestic mammals (including few zoo animals; Table 1 ; Supplementary Table 1 ). Some, but not all, of these infections had already been diagnosed by the submitting diagnostic laboratories. In addition, brain samples from 29 archived or recent human BoDV-1 encephalitis cases were obtained from diagnostic centres and pathologists in Germany. These cases included unpublished cases as well as previously published cases without available BoDV-1 sequence 7 , 9 , 14 , 16 , 17 , 18 (Table 1 ). In addition, samples from seven BoDV-1-positive bicoloured white-toothed shrews were obtained from an ongoing large-scale small mammal screening study (Haring et al., manuscript in preparation; Table 1 ). Furthermore, an original vaccine vial containing the historic BoDV-1 live vaccine strain ‘Dessau’, herein referred to as ‘DessauVac’ (batch 193 02 90; kindly provided by Sven Springer, IDT Biologika, now Ceva Santé Animale, Dessau-Rosslau, Germany), and the cell culture isolate H24 39 were included for sequence analysis. In addition, the horse-derived cell culture isolates H640 and H3053 38 were kindly provided for resequencing by Sybille Herzog (Gießen, Germany).
Acquisition of sample metadata
In order to facilitate spatio-temporal analyses, detailed metadata were requested from the submitters, including geographic location (postal code) and date of sampling, which was usually the date of death. Age, sex and date of hospital admission were recorded additionally for human cases. For animal cases, the accuracy of the geographic location was non-hierarchically categorised as follows: (1) the location of the animal husbandry is known, (2) the address of the owner is known, but the location of the husbandry is unknown and may be different, (3) only the submitting veterinary practice/clinic is known, (4) only the administrative district of origin is known, and (5) no information on the accuracy of the location is available.
In addition to the samples analysed in this study, previously published BoDV-1 sequences available via GenBank were included in the phylogeographic analysis (Table 1 ). The metadata described above (location, accuracy of location, host species, date of death or sampling) were assembled also for these cases, based on the available literature 4 , 6 , 7 , 9 , 10 , 11 , 12 , 13 , 15 , 21 , 25 , 33 , 38 , 39 , 40 , 55 . Missing data were completed by contacting the authors and/or the initial submitters, if possible.
The species of the seven analysed bicoloured white-toothed shrews had been confirmed by sequence analysis of the cytochrome B gene 56 .
Extraction of total RNA
Fresh-frozen samples were mechanically disrupted in 1 ml TRIzol reagent (Life Technologies, Darmstadt, Germany) by using the TissueLyser II (Qiagen, Hilden, Germany), according to the manufacturers’ instructions. After the addition of 200 µl chloroform and a centrifugation step (14,000× g , 10 min, 4 °C), the aqueous phase was collected and added to 250 µl isopropanol. Total RNA was extracted using the silica bead-based NucleoMagVet kit (Macherey & Nagel, Düren, Germany) with the KingFisher™ Flex Purification System (Thermo Fisher Scientific, Waltham, MA, USA) according to the manufacturers’ instructions.
Additional RNA extraction from fresh-frozen samples selected for HTS was performed according to Wylezich et al. 57 . Briefly, the tissue was rapidly frozen in liquid nitrogen and subsequently pulverised using the Covaris cryoPREP (Covaris, Brighton, UK). The resulting powdered tissue was then dissolved in pre-warmed 1 ml lysis buffer AL (Qiagen). RNA was extracted using the RNAdvance tissue kit (Beckman Coulter, Germany), including a DNase I (Qiagen) digestion step, in combination with a KingFisher Flex purification system (Thermo Fisher Scientific, Germany), according to the manufacturers’ instructions. Total RNA was eluted in 100 µl nuclease-free water.
Total RNA from FFPE brain tissues was extracted as described previously 58 . Briefly, two FFPE sections of <10 µm thickness underwent deparaffinisation and proteinase K digestion employing the Covaris truXTRAC FFPE total NA kit before RNA extraction, according to the manufacturer’s instructions, resulting in 100 µl supernatant. To prevent the transfer of paraffin residues, formalin de-crosslinking was carried out using 85 µl of the supernatant in a clean 1.5 ml reaction tube (80 °C, 30 min, thermomixer). Subsequently, 175 µl of B1 Buffer from the Covaris kit and 250 µl of 65% isopropanol were added, mixed, and briefly centrifuged. Subsequently, RNA extraction was performed using the Agencourt RNAdvance Tissue Kit, as described above.
Detection of BoDV-1 RNA by RT-qPCR
BoDV-1 RNA was detected using two BoDV-1-specific RT-qPCR assays (Mix-1 & Mix-6) detecting P and M gene RNA, respectively (Supplementary Table 2 ), as described in detail elsewhere 8 . Exogenously supplemented, in vitro-transcribed RNA of the enhanced green fluorescence protein (eGFP) gene or host-derived beta-actin RNA were amplified as extraction control or RNA quality control, respectively, following previously described protocols 59 , 60 (Supplementary Table 2 ).
Selection of samples for BoDV-1 sequencing
HTS was performed for 114 selected BoDV-1 cases, including the cell culture isolates H24 and DessauVac (Table 1 ). Selection criteria for animal samples included spatial proximity to known human BoDV-1 cases or the occurrence in regions from which no or only few BoDV-1 sequences were available. If more than one case was available from a particular location, samples with higher predicted ratios of BoDV-1 RNA (indicated by lower RT-qPCR Cq values) versus total RNA concentration were selected 61 . Enrichment of BoDV-1 specific library DNA fragments by hybridisation-based capture technology was performed for 16 selected samples for which insufficient BoDV-1 sequence information had been obtained by standard HTS (Table 1 ).
For additional 43 BoDV-1-positive fresh-frozen brain samples from domestic mammals, humans or shrews, partial BoDV-1 genome sequences were generated by Sanger sequencing (Table 1 ).
High-throughput sequencing
Libraries with an average DNA fragment size of 500 bp were prepared from fresh-frozen BoDV-1-positive brain samples with sufficient RNA quality following the procedure described by Wylezich et al. 57 with modifications by Szillat et al. 62 . Modified library preparation protocols were used for FFPE-samples as well as for fresh-frozen samples with lower RNA quality, resulting in a mean DNA fragment size of 200 bp 7 . Library quantification was carried out with the QIAseq Library Quant Assay Kit (Qiagen) and fragment size of each library was analysed using Agilent High Sensitivity DNA kit implemented in 2100 BioAnalyzer Instrument (Agilent). Libraries of 500 bpfragment size were sequenced400 bp runs using an Ion Torrent S5 XL instrument (Thermo Fisher Scientific). Libraries of 500 bp DNA fragment size were sequenced in 400 bp runs using Ion 530 chips, while libraries of 200 bp DNA fragment size were sequenced in 200 bp runs using Ion 540 or Ion 550 chips on an Ion S5 XL instrument.
BoDV-1 target enrichment by hybridisation-based capture technology
For enrichment of BoDV-1 specific library DNA fragments, an RNA bait set was designed for sequences representing all known members of the family Bornaviridae 5 , resulting in 17,858 non-redundant specific RNA baits and providing a three-fold genome coverage with a length of 80 nt per probe (myBaits® kit with 1–20 K unique baits; Arbor Bioscience, Ann Arbor, MI, USA). The procedure was performed according to the manufacturer’s instructions with minor modifications. Briefly, 7 µl of each DNA library were combined with the blocking reagent mix of the kit. After denaturation, 20 µl of a pre-warmed hybridisation mix, including the baits, was added. One volume of mineral oil was used to seal the reaction mix before incubation for 24 h at 65 °C and shaking at 550 rpm in a thermomixer. The aqueous phase was then transferred to a low-binding tube and purified using the binding beads from the myBaits® kit. The enriched target library DNA was finally eluted in 35 μl of 10 mM Tris-HCl, 0.05% Tween-20 solution (pH 8.0-8.5) and amplified in duplicates (16 µl DNA each) using the GeneRead DNA Library L amplification Kit (Qiagen) with 10 cycles (denaturation: 2 min at 98 °C; amplification for 10 cycles: 20 s at 98 °C, 30 s 60 °C, and 30 s at 72 °C; final elongation: 1 min at 72 °C). Subsequently, both duplicates were pooled and purified twice by adding 0.65 or 1.2 volumes of Agencourt AMPure XP Beads (Beckman Coulter) for 500 bp or 200 bp libraries, respectively. Enriched libraries were eluted in 30 µl buffer EB (Qiagen).
Bioinformatic analysis and quality control of HTS datasets
HTS datasets originating from sequencing with or without BoDV-1 target enrichment were adaptor- and quality-trimmed using the default settings of the 454 software suite (v3.0; Roche) before BoDV-1 reads were identified and extracted by mapping to the BoDV-1 reference sequence NC_001607. Duplicate BoDV-1 reads caused by library amplification were then removed using the SeqKit tool version 0.15.0 63 . Mapping to the reference sequence was repeated for the remaining BoDV-1 reads. In parallel, the remaining BoDV-1 reads were de novo assembled using the 454 software suite and SPAdes v3.13.1 64 . The accuracy of the resulting contigs was checked by comparing the consensus sequences generated by both approaches with each other and by sequence annotation as described below. Discrepancies at single and polynucleotide level were checked by reviewing the raw data quality and data coverage from mapping and assembly. In addition, the effects of discrepancies on gene annotation and frameshifting were checked. If sequence quality and/or coverage was insufficient, Sanger sequencing of RT-PCR amplicons covering the respective positions was performed for confirmation as described below.
Sanger sequencing
Partial BoDV-1 genome sequences were generated by Sanger sequencing for 43 BoDV-1-positive fresh-frozen brain samples (Table 1 ), following previously described procedures 25 . Briefly, a 2,272- nt long sequence representing BoDV-1 genome positions 20 to 2,291 (spanning the N, X, P and partial M genes) was determined by sequencing two overlapping PCR products using BoDV-1-specific primers (Supplementary Table 2 ). The final consensus sequence was generated by assembly of the overlapping raw sequences after trimming of primer-derived sequence ends and manual quality control.
Sanger sequencing was also used to fill gaps or confirm not sufficiently reliable positions in sequences generated by HTS. For this purpose, BoDV-1-specific primer pairs were selected to generate amplicons of approximately 120 to 180 bp length to cover the respective sequence regions. Primer sequences are available upon request.
Sequence annotation and database submission
BoDV-1 sequences of sufficient length were generated from 136 of 157 selected individuals, including 102 domestic mammals, 25 humans, all seven bicoloured white-toothed shrews as well as the laboratory isolates DessauVac and H24 (Table 1 ). These sequences included 54 complete coding genomes and 82 sequences covering at least the N-X/P genes (Table 1 ).
Open reading frames (ORFs) were identified by ORF Finder (implemented in Geneious Prime® 2021.0.1) and verified by sequence alignment to the reference sequence. All sequences generated in this study are available in the INSDC databases under accession numbers OR203629, OR203630, OR468838 to OR468971.
Two previously published isolates (H640 and H3053) were re-analysed, because it was suspected that they may have been interchanged in the original study 38 . As the re-analysis of the original isolates confirmed this suspicion, the corresponding GenBank entries have now been corrected (accession numbers AY374523.2 and AY374537.2).
Phylogenetic analysis
Phylogenetic analysis of sequences generated in this study was performed together with those publicly available BoDV-1 sequences, which covered at least the N-X/P genes and for which sufficient metadata are available. The used public sequences originated from 55 domestic mammals, 16 human cases, 36 shrews and three laboratory strains isolated from domestic mammals (Table 1 ). Duplicate sequences originating from the same individual as well as sequences previously identified as laboratory contaminants were excluded from the analysis 7 , 39 , 40 .
ML trees were constructed individually for 90 complete coding sequences of BoDV-1 genomes and 246 sequences spanning the N-X/P genes (1824 nt, corresponding to genome positions 54 to 1,877). For these analyses, the BoDV-2 No/98 sequence (AJ311524) was used as an outgroup. After sequence alignment using MUSCLE (version 3.8.425) 65 , the IQ-TREE software (version 2.2.2.6) 66 was used for phylogenetic reconstruction with automatic model selection. In detail, for complete genome and for N-X/P gene alignments the symmetric model with discrete Gamma model “SYM + G4” and general time reversible model with empirically estimated base frequencies, invariable sites plus discrete Gamma model “GTR + F + I + G4”, were selected respectively 67 . Branch support was assessed using SH-aLRT and ultrafast bootstrap tests, with 100,000 replicates for each test 68 , 69 . For detailed visualisation of the phylogenetic tree without the outgroup, and to display the single phylogenetic clusters and subclusters in separate panels, nodes were extracted using the ggtree R package 70 in R Studio 71 with R v4.0.2 72 exclusively. Heatmap analysis of the genetic cluster similarities was performed using the pheatmap R-package 73 .
Temporal and spatial correlation analysis
Root-to-tip distances and pairwise patristic distances (as nt substitutions per position) were inferred from the ML tree of 247 N-X/P sequences (incl. the BoDV-2 sequence). Temporal correlations were tested by linear regression analysis of root-to-tip distances against year of sampling 74 . Sequences originating from laboratory strains were excluded from the analysis to avoid an impact of adaptive mutations acquired during passaging in cell culture or experimental animals.
IBD analysis was performed, testing the correlation of pairwise patristic distances and geographic distances for all BoDV-1 sequences with available location ( n = 238) as well as within the individual BoDV-1 clusters and subclusters. IBD matrix correlations were tested in R using the “mantel” function of the “vegan” package (Spearman’s rho statistic and 9999 permutations).
Phylogeographic analysis and determination of BoDV-1 endemic areas
Geospatial data analysis and modelling was performed in R Studio with the packages rnaturalearth 75 and ggplot2 76 . Investigated cases were mapped according to the geographic location (postal code) available for the metadata assembly. Human cases were visualised no more precise than to the centre of their administrative district (Figs. 1 and 3 ). Cases with available BoDV-1 sequences were linked to the respective phylogenetic trees by use of colour codes representing phylogenetic clusters and subclusters or subclades (Figs. 1 B, C and 3 ).
Non-parametric KDE was used to visualise spatial distribution patterns of mapped BoDV-1 cases. KDE was performed independently for each phylogenetic cluster or subcluster as well as for sequences of all clusters and subclusters combined. BoDV-1 sequences identified as phylogeographic outliers by using the outlier definition described in detail in the results section (presence of no other BoDV-1 N-X/P sequence with ≥98.6% nt identity within a distance of ≤37.9 km) were excluded from the KDE.
The two-dimensional KDE, implemented in ggplot as the “stat_density_2d” function 77 , was used with a polygon as the bounding box of estimated endemic regions. To smoothen the polygon, n = 100 grid points were defined in each direction. A low bandwidth (h) was set empirically for both approaches in order to minimise the extent of the estimated areas beyond the confirmed cases (subcluster 1A: 1; subcluster 1B: 0.5; cluster 2: 0.6; cluster 3: 0.75; cluster 4: 0.65; combination of all clusters: 1.0).
Ethics statement
Ethical approval of the analysis of archived human samples was obtained from the local ethical commission of the Faculty for Medicine, University of Regensburg (ref. no. 18-1248-101), the Technical University Munich (577/19 S), the Ludwig-Maximilians University Munich (23-0267) and the Medical Board of Hamburg (PV5616). Samples of BoDV-1-positive bicoloured white-toothed shrews were obtained from an ongoing large-scale small mammal screening study (Haring et al., manuscript in preparation). Shrew KS20/0026 originated from a project that was commissioned by the Federal Environment Agency as part of the Environmental Research Plan (Research Code 3718 48 4250; animal ethics permit: 42502-2-1548 Uni Leipzig) and was financed with federal funds. All other shrew carcasses included in this study were found dead or preyed by cats. Samples from domestic mammals originated from diagnostic necropsies. No living animals were handled or killed for the purpose of this study.
Reporting summary
Further information on research design is available in the Nature Portfolio Reporting Summary linked to this article.
Data availability
All novel BoDV-1 sequences are available from the INSDC databases under accession numbers OR203629, OR203630, OR468838 to OR468971 ( https://www.ncbi.nlm.nih.gov/nucleotide/ ). Accession numbers and metadata are listed in a separate file (Supplemental Data Set 1 ). BoDV-1 sequences of reanalysed previously published isolates (H640 and H3053) are available under accession numbers AY374523.2 and AY374537.2. Source data are provided with this paper.
Code availability
Genome reconstruction (mapping, assembly) was performed using 454 software suite and SPAdes v3.13.1 51 64 . Sequence analysis was performed using Geneious Prime 2021.0.1; Biomatters, Auckland, New Zealand. Phylogenetic analysis was performed for complete-coding and partial seqeuences (N-X-P genes) using IQ-TREE software (version 2.2.2.6) 66 , based on a MUSCLE-Alignments 65 . Heatmap analysis was performed using pheatmap R-package 73 . Root-to-tip distances were calculated from the ML tree of N-X/P sequences using TempEst 74 . Isolation by distance analysis was perfomed using the “mantel” function of the “vegan” R-package. Geospatial data analysis and modelling was performed in R Studio (R v4.0.2) with the packages rnaturalearth 75 and ggplot2 76 . Non-parametric two-dimensional kernel density estimation was used to visualise spatial distribution patterns of mapped BoDV-1 cases, using “stat_density_2d” function in ggplot.
Dürrwald, R., Nowotny, N., Beer, M. & Kuhn JH. Clinical Virology (eds Richman D. D., Whitley R. J., Hayden F. G.). 4th edn. (American Society for Microbiology, 2016).
Richt, J. A. & Rott, R. Borna disease virus: a mystery as an emerging zoonotic pathogen. Vet. J. 161 , 24–40 (2001).
Article PubMed Google Scholar
Staeheli, P., Sauder, C., Hausmann, J., Ehrensperger, F. & Schwemmle, M. Epidemiology of Borna disease virus. J. Gen. Virol. 81 , 2123–2135 (2000).
Weissenböck, H. et al. Infections of horses and shrews with bornaviruses in Upper Austria: a novel endemic area of Borna disease. Emerg. Microbes Infect. 6 , e52 (2017).
Article PubMed PubMed Central Google Scholar
Rubbenstroth, D. et al. ICTV virus taxonomy profile: Bornaviridae . J. Gen. Virol. 102 , 001613 (2021).
Korn, K. et al. Fatal encephalitis associated with borna disease virus 1. N. Engl. J. Med. 379 , 1375–1377 (2018).
Niller, H. H. et al. Zoonotic spillover infections with Borna disease virus 1 leading to fatal human encephalitis, 1999-2019: an epidemiological investigation. Lancet Infect. Dis. 20 , 467–477 (2020).
Schlottau, K. et al. Fatal encephalitic Borna disease virus 1 in solid-organ transplant recipients. N. Engl. J. Med. 379 , 1377–1379 (2018).
Liesche, F. et al. The neuropathology of fatal encephalomyelitis in human Borna virus infection. Acta Neuropathol. 138 , 653–665 (2019).
Coras, R., Korn, K., Kuerten, S., Huttner, H. B. & Ensser, A. Severe bornavirus-encephalitis presenting as Guillain-Barre-syndrome. Acta Neuropathol. 137 , 1017–1019 (2019).
Eisermann, P. et al. Active case finding of current bornavirus infections in human encephalitis cases of unknown etiology, Germany, 2018-2020. Emerg. Infect. Dis. 27 , 1371–1379 (2021).
Tappe, D. et al. Investigation of fatal human Borna disease virus 1 encephalitis outside the previously known area for human cases, Brandenburg, Germany - a case report. BMC Infect. Dis. 21 , 787 (2021).
Frank, C. et al. Human Borna disease virus 1 (BoDV-1) encephalitis cases in the north and east of Germany. Emerg. Microbes Infect. 11 , 6–13 (2022).
Meier, H. et al. [Bornavirus encephalitis as a differential diagnosis to seronegative autoimmune encephalitis]. Nervenarzt 93 , 835–837 (2022).
Grosse, L. et al. First detected geographical cluster of BoDV-1 encephalitis from same small village in two children: therapeutic considerations and epidemiological implications. Infection https://doi.org/10.1007/s15010-023-01998-w (2023).
Neumann, B. et al. Antibodies against viral nucleo-, phospho-, and X protein contribute to serological diagnosis of fatal Borna disease virus 1 infections. Cell Rep. Med. 3 , 100499 (2022).
Pörtner, K. et al. Risk factors for Borna disease virus 1 encephalitis in Germany - a case-control study. Emerg. Microbes Infect. 12 , e2174778 (2023).
Article ADS PubMed PubMed Central Google Scholar
Liesche-Starnecker, F. et al. Hemorrhagic lesion with detection of infected endothelial cells in human bornavirus encephalitis. Acta Neuropathol. 144 , 377–379 (2022).
Stitz, L., Bilzer, T. & Planz, O. The immunopathogenesis of Borna disease virus infection. Front. Biosci. 7 , d541–d555 (2002).
Fürstenau, J. et al. Borna disease virus 1 infection in alpacas: Comparison of pathological lesions and viral distribution to other dead-end hosts. Vet. Pathol . https://doi.org/10.1177/03009858231185107 (2023).
Jacobsen, B. et al. Borna disease in an adult alpaca stallion ( Lama pacos ). J. Comp. Pathol. 143 , 203–208 (2010).
Priestnall, S. L. et al. Borna disease virus infection of a horse in Great Britain. Vet. Rec. 168 , 380b (2011).
Carbone, K. M., Duchala, C. S., Griffin, J. W., Kincaid, A. L. & Narayan, O. Pathogenesis of Borna disease in rats: evidence that intra-axonal spread is the major route for virus dissemination and the determinant for disease incubation. J. Virol. 61 , 3431–3440 (1987).
Caplazi, P. et al. Borna disease in Switzerland and in the principality of Liechtenstein. Schweiz Arch. Tierheilkd. 141 , 521–527 (1999).
PubMed Google Scholar
Schulze, V. et al. Borna disease outbreak with high mortality in an alpaca herd in a previously unreported endemic area in Germany. Transbound. Emerg. Dis. 67 , 2093–2107 (2020).
Jordan, I., Briese, T., Averett, D. R. & Lipkin, W. I. Inhibition of Borna disease virus replication by ribavirin. J. Virol. 73 , 7903–7906 (1999).
Lee, B. J., Matsunaga, H., Ikuta, K. & Tomonaga, K. Ribavirin inhibits Borna disease virus proliferation and fatal neurological diseases in neonatally infected gerbils. Antivir. Res 80 , 380–384 (2008).
Reuter, A. et al. Synergistic antiviral activity of ribavirin and interferon-alpha against parrot bornaviruses in avian cells. J. Gen. Virol. 97 , 2096–2103 (2016).
Tokunaga, T., Yamamoto, Y., Sakai, M., Tomonaga, K. & Honda, T. Antiviral activity of favipiravir (T-705) against mammalian and avian bornaviruses. Antivir. Res 143 , 237–245 (2017).
Dürrwald, R. et al. Vaccination against borna disease: overview, vaccine virus characterization and investigation of live and inactivated vaccines. Viruses 14 , 2706 (2022).
Hilbe, M. et al. Shrews as reservoir hosts of borna disease virus. Emerg. Infect. Dis. 12 , 675–677 (2006).
Puorger, M. E. et al. Distribution of Borna disease virus antigen and RNA in tissues of naturally infected bicolored white-toothed shrews, Crocidura leucodon , supporting their role as reservoir host species. Vet. Pathol. 47 , 236–244 (2010).
Dürrwald, R., Kolodziejek, J., Weissenböck, H. & Nowotny, N. The bicolored white-toothed shrew Crocidura leucodon (HERMANN 1780) is an indigenous host of mammalian Borna disease virus. PLoS ONE 9 , e93659 (2014).
Nobach, D. et al. Shedding of infectious Borna disease virus 1 in living bicolored white-toothed shrews. PLoS ONE 10 , e0137018 (2015).
Rubbenstroth, D., Schlottau, K., Schwemmle, M., Rissland, J. & Beer, M. Human bornavirus research: back on track! PLoS Pathog. 15 , e1007873 (2019).
Burgin C. J., He K. Family Soricidae (Shrews). In: Handbook of the Mammals of the World: Insectivores, Sloths and Colugos (eds Wilson D.E., Mittermeier R.A.) (Lynx Edicions, 2018).
Krapp F. Handbuch der Säugetiere Europas [Handbook of European mammals] (eds Niethammer J., Krapp F.) (Aula Verlag GmbH, 1990).
Kolodziejek, J. et al. Genetic clustering of Borna disease virus natural animal isolates, laboratory and vaccine strains strongly reflects their regional geographical origin. J. Gen. Virol. 86 , 385–398 (2005).
Dürrwald, R., Kolodziejek, J., Herzog, S. & Nowotny, N. Meta-analysis of putative human bornavirus sequences fails to provide evidence implicating Borna disease virus in mental illness. Rev. Med. Virol. 17 , 181–203 (2007).
Dürrwald, R., Kolodziejek, J., Muluneh, A., Herzog, S. & Nowotny, N. Epidemiological pattern of classical Borna disease and regional genetic clustering of Borna disease viruses point towards the existence of to-date unknown endemic reservoir host populations. Microbes Infect. 8 , 917–929 (2006).
Rubbenstroth, D. et al. Phylogenetic analysis supports horizontal transmission as a driving force of the spread of avian bornaviruses. PLoS ONE 11 , e0160936 (2016).
Bourg, M. et al. Bicolored white-toothed shrews as reservoir for Borna disease virus, Bavaria, Germany. Emerg. Infect. Dis. 19 , 2064–2066 (2013).
Allartz, P. et al. Detection of bornavirus-reactive antibodies and BoDV-1 RNA only in encephalitis patients from virus endemic areas: a comparative serological and molecular sensitivity, specificity, predictive value, and disease duration correlation study. Infection https://doi.org/10.1007/s15010-023-02048-1 (2023).
Neumann, B. et al. Cerebrospinal fluid in Borna disease virus 1 (BoDV-1) encephalitis. J. Neurol. Sci. 446 , 120568 (2023).
Bauswein, M. et al. Human infections with borna disease virus 1 (BoDV-1) primarily lead to severe encephalitis: further evidence from the seroepidemiological BoSOT study in an endemic region in Southern Germany. Viruses 15 , 188 (2023).
Rubbenstroth, D., Niller, H. H., Angstwurm, K., Schwemmle, M. & Beer, M. Are human Borna disease virus 1 infections zoonotic and fatal? - Authors’ reply. Lancet Infect. Dis. 20 , 651 (2020).
Ludwig, H. Essentials in bornavirus virology - an epilogue. APMIS Suppl. 124 , 94–97 (2008).
Article Google Scholar
Bode, L., Guo, Y. & Xie, P. Molecular epidemiology of human Borna disease virus 1 infection revisited. Emerg. Microbes Infect. 11 , 1335–1338 (2022).
Bilzer, T., Planz, O., Lipkin, W. I. & Stitz, L. Presence of CD4+ and CD8+ T cells and expression of MHC class I and MHC class II antigen in horses with Borna disease virus-induced encephalitis. Brain Pathol. 5 , 223–230 (1995).
Tappe, D. et al. Low prevalence of Borna disease virus 1 (BoDV-1) IgG antibodies in humans from areas endemic for animal Borna disease of Southern Germany. Sci. Rep. 9 , 20154 (2019).
Kupke, A. et al. Intranasal Borna disease virus (BoDV-1) infection: insights into initial steps and potential contagiosity. Int. J. Mol. Sci. 20 , 1318 (2019).
Morales, J. A., Herzog, S., Kompter, C., Frese, K. & Rott, R. Axonal transport of Borna disease virus along olfactory pathways in spontaneously and experimentally infected rats. Med. Microbiol. Immunol. 177 , 51–68 (1988).
Sauder, C. & Staeheli, P. Rat model of borna disease virus transmission: epidemiological implications. J. Virol. 77 , 12886–12890 (2003).
Krey, H. Ocular involvement in BDV-infected rabbits and primates. APMIS Suppl. 124 , 58–60 (2008).
Malbon, A. J. et al. New World camelids are sentinels for the presence of Borna disease virus. Transbound. Emerg. Dis. 69 , 451–464 (2021).
Schlegel, M. et al. Molecular identification of small mammal species using novel cytochrome B gene-derived degenerated primers. Biochem. Genet. 50 , 440–447 (2012).
Wylezich, C., Papa, A., Beer, M. & Höper, D. A versatile sample processing workflow for metagenomic pathogen detection. Sci. Rep. 8 , 13108 (2018).
Matiasek, K. et al. Mystery of fatal ‘staggering disease’ unravelled: novel rustrela virus causes severe meningoencephalomyelitis in domestic cats. Nat. Commun. 14 , 624 (2023).
Toussaint, J. F., Sailleau, C., Breard, E., Zientara, S. & De Clercq, K. Bluetongue virus detection by two real-time RT-qPCRs targeting two different genomic segments. J. Virol. Methods 140 , 115–123 (2007).
Hoffmann, B., Depner, K., Schirrmeier, H. & Beer, M. A universal heterologous internal control system for duplex real-time RT-PCR assays used in a detection system for pestiviruses. J. Virol. Methods 136 , 200–209 (2006).
Ebinger, A., Fischer, S. & Höper, D. A theoretical and generalized approach for the assessment of the sample-specific limit of detection for clinical metagenomics. Comput. Struct. Biotechnol. J. 19 , 732–742 (2020).
Szillat, K. P., Höper, D., Beer, M. & König, P. Full-genome sequencing of German rabbit haemorrhagic disease virus uncovers recombination between RHDV (GI.2) and EBHSV (GII.1). Virus Evol. 6 , veaa080 (2020).
Shen, W., Le, S., Li, Y. & Hu, F. SeqKit: a cross-platform and ultrafast toolkit for FASTA/Q file manipulation. PLoS ONE 11 , e0163962 (2016).
Bankevich, A. et al. SPAdes: a new genome assembly algorithm and its applications to single-cell sequencing. J. Comput. Biol. 19 , 455–477 (2012).
Article MathSciNet PubMed PubMed Central Google Scholar
Edgar, R. C. MUSCLE: multiple sequence alignment with high accuracy and high throughput. Nucleic Acids Res. 32 , 1792–1797 (2004).
Minh, B. Q. et al. IQ-TREE 2: new models and efficient methods for phylogenetic inference in the genomic era. Mol. Biol. Evol. 37 , 1530–1534 (2020).
Kalyaanamoorthy, S., Minh, B. Q., Wong, T. K. F., von Haeseler, A. & Jermiin, L. S. ModelFinder: fast model selection for accurate phylogenetic estimates. Nat. Methods 14 , 587–589 (2017).
Hoang, D. T., Chernomor, O., von Haeseler, A., Minh, B. Q. & Vinh, L. S. UFBoot2: improving the ultrafast bootstrap approximation. Mol. Biol. Evol. 35 , 518–522 (2018).
Guindon, S. et al. New algorithms and methods to estimate maximum-likelihood phylogenies: assessing the performance of PhyML 3.0. Syst. Biol. 59 , 307–321 (2010).
Yu, G. Using ggtree to visualize data on tree-like structures. Curr. Protoc. Bioinform. 69 , e96 (2020).
RStudio Team. RStudio: Integrated Development for R (2020).
R Core Team. R: A Language and Environment for Statistical Computing (2020).
Kolde R. pheatmap: Pretty Heatmaps (2019).
Rambaut, A., Lam, T. T., Max Carvalho, L. & Pybus, O. G. Exploring the temporal structure of heterochronous sequences using TempEst (formerly Path-O-Gen). Virus Evol. 2 , vew007 (2016).
Massicotte P. & South A. rnaturalearth: World Map Data from Natural Earth (2023).
Wickham H. ggplot2: Elegant Graphics for Data Analysis . (Springer-Verlag New York, 2016).
Venables W. N., Ripley B. D. Modern Applied Statistics with S , 4th edn. (Springer, 2002).
Download references
Acknowledgements
We would like to thank Patrick Zitzow, Kathrin Steffen, Weda Hoffmann, Lukas Wessler, Jessica Geers and Elsbeth Keller-Gautschi for their outstanding technical assistance. Brigitte Böhm, Eva Kappe (both Poing, Germany), Wolfram Breuer, Melanie Bühler, Anne Kupca (all Oberschleissheim, Germany), Gesine Buhmann, Karin Weber (both Munich, Germany), Klaus-Jürgen Danner (Freiburg, Germany), Vanessa Franzen (Munich, Germany), Sascha Gerst (Rostock, Germany), Ernst Großmann (Aulendorf, Germany), Wolfram Haider (Berlin, Germany), Anja Heinrich, Claudia Kiesow (both Stendal, Germany), Christian Imholt, Jens Jacob, Philipp Koch (Münster, Germany), Andrea Konrath, Martin Pfeffer (Leipzig, Germany), Martin Peters (Arnsberg, Germany), Dietrich Pöhle (Dresden, Germany), Ingo Schwabe (Fellbach, Germany), Christoph Schulze (Frankfurt/Oder, Germany), Herbert Weissenböck (Vienna, Austria) and Eva-Maria Wittauer (Bad Kissingen, Germany) submitted diagnostic material from confirmed or suspected cases of Borna disease or BoDV-1-infected shrews. Furthermore, we like to thank all veterinarians and physicians treating the analysed animals and human patients, respectively. We are grateful to Sybille Herzog (Giessen, Germany) for providing BoDV-1 isolates for re-sequencing, Sven Springer (IDT Biologika, now Ceva Santé Animale, Dessau-Rosslau, Germany) for kindly providing a vial of the bornavirus live vaccine ‘Dessau’ and Christiane Herden (Giessen, Germany) for providing the laboratory strain H24. We like to thank Dirk Höper for providing funding, technical supervision and advice as well as for critically discussing the data analysis and the manuscript. This work was supported by the Federal Ministry of Education and Research within the research consortium “ZooBoCo” (Grant no. 01KI1722 and 01KI2005 donated to Martin Beer, Dirk Höper, Timo Homeier-Bachmann, Kirsten Pörtner, Dennis Rubbenstroth, Dennis Tappe and Rainer G. Ulrich) and the projects “ZooKoInfekt” (01KI1903B; Rainer G. Ulrich and Dennis Rubbenstroth) and “Bornavirus - Focal Point Bavaria” (01KI2002; Barbara Schmidt). Friederike Liesche-Starnecker received funding from the German Research Foundation (DFG; no. 504757758).
Open Access funding enabled and organized by Projekt DEAL.
Author information
Authors and affiliations.
Institute of Diagnostic Virology, Friedrich-Loeffler-Institut, Greifswald-Insel Riems, Germany
Arnt Ebinger, Pauline D. Santos, Florian Pfaff, Kore Schlottau, Bernd Hoffmann, Martin Beer & Dennis Rubbenstroth
Robert Koch Institute, Department of Infectious Diseases, Unit 17 Influenza and Other Respiratory Viruses, National Reference Centre for Influenza, Berlin, Germany
Ralf Dürrwald
Institute of Virology, University of Veterinary Medicine Vienna, Vienna, Austria
Jolanta Kolodziejek & Norbert Nowotny
Center for Neuropathology and Prion Research, Faculty of Medicine, Ludwig-Maximilians-Universität München, Munich, Germany
Viktoria Ruf & Jochen Herms
Department of Neuropathology, Pathology, Medical Faculty, University of Augsburg, Augsburg, Germany
Friederike Liesche-Starnecker
Pathology, Medical Faculty, University of Augsburg, Augsburg, Germany
Institute of Virology, University Hospital Erlangen, Friedrich-Alexander Universität Erlangen-Nürnberg (FAU), Erlangen, Germany
Armin Ensser & Klaus Korn
Institute of Veterinary Pathology, Faculty of Veterinary Medicine, Leipzig University, Leipzig, Germany
Reiner Ulrich, Florian Hansmann & Marcel Suchowski
Institute of Veterinary Pathology, Freie Universität Berlin, Berlin, Germany
Jenny Fürstenau & Lars Mundhenk
Section of Clinical & Comparative Neuropathology, Centre for Clinical Veterinary Medicine, Ludwig-Maximilians-Universität München, Munich, Germany
Kaspar Matiasek
Department of Pathology, University of Veterinary Medicine Hannover, Hannover, Germany
Florian Hansmann & Wolfgang Baumgärtner
Division of Neurological Sciences, Vetsuisse Faculty, University of Bern, Bern, Switzerland
Torsten Seuberlich
Institute of Veterinary Pathology, Justus-Liebig-University Giessen, Giessen, Germany
Daniel Nobach
Chemical and Veterinary Analysis Agency Stuttgart (CVUAS), Fellbach, Germany
Bavarian Health and Food Safety Authority, Erlangen, Germany
Matthias Müller
Bavarian Health and Food Safety Authority, Oberschleißheim, Germany
Antonie Neubauer-Juric & Marcel Suchowski
Institute of Clinical Microbiology and Hygiene, Regensburg University Hospital, Regensburg, Germany
Markus Bauswein & Barbara Schmidt
Institute for Medical Microbiology, Regensburg University, Regensburg, Germany
Hans-Helmut Niller
Bernhard Nocht-Institute for Tropical Medicine, Hamburg, Germany
Dennis Tappe & Daniel Cadar
Institute of Epidemiology, Friedrich-Loeffler-Institut, Greifswald-Insel Riems, Germany
Timo Homeier-Bachmann
Institute of Novel and Emerging Infectious Diseases, Friedrich-Loeffler-Institut, Greifswald-Insel Riems, Germany
Viola C. Haring & Rainer G. Ulrich
Robert Koch Institute, Department of Infectious Disease Epidemiology, Berlin, Germany
Kirsten Pörtner & Christina Frank
Department of Basic Medical Sciences, College of Medicine, Mohammed Bin Rashid University of Medicine and Health Sciences, Dubai, United Arab Emirates
Norbert Nowotny
Department of Neuropathology, School of Medicine, Institute of Pathology, Technical University Munich, Munich, Germany
Jürgen Schlegel
You can also search for this author in PubMed Google Scholar
Contributions
D.R. initiated and conceptualised the study. R.D., J.K., V.R., F.L.S., R.U., J.F., K.M., F.H., T.S., D.N., M.M., A.N.J., M.S., M.Ba., H.H.N., B.S., V.C.H., K.P., C.F., L.M., J.H., W.B., N.N., J.S. and R.G.U. provided samples together with clinical, histopathological and initial laboratory diagnosis and assembly of metadata. A.Eb., P.D.S., D.R., D.T., D.C., K.K., A.En., J.K., K.S., B.H. and D.N. performed PCR assays and sequencing. D.R., A.Eb., F.P. and T.H.B. analysed and visualised the data. R.G.U. and M.Be. supervised parts of the study. D.R. and A.Eb. wrote and D.R. finalised the manuscript. The manuscript was critically reviewed through the contributions of all authors.
Corresponding author
Correspondence to Dennis Rubbenstroth .
Ethics declarations
Competing interests.
The authors declare no competing interests.
Peer review
Peer review information.
Nature Communications thanks Andrés Velasco-Villa and the other, anonymous, reviewer(s) for their contribution to the peer review of this work. A peer review file is available.
Additional information
Publisher’s note Springer Nature remains neutral with regard to jurisdictional claims in published maps and institutional affiliations.
Supplementary information
Supplementary information, peer review file, description of additional supplementary files, supplementary data 1, supplementary data 2, reporting summary, source data, source data, rights and permissions.
Open Access This article is licensed under a Creative Commons Attribution 4.0 International License, which permits use, sharing, adaptation, distribution and reproduction in any medium or format, as long as you give appropriate credit to the original author(s) and the source, provide a link to the Creative Commons licence, and indicate if changes were made. The images or other third party material in this article are included in the article’s Creative Commons licence, unless indicated otherwise in a credit line to the material. If material is not included in the article’s Creative Commons licence and your intended use is not permitted by statutory regulation or exceeds the permitted use, you will need to obtain permission directly from the copyright holder. To view a copy of this licence, visit http://creativecommons.org/licenses/by/4.0/ .
Reprints and permissions
About this article
Cite this article.
Ebinger, A., Santos, P.D., Pfaff, F. et al. Lethal Borna disease virus 1 infections of humans and animals – in-depth molecular epidemiology and phylogeography. Nat Commun 15 , 7908 (2024). https://doi.org/10.1038/s41467-024-52192-x
Download citation
Received : 20 November 2023
Accepted : 27 August 2024
Published : 10 September 2024
DOI : https://doi.org/10.1038/s41467-024-52192-x
Share this article
Anyone you share the following link with will be able to read this content:
Sorry, a shareable link is not currently available for this article.
Provided by the Springer Nature SharedIt content-sharing initiative
By submitting a comment you agree to abide by our Terms and Community Guidelines . If you find something abusive or that does not comply with our terms or guidelines please flag it as inappropriate.
Quick links
- Explore articles by subject
- Guide to authors
- Editorial policies
Sign up for the Nature Briefing newsletter — what matters in science, free to your inbox daily.

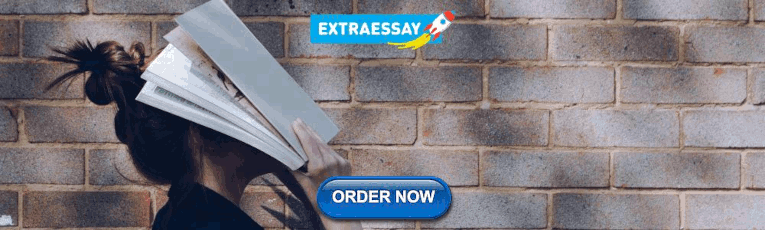
IMAGES
COMMENTS
Whether infection is a risk factor for development of T2D is mostly shown for chronic viruses. A population-based matched case-control cohort study in Korea selected 576 patients infected with cytomegalovirus (CMV), but without T2D and 2.880 matched controls without either condition and followed them for 5 years for development of new onset T2D ...
This case asks students to examine data on children diagnosed with Type 1 Diabetes after reading a short story about a young girl who was losing weight and feeling thirsty. The content looks at a possible connection between diabetes and infection by the coxsackie virus, a virus that is responsible for "hand, foot, and mouth" disease.
Several studies reported that viruses can promote the ... M. et al. Kaposi's sarcoma-associated herpesvirus seropositivity is associated with type 2 diabetes mellitus: a case-control study in ...
For over a century, viruses have left a long trail of evidence implicating them as frequent suspects in the development of type 1 diabetes. Through vigorous interrogation of viral infections in individuals with islet autoimmunity and type 1 diabetes using serological and molecular virus detection methods, as well as mechanistic studies of virus-infected human pancreatic β-cells, the prime ...
In this case, the factor they are investigating is the exposure to the Coxsackievirus B and whether that increases the risk of diabetes. There are two methods for conducting this type of study: Case Control Studies examine a series of patients who have a disease and a control group that does not have the disease.
Some observational studies and meta-analyses suggested an increase in the incidence of diabetes mellitus or a correlation between SARS-CoV-2 infection and new-onset diabetes mellitus, without ...
A significant number of viruses have been associated with type 1 diabetes, including enteroviruses such as Coxsackievirus B (CVB) (), but also rotavirus (5,6), mumps virus (), and cytomegalovirus ().Rubella virus has been suggested to cause type 1 diabetes, but so far only congenital rubella syndrome has conclusively been associated with the disease (9-11).
How COVID-19 Can Lead to Diabetes. Submitted by Anonymous (not verified) on June 8, 2021. Along with the pneumonia, blood clots, and other serious health concerns caused by SARS-CoV-2, the COVID-19 virus, some studies have also identified another troubling connection. Some people can develop diabetes after an acute COVID-19 infection.
The latest clue comes from an experimental study in miniature lab-grown pancreases published last week 8 suggests that the virus might trigger diabetes by damaging the cells that control blood ...
Altogether, it is clear that viruses can induce T1DM through different mechanisms (Fig. 1), and susceptible individuals are more prone to develop this disease after infection.A list of viruses that induce T1DM and their main outcomes are summarized in Table 1.However, albeit difficult, extensive studies should be made to calculate the influence of virus infection in new diagnosed T1DM cases.
Type of study (case/control) Test and Sample Virus Findings; Schulte BM et al. 2010 : Case control (10/20) ... which can cause increased secretion of aldosterone and renal potassium loss. ... Finnish Trigr Study Group Enterovirus infections as a risk factor for type I diabetes: virus analyses in a dietary intervention trial. Clin Exp Immunol ...
In one study, researchers examined lab-grown beta cells infected with COVID-19 and found that they made less insulin when infected with the virus. Some of the cells died outright. In the other study, researchers found that infection effectively reprogrammed some of those lab-grown cells. Instead of producing insulin, which breaks down blood ...
Research team describes how a virus can cause diabetes. It has recently been described that infection by some enteroviruses—a genus of viruses that commonly cause diseases of varying severity ...
New cases of diabetes. At the start of the coronavirus pandemic, doctors started to raise concerns around new cases of both type 1 and type 2 diabetes in people who had caught the virus.. Type 1 diabetes. Since then, small studies have suggested that rates of new type 1 diabetes diagnoses in children are higher in 2020 compared to average rates in previous years.
This high level of glucose, termed hyperglycemia, is the hallmark of diabetes. "Earlier lab studies had suggested that [the COVID-19 virus] can infect human beta cells," said Dr. Francis ...
The question has been raised as to whether SARS-CoV-2, the pathogenic virus underlying COVID-19, can cause diabetes, among its many effects. But attribution beyond the occasional coincidence ...
The same is true of the association between COVID-19 and diabetes, Dr. Duggal says. It could be that a COVID-19 infection triggers changes in your body that can lead to diabetes in some people. Or ...
The COVID-19 pandemic with the outbreak of the severe acute respiratory syndrome coronavirus 2 (SARS-CoV-2) virus has been one of the largest topics of discussion in the medical world over the last few years. Most of the research has focused on the risks and correlation of chronic diseases and immunosuppression with the severity and mortality of the viral infection.
Type 1 diabetes is an autoimmune condition where the pancreas cannot produce insulin, whereas type 2 diabetes is the body's resistance to insulin. Previous studies have linked viruses to ...
One study demonstrated that acute respiratory virus infection increases IFNγ production, and it causes muscle insulin resistance in humans, which drives compensatory hyperinsulinaemia to maintain ...
comparing type 1 diabetes to ".. having a key," and type 2 diabetes as ".. a broken. key." Our case study assignment touches on type 1 diabetes and highlights some of the same. symptoms of the article in the example of the little girl Rianne. Rianne is thirsty and has. increased her fluids, she is tired, and she has lost weight.
Elevated blood glucose concentration, also known as hyperglycemia, has been identified as a significant factor influencing the prognosis of COVID-19, alongside the impact of the SARS-CoV-2 infection itself. This research is a cross-sectional investigation that examined the relationship between COVID-19 and hyperglycemia in patients admitted to Afzalipour Hospital in Kerman, Iran, from July to ...
Certain viruses might promote autoimmunity. A significant number of viruses have been associated with type 1 diabetes, including enteroviruses such as Coxsackievirus B (CVB) (), but also rotavirus (5,6), mumps virus (), and cytomegalovirus ().Rubella virus has been suggested to cause type 1 diabetes, but so far only congenital rubella syndrome has conclusively been associated with the disease ...
Borna disease virus 1 (BoDV-1) is a zoonotic pathogen endemic in bicoloured white-toothed shrews in Central Europe that can cause fatal encephalitis in humans and other mammals. Here, the authors ...