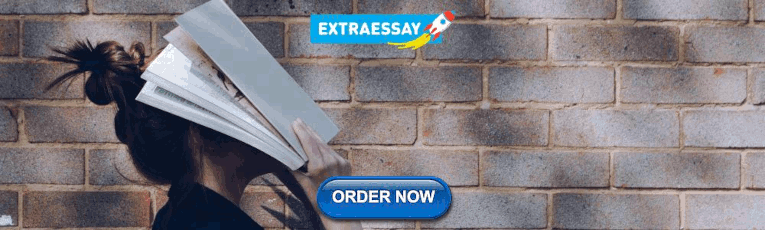
Molecular Genetics and Genomics
Molecular Genetics and Genomics is a peer-reviewed journal covering all areas of genetics and genomics, spanning experimental, theoretical, and synthetic approaches.
- The first journal on genetics, founded in 1908.
- Publishes research that provides provide new insights into the fundamental mechanisms underlying genetic and genomic processes.
- Authors have the choice to publish using either the traditional publishing route or immediate gold Open Access.
- There are no publication charges except for special services.
- Free of charge for color art in both print and online publication.
- Joan Cerdà,
Latest articles
Genotypic spectrum of abca4 -associated retinal degenerations in 211 unrelated mexican patients: identification of 22 novel disease-causing variants.
- Oscar F. Chacon-Camacho
- Nancy Xilotl-de Jesús
- Juan Carlos Zenteno
Forensic height estimation using polygenic score in Korean population
- Hye-Won Cho
- Hyun-Seok Jin
- Yong-Bin Eom
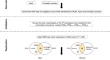
CACNA1H restrains chemotherapy resistance in ovarian clear cell carcinoma cells by repressing autophagy
- Huaijing Shi
- Liang Zheng
- Hongju Chen
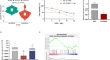
Identifying the key hub genes linked with lung squamous cell carcinoma by examining the differentially expressed and survival genes
- Anushka Pravin Chawhan
- Norine Dsouza
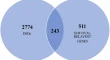
CRISPR-Cpf1 system and its applications in animal genome editing
- Lihong Guan
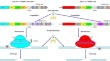
Journal updates
Editor-in-chief stefan hohmann passed away on 2 july 2021, top 10 downloaded articles 2020, journal information.
- Biological Abstracts
- CAB Abstracts
- Chemical Abstracts Service (CAS)
- Current Contents/Life Sciences
- Google Scholar
- IFIS Publishing
- Japanese Science and Technology Agency (JST)
- Norwegian Register for Scientific Journals and Series
- OCLC WorldCat Discovery Service
- Pathway Studio
- Science Citation Index Expanded (SCIE)
- TD Net Discovery Service
- UGC-CARE List (India)
- Zoological Record
Rights and permissions
Editorial policies
© Springer-Verlag GmbH Germany, part of Springer Nature
- Find a journal
- Publish with us
- Track your research
Genetics and Molecular Research
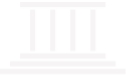
Subject Area and Category
- Molecular Biology
- Medicine (miscellaneous)
Fundacao de Pesquisas Cientificas de Ribeirao Preto
Publication type
Information.
How to publish in this journal
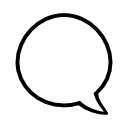
The set of journals have been ranked according to their SJR and divided into four equal groups, four quartiles. Q1 (green) comprises the quarter of the journals with the highest values, Q2 (yellow) the second highest values, Q3 (orange) the third highest values and Q4 (red) the lowest values.
Category | Year | Quartile |
---|---|---|
Genetics | 2003 | Q4 |
Genetics | 2004 | Q4 |
Genetics | 2005 | Q3 |
Genetics | 2006 | Q4 |
Genetics | 2007 | Q4 |
Genetics | 2008 | Q4 |
Genetics | 2009 | Q4 |
Genetics | 2010 | Q4 |
Genetics | 2011 | Q4 |
Genetics | 2012 | Q4 |
Genetics | 2013 | Q4 |
Genetics | 2014 | Q4 |
Genetics | 2015 | Q4 |
Genetics | 2016 | Q4 |
Genetics | 2017 | Q4 |
Genetics | 2018 | Q4 |
Genetics | 2019 | Q4 |
Genetics | 2020 | Q4 |
Genetics | 2021 | Q4 |
Genetics | 2022 | Q4 |
Genetics | 2023 | Q4 |
Medicine (miscellaneous) | 2003 | Q3 |
Medicine (miscellaneous) | 2004 | Q2 |
Medicine (miscellaneous) | 2005 | Q2 |
Medicine (miscellaneous) | 2006 | Q2 |
Medicine (miscellaneous) | 2007 | Q2 |
Medicine (miscellaneous) | 2008 | Q3 |
Medicine (miscellaneous) | 2009 | Q2 |
Medicine (miscellaneous) | 2010 | Q2 |
Medicine (miscellaneous) | 2011 | Q2 |
Medicine (miscellaneous) | 2012 | Q2 |
Medicine (miscellaneous) | 2013 | Q3 |
Medicine (miscellaneous) | 2014 | Q3 |
Medicine (miscellaneous) | 2015 | Q3 |
Medicine (miscellaneous) | 2016 | Q3 |
Medicine (miscellaneous) | 2017 | Q3 |
Medicine (miscellaneous) | 2018 | Q2 |
Medicine (miscellaneous) | 2019 | Q3 |
Medicine (miscellaneous) | 2020 | Q3 |
Medicine (miscellaneous) | 2021 | Q4 |
Medicine (miscellaneous) | 2022 | Q4 |
Medicine (miscellaneous) | 2023 | Q4 |
Molecular Biology | 2003 | Q4 |
Molecular Biology | 2004 | Q4 |
Molecular Biology | 2005 | Q4 |
Molecular Biology | 2006 | Q4 |
Molecular Biology | 2007 | Q4 |
Molecular Biology | 2008 | Q4 |
Molecular Biology | 2009 | Q4 |
Molecular Biology | 2010 | Q4 |
Molecular Biology | 2011 | Q4 |
Molecular Biology | 2012 | Q4 |
Molecular Biology | 2013 | Q4 |
Molecular Biology | 2014 | Q4 |
Molecular Biology | 2015 | Q4 |
Molecular Biology | 2016 | Q4 |
Molecular Biology | 2017 | Q4 |
Molecular Biology | 2018 | Q4 |
Molecular Biology | 2019 | Q4 |
Molecular Biology | 2020 | Q4 |
Molecular Biology | 2021 | Q4 |
Molecular Biology | 2022 | Q4 |
Molecular Biology | 2023 | Q4 |
The SJR is a size-independent prestige indicator that ranks journals by their 'average prestige per article'. It is based on the idea that 'all citations are not created equal'. SJR is a measure of scientific influence of journals that accounts for both the number of citations received by a journal and the importance or prestige of the journals where such citations come from It measures the scientific influence of the average article in a journal, it expresses how central to the global scientific discussion an average article of the journal is.
Year | SJR |
---|---|
2003 | 0.146 |
2004 | 0.228 |
2005 | 0.539 |
2006 | 0.483 |
2007 | 0.334 |
2008 | 0.273 |
2009 | 0.363 |
2010 | 0.337 |
2011 | 0.371 |
2012 | 0.369 |
2013 | 0.380 |
2014 | 0.379 |
2015 | 0.371 |
2016 | 0.380 |
2017 | 0.439 |
2018 | 0.446 |
2019 | 0.439 |
2020 | 0.356 |
2021 | 0.244 |
2022 | 0.217 |
2023 | 0.173 |
Evolution of the number of published documents. All types of documents are considered, including citable and non citable documents.
Year | Documents |
---|---|
2002 | 36 |
2003 | 41 |
2004 | 50 |
2005 | 81 |
2006 | 82 |
2007 | 124 |
2008 | 149 |
2009 | 162 |
2010 | 260 |
2011 | 420 |
2012 | 495 |
2013 | 713 |
2014 | 1143 |
2015 | 2058 |
2016 | 1315 |
2017 | 480 |
2018 | 81 |
2019 | 115 |
2020 | 88 |
2021 | 84 |
2022 | 36 |
2023 | 31 |
This indicator counts the number of citations received by documents from a journal and divides them by the total number of documents published in that journal. The chart shows the evolution of the average number of times documents published in a journal in the past two, three and four years have been cited in the current year. The two years line is equivalent to journal impact factor ™ (Thomson Reuters) metric.
Cites per document | Year | Value |
---|---|---|
Cites / Doc. (4 years) | 2002 | 0.000 |
Cites / Doc. (4 years) | 2003 | 0.278 |
Cites / Doc. (4 years) | 2004 | 0.727 |
Cites / Doc. (4 years) | 2005 | 1.291 |
Cites / Doc. (4 years) | 2006 | 1.240 |
Cites / Doc. (4 years) | 2007 | 1.252 |
Cites / Doc. (4 years) | 2008 | 1.000 |
Cites / Doc. (4 years) | 2009 | 1.078 |
Cites / Doc. (4 years) | 2010 | 1.122 |
Cites / Doc. (4 years) | 2011 | 1.314 |
Cites / Doc. (4 years) | 2012 | 1.196 |
Cites / Doc. (4 years) | 2013 | 1.224 |
Cites / Doc. (4 years) | 2014 | 1.167 |
Cites / Doc. (4 years) | 2015 | 1.056 |
Cites / Doc. (4 years) | 2016 | 1.012 |
Cites / Doc. (4 years) | 2017 | 0.985 |
Cites / Doc. (4 years) | 2018 | 0.986 |
Cites / Doc. (4 years) | 2019 | 1.108 |
Cites / Doc. (4 years) | 2020 | 1.068 |
Cites / Doc. (4 years) | 2021 | 1.058 |
Cites / Doc. (4 years) | 2022 | 0.764 |
Cites / Doc. (4 years) | 2023 | 0.613 |
Cites / Doc. (3 years) | 2002 | 0.000 |
Cites / Doc. (3 years) | 2003 | 0.278 |
Cites / Doc. (3 years) | 2004 | 0.727 |
Cites / Doc. (3 years) | 2005 | 1.291 |
Cites / Doc. (3 years) | 2006 | 1.256 |
Cites / Doc. (3 years) | 2007 | 1.038 |
Cites / Doc. (3 years) | 2008 | 0.885 |
Cites / Doc. (3 years) | 2009 | 1.031 |
Cites / Doc. (3 years) | 2010 | 1.037 |
Cites / Doc. (3 years) | 2011 | 1.319 |
Cites / Doc. (3 years) | 2012 | 1.171 |
Cites / Doc. (3 years) | 2013 | 1.197 |
Cites / Doc. (3 years) | 2014 | 1.084 |
Cites / Doc. (3 years) | 2015 | 1.011 |
Cites / Doc. (3 years) | 2016 | 0.979 |
Cites / Doc. (3 years) | 2017 | 0.985 |
Cites / Doc. (3 years) | 2018 | 0.982 |
Cites / Doc. (3 years) | 2019 | 1.087 |
Cites / Doc. (3 years) | 2020 | 1.071 |
Cites / Doc. (3 years) | 2021 | 0.725 |
Cites / Doc. (3 years) | 2022 | 0.652 |
Cites / Doc. (3 years) | 2023 | 0.486 |
Cites / Doc. (2 years) | 2002 | 0.000 |
Cites / Doc. (2 years) | 2003 | 0.278 |
Cites / Doc. (2 years) | 2004 | 0.727 |
Cites / Doc. (2 years) | 2005 | 1.352 |
Cites / Doc. (2 years) | 2006 | 0.855 |
Cites / Doc. (2 years) | 2007 | 0.902 |
Cites / Doc. (2 years) | 2008 | 0.791 |
Cites / Doc. (2 years) | 2009 | 0.901 |
Cites / Doc. (2 years) | 2010 | 1.023 |
Cites / Doc. (2 years) | 2011 | 1.273 |
Cites / Doc. (2 years) | 2012 | 1.085 |
Cites / Doc. (2 years) | 2013 | 1.004 |
Cites / Doc. (2 years) | 2014 | 0.962 |
Cites / Doc. (2 years) | 2015 | 0.939 |
Cites / Doc. (2 years) | 2016 | 0.943 |
Cites / Doc. (2 years) | 2017 | 0.952 |
Cites / Doc. (2 years) | 2018 | 0.891 |
Cites / Doc. (2 years) | 2019 | 0.950 |
Cites / Doc. (2 years) | 2020 | 0.587 |
Cites / Doc. (2 years) | 2021 | 0.616 |
Cites / Doc. (2 years) | 2022 | 0.453 |
Cites / Doc. (2 years) | 2023 | 0.400 |
Evolution of the total number of citations and journal's self-citations received by a journal's published documents during the three previous years. Journal Self-citation is defined as the number of citation from a journal citing article to articles published by the same journal.
Cites | Year | Value |
---|---|---|
Self Cites | 2002 | 0 |
Self Cites | 2003 | 3 |
Self Cites | 2004 | 1 |
Self Cites | 2005 | 7 |
Self Cites | 2006 | 5 |
Self Cites | 2007 | 17 |
Self Cites | 2008 | 28 |
Self Cites | 2009 | 36 |
Self Cites | 2010 | 33 |
Self Cites | 2011 | 56 |
Self Cites | 2012 | 53 |
Self Cites | 2013 | 146 |
Self Cites | 2014 | 192 |
Self Cites | 2015 | 312 |
Self Cites | 2016 | 740 |
Self Cites | 2017 | 263 |
Self Cites | 2018 | 55 |
Self Cites | 2019 | 62 |
Self Cites | 2020 | 34 |
Self Cites | 2021 | 13 |
Self Cites | 2022 | 9 |
Self Cites | 2023 | 7 |
Total Cites | 2002 | 0 |
Total Cites | 2003 | 10 |
Total Cites | 2004 | 56 |
Total Cites | 2005 | 164 |
Total Cites | 2006 | 216 |
Total Cites | 2007 | 221 |
Total Cites | 2008 | 254 |
Total Cites | 2009 | 366 |
Total Cites | 2010 | 451 |
Total Cites | 2011 | 753 |
Total Cites | 2012 | 986 |
Total Cites | 2013 | 1406 |
Total Cites | 2014 | 1764 |
Total Cites | 2015 | 2376 |
Total Cites | 2016 | 3833 |
Total Cites | 2017 | 4448 |
Total Cites | 2018 | 3784 |
Total Cites | 2019 | 2039 |
Total Cites | 2020 | 724 |
Total Cites | 2021 | 206 |
Total Cites | 2022 | 187 |
Total Cites | 2023 | 101 |
Evolution of the number of total citation per document and external citation per document (i.e. journal self-citations removed) received by a journal's published documents during the three previous years. External citations are calculated by subtracting the number of self-citations from the total number of citations received by the journal’s documents.
Cites | Year | Value |
---|---|---|
External Cites per document | 2002 | 0 |
External Cites per document | 2003 | 0.194 |
External Cites per document | 2004 | 0.714 |
External Cites per document | 2005 | 1.236 |
External Cites per document | 2006 | 1.227 |
External Cites per document | 2007 | 0.958 |
External Cites per document | 2008 | 0.787 |
External Cites per document | 2009 | 0.930 |
External Cites per document | 2010 | 0.961 |
External Cites per document | 2011 | 1.221 |
External Cites per document | 2012 | 1.108 |
External Cites per document | 2013 | 1.072 |
External Cites per document | 2014 | 0.966 |
External Cites per document | 2015 | 0.878 |
External Cites per document | 2016 | 0.790 |
External Cites per document | 2017 | 0.927 |
External Cites per document | 2018 | 0.968 |
External Cites per document | 2019 | 1.054 |
External Cites per document | 2020 | 1.021 |
External Cites per document | 2021 | 0.680 |
External Cites per document | 2022 | 0.620 |
External Cites per document | 2023 | 0.452 |
Cites per document | 2002 | 0.000 |
Cites per document | 2003 | 0.278 |
Cites per document | 2004 | 0.727 |
Cites per document | 2005 | 1.291 |
Cites per document | 2006 | 1.256 |
Cites per document | 2007 | 1.038 |
Cites per document | 2008 | 0.885 |
Cites per document | 2009 | 1.031 |
Cites per document | 2010 | 1.037 |
Cites per document | 2011 | 1.319 |
Cites per document | 2012 | 1.171 |
Cites per document | 2013 | 1.197 |
Cites per document | 2014 | 1.084 |
Cites per document | 2015 | 1.011 |
Cites per document | 2016 | 0.979 |
Cites per document | 2017 | 0.985 |
Cites per document | 2018 | 0.982 |
Cites per document | 2019 | 1.087 |
Cites per document | 2020 | 1.071 |
Cites per document | 2021 | 0.725 |
Cites per document | 2022 | 0.652 |
Cites per document | 2023 | 0.486 |
International Collaboration accounts for the articles that have been produced by researchers from several countries. The chart shows the ratio of a journal's documents signed by researchers from more than one country; that is including more than one country address.
Year | International Collaboration |
---|---|
2002 | 25.00 |
2003 | 21.95 |
2004 | 18.00 |
2005 | 1.23 |
2006 | 12.20 |
2007 | 11.29 |
2008 | 6.71 |
2009 | 12.96 |
2010 | 6.54 |
2011 | 9.29 |
2012 | 3.43 |
2013 | 10.24 |
2014 | 7.61 |
2015 | 5.20 |
2016 | 7.45 |
2017 | 10.83 |
2018 | 13.58 |
2019 | 15.65 |
2020 | 17.05 |
2021 | 4.76 |
2022 | 2.78 |
2023 | 12.90 |
Not every article in a journal is considered primary research and therefore "citable", this chart shows the ratio of a journal's articles including substantial research (research articles, conference papers and reviews) in three year windows vs. those documents other than research articles, reviews and conference papers.
Documents | Year | Value |
---|---|---|
Non-citable documents | 2002 | 0 |
Non-citable documents | 2003 | 0 |
Non-citable documents | 2004 | 0 |
Non-citable documents | 2005 | 0 |
Non-citable documents | 2006 | 2 |
Non-citable documents | 2007 | 4 |
Non-citable documents | 2008 | 6 |
Non-citable documents | 2009 | 5 |
Non-citable documents | 2010 | 4 |
Non-citable documents | 2011 | 2 |
Non-citable documents | 2012 | 4 |
Non-citable documents | 2013 | 7 |
Non-citable documents | 2014 | 8 |
Non-citable documents | 2015 | 9 |
Non-citable documents | 2016 | 8 |
Non-citable documents | 2017 | 14 |
Non-citable documents | 2018 | 11 |
Non-citable documents | 2019 | 8 |
Non-citable documents | 2020 | 1 |
Non-citable documents | 2021 | 0 |
Non-citable documents | 2022 | 1 |
Non-citable documents | 2023 | 1 |
Citable documents | 2002 | 0 |
Citable documents | 2003 | 36 |
Citable documents | 2004 | 77 |
Citable documents | 2005 | 127 |
Citable documents | 2006 | 170 |
Citable documents | 2007 | 209 |
Citable documents | 2008 | 281 |
Citable documents | 2009 | 350 |
Citable documents | 2010 | 431 |
Citable documents | 2011 | 569 |
Citable documents | 2012 | 838 |
Citable documents | 2013 | 1168 |
Citable documents | 2014 | 1620 |
Citable documents | 2015 | 2342 |
Citable documents | 2016 | 3906 |
Citable documents | 2017 | 4502 |
Citable documents | 2018 | 3842 |
Citable documents | 2019 | 1868 |
Citable documents | 2020 | 675 |
Citable documents | 2021 | 284 |
Citable documents | 2022 | 286 |
Citable documents | 2023 | 207 |
Ratio of a journal's items, grouped in three years windows, that have been cited at least once vs. those not cited during the following year.
Documents | Year | Value |
---|---|---|
Uncited documents | 2002 | 0 |
Uncited documents | 2003 | 28 |
Uncited documents | 2004 | 49 |
Uncited documents | 2005 | 56 |
Uncited documents | 2006 | 95 |
Uncited documents | 2007 | 100 |
Uncited documents | 2008 | 146 |
Uncited documents | 2009 | 172 |
Uncited documents | 2010 | 207 |
Uncited documents | 2011 | 240 |
Uncited documents | 2012 | 390 |
Uncited documents | 2013 | 518 |
Uncited documents | 2014 | 772 |
Uncited documents | 2015 | 1149 |
Uncited documents | 2016 | 1876 |
Uncited documents | 2017 | 2159 |
Uncited documents | 2018 | 1843 |
Uncited documents | 2019 | 839 |
Uncited documents | 2020 | 317 |
Uncited documents | 2021 | 171 |
Uncited documents | 2022 | 178 |
Uncited documents | 2023 | 141 |
Cited documents | 2002 | 0 |
Cited documents | 2003 | 8 |
Cited documents | 2004 | 28 |
Cited documents | 2005 | 71 |
Cited documents | 2006 | 77 |
Cited documents | 2007 | 113 |
Cited documents | 2008 | 141 |
Cited documents | 2009 | 183 |
Cited documents | 2010 | 228 |
Cited documents | 2011 | 331 |
Cited documents | 2012 | 452 |
Cited documents | 2013 | 657 |
Cited documents | 2014 | 856 |
Cited documents | 2015 | 1202 |
Cited documents | 2016 | 2038 |
Cited documents | 2017 | 2357 |
Cited documents | 2018 | 2010 |
Cited documents | 2019 | 1037 |
Cited documents | 2020 | 359 |
Cited documents | 2021 | 113 |
Cited documents | 2022 | 109 |
Cited documents | 2023 | 67 |
Evolution of the percentage of female authors.
Year | Female Percent |
---|---|
2002 | 41.96 |
2003 | 42.11 |
2004 | 47.94 |
2005 | 45.22 |
2006 | 38.26 |
2007 | 37.90 |
2008 | 47.20 |
2009 | 43.05 |
2010 | 43.95 |
2011 | 38.79 |
2012 | 40.28 |
2013 | 40.59 |
2014 | 39.95 |
2015 | 40.36 |
2016 | 41.53 |
2017 | 41.01 |
2018 | 43.05 |
2019 | 42.22 |
2020 | 42.14 |
2021 | 43.40 |
2022 | 53.47 |
2023 | 43.14 |
Evolution of the number of documents cited by public policy documents according to Overton database.
Documents | Year | Value |
---|---|---|
Overton | 2002 | 0 |
Overton | 2003 | 0 |
Overton | 2004 | 0 |
Overton | 2005 | 0 |
Overton | 2006 | 0 |
Overton | 2007 | 0 |
Overton | 2008 | 6 |
Overton | 2009 | 15 |
Overton | 2010 | 11 |
Overton | 2011 | 9 |
Overton | 2012 | 0 |
Overton | 2013 | 1 |
Overton | 2014 | 0 |
Overton | 2015 | 2 |
Overton | 2016 | 18 |
Overton | 2017 | 7 |
Overton | 2018 | 1 |
Overton | 2019 | 0 |
Overton | 2020 | 0 |
Overton | 2021 | 0 |
Overton | 2022 | 0 |
Overton | 2023 | 0 |
Evoution of the number of documents related to Sustainable Development Goals defined by United Nations. Available from 2018 onwards.
Documents | Year | Value |
---|---|---|
SDG | 2018 | 29 |
SDG | 2019 | 42 |
SDG | 2020 | 30 |
SDG | 2021 | 36 |
SDG | 2022 | 16 |
SDG | 2023 | 14 |
Leave a comment
Name * Required
Email (will not be published) * Required
* Required Cancel
The users of Scimago Journal & Country Rank have the possibility to dialogue through comments linked to a specific journal. The purpose is to have a forum in which general doubts about the processes of publication in the journal, experiences and other issues derived from the publication of papers are resolved. For topics on particular articles, maintain the dialogue through the usual channels with your editor.
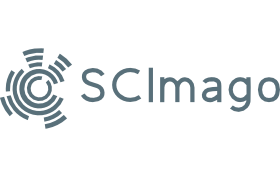
Follow us on @ScimagoJR Scimago Lab , Copyright 2007-2024. Data Source: Scopus®

Cookie settings
Cookie Policy
Legal Notice
Privacy Policy

An official website of the United States government
The .gov means it’s official. Federal government websites often end in .gov or .mil. Before sharing sensitive information, make sure you’re on a federal government site.
The site is secure. The https:// ensures that you are connecting to the official website and that any information you provide is encrypted and transmitted securely.
- Publications
- Account settings
Preview improvements coming to the PMC website in October 2024. Learn More or Try it out now .
- Advanced Search
- Journal List
- BMC Res Notes

Methods in molecular biology and genetics: looking to the future
Diego a. forero.
1 School of Health and Sport Sciences, Fundación Universitaria del Área Andina, Bogotá, Colombia
Vaibhav Chand
2 Department of Biochemistry and Molecular Genetics, University of Illinois at Chicago, Chicago, USA
Associated Data
Not applicable.
In recent decades, advances in methods in molecular biology and genetics have revolutionized multiple areas of the life and health sciences. However, there remains a global need for the development of more refined and effective methods across these fields of research. In this current Collection, we aim to showcase articles presenting novel molecular biology and genetics techniques developed by scientists from around the world.
A brief overview of the development of methods of molecular biology and genetics
Since ancient times, humankind has recognized the influence of heredity, based on familial resemblance, selective breeding of livestock, and climate-adapted crops. Prior to Gregor Johann Mendel’s work in the nineteenth century, there was no clear scientific theory to explain heredity. Mendel’s work remained essentially theoretical until the discovery of DNA and confirmation of its role as the principal agent of heredity in organisms in the twentieth century [ 1 ]. In addition, the resolution of the DNA structure paved the way for the invention of the Polymerase Chain Reaction (PCR) (by Kary Mullis), nucleotide synthesis [ 2 ] and the Sanger sequencing method [ 3 ] which revolutionized the field of genetics and led to the development of several sub-disciplines, including cytogenetics, biotechnology, bioprocess technology, and molecular biology. Automation of Sanger sequencing led to the Human Genome Project in 1990 [ 1 ], soon followed by sequencing the complete genomes of numerous other species of flora and fauna [ 4 ].
In recent decades, advances in methods in molecular biology and genetics have revolutionized multiple areas of life and health sciences [ 2 ]. As a major example from health sciences, PCR-based methods have advanced our understanding of the aetiology of a myriad of acute and chronic diseases, in addition to allowing the diagnosis of multiple disorders [ 1 , 5 ]. As a recent global application of molecular methods, the PCR-based approaches have led to the processing of hundreds of millions of samples for the analysis of the SARS-CoV-2 virus [ 6 ]. In addition, molecular methods have been key for the creation of multiple companies, products and jobs [ 7 ].
The development of sequencing technologies and their iterative improvements have been instrumental in advancing the understanding of DNA and RNA, their identification, association with various proteins, their covalent modifications, the function of the genes they carry, and the function of the non-coding portion of DNA and RNA in normal and diseased cells, in pathogenic bacteria and viruses, and in plants [ 8 , 9 ]. By producing RNA-based vaccines, we were able to combat the recent SARS-CoV2 pandemic. This was made possible by sequencing and in vitro nucleotide synthesis technologies [ 10 ].
Gene editing technologies, such as restriction endonuclease digestion, transcription activator-like effector nucleases (TALENs), and the clustered regularly interspaced short palindromic repeats (CRISPR-Cas) system, are an additional development in the field of molecular biology that has aided in the understanding of DNA and genes. There is optimism about the use of CRISPR-Cas9 technology in the treatment of a wide variety of diseases, such as cancer, blood-related diseases, hereditary blindness, cystic fibrosis, viral diseases, muscular dystrophy, and Huntington´s disease, due to its precision and its constant improvement, in comparison with other gene-editing technologies [ 15 ].
Need for novel methods in molecular biology and genetics
There is a global need for the development of novel methods for molecular biology and genetics. Particularly, in the area of human health, there is a need for further approaches that facilitate point-of-care molecular analysis (particularly miniaturized and portable platforms), for infectious and non-transmissible diseases [ 11 ], the development of more efficient methods for DNA sequencing [ 3 ], which facilitate cost-effective genome-wide analysis of patients, among others.
In addition, three key factors would also help push this field forward: additional research comparing the performance of different methods for molecular biology [ 12 ], the broader use of reporting standards (such as the Minimum Information for Publication of Quantitative Real-Time PCR Experiments -MIQE-, which describes details of experimental conditions) [ 13 ], and the increased participation of scientists from the Global South.
Although older techniques, such as x-ray crystallography, gene cloning, PCR, and sequencing, have been instrumental in the study of various aspects of genetics, these techniques have several limitations that result in gaps, missing links, and incomplete understanding of the genome. Advances in these techniques are needed to fill in these missing pieces of the puzzle to better comprehend genetics and accelerate the discovery of the causes of various genetically linkeddiseases. From a technological standpoint, the accuracy of sequencing and coverage across the genome remain major issues, especially for GC-rich regions and long homopolymer stretches of DNA. Furthermore, the short read lengths generated by the majority of current platforms severely restrict our ability to accurately characterize large repeat regions, numerous indels, and structural variation, rendering large portions of the genome opaque or inaccurate. Fragmentation of the genome for sequencing continues to be a major source of disruption in the continuity of the correct genomic sequence [ 14 , 15 ].
Recent advances in CRISPR technology provide hope for the medical treatment of cancer and other fatal diseases. Despite significant advances in this field, a number of technical obstacles remain, including off-target activity, insufficient indel or low homology-directed repair (HDR) efficiency, in vivo delivery of the Cas system components, and immune responses. This requires a substantial amount of technological advancement or the creation of new, superior methods to combat severe diseases with minimal side effects [ 14 , 16 ].
Additional considerations
As high-throughput, automated methods commonly produce very large amounts of data, deeper interaction between wet-lab and dry-lab researchers is required, to facilitate the design of efficient assays [ 17 ] and allow effective analysis and interpretation of results. Interdisciplinary collaborations, between biologists, engineers and professionals in the health sciences, might lead to newer and better methods of addressing current and future needs.
Further collaborations between scientists from academia and industry (in addition to researchers from government agencies) [ 18 ] would help to facilitate the development of novel methods, and aid in promoting their implementation around the world. For many countries, the main barrier to the broad use of molecular methods is the high cost of equipment and reagents [ 19 ]. Strategies aimed at lowering costs would be helpful for multiple institutions around the globe. In terms of intellectual property, fair licensing to institutions in the Global South as well as the implementation of Open Innovation and Open Science policies would be appropriate [ 20 ].
Overview of the current collection
In this current Collection, we are calling for articles showcasing novel methods from molecular biology and genetics, written by scientists from around the world. It is our goal to compile a set of articles that will help to address the challenges faced by the fields of molecular biology and genetics and broaden our understanding of genetic disorders and potential treatment strategies. We invite researchers working on such methods to consider submitting to our collection.
Acknowledgements
DAF has been previously supported by research grants from Minciencias and Areandina. VC has been previously supported by research grants from NIH and VA.
Author contributions
DAF and VC wrote an initial draft of the manuscript. All authors read and approved the final manuscript.
Data availability
Declarations.
DAF is a Senior Editorial Board Member of BMC Research Notes. VC is a Guest Editorial Board Member of BMC Research Notes.
DAF is a medical doctor, Ph.D. in Biomedical Sciences and Professor and Research Leader at the School of Health and Sport Sciences, Fundación Universitaria del Área Andina (Bogotá, Colombia). He has worked with multiple methods of molecular biology and genetics and is an author of more than 100 articles in international journals, has been peer reviewer for more than 115 international scientific journals, in addition to being part of editorial boards of several international journals. VC is a Research Assistant Professor in the Department of Biochemistry and Molecular Genetics at the University of Illinois at Chicago. His expertise in Biochemistry, Molecular Biology, Genetics, Oncology, and Cancer Biology is extensive. He is an invited reviewer for more than fourteen international peer review journals and is the author of fourteen articles with high impact.
Publisher’s note
Springer Nature remains neutral with regard to jurisdictional claims in published maps and institutional affiliations.
Contributor Information
Diego A. Forero, Email: oc.ude.anidnaera@14orerofd .
Vaibhav Chand, Email: ude.ciu@50dnahcv .

- Cornell University Home
- College of Agriculture & Life Sciences Home
Molecular Biology and Genetics

Faculty and Research
Researching life at the molecular level.
Biophysicists seek to understand the fundamental processes of life by applying the methods of physics and chemistry to biological systems. Understanding these biological systems and their complex processes requires exquisitely detailed knowledge of molecular structures and molecular functions. To investigate life at this most basic level, biophysicists use some of the most powerful tools available-X ray crystallography, optical and laser spectroscopy, nuclear magnetic resonance (NMR) spectroscopy and advanced computational science.

Research Areas
Research area faculty.
Nozomi Ando , Associate Professor, Chemistry & Chemical Biology
Research Interests: new structural biology tools to study protein allostery and enzymes dynamics; x-ray techniques, cryo-electron microscopy, and bioinformatics
Barbara Baird , Horace White Professor, Chemistry & Chemical Biology
Research Interests: Molecular mechanisms of cell surface receptors, and participation of plasma membrane in signaling events
Peng Chen , Peter J. W. Debye Professor, Chemistry & Chemical Biology
Research Interests: Single-molecule super-resolution imaging, protein-DNA/protein interactions, membrane complex assembly, single-cell imaging, nanoscale (photo)(electro)catalysis, polymerization catalysis
Iwijn De Vlaminck , Assistant Professor, Biomedical Engineering
Research Interests: development of precision medicine technologies for microbiology and immunology and the application of these technologies in the monitoring of infectious diseases and immune-related complications
Matthew Paszek , Associate Professor, Chemical and Biomolecular Engineering
Research Interests: Mechano-transduction; membrane biophysics; super-resolution imaging; cancer biophysics
Lois Pollack , Professor, Applied and Engineering Physics
Research Interests: The Pollack lab develops and applies new tools to measure the structural dynamics of macromolecules and their complexes
Michelle Wang , James Gilbert White Distinguished Professor of Physical Sciences and Howard Hughes Medical Institute Investigator, Physics
Research Interests: Mechanisms of motor protein collision and navigation; chromatin dynamics and remodeling; torsional mechanics of replication and transcription; development of innovative single-molecule mechanical manipulation techniques
Mingming Wu , Associate Professor, Biological and Environmental Engineering
Research Interests: Single cell analysis, cell mechanics, microfluidics and optical imaging
Chris Xu , IBM Chair, Professor of Engineering, Applied and Engineering Physics
Research Interests: Biomedical imaging, fiber optics, optical instrumentation
Warren Zipfel , Associate Professor, Biomedical Engineering
Research Interests: optical imaging, bioanalytical instrumentation and single molecule methodologies that enhance biophysical and biomedical research; cancer biology and transcriptional regulation
Richard Cerione , Goldwin Smith Professor, Chemistry & Chemical Biology,Molecular Medicine
Research Interests: Biophysics of cellular signal transduction; X-ray and cryo-EM structures of complex signaling systems
Brooks Crickard, Assistant Professor, Molecular Biology & Genetics
Research Interests: mechanisms of chromosome maintenance and genomic stability; biophysical approaches with special emphasis on single molecule fluorescence microscopy
Laura Gunn , Assistant Professor, Integrative Plant Sciences
Research Interests: sequence-structure-function of nature’s carbon-fixing enzyme, Rubisco, using structural biology, synthetic biology and protein engineering approaches
Toshi Kawate , Associate Professor, Molecular Medicine
Research Interests: Molecular mechanisms of extracellular signaling; membrane receptors, channels, and enzymes; X-ray crystallography; Cryo-EM; electrophysiology; functional reconstitution of integral membrane proteins
Ailong Ke , Associate Professor, Molecular Biology & Genetics
Research Interests: (1) Mechanistic dissection of RNA-guided processes, such as CRISPR-Cas; (2) Genome engineering using RNA-guided enzymes; (3) Gene regulation by non-coding RNAs
Linda Nicholson , Professor, Molecular Biology & Genetics
Research Interests: structural and dynamics studies of proteins
Research Interests: develop and apply new tools to measure the structural dynamics of macromolecules and their complexes
Erik Thiede , Assistant Professor, Chemistry & Chemical Biology
Research Interests: AI with physical simulation to understand protein conformational change; Integrating Cryo-EM with Molecular Simulation; AI-accelerated allosteric drug discovery; New Markov State Modelling algorithms
Robert Thorne , Professor, Physics
Research Interests: new methods for probing protein structure and dynamics using X-rays; variable temperature crystallography; cryogenic temperature small angle X-ray scattering; radiation damage by X-rays; ice and glass formation in nanoconfined and biological systems
Haiyuan Yu , Associate Professor, Biological Statistics & Computational Biology
Research Interests: Quantitative biomedical systems biology; functional and comparative genomics/proteomics; biological networks and their changes across human populations and in disease
Itai Cohen , Professor, Physics
Research Interests: Origami, origami mechanics, origami metamaterials, origami robotics, complex fluids, colloids, rheology, colloid rheology, shear thickening, tunable shear thickening, shear thinning, confocal rheoscope, SALSA, suspension stresses, cartilage mechanics
Research Interests: development of precision medicine technologies for microbiology and immunology; applications in the monitoring of infectious diseases and immune-related complications
Eric Dufrasne , Professor
Research Interests: Physics of biological materials, including biomolecular condensates, cytoskeletal filaments and networks, lipid membranes, and extra-cellular matrix
James Sethna , Professor, Physics
Research Interests: Statistical mechanics; condensed-matter physics; biophysics; systems biology; materials science
Z. Jane Wang , Professor, Mechanical & Aerospace Engineering, Physics
Research Interests: Physics of living organisms; insect flight; fluid dynamics; computational and applied mathematics
Research Interests: Origami, origami mechanics, origami metamaterials, origami robotics, complex fluids, colloids, rheology, colloid rheology, shear thickening, tunable shear thickening, shear thinning, confocal rheoscope, SALSA, suspension stresses, cartilage mechanics
Research Interests: Mechano-transduction; membrane biophysics; super-resolution imaging; cancer biophysics
Barbara Baird, Horace White Professor, Chemistry & Chemical Biology
Research Interests: Molecular mechanisms of cell surface receptors, and participation of plasma membrane in signaling events
Richard Cerione , Goldwin Smith Professor, Chemistry & Chemical Biology, Molecular Medicine
Research Interests: Single-molecule super-resolution imaging, protein-DNA/protein interactions, membrane complex assembly, single-cell imaging, nanoscale (photo)(electro)catalysis, polymerization catalysis
Roger Loring , Professor, Chemistry & Chemical Biology
Research Interests: Nonequilibrium statistical mechanics; semiclassical approximations to quantum dynamics; nonlinear infrared spectroscopy of biomolecules
Michelle Wang, James Gilbert White Distinguished Professor of Physical Sciences and Howard Hughes Medical Institute Investigator, Physics
Research Interests: optical imaging, bioanalytical instrumentation and single molecule methodologies; cancer biology and transcriptional regulation
Research Interests: Biophysics of cellular signal transduction; X-ray and cryo-EM structures of complex signaling system
Research Interests: Single-molecule super-resolution imaging, protein-DNA/protein interactions, membrane complex assembly, single-cell imaging, nanoscale (photo)(electro)catalysis, polymerization catalysis
Research Interests: Nonequilibrium statistical mechanics; semiclassical approximations to quantum dynamics; nonlinear infrared spectroscopy of biomolecules
Research Interests: AI with physical simulation to understand protein conformational change; Integrating Cryo-EM with Molecular Simulation; AI-accelerated allosteric drug discovery; New Markov State Modelling algorithms
Research shows our bodies go through rapid changes in our 40s and our 60s
For many people, reaching their mid-40s may bring unpleasant signs the body isn’t working as well as it once did. Injuries seem to happen more frequently. Muscles may feel weaker.
A new study, published Wednesday in Nature Aging , shows what may be causing the physical decline. Researchers have found that molecules and microorganisms both inside and outside our bodies are going through dramatic changes, first at about age 44 and then again when we hit 60. Those alterations may be causing significant differences in cardiovascular health and immune function.
The findings come from Stanford scientists who analyzed blood and other biological samples of 108 volunteers ages 25 to 75, who continued to donate samples for several years.
“While it’s obvious that you’re aging throughout your entire life, there are two big periods where things really shift,” said the study’s senior author, Michael Snyder, a professor of genetics and director of the Center for Genomics and Personalized Medicine at Stanford Medicine. For example, “there’s a big shift in the metabolism of lipids when people are in their 40s and in the metabolism of carbohydrates when people are in their 60s.”
Lipids are fatty substances, including LDL, HDL and triglycerides, that perform a host of functions in the body, but they can be harmful if they build up in the blood.
The scientists tracked many kinds of molecules in the samples, including RNA and proteins, as well as the participants’ microbiomes.
The metabolic changes the researchers discovered indicate not that people in their 40s are burning calories more slowly but rather that the body is breaking food down differently. The scientists aren’t sure exactly what impact those changes have on health.
Previous research showed that resting energy use, or metabolic rate , didn’t change from ages 20 to 60. The new study’s findings don't contradict that.
The changes in metabolism affect how the body reacts to alcohol or caffeine, although the health consequences aren’t yet clear. In the case of caffeine, it may result in higher sensitivity.
It’s also not known yet whether the shifts could be linked to lifestyle or behavioral factors. For example, the changes in alcohol metabolism might be because people are drinking more in their mid-40s, Snyder said.
For now, Snyder suggests people in their 40s keep a close eye on their lipids, especially LDL cholesterol.
“If they start going up, people might want to think about taking statins if that’s what their doctor recommends,” he said. Moreover, “knowing there’s a shift in the molecules that affect muscles and skin, you might want to warm up more before exercising so you don’t hurt yourself.”
Until we know better what those changes mean, the best way to deal with them would be to eat healthy foods and to exercise regularly, Snyder said.Dr. Josef Coresh, founding director of the Optimal Aging Institute at the NYU Grossman School of Medicine, compared the new findings to the invention of the microscope.
“The beauty of this type of paper is the level of detail we can see in molecular changes,” said Coresh, a professor of medicine at the school. “But it will take time to sort out what individual changes mean and how we can tailor medications to those changes. We do know that the origins of many diseases happen in midlife when people are in their 40s, though the disease may occur decades later.”
The new study “is an important step forward,” said Dr. Lori Zeltser, a professor of pathology and cell biology at the Columbia University Vagelos College of Physicians and Surgeons. While we don’t know what the consequences of those metabolic changes are yet, “right now, we have to acknowledge that we metabolize food differently in our 40s, and that is something really new.”
The shifts the researchers found might help explain numerous age-related health changes, such as muscle loss, because “your body is breaking down food differently,” Zeltser said.
Linda Carroll is a regular health contributor to NBC News. She is coauthor of "The Concussion Crisis: Anatomy of a Silent Epidemic" and "Out of the Clouds: The Unlikely Horseman and the Unwanted Colt Who Conquered the Sport of Kings."
Thank you for visiting nature.com. You are using a browser version with limited support for CSS. To obtain the best experience, we recommend you use a more up to date browser (or turn off compatibility mode in Internet Explorer). In the meantime, to ensure continued support, we are displaying the site without styles and JavaScript.
- View all journals
- Explore content
- About the journal
- Publish with us
- Sign up for alerts
- Open access
- Published: 19 August 2024
Comprehensive molecular profiling of multiple myeloma identifies refined copy number and expression subtypes
- Sheri Skerget 1 ,
- Daniel Penaherrera 1 ,
- Ajai Chari ORCID: orcid.org/0000-0002-0405-7480 2 ,
- Sundar Jagannath ORCID: orcid.org/0000-0003-2934-6518 2 ,
- David S. Siegel ORCID: orcid.org/0000-0002-3604-2574 3 ,
- Ravi Vij 4 ,
- Gregory Orloff 5 ,
- Andrzej Jakubowiak 6 ,
- Ruben Niesvizky 7 ,
- Darla Liles ORCID: orcid.org/0000-0003-1480-7887 8 ,
- Jesus Berdeja ORCID: orcid.org/0000-0003-4362-0376 9 ,
- Moshe Levy 10 ,
- Jeffrey Wolf 11 ,
- Saad Z. Usmani ORCID: orcid.org/0000-0002-5484-8731 12 ,
- The MMRF CoMMpass Network ,
- Austin W. Christofferson 1 ,
- Sara Nasser 1 ,
- Jessica L. Aldrich 1 ,
- Christophe Legendre 1 ,
- Brooks Benard ORCID: orcid.org/0000-0001-7154-744X 1 ,
- Chase Miller 1 ,
- Bryce Turner 1 ,
- Ahmet Kurdoglu 1 ,
- Megan Washington 1 ,
- Venkata Yellapantula ORCID: orcid.org/0000-0003-1592-7741 1 ,
- Jonathan R. Adkins 1 ,
- Lori Cuyugan 1 ,
- Martin Boateng 1 ,
- Adrienne Helland 1 ,
- Shari Kyman ORCID: orcid.org/0000-0001-5386-5866 1 ,
- Jackie McDonald 1 ,
- Rebecca Reiman 1 ,
- Kristi Stephenson 1 ,
- Erica Tassone 1 ,
- Alex Blanski 13 ,
- Brianne Livermore 13 ,
- Meghan Kirchhoff 13 ,
- Daniel C. Rohrer ORCID: orcid.org/0000-0002-1784-9196 13 ,
- Mattia D’Agostino 14 ,
- Manuela Gamella 14 ,
- Kimberly Collison 15 ,
- Jennifer Stumph ORCID: orcid.org/0000-0003-2423-0241 15 ,
- Pam Kidd 15 ,
- Andrea Donnelly 16 ,
- Barbara Zaugg 16 ,
- Maureen Toone 17 ,
- Kyle McBride 17 ,
- Mary DeRome 18 ,
- Jennifer Rogers 18 ,
- David Craig 1 ,
- Winnie S. Liang ORCID: orcid.org/0000-0002-5698-7735 1 ,
- Norma C. Gutierrez ORCID: orcid.org/0000-0001-5834-9510 19 ,
- Scott D. Jewell ORCID: orcid.org/0000-0001-9469-6261 13 ,
- John Carpten 1 ,
- Kenneth C. Anderson ORCID: orcid.org/0000-0002-6418-0886 20 ,
- Hearn Jay Cho 2 , 18 ,
- Daniel Auclair ORCID: orcid.org/0000-0001-5151-3058 18 ,
- Sagar Lonial 21 &
- Jonathan J. Keats ORCID: orcid.org/0000-0003-4375-7399 1
Nature Genetics ( 2024 ) Cite this article
1 Altmetric
Metrics details
- DNA sequencing
- RNA sequencing
Multiple myeloma is a treatable, but currently incurable, hematological malignancy of plasma cells characterized by diverse and complex tumor genetics for which precision medicine approaches to treatment are lacking. The Multiple Myeloma Research Foundation’s Relating Clinical Outcomes in Multiple Myeloma to Personal Assessment of Genetic Profile study ( NCT01454297 ) is a longitudinal, observational clinical study of newly diagnosed patients with multiple myeloma ( n = 1,143) where tumor samples are characterized using whole-genome sequencing, whole-exome sequencing and RNA sequencing at diagnosis and progression, and clinical data are collected every 3 months. Analyses of the baseline cohort identified genes that are the target of recurrent gain-of-function and loss-of-function events. Consensus clustering identified 8 and 12 unique copy number and expression subtypes of myeloma, respectively, identifying high-risk genetic subtypes and elucidating many of the molecular underpinnings of these unique biological groups. Analysis of serial samples showed that 25.5% of patients transition to a high-risk expression subtype at progression. We observed robust expression of immunotherapy targets in this subtype, suggesting a potential therapeutic option.
Similar content being viewed by others
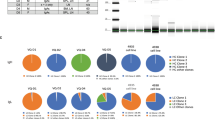
Molecular characterization stratifies VQ myeloma cells into two clusters with distinct risk signatures and drug responses
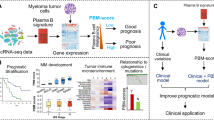
Enhancing prognostic power in multiple myeloma using a plasma cell signature derived from single-cell RNA sequencing
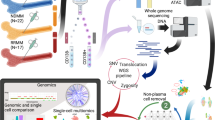
1q amplification and PHF19 expressing high-risk cells are associated with relapsed/refractory multiple myeloma
Multiple myeloma is a treatable, but currently incurable, hematological malignancy of plasma cells (PCs). The incorporation of new treatment modalities over the last two decades has vastly improved outcomes of patients with myeloma; however, patients still relapse, and some have poor outcomes. Despite substantial efforts to understand the molecular basis of the disease, predicting patient outcomes and identifying high-risk patients remains a challenge.
Multiple myeloma is a genetically heterogeneous disease with two broad karyotypic groups. A hyperdiploid (HRD) phenotype, with characteristic trisomies of chromosomes (chr) 3, 5, 7, 9, 11, 15, 19 and 21, is present in 50–60% of tumors 1 , 2 , 3 . The remaining non-HRD (NHRD) tumors typically have an immunoglobulin translocation dysregulating NSD2 / WHSC1 / MMSET , MYC , CCND1 or MAF transcription factors 4 , 5 , 6 , 7 , 8 , 9 . Tumors harbor many other genetic aberrations, including nonimmunoglobulin structural abnormalities and mutations 10 , 11 , 12 , 13 . Although previous genomic studies were instrumental in deconvoluting the genetic heterogeneity of myeloma, they are mostly limited by small cohort sizes, the number and types of assays performed, a lack of longitudinal sampling, clinical follow-up and biased inclusion of heavily pretreated patients, limiting our comprehensive understanding of the disease.
To understand the impact of tumor genetic profile on patient outcomes and treatment response, the Multiple Myeloma Research Foundation (MMRF) sponsored the Relating Clinical Outcomes in Multiple Myeloma to Personal Assessment of Genetic Profile (CoMMpass) study ( NCT01454297 ). CoMMpass was a prospective, longitudinal, observational clinical study that accrued 1,143 newly diagnosed, previously untreated patients with multiple myeloma from sites throughout the United States, Canada, Spain and Italy between 2011 and 2016. Tumor samples collected at diagnosis and each progression event were profiled using whole-genome sequencing (WGS), whole-exome sequencing (WES) and RNA sequencing (RNA-seq). Clinical parameters were collected every 3 months through the 8-year observation period.
We present a molecular analysis of the complete baseline cohort, with a median follow-up of 4 years, identifying recurrent loss-of-function (LOF) and gain-of-function (GOF) events and distinct copy number and gene expression subtypes of myeloma. The comprehensive nature of this dataset and our integrated analysis framework define both the overall frequency of gene alterations in myeloma and the genetic basis of a high-risk patient population that does not benefit from current therapies.
Cohort description
The demographic and clinical parameters of the cohort follow expected distributions with a median diagnostic age of 63 years (range = 27–93 years), the expected over-representation of males (60.4%) and an international staging system (ISS) distribution of 35.1% ISSI, 35.1% ISSII and 27.2% ISSIII 14 (Table 1 and Supplementary Table 1 ). This cohort is primarily from the United States, and, unlike most clinical trials, the distribution of self-reported ancestry reflects US Census Bureau statistics with 80.6% Caucasian, 17.5% Black and 1.9% Asian.
Each patient’s cytogenetic phenotype was defined by WGS with 57.2% HRD and 42.8% NHRD, 24.3% del(1p22), 35.2% gain(1q21), 52.0% del(13q14) and 12.5% del(17p13). Translocations involving common target genes from any of the three immunoglobulin loci occurred at the following frequencies: 20.0% CCND1 , 1.2% CCND2 , 1.8% CCND3 , 4.0% MAF , 0.7% MAFA , 1.3% MAFB , 14.3% MYC and 12.8% WHSC1 . Of these events, 83.0% involved the IgH locus, while 5.3% and 11.7% involved the κ and λ loci, respectively.
Irrespective of treatment, the cohort median time to second-line therapy was 38.1 months, and the median overall survival (OS) was 103.6 months (Extended Data Fig. 1a,b ). The median OS for ISSIII patients was 53.9 months, while the median OS for ISSI and ISSII patients could not be confidently predicted (Extended Data Fig. 1c,d ). Patients with at least one high-risk cytogenetic feature had worse OS outcomes, even with uniform usage of new agents (Supplementary Fig. 1 ) 15 .
Integrated analysis for gain and LOF genes
To comprehensively identify LOF and GOF events in patients with myeloma, an integrated model was developed to overcome the limitations of analyzing any one data type by combining measurements from WES, WGS and RNA-seq to assign a functional state to each gene (Supplementary Tables 2 – 6 ). In the LOF model, a single event in a gene was designated as partial LOF, whereas genes with two or more events were designated as complete LOF. At diagnosis, 592 patients had all three sequencing assays performed and were included in the analysis (Supplementary Fig. 2 ). We identified 70 genes where a complete LOF event was identified in ≥5 patients (Fig. 1a ). Complete LOF was observed in 12 genes in >2% of the cohort, including TRAF3 (10.1%), DIS3 (6.9%), TENT5C / FAM46C (5.1%), CYLD (4.7%), TP53 (4.1%), MAX (3.5%), RB1 (3.2%), WWOX (3.2%), HUWE1 (2.7%), PVT1 (2.5%), CDC42BPB (2.0%) and MAGEC1 (2.0%). However, CDC42BPB is in a contiguous gene region on chr14 with TRAF3 , which was previously shown to be the target of bi-allelic loss in this region 16 .
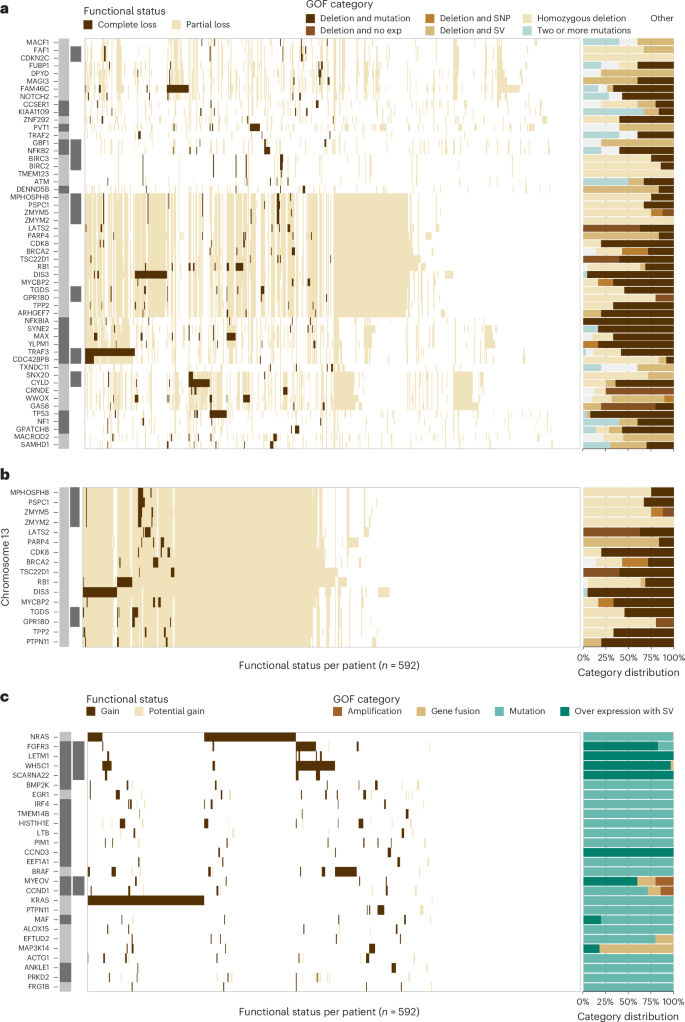
The location and proximity of individual genes are shown next to each gene with the alternating gray and black bars illustrating when the chromosomal location changes, while black bars directly to the right denote contiguous genes. Each tick along the x axis represents a patient with the corresponding event. a , Complete LOF was observed in 53 autosomally located genes. b , Genes on chr13q that were the target of complete LOF events in at least five patients in the baseline cohort. c , GOF events were detected in 27 autosomal genes due to amplification, gene fusions, mutations or over expression associated with a structural variant (SV).
The target gene(s) of chr13 loss continues to be controversial. WGS data detected 13q14 deletion in 52.0% of patients, while LOF analysis identified that 26.5% of patients had complete LOF of one or more genes on chr13. The commonly assumed target, RB1 , showed complete LOF in 3.2% of patients, while DIS3 complete LOF was detected in 6.9% of patients; however, a striking number of additional genes were independently knocked out in myeloma (Fig. 1b ). The following two contiguous gene regions with complete LOF were identified: the first comprising MPHOSPH8 (1.4%), PSPC1 (1.5%), ZMYM5 (1.4%), ZMYM2 (1.0%), and the second comprising TGDS (1.9%) and GPR180 (0.8%) where the minimal region of deletion and LOF frequency suggest that the targets are PSPC1 and TGDS , respectively. Additional complete LOF events were identified targeting LATS2 (1.4%), BRCA2 (1.2%), PARP4 (1.0%), MYCBP2 (1.0%), TPP2 (1%), CDK8 (0.8%), TSC22D1 (0.8%) and ARHGEF7 (0.8%). These results highlight that monosomy 13 is associated with multiple independent gene inactivation events.
The GOF analysis identified an event in 92% of patients at diagnosis and 27 genes where a GOF event was identified in five or more patients (Fig. 1c ). There were seven genes in which a GOF event was identified in greater than 2% of the cohort, including KRAS (23.6%), NRAS (21.6%), WHSC1 (10.3%), BRAF (7.1%), FGFR3 (4.9%), HIST1H1E (3.2%) and EGR1 (2.5%).
Identification of copy number subtypes of multiple myeloma
To discover potential underlying phenotypes of myeloma beyond the known dichotomy of HRD and NHRD karyotypes, unsupervised consensus clustering was performed on copy number data from 871 patients (Extended Data Fig. 2 ). Three independent trials were highly consistent and identified eight subtypes as the optimal solution (Supplementary Figs. 3 and 4 ).
The copy number subtypes consisted of five HRD and three NHRD subtypes and were annotated based on defining features (Fig. 2a ). The HRD classic subtype had gains of classic HRD chromosomes, and the remaining HRD subtypes were annotated based on deviations from this phenotype. The subtype designated as HRD, ++15 exhibited tetrasomy of chr15 (Supplementary Fig. 5 ), while two subtypes (HRD, diploid 7 and HRD, diploid 3, 7) were defined by the absence of chr7 and chr3 trisomies. Finally, the complex HRD, +1q, diploid 11, −13 subtype lacked chr11 trisomy but harbored gain of chr1q and loss of chr13. Of the NHRD subtypes, the diploid subtype was mostly devoid of copy number events and highly associated with translocations targeting a D-type cyclin (71.3%). The remaining two NHRD groups were strongly associated with canonical immunoglobulin translocations (71.1%) and were defined by chr13 loss. The −13 subtype contained a subpopulation of patients with chr14 loss, while the +1q, −13 subtype had gains of 1q.
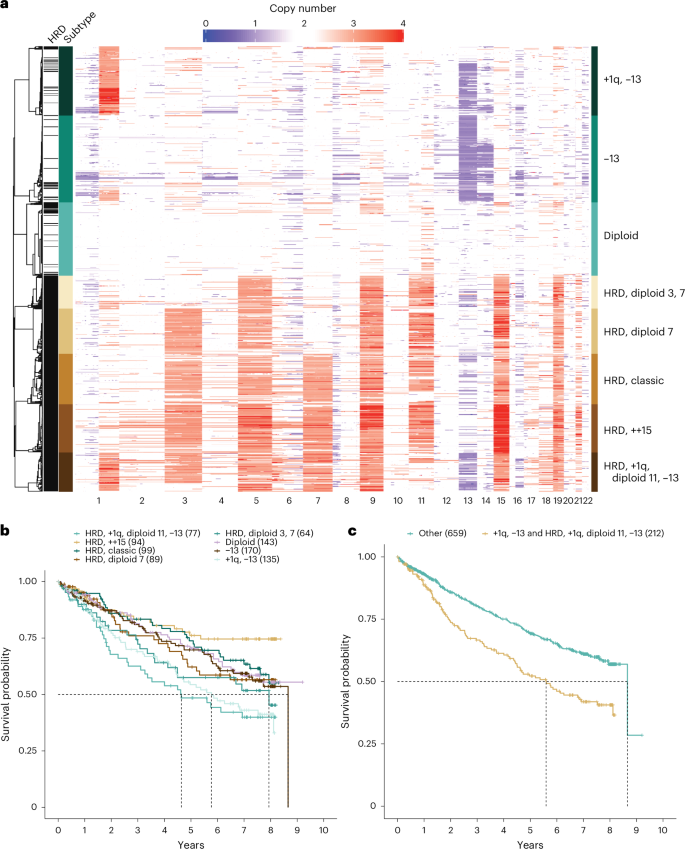
a , Consensus clustering of WGS copy number data identified eight unique copy number subtypes, comprising five HRD and three NHRD clusters that were annotated based on common copy number features. b , Pointwise OS estimates are shown by the respective lines for each copy number subtype. Median OS was met for the HRD, +1q, diploid 11, −13 (55.7 months, 95% CI = 31.7–NA (not available)), +1q, −13 (69.3 months, 95% CI = 53.0–97.4), HRD, diploid 3, 7 (95.3 months, 95% CI = 43.6–NA) and −13 (103.9 months, 95% CI = 79.2–NA) subtypes. Pairwise outcome comparisons identified eight significantly different subtypes by the log-rank test after multiple testing corrections using the Benjamini–Hochberg method. The significant differences were between the HRD, +1q, diploid 11, −13 subtype and HRD, ++15 ( P = 0.0015), HRD, classic ( P = 0.0373), diploid ( P = 0.0373) or −13 ( P = 0.0373); between the +1q, −13 subtype and HRD, ++15 ( P = 0.0015), HRD, classic ( P = 0.0373) or diploid ( P = 0.0448); and between HRD, ++15 and HRD, diploid 3, 7 ( P = 0.03733). c , Pointwise OS estimates are shown by the respective lines, and pairwise outcomes were compared by the log-rank test, which showed a significant difference ( P = 9.5 × 10 −6 ) between patients in the +1q, −13 and HRD, +1q, diploid 11, −13 groups (median = 67.2 months, 95% CI = 53.0–83.2) versus patients in other copy number subtypes (median = 103.9, 95% CI = 103.9–NA).
There was no difference in outcomes between HRD and NHRD patients (Supplementary Fig. 6 ). However, the HRD and NHRD subtypes with both 1q gain and chr13 loss had inferior OS outcomes when compared to patients in other copy number subtypes (Fig. 2b ), suggesting that HRD patients should not be universally considered as a group with good outcomes. Combining these two subtypes identified a group with inferior outcomes as compared to patients with other genetic backgrounds (Fig. 2c ; hazard ratio (HR) = 1.732, 95% confidence interval (CI) = 1.354–2.215, P < 0.001). The 35-month difference in OS outcomes between NHRD patients in the +1q, −13 subtype and the −13 subtype suggested 1q gain, rather than 13q loss, is the predictor of poor outcome; however, a Cox proportional hazard model examining the contribution of 13q14 and 1q21 copy numbers on OS outcomes did not identify gain(1q21) as an independent feature (Extended Data Fig. 3 ).
RNA subtypes of multiple myeloma
Consensus clustering was performed on RNA-seq results from 714 baseline samples to identify subtypes of myeloma defined by gene expression (Extended Data Fig. 4 ). Three independent replicates identified 12 clusters as the optimum solution; two were identical, and a third had 20 (2.8%) patients assigned to different classes (Supplementary Figs. 7 and 8 ). Many of the observed subtypes were associated with known immunoglobulin translocations and copy number states (Fig. 3a and Extended Data Fig. 5 ), and there were clear relationships with subtypes identified in previous studies (Supplementary Figs. 9 and 10 ) 17 , 18 . Four subtypes were identified across all studies, including MMSET expressing (MS) (characterized by t(4;14) patients), MAF family transcription factor expressing (MAF) (primarily characterized by t(14;16) patients), cyclin D expressing group 1 (CD1) (characterized by t(11;14) patients) and proliferation (PR) (characterized by patients with a high proliferation index). To maintain consistency across studies, we used subtype names from previous studies when appropriate but otherwise assigned names based on common molecular features.
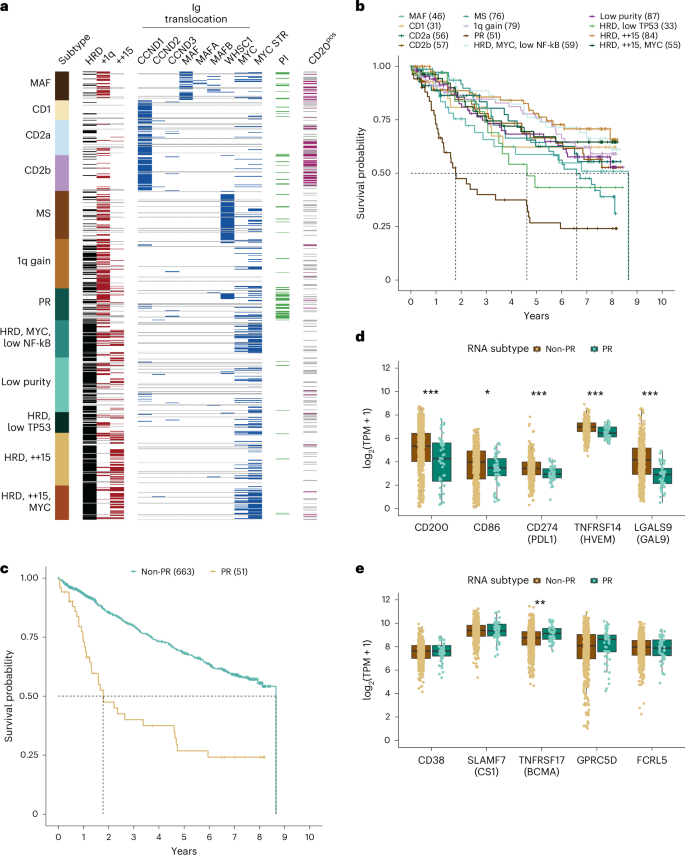
a , Consensus clustering of RNA-seq data revealed 12 RNA subtypes of multiple myeloma. The MYC STR flag indicates the detection of a MYC translocation (Ig or non-Ig) or intrachromosomal deletion centromeric of MYC. b , Pointwise OS estimates are shown by the respective lines for each RNA subtype. Median OS was reached for the PR (21.3 months, 95% CI = 15.0–55.3); HRD, low TP53 (55.4 months, 95% CI = 36.7–NA); MS (79.2 months, 95% CI = 57.3–97.4) and MAF (103.9 months, 95% CI = 34.4–NA) subtypes. Pairwise outcome comparisons identified ten significantly different subtypes by the log-rank test after multiple testing corrections using the Benjamini–Hochberg method. All significantly different pairs were compared against the PR subtype, with P values ranging from 0.03305 compared to MAF and 1.3 × 10 −6 compared to HRD, ++15. The only PR pairwise comparison that was not significant was against HRD, low TP53, the subtype with the second lowest median OS. c , Pointwise OS estimates of patients in the PR (21.3 months, 95% CI = 15.0–55.3) versus non-PR (median = 103.9 months, 95% CI = 97.4–NA) subtype at diagnosis ( P = 1.1 × 10 −10 ), HR = 3.16 (95% CI = 2.19–4.57). d , e , Expressed (median transcripts per million (TPM) > 1 in at least one group) checkpoint inhibitor ( d ) and immunotherapy ( e ) targets in independent non-PR ( n = 663) versus PR ( n = 51) patients. Significant differences in median expression between the two groups were determined using a two-sided unpaired Wilcoxon rank sum test and are indicated when significant (* P < 0.05, ** P < 0.01 and *** P < 0.001).
The MS subtype comprised 10.6% of patients for whom a t(4;14)- WHSC1 was detected in 62 of 67 (92.5%) samples by WGS. One patient had a t(2;4) bringing the κ enhancer close to WHSC1 , while two others had fusion transcripts between WHSC1 and the highly expressed genes FUT8 or CXCR4 . The MAF subtype included 6.4% of patients, of whom 38 of 41 (92.7%) patients had translocations (27 t(14;16)- MAF , 4 t(8;14)- MAFA , 6 t(14;20)- MAF B and 1 t(20;22)- MAFB ). All three patients with undetectable immunoglobulin translocations had high expression of an MAF family gene. One had a t(1;16) juxtaposing the FAM46C super-enhancer with MAF 19 , 20 . Another had an atypical insertion of a class-switch circle telomeric of MAF . One patient had both a t(14;16)-MAF and a t(4;14)-WHSC1 yet strongly associated with the MAF subtype, suggesting the MAF expression signature overpowers the MS signature. Immunoglobulin translocations targeting MAF family transcription factors are associated with higher mutation load 21 , and in this cohort, 8 of 10 patients with high tumor mutation burden (>10 mutations per Mb) were in the MAF subtype and could qualify to receive a checkpoint inhibitor.
Three subtypes were highly associated with overexpression of a D-type cyclin caused by t(11;14)- CCND1 , t(12;14)- CCND2 , or t(6;14)- CCND3 (Supplementary Fig. 11a–c ). The CD1 subtype included 4.3% of patients, of whom 24 of 25 (96%) patients had a D-type cyclin targeting translocation. One patient had a t(9;14), resulting in the overexpression of the B-cell master regulator PAX5 (Supplementary Fig. 11d ). Unlike previous studies that identified a single cyclin D expressing group 2 (CD2) subtype, we identified two related subtypes designated as CD2a and CD2b. The CD2a subtype comprised 7.8% of patients, of whom 40 of 47 (85.1%) patients had a detected D-type cyclin IgH translocation. The CD2b subtype included 8.0% of patients, of whom 51 of 56 (91.1%) patients had a detected D-type cyclin targeting translocation. Both the CD2a and CD2b subtypes were associated with cell surface expression of CD20, which is largely absent in other RNA subtypes, including CD1. Compared to CD2a, patients in the CD2b subtype had higher proliferative index scores ( P < 0.005), but there is not a significant difference in OS or time to second-line therapy between these groups, and unexpectedly, CD2a patients start second-line therapy 8.4 months earlier.
The PR subtype contained 7.1% of patients with an admixture of classic genetic subtypes and very poor clinical outcomes, with a median OS of 21 months (Fig. 3b,c ). High proliferation index scores were also concentrated in this subtype (Extended Data Fig. 6 ). Clearly, current treatment regimens are ineffective for these patients. We compared the expression of current checkpoint and immunotherapy targets in non-PR versus PR patients and observed that all five checkpoint targets ( CD200 , CD86 , CD274 / PDL1 , TNFRSF14 / HVEM and LGALS9 / GAL9 ) were decreased in the PR group, whereas TNFRSF17 / BCMA was increased, and the other four immunotherapy targets ( CD38 , SLAMF7 / CS1 , GPRC5D and FCRL5 / FCRH5 ) showed no difference in expression in PR patients (Fig. 3d,e ).
A subtype representing 11.1% of patients most closely resembled the previously defined low bone subtype 17 (Supplementary Fig. 9 ); however, there was no decrease in bone lesions (Supplementary Fig. 12 ). This subtype comprised an admixture of 59.2% HRD and 40.8% NHRD patients, but 74.0% had a gain of chr1q with 26.0% having ≥4 copies and was thus termed the 1q gain subtype.
Four of the RNA subtypes were associated with HRD (Extended Data Fig. 5 ) and either did not uniquely associate with a subtype from a previous study, or the original name could not be justified. Two HRD subtypes associated closely with the previously identified HY subtype, but differed due to an enrichment of tetrasomy 15, observed in 58.7% and 60.8% of patients. Because structural events involving MYC are associated with HRD karyotypes 22 , we investigated the association between these two groups and MYC rearrangements. We identified 37 of 49 (75.5%) versus 23 of 76 (30.3%) patients who had MYC rearrangements, and thus these subtypes were named HRD, ++15, MYC and HRD, ++15, respectively. A third HRD subtype comprising 8.3% of patients most closely associated with the PRL3 subtype 18 ; however, the signature was elevated in four subtypes (Supplementary Fig. 10 ). A MYC structural event was identified in 35 of 49 (71.4%) of these patients, and this group was also distinguished from all others except PR in having a low nuclear factor kappa-light-chain-enhancer of activated B cells (NF-kB) index (Extended Data Fig. 7 ) and was thus named HRD MYC, low NF-kB. The smallest HRD group contained 4.6% of patients and was associated with the previously defined NF-kB subtype 18 ; however, no clear association existed with the NF-kB index used to define the subtype (Supplementary Fig. 10 and Extended Data Fig. 7 ). One of the predictors of this RNA subtype was overexpression of NINJ1 (Supplementary Table 7 and Supplementary Fig. 13a ), which inhibits translation of TP53 (ref. 23 ). TP53 was also found to be underexpressed, exhibiting the lowest median expression in this subtype as compared to all other RNA subtypes (Supplementary Fig. 13b ). Taken together, this subtype was termed HRD, low TP53. The final subtype, termed low purity, with 12.2% of patients correlated with the previously defined myeloid group but was dominated by lower purity samples (Supplementary Fig. 10 and Extended Data Fig. 8 ) 18 .
Clinical and molecular associations with RNA subtypes
To identify additional defining features of each RNA subtype, we tested for significant associations between clinical and molecular features, including complete LOF and GOF events. Overall, 21 genes with complete LOF or GOF were identified to have a significant association with one or more RNA subtypes (Fig. 4 ). As expected, GOF was detected in the translocation target genes associated with the MAF, MS, and CD subtypes. Although the loss of one WWOX allele is expected in t(14;16), we frequently detected complete LOF of WWOX ( P < 0.001), supporting a possible role of WWOX in myeloma. Both the MS and 1q gain subtypes were diminished for NRAS GOF, and the latter was enriched for TRAF3 LOF. The CD2a subtype was enriched for GOF events in NRAS ( P < 0.005) and IRF4 ( P < 0.005), while the CD2b subtype was enriched for GOF events in IRF4 ( P < 0.005) and EFTUD2 ( P < 0.01), representing potential subtype-specific therapeutic targets. In general, the HRD RNA subtypes were not enriched for any GOF or LOF events aside from the HRD, ++15, MYC subtype, which was enriched for LOF events in FAM46C ( P < 0.001).
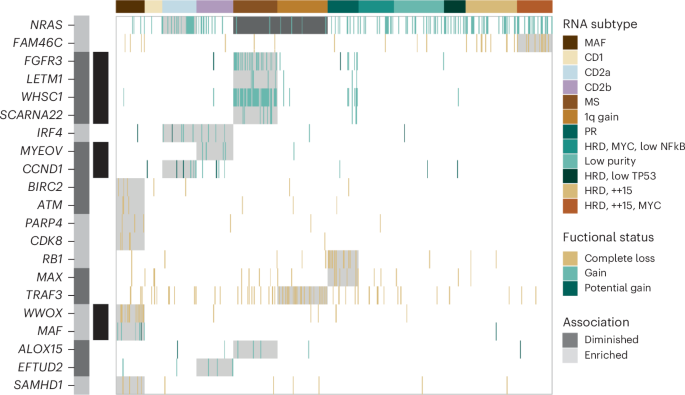
The location and proximity of individual genes are shown next to each gene with the alternating gray and black bars illustrating when the chromosomal location changes, while black bars directly to the right denote contiguous genes. LOF and GOF features that are significantly enriched or depleted within a specific RNA subtype are highlighted. Enrichment analysis was conducted using a two-tailed Fishers test, and the Benjamini–Hochberg test correction was used for multiple testing to compute a positive false discovery rate (pFDR) value. Genes are only included when pFDR ≤ 0.1, P < 0.05.
The PR subtype was enriched for LOF of RB1 ( P < 0.001) and MAX ( P < 0.01), gain(1q21) ( P < 0.001), del(13q14) ( P < 0.001) and ISSIII patients ( P < 0.001). Interestingly, 50% of PR patients were ISSIII, while 22% and 28% of PR patients were ISSI and ISSII, respectively, highlighting that ISS underestimates disease severity in half of these high-risk patients. Complete loss of RB1 typically involves a one-copy deletion of 13q coupled with a second molecular event (Supplementary Fig. 14 ). Identifying LOF of RB1 and MAX represents defining genetic features of the high-risk PR phenotype.
Association of G1/S checkpoint with transition to PR subtype
We developed a predictive model to assign the serial samples to the subtypes with the highest class probability (Supplementary Table 7 ). Overall, 71 patients were assigned a subtype at two or more time points, with 55 patients assigned a subtype other than low purity for at least two time points. At diagnosis, five serial patients were classified as low purity; however, at progression, they all had a subtype other than low purity (one each CD1; 1q gain; PR; HRD, ++15 and HRD, ++15, MYC), further supporting that this phenotype is driven by relative sample purity rather than distinct disease biology (Extended Data Fig. 9 ). Although most patients remained in the same subtype throughout their disease course, 13 of 49 (26.5%) patients who were not classified as low purity or PR at baseline, transitioned into the PR subtype at progression (Fig. 5a ). Patients who transitioned to the PR subtype rapidly succumbed to their disease (Fig. 5b ), with a median OS after the detected progression of 88 days (Supplementary Fig. 15 ), and had inferior outcomes compared to other patients who also progressed (Fig. 5c ).
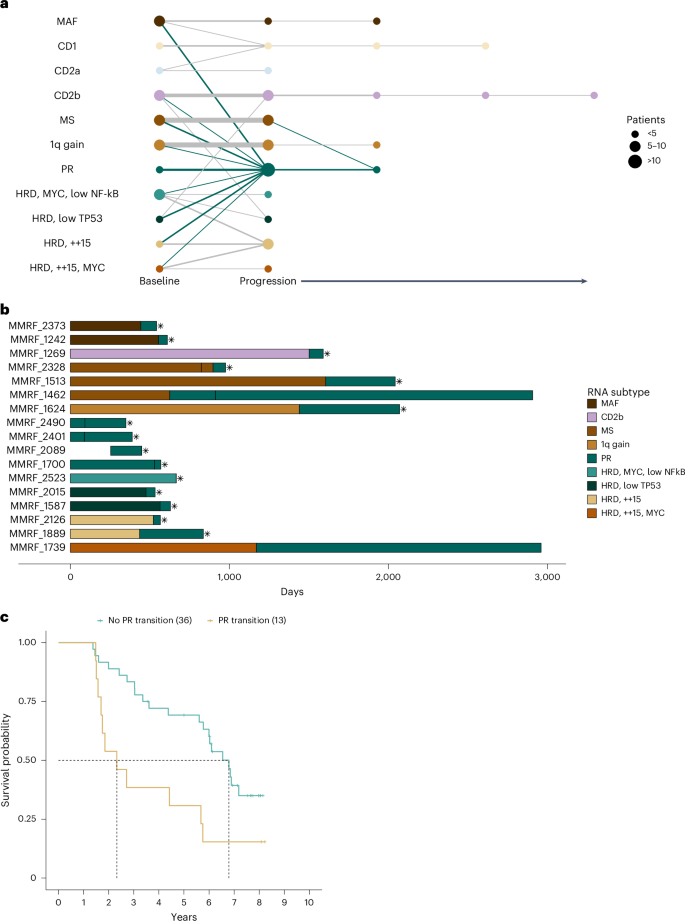
a , Node size reflects the relative number of patients in each RNA subtype at each time point, while edge width reflects the relative number of patients remaining in, or transitioning to, a particular RNA subtype, with the thinnest line and thickest line representing one and seven patients, respectively. b , Swimmers plot of patients in the PR subtype at either baseline or progression. Vertical breaks indicate visits with available RNA-seq data for RNA subtype prediction. Fill color indicates RNA subtype between visits. Asterisks denote OS events. c , Pointwise OS outcomes for serial patients who transition to the PR subtype at progression (median = 27.9 months, 95% CI = 18.9–68.1) versus those that do not (median = 81.5 months, 95% CI = 67.3–NA) are shown. The pairwise outcomes were significantly different ( P = 0.0081) by the log-rank test.
To identify molecular events potentially driving the transition of patients to the PR subtype, gene functional status was compared at the PR and prior non-PR time points. Molecular data were available for comparison at both time points for 9 of 13 patients who transitioned to PR. Despite the prevalence at baseline, none of the patients transitioning to PR acquired complete LOF of RB1 . However, three patients (33%) had complete LOF of a cyclin-dependent kinase inhibitor at progression. Two patients had complete LOF of CDKN2C at progression due to homozygous deletions (Extended Data Fig. 10 ). One patient acquired two independent deletions at progression, while the other had one clonal deletion at diagnosis, and the second increased from 26% to 100% at progression, suggesting this aggressive clone existed before treatment. One patient acquired complete loss of CDKN1B at progression from a pre-existing deletion, and a clonal frameshift mutation was detected only at progression (Supplementary Fig. 16 ). Similar to the baseline observations, there are multiple genetic defects in G1/S checkpoint genes that can result in the PR phenotype.
The MMRF CoMMpass study represents—to the best of our knowledge—the largest single sequencing study of patients with multiple myeloma undertaken to date based on the number of enrolled patients and the total number of sequencing assays performed. The cohort has facilitated the identification of distinct copy number and expression subtypes of myeloma, as well as both recurrent and rare molecular events that occur at frequencies that would not be detected in smaller patient cohorts.
A diverse array of genetic events can contribute to the development or progression of cancer, with individual genes often being affected by multiple types of alterations; however, these diverse processes are generally summarized in isolation, thus underrepresenting a gene’s contribution. To accurately summarize the frequency of these changes, we integrated seven different data formats extracted from WES, WGS and RNA-seq data and identified 70 LOF and 27 GOF genes occurring in five or more patients. Differentiating between partial and complete LOF is pertinent for the accurate identification of high-risk patient populations. For TP53 , solitary deletions or mutations have been associated with poor prognosis; however, only patients with complete LOF of TP53 have poor outcomes, suggesting that only one-third of patients with del(17p13) identified by clinical cytogenetic assays are true high-risk patients 12 , 24 , 25 , 26 . Finally, there is a long-standing interest in determining the gene associated with monosomy 13 in myeloma, detected in 44.9% of patients. Our analysis not only identified recurrent complete loss events in RB1 and DIS3 but also identified independent complete loss events in PSPC1 , TGDS , LATS2 , BRCA2 , PARP4 , MYCBP2 , TPP2 , CDK8 , TSC22D1 and ARHGEF7 , suggesting that multiple genes on chr13 can independently contribute to myelomagenesis. Oddly, DIS3 LOF events almost always maintain a full-length polypeptide, suggesting the dual function of this gene in PCs. Notably, no complete loss event was identified in nearly half of the patients (48.1%) with monosomy 13, thus haploinsufficiency, as observed in Mir15a/Mir16-1, may also be a contributing factor 27 .
We identified eight distinct copy number subtypes, including five HRD and three NHRD subtypes. Although previous studies have shown that HRD patients have favorable outcomes compared to NHRD patients, in CoMMpass there was no difference in outcomes. This large cohort analysis revealed a number of seemingly interrelated events, such as 1q gains and monosomy 13, HRD patients lacking both trisomy 3 and trisomy 7, and groups with a classic HRD phenotype defined by trisomy or tetrasomy 15. Interestingly, the HRD subtype with 1q gain and chr13 loss lacks the classic trisomy 11, suggesting that the combination of these events can phenocopy the benefits of trisomy 11. Although patients with 1q gain and 13 loss represent poor outcome copy number subtypes, the median OS of these patients was between 56 and 69 months, comparable to the 54 months observed for ISSIII, but not the 44-month OS associated with R-ISSIII, which includes patients with high-risk clinical and cytogenetic features 15 . Taken together, this highlights that copy number features alone are insufficient to distinguish the subset of ultra-high-risk patients.
Previous studies clustering microarray gene expression data identified eight to ten unique subtypes, many of which were consistent among studies including the MS, MAF, CD1, CD2 and PR subtypes 17 , 18 . Our consensus clustering of RNA-seq data identified 12 unique RNA subtypes. Some previously identified subtypes were further subdivided, while others were renamed to better reflect the underlying biology, aided by incorporating the diverse data types in this study. For instance, several studies have sought to identify treatment strategies for CD20-positive patients, but based on the distribution within CD2a and CD2b, it may be pertinent to consider that these patients originate from two unique populations. In the context of precision medicine, the strong link between t(11;14) and the CD subtypes leads to the question of whether one of the CD subtypes better predicts response to venetoclax than t(11;14) 28 .
The PR RNA subtype defined a group of patients with extremely poor OS, high proliferative index scores and nearly ubiquitous 1q gains. This group has remained controversial because of the competing supervised patient segregation TC classification models 29 , 30 that argue to group patients by defined genetic features, while this subtype has a mixture of those genetic events. Through our integrated analysis, we identified LOF of RB1 or MAX as common genetic events in baseline PR patients, providing a genomic link to this gene expression phenotype. Loss of MAX was recently associated with transformation and increased proliferation in small cell lung cancer 31 , and thus, LOF of RB1 or MAX likely contributes to the highly proliferative phenotype observed in PR patients. PR patients exhibited lower median expression of checkpoint targets but equivalent or higher expression of most immunotherapy targets when compared to non-PR patients, suggesting that immunotherapies may represent a viable therapeutic option in these high-risk patients and highlighting the importance of identifying these patients in future clinical studies.
There was also a strong tendency for patients to transition to the PR subtype at progression, with 26.5% of serial patients in a non-PR subtype at diagnosis transitioning to PR, similar to recent results 32 . Patients who transitioned to the PR subtype had extremely poor outcomes after the transition, with a median survival of less than 3 months after their progression visit. An acquired complete loss of a cyclin-dependent kinase inhibitor, such as CDKN2C or CDKN1C , was observed in 33% of patients transitioning to the PR subtype at progression, suggesting that transition to the PR phenotype at progression is associated with genetic events disrupting cell cycle control.
These findings demonstrate that advanced molecular diagnostics such as WGS and RNA-seq are better predictors of disease behavior than current staging systems based on clinical laboratory, conventional cytogenetic and fluorescence in situ hybridization (FISH) data. These assays also identify therapeutic targets, including RAS, BRAF V600E and FGFR3 mutations, that are actionable with agents already approved for other cancer indications. In fact, early CoMMpass findings drove the development of a number of clinical trials, notably MMRC-085 Myeloma-Developing Regimens Using Genomics ( NCT03732703 ), an umbrella trial using targeted exome and transcriptome sequencing to stratify subjects into subprotocols with approved targeted agents. These findings favor the adoption of advanced molecular diagnostics into the routine care of myeloma, especially as the breadth of clinical impact broadens and costs decrease.
Comprehensive molecular analyses of the baseline CoMMpass cohort have permitted a more thorough understanding of the genetic diversity and subtypes of the disease. This approach clearly defined the primary molecular features driving different subtypes of multiple myeloma and identified high-risk patients at both diagnosis and progression. Innovative clinical trials targeting this high-risk population are needed given the current poor outcomes with therapies that are otherwise highly effective in other subtypes. Given that patients frequently transition to the high-risk PR subtype at progression, it will be important to know the percentage of PR patients in clinical trial populations, particularly in the relapse/refractory setting, to understand if the arms are balanced and if there is a difference in response between these groups. The identification of unique subtypes and the frequency of target gene dysregulation, via our integrated analysis, provide a solid foundation to prioritize targets for precision medicine approaches in multiple myeloma.
Sample collection and biobanking
Patients were enrolled in the CoMMpass study ( NCT01454297 ), sponsored by the MMRF in accordance with the Declaration of Helsinki. The study was approved by panel 2 of the Western Institutional Review Board and the Internal Review Board at each participating site, and the patients were not compensated for participation and were only enrolled after providing written informed consent. All samples analyzed originate from interim analysis 14, and clinical data analyzed in this study were collected as of interim analysis 22. The MMRF CoMMpass study accrued patients from clinical sites in Canada, Italy, Spain and the United States. All patient samples were shipped to one of the following three biobanking operations: Van Andel Research Institute (VARI) in Grand Rapids, Michigan, for all samples collected in Canada or the United States; University Hospital of Salamanca for samples collected in Spain; or University of Torino for samples collected in Italy. In North America and Spain, potassium ethylenediaminetetraacetic acid (K2-EDTA) tubes were used for the collection of peripheral blood (PB), and sodium heparin tubes were used for the collection of bone marrow (BM) aspirates. These samples were shipped to their respective biobanks using CoMMpass study kits that maintained samples at 7–12 °C. In Italy, clinical sites participating in the FORTE clinical trial ( NCT02203643 ) collected BM and PB sample aspirates in sodium citrate vacutainers. Samples collected at sites in Italy were shipped at ambient temperature to the biorepository site.
At VARI, the received BM and PB specimens were first quality controlled by flow cytometry to determine the percentage of PCs in the PB and BM specimens. Patients were only included in the study when the submitted BM contained at least 1% PCs. If the PB showed less than 1% circulating PCs, white blood cells were used as the constitutional DNA source; however, when >1% circulating PCs were observed, enriched CD3-positive T cells were used. Whole BM PC enrichment, or PB PC enrichment when >5% circulating PCs were detected, was performed using the Miltenyi autoMACS Pro Separator using anti-CD138 microbeads. The purity of the enriched samples was assessed using a three-color slide-based immunofluorescence assay that identified cells with DAPI and the presence or absence of κ or λ light chains. Clinically eligible baseline patients with tumor samples with greater than 250,000 cells recovered after CD138 enrichment and monoclonal purity greater than or equal to 80% moved forward for nucleic acid extraction. For progression samples, the cell recovery requirement was 200,000 cells. To minimize nucleic acid isolation failures, the first 750,000 cells were used exclusively for DNA isolation. When more than 750,000 cells were recovered, the sample was split 50/50 for DNA and RNA isolations. When more than 4 million cells were recovered, multiple aliquots were stored for future use. Cells destined for DNA isolation were stored as snap-frozen pellets, while samples for RNA extraction were lysed in QIAzol before long-term storage at −80 °C.
Samples from clinical sites in Spain were collected and shipped using the provided CoMMpass collection kits. Red blood cells were removed from the PB and BM specimens using a red cell lysis buffer, and, following a PBS wash, the remaining white blood cells were counted. After red cell lysis, the isolated cells were quality controlled using flow cytometry to determine the percentage of PCs in the PB and BM specimens. If the PB showed less than 1% circulating PCs, white blood cells were used as the constitutional DNA source; however, when >1% circulating PCs were observed, enriched CD3-positive T cells were used. The isolated PB cells (1–5 million cells) were snap-frozen as dry pellets for constitutional DNA isolation. The isolated BM cells were stained with anti-CD138 microbeads, and PCs were enriched using a Miltenyi autoMACS Pro Separator. The enriched CD138 + cells were stored as snap-frozen dry pellets, and, when possible, a separate aliquot was lysed with QIAzol and stored at −80 °C until shipped on dry ice to VARI for isolation. The purity of the CD138-enriched PC fractions was determined using flow cytometry with antibodies against CD38, CD138 and CD45.
Samples collected in Italy were treated with red cell lysis buffer. After washing the remaining WBC with PBS, the cells were counted. The isolated PB cells (1–5 million cells) were snap-frozen as dry pellets for constitutional DNA isolation. The BM WBC were stained with anti-CD38 magnetic beads, and PCs were enriched using a Miltenyi autoMACS Pro Separator. After sorting, the purity of the enriched specimens was assessed by flow cytometry using a fluorescent anti-CD38 antibody. Cells destined for DNA isolation were stored as snap-frozen pellets, while samples for RNA extraction were lysed in QIAzol before long-term storage at −80 °C.
Flow cytometry phenotyping and quality control process
All samples received at VARI were tested by flow cytometry to phenotype and quality control the received specimens. The antigens and corresponding commercial antibodies used in the flow cytometry assays are as follows: CD38 (BD Biosciences, 340677), CD45/PTPRC (BD Biosciences, 340665), CD138/SDC1 (BD Biosciences, 347205), CD319/SLAMF7 (Invitrogen/eBioscience, 12-2229-42), CD13/ANPEP (BD Biosciences, 340686), CD19 (BD Biosciences, 340720), CD20/MS4A1 (BD Biosciences, 346581), CD27 (BD Biosciences, 654665), CD28 (BD Biosciences, 348047), CD33 (BD Biosciences, 340679), CD52 (Life Technologies, MHCD5204), CD56/NCAM1 (BD Biosciences, 340724), CD117/KIT (BD Biosciences, 340867), FGFR3/CD333 (R&D Systems, FAB766P), κ (BD Biosciences, 643774) and λ (Life Technologies, MH10614). Flow panels performed included CD38 × CD45 × CD138 × CD56 (initial BM and PB screening panel 1), CD38 × CD45 × CD138 × CD319 (updated screening panel 1 after the introduction of daratumumab), CD38 × CD45 × cytoplasmic κ × cytoplasmic λ (BM and PB screening panel 2), CD38 × CD45 × CD138 × either CD13, CD19, CD20, CD27, CD28, CD33, CD52, CD117 or FGFR3, and propidium iodide-stained nuclei to determine the DNA content of each tumor.
Nucleic acid isolation
All nucleic acid isolations were performed at VARI. DNA was extracted from the dry cell pellets with the Qiagen Gentra Puregene Tissue Kit (Qiagen, 158667) with isolated DNA suspended in Qiagen buffer ATE and stored at −20 °C. DNA was extracted from PB samples using the Qiagen QIAsymphony, which uses magnetic beads for automated sample processing. Blood tubes were either processed immediately upon receipt or frozen at −20 °C and processed in batches. QIAsymphony extractions were performed using the DSP DNA Midi Kit (Qiagen, 937255). DNA was eluted in Qiagen buffer ATE and stored at −20 °C. DNA was quantified by NanoDrop spectrophotometric analysis as well as by fluorescence using Qubit 2.0 to determine dsDNA content. Sample quality was determined by agarose gel or Agilent TapeStation Genomic ScreenTape. Samples with at least 250 ng of dsDNA were submitted to TGen for analysis.
Tumor cells designated for RNA extraction were dissolved in QIAzol Lysis Reagent (Qiagen, 79306), stored at −80 °C and extracted with the Qiagen RNeasy Plus Universal Mini Kit (Qiagen, 73404). RNA was eluted in nuclease-free water and stored at −80 °C. RNA was quantified by NanoDrop spectrophotometric analysis, and RNA quality was evaluated using the Agilent Bioanalyzer 2100. Samples with a RNA integrity number (RIN) ≥ 6.0 and at least 200 ng of RNA were submitted to TGen for analysis.
Sequencing library construction
Details on the specific procedures used for genome, exome and RNA-seq library construction and Illumina-based sequencing are provided in the accompanying Supplementary Information .
Primary sequencing data analysis
Analysis of all sequencing data was performed at TGen on a high-performance computing system using an internally developed analysis pipeline (Medusa Subversion, https://github.com/tgen/medusaPipe ) and the MMRF CoMMpass-specific TGen05 recipe. This recipe is based on the hs37d5 version of the GRCh37 reference genome used by the 1000 Genomes Project, with gene and transcript models from Ensembl v74. Additional automated CoMMpass-specific primary processing was also performed ( https://github.com/tgen/Post_Medusa_Processing ). Code for the creation of the reference genome and gene models used, as well as secondary and tertiary analysis methods, are available on GitHub ( https://github.com/tgen/MMRF_CoMMpass ).
The paired-end fastq files generated in the long-insert whole genome sequencing (LI-WGS) and WES assays from each sequencing lane were aligned with bwa (v0.7.8-r455). The output SAM file was converted to a BAM file and sorted using SAMtools (v0.1.19-44428cd), after which base recalibration was performed using GATK (3.1-1-g07a4bf8). When multiple lanes existed, they were merged into a single BAM file, duplicate reads were marked using Picard (v1.111(1901)) and joint indel realignment was performed using GATK to produce the final BAM files used for analysis. The quality of each assay was determined using multiple Picard and SAMtools quality control metrics. To be included in the analysis, both the tumor and constitutional sample needed to meet the following criteria for genomes: physical coverage of ≥25×, chimera read rate of <3% and derivative log ratio spread (DLRs) of ≤0.2. To be included in the analysis, both the tumor and constitutional sample needed to meet the following criteria for exomes: >90% target bases at 20× coverage and chimera read rate of <3%. Somatic mutations were identified using Seurat (v2.6, https://github.com/tgen/seurat ), Strelka (v1.0.13) and MuTect (v2.2-25-g2a68eab), and their outputs were combined to identify somatic events called by at least two callers. The coding effect of each mutation was determined using snpEFF (v4.2 (build 2015-12-05)), and additional annotations were added using snpSIFT. Somatic structural abnormalities were detected using Delly (v0.7.6), to which additional filtering fields were added to ensure that informative read pairs spanned at least a 100 bp window on both breakends. The MYC STR flag was derived from Delly structural calls and indicates the detection of either (1) a MYC translocation (Ig or non-Ig) or (2) an intrachromosomal deletion with a break end of <3 Mb centromeric of, but not spanning, MYC. Somatic copy number abnormalities were identified with a CoMMpass-specific implementation of tCoNut ( https://github.com/tgen/MMRF_CoMMpass ).
Paired-end fastq files from the RNA-seq assays were aligned using STAR (v2.3.1z, 24 January 2013), and the output SAM file was converted to a BAM file and sorted using SAMtools followed by duplicate marking with Picard. For RNA-seq to be included in the analyses, we required at least 50 million read pairs (100 million reads) generated from each library, a 5′ bias ratio ≥0.5 and a 5′/3′ bias ratio ≥0.75. Gene expression estimates were determined using multiple tools. Counts were extracted from the unsorted SAM file using HtSeq (v0.6.0). TPMs were estimated with Salmon (0.7.2) using the fastq reads as input for quasi-alignment to a transcriptome defined by the GTF gene model. To correct for the variable level of immunoglobulin transcription between samples that compress the TPM values, we removed plasma-cell specific transcripts, including immunoglobulin, mitochondrial, rRNA and chrY genes, from the final TPM calculation ( https://github.com/tgen/Post_Medusa_Processing ). Gene fusion events were identified using the TopHat-Fusion workflow in TopHat2 (2.0.8b) followed by independent cross-validation that an associated genomic event existed in the matched LI-WGS assay.
The genotypes of each result file were compared using SNPsniffer (v5; https://github.com/tgen/snpSniffer ) to ensure that the constitutional DNA, tumor DNA and tumor RNA were from the same individual and that each patient was uniquely represented. To ensure accurate alignment with clinical data, molecular predicted sex was required to match the clinically recorded sex, and, when available, the clinical immunoglobulin isotype was confirmed to match the isotype defined by flow cytometry and RNA-seq. All constitutional DNA samples were manually reviewed to ensure that they represented a diploid genome. Potential low-level cross-contamination of the tumor DNA specimens was determined by comparing the number of high-confidence mutations detected versus the percentage of those mutations that exist in dbSNP.
Survival analyses
Survival curves were computed using the Kaplan–Meier method as implemented in R by the survfit function from the survival (v3.1-8) package and plotted using the ggsurvplot function from the survminer (v0.4.6) package. Pairwise comparisons of survival curves were calculated using pairwise_survdiff from the survminer package. Time to second-line survival estimates were computed using the TTSLT_Censor_Flag (censt2line_curated) and Time_To_Second_Line_Therapy (ttct2line_curated) fields (Supplementary Table 1 ). OS estimates were computed using OS_Censor_Flag (censos) and Time_To_Censored_OS (ttcos) fields. Univariate and multivariate Cox proportional hazards models were calculated using the coxph function from the survival package.
Secondary sequencing data analysis
Details on the specific secondary analysis procedures used for the integrated analysis, consensus clustering, along with clinical and molecular associations with subtypes are outlined in detail in the accompanying Supplementary Note .
Statistics and reproducibility
No statistical method was used to predetermine sample sizes, and each experiment used all samples meeting the outline quality control requirements. The investigators were not blinded to allocation during experiments, as subset allocation was based on observed features and outcome assessment.
Reporting summary
Further information on research design is available in the Nature Portfolio Reporting Summary linked to this article.
Data availability
The MMRF CoMMpass study was funded in part by a precompetitive consortium of pharmaceutical companies that had early access to each data release. This body of work uses data from Interim Analysis 22, the most recent nonembargoed dataset available at this time, which includes the full 8 years of planned observation for 963 of 1,143 (84.3%) patients. All of the data needed to reproduce the presented observations are fully accessible. These data have been available on two platforms for the majority of this study. The nonidentifiable clinical data and processed datasets are on the MMRF researcher gateway ( https://research.themmrf.org/ ), where data are freely accessible for all academic researchers who register. For-profit entities can contact the MMRF to negotiate access. Identifiable raw DNA and RNA-seq read data are deposited in dbGAP under accession phs000748. All sequencing read data in dbGAP are available under the General Research Use consent group and can be freely accessed through the standard dbGAP data access request process. As part of a data-sharing agreement with the National Institutes of Health—National Cancer Institute, the IA11 dataset was added to the Genomic Data Commons (GDC; https://gdc.cancer.gov/ ). Nonidentifiable clinical data and processed sequencing data from that release are available from the GDC, where the processed data originates from their harmonized data processing pipeline. Going forward, the clinical and molecular datasets will also be available at https://www.mmrfvirtuallab.org/ and can be accessed through an email to [email protected]. Supplementary Tables 1–7 referenced in this manuscript are also freely available on Zenodo ( https://doi.org/10.5281/zenodo.10608273 ).
Code availability
The complete analysis pipeline used to process all sequencing result files is publicly available on the TGen GitHub repository ( https://github.com/tgen ). The pipeline code base, individual repositories and software version are noted in the Methods .
Lewis, J. P. & Mackenzie, M. R. Non‐random chromosomal aberrations associated with multiple myeloma. Hematol. Oncol. 2 , 307–317 (1984).
Article CAS PubMed Google Scholar
Avet-Loiseau, H. et al. Prognostic significance of copy-number alterations in multiple myeloma. J. Clin. Oncol. 27 , 4585–4590 (2009).
Article CAS PubMed PubMed Central Google Scholar
Carrasco, D. R. et al. High-resolution genomic profiles define distinct clinico-pathogenetic subgroups of multiple myeloma patients. Cancer Cell 9 , 313–325 (2006).
Venti, G., Mecucci, C., Donti, E. & Tabilio, A. Translocation t(11;14) and trisomy 11q13-qter in multiple myeloma. Ann. Genet. 27 , 53–55 (1984).
CAS PubMed Google Scholar
Bergsagel, P. L. et al. Promiscuous translocations into immunoglobulin heavy chain switch regions in multiple myeloma. Proc. Natl Acad. Sci. USA 93 , 13931–13936 (1996).
Shaughnessy, J. et al. Cyclin D3 at 6p21 is dysregulated by recurrent chromosomal translocations to immunoglobulin loci in multiple myeloma. Blood 98 , 217–223 (2001).
Avet-Loiseau, H. et al. Oncogenesis of multiple myeloma: 14q32 and 13q chromosomal abnormalities are not randomly distributed, but correlate with natural history, immunological features, and clinical presentation. Blood 99 , 2185–2191 (2002).
Boersma‐Vreugdenhil, G. R. et al. The recurrent translocation t(14;20)(q32;q12) in multiple myeloma results in aberrant expression of MAFB: a molecular and genetic analysis of the chromosomal breakpoint. Br. J. Haematol. 126 , 355–363 (2004).
Article PubMed Google Scholar
Fonseca, R. et al. The recurrent IgH translocations are highly associated with nonhyperdiploid variant multiple myeloma. Blood 102 , 2562–2567 (2003).
Bolli, N. et al. Heterogeneity of genomic evolution and mutational profiles in multiple myeloma. Nat. Commun. 5 , 2997 (2014).
Lohr, J. G. et al. Widespread genetic heterogeneity in multiple myeloma: implications for targeted therapy. Cancer Cell 25 , 91–101 (2014).
Walker, B. A. et al. Mutational spectrum, copy number changes, and outcome: results of a sequencing study of patients with newly diagnosed myeloma. J. Clin. Oncol. 33 , 3911–3920 (2015).
Chapman, M. A. et al. Initial genome sequencing and analysis of multiple myeloma. Nature 471 , 467–472 (2011).
Greipp, P. R. et al. International staging system for multiple myeloma. J. Clin. Oncol. 23 , 3412–3420 (2005).
Palumbo, A. et al. Revised international staging system for multiple myeloma: a report from International Myeloma Working Group. J. Clin. Oncol. 33 , 2863–2869 (2015).
Keats, J. J. et al. Promiscuous mutations activate the noncanonical NF-κB pathway in multiple myeloma. Cancer Cell 12 , 131–144 (2007).
Zhan, F. The molecular classification of multiple myeloma. Blood 108 , 2020–2028 (2006).
Broyl, A. et al. Gene expression profiling for molecular classification of multiple myeloma in newly diagnosed patients. Blood 116 , 2543–2553 (2010).
Dunham, I. et al. An integrated encyclopedia of DNA elements in the human genome. Nature 489 , 57–74 (2012).
Article CAS Google Scholar
Davis, C. A. et al. The Encyclopedia of DNA Elements (ENCODE): data portal update. Nucleic Acids Res. 46 , gkx1081 (2017).
Google Scholar
Walker, B. A. et al. APOBEC family mutational signatures are associated with poor prognosis translocations in multiple myeloma. Nat. Commun. 6 , 6997 (2015).
Misund, K. et al. MYC dysregulation in the progression of multiple myeloma. Leukemia 34 , 322–326 (2020).
Cho, S.-J. et al. Ninjurin1, a target of p53, regulates p53 expression and p53-dependent cell survival, senescence, and radiation-induced mortality. Proc. Natl Acad. Sci. USA 110 , 9362–9367 (2013).
Chng, W. J. et al. Clinical significance of TP53 mutation in myeloma. Leukemia 21 , 582–584 (2007).
Boyd, K. D. et al. A novel prognostic model in myeloma based on co-segregating adverse FISH lesions and the ISS: analysis of patients treated in the MRC Myeloma IX trial. Leukemia 26 , 349–355 (2012).
Walker, B. A. et al. A high-risk, double-hit, group of newly diagnosed myeloma identified by genomic analysis. Leukemia 33 , 159–170 (2019).
Chesi, M. et al. Monosomic loss of MIR15A/MIR16-1 is a driver of multiple myeloma proliferation and disease progression. Blood Cancer Discov. 1 , 68–81 (2020).
Kumar, S. et al. Efficacy of venetoclax as targeted therapy for relapsed/refractory t(11;14) multiple myeloma. Blood 130 , 2401–2409 (2017).
Bergsagel, P. L. et al. Cyclin D dysregulation: an early and unifying pathogenic event in multiple myeloma. Blood 106 , 296–303 (2005).
Stein, C. K. et al. The varied distribution and impact of RAS codon and other key DNA alterations across the translocation cyclin D subgroups in multiple myeloma. Oncotarget 8 , 27854–27867 (2017).
Article PubMed PubMed Central Google Scholar
Augert, A. et al. MAX functions as a tumor suppressor and rewires metabolism in small cell lung cancer. Cancer Cell 38 , 97–114 (2020).
Boyle, E. M. et al. High‐risk transcriptional profiles in multiple myeloma are an acquired feature that can occur in any subtype and more frequently with each subsequent relapse. Br. J. Haematol. 195 , 283–286 (2021).
Annunziata, C. M. et al. Frequent engagement of the classical and alternative NF-kappaB pathways by diverse genetic abnormalities in multiple myeloma. Cancer Cell 12 , 115–130 (2007).
Demchenko, Y. N. et al. Classical and/or alternative NF-kappaB pathway activation in multiple myeloma. Blood 115 , 3541–3552 (2010).
Download references
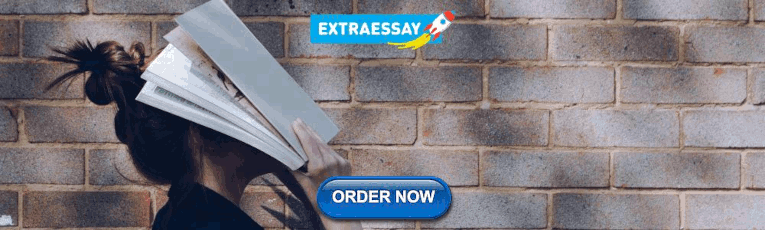
Acknowledgements
The CoMMpass study was funded by a grant from the MMRF (to J.J.K., J.C., S.D.J., P.K. and S.L.). The authors would like to thank all of the MMRF CoMMpass study participants and their families for making this research possible. The authors would also like to thank all of the laboratory staff at the Spectrum Health Advanced Technology Laboratory who assisted with sample processing and flow cytometry analysis.
Author information
Authors and affiliations.
Integrated Cancer Genomics Division, Translational Genomics Research Institute, Phoenix, AZ, USA
Sheri Skerget, Daniel Penaherrera, Austin W. Christofferson, Sara Nasser, Jessica L. Aldrich, Christophe Legendre, Brooks Benard, Chase Miller, Bryce Turner, Ahmet Kurdoglu, Megan Washington, Venkata Yellapantula, Jonathan R. Adkins, Lori Cuyugan, Martin Boateng, Adrienne Helland, Shari Kyman, Jackie McDonald, Rebecca Reiman, Kristi Stephenson, Erica Tassone, David Craig, Winnie S. Liang, John Carpten & Jonathan J. Keats
Tisch Cancer Institute, Icahn School of Medicine at Mount Sinai, New York City, NY, USA
Ajai Chari, Sundar Jagannath & Hearn Jay Cho
Hackensack University Medical Center, Hackensack, NJ, USA
David S. Siegel
Division of Oncology, Washington University, St. Louis, MO, USA
Virginia Cancer Specialists, Fairfax, VA, USA
Gregory Orloff
University of Chicago Medical Center, Chicago, IL, USA
Andrzej Jakubowiak
Weill Cornell Medicine, New York City, NY, USA
Ruben Niesvizky
Division of Hematology/Oncology, East Carolina University, Greenville, NC, USA
Darla Liles
Sarah Cannon Research Institute, Nashville, TN, USA
Jesus Berdeja
Baylor Scott and White Research Institute, Dallas, TX, USA
Department of Medicine, UCSF Medical Center, San Francisco, CA, USA
Jeffrey Wolf
Levine Cancer Institute, Charlotte, NC, USA
Saad Z. Usmani
Van Andel Institute, Grand Rapids, MI, USA
Alex Blanski, Brianne Livermore, Meghan Kirchhoff, Daniel C. Rohrer & Scott D. Jewell
Division of Hematology, AOU Città della Salute e della Scienza di Torino, University of Torino and Department of Molecular Biotechnology and Health Sciences, Torino, Italy
Mattia D’Agostino & Manuela Gamella
Spectrum Health, Grand Rapids, MI, USA
Kimberly Collison, Jennifer Stumph & Pam Kidd
Precision for Medicine, Flemington, NJ, USA
Andrea Donnelly & Barbara Zaugg
InStat Services, Chatham, NJ, USA
Maureen Toone & Kyle McBride
Multiple Myeloma Research Foundation, Norwalk, CT, USA
Mary DeRome, Jennifer Rogers, Hearn Jay Cho & Daniel Auclair
Department of Hematology, University Hospital of Salamanca, IBSAL, Cancer Research Center-IBMCC (USAL-CSIC), CIBERONC, Salamanca, Spain
Norma C. Gutierrez
Dana–Farber Cancer Institute, Harvard Cancer Center, Boston, MA, USA
Kenneth C. Anderson
Department of Hematology and Medical Oncology, Emory University School of Medicine, Atlanta, GA, USA
Sagar Lonial
Rocky Mountain Cancer Centers, Denver, CO, USA
- Robert Rifkin
Norris Cotton Cancer Center, Dartmouth–Hitchcock Medical Center, Lebanon, NH, USA
Kenneth Meehan
Ohio State University Comprehensive Cancer Center, Columbus, OH, USA
Barbara Ann Karmanos Cancer Institute, Detroit, MI, USA
Jeffrey Zonder
Washington DC VA Medical Center, Washington, DC, USA
Joao Ascensao
Duke University Health System, Durham, NC, USA
Cristina Gasparetto
Hospital Universitario de Canarias, Santa Cruz, Spain
Miguel Hernández
Princess Margaret Cancer Centre, Toronto, Ontario, Canada
Suzanne Trudel
Cancer Center of Kansas, Wichita, KS, USA
Shaker Dakhil
Tom Baker Cancer Centre, Calgary, Alberta, Canada
Nizar Bahlis
Infanta Sofia University Hospital, Madrid, Spain
Juan Vazquez Paganini
Hospital Nuestra Senora de la Candelaria, Santa Cruz, Spain
Hospital Universitario Son Espases, Palma, Spain
Antonia Sampol
Yakima Valley Memorial Hospital/North Start Lodge, Yakima, WA, USA
Siva Mannem
Oregon Health and Science University, Portland, OR, USA
Rebecca Silbermann
University of Nebraska Medical Center, Omaha, NE, USA
Matthew Lunning
Cross Cancer Institute, Edmonton, Alberta, Canada
Michael Chu
University of Mississippi Medical Center, Jackson, MS, USA
Carter Milner
Cancer Care Centers of South Texas, San Antonio, TX, USA
Allyson Harroff
Waverly Hematology Oncology, Cary, NC, USA
Mark Graham
Northwest Cancer Specialists, P.C., Tualatin, OR, USA
Spencer Shao
Central Texas VA Healthcare Center, Temple, TX, USA
Jyothi Dodlapati
Hospital Clinic i Provincial de Barcelona, Barcelona, Spain
Carlos Fernández de Larrea
Illinois Cancer Specialists, Niles, IL, USA
Leonard Klein
FirstHealth of the Carolinas Outpatient Cancer Center, Pinehurst, NC, USA
Charles Kuzma
Mayo Clinic, Phoenix, AZ, USA
Rafael Fonseca
Hospital Clinica Universitari Lozano Blesa, Zaragoza, Spain
Gemma Azaceta
Hospital Santa Creu I Sant Pau, Barcelona, Spain
Miquel Granell
Hospital Quirón de Madrid, Madrid, Spain
Carmen Martinez-Chamorro
Ocala Oncology Center, P.L., Ocala, FL, USA
Rama Balaraman
Billings Clinic Cancer Research, Billings, MT, USA
Carlos Silva
Hospital Ramón y Cajal, Madrid, Spain
Anabelle Chinea
UC San Diego Moores Cancer Center, La Jolla, CA, USA
Caitlin Costello
Kansas City VA Medical Center, Kansas City, MO, USA
Suman Kambhampati
Randolph Cancer Center, Asheboro, NC, USA
DeQuincy Lewis
New York University School of Medicine, New York City, NY, USA
Michael Grossbard
Grand Rapids Clinical Oncology Program, Grand Rapids, MI, USA
Kathleen Yost
Bay Area Cancer Research Group, LLC, Pleasant Hill, CA, USA
Robert Robles
McGill University Health Center, Royal Victoria Hospital, Montreal, Quebec, Canada
Michael Sebag
Atlanta VA Medical Center, Decatur, GA, USA
Wayne Harris
Peninsula Regional Cancer Center, Salisbury, MD, USA
Justinian Ngaiza
Carl and Dorothy Bennett Cancer Center, Stamford, CT, USA
Michael Bar
Sharp Healthcare, San Diego, CA, USA
Marie Shieh
Holy Cross Hospital, Silver Spring, MD, USA
Fredrick Min
Marshfield Clinic Research Foundation, Marshfield, WI, USA
Adedayo Onitilo
Sciode Medical Associates, PLLC, Bronx, NY, USA
Fabio Volterra
San Diego VA Medical Center, San Diego, CA, USA
William Wachsman
Broome Oncology, LLC, Johnson City, NY, USA
Madhuri Yalamachili
Hospital del Mar, Barcelona, Spain
Eugenia Abella
University of Texas Southwest Medical Center, Dallas, TX, USA
Larry Anderson
Hospital Son Llàtze, Palma, Spain
Joan Bargay
Memorial Sloan-Kettering Cancer Center, New York City, NY, USA
Hani Hassoun
San Francisco VA Medical Center, San Francisco, CA, USA
Cancer Care Northwest, P.S., Spokane, WA, USA
Kaiser Permanente of Colorado, Denver, CO, USA
Alex Menter
St Francis Hospital, East Hills, NY, USA
Dilip Patel
Texas Oncology (P.A.)—Tyler, Tyler, TX, USA
Donald Richards
Maimonides Cancer Center, Brooklyn, NY, USA
William Solomon
Texas Oncology (P.A.)—Waco, Waco, TX, USA
Robert Anderson
Medical Associates of Brevard, Melbourne, FL, USA
Sumeet Chandra
Saint Barnabas Medical Center, Livingston, NJ, USA
Miguel Conde
University of Cincinnati Cancer Institute, Cincinnati, OH, USA
Saulias Girnius
Regional Cancer Care Associates, Sparta, NJ, USA
May Matkiwsky
Hospital Puerta de Hierro, Madrid, Spain
Isabel Krsnik
Mayo Clinic Department of Hematology, Rochester, MN, USA
Shaji Kumar
H. U. Germans Trias i Pujol, Barcelona, Spain
Albert Oriol
Clínica Universitaria de Navarra, Pamplona, Spain
Paula Rodríguez
Mayo Clinic, Jacksonville, FL, USA
East Orange VA Medical Center, East Orange, NJ, USA
Shanti Srinivas
Atlanta Cancer Care, Alpharetta, GA, USA
Ronald Steis
You can also search for this author in PubMed Google Scholar
The MMRF CoMMpass Network
- , Kenneth Meehan
- , Don Benson
- , Jeffrey Zonder
- , Joao Ascensao
- , Cristina Gasparetto
- , Miguel Hernández
- , Suzanne Trudel
- , Shaker Dakhil
- , Nizar Bahlis
- , Juan Vazquez Paganini
- , Pablo Rios
- , Antonia Sampol
- , Siva Mannem
- , Rebecca Silbermann
- , Matthew Lunning
- , Michael Chu
- , Carter Milner
- , Allyson Harroff
- , Mark Graham
- , Spencer Shao
- , Jyothi Dodlapati
- , Carlos Fernández de Larrea
- , Leonard Klein
- , Charles Kuzma
- , Rafael Fonseca
- , Gemma Azaceta
- , Miquel Granell
- , Carmen Martinez-Chamorro
- , Rama Balaraman
- , Carlos Silva
- , Anabelle Chinea
- , Caitlin Costello
- , Suman Kambhampati
- , DeQuincy Lewis
- , Michael Grossbard
- , Kathleen Yost
- , Robert Robles
- , Michael Sebag
- , Wayne Harris
- , Justinian Ngaiza
- , Michael Bar
- , Marie Shieh
- , Fredrick Min
- , Adedayo Onitilo
- , Fabio Volterra
- , William Wachsman
- , Madhuri Yalamachili
- , Eugenia Abella
- , Larry Anderson
- , Joan Bargay
- , Hani Hassoun
- , Gerald Hsu
- , Hakan Kaya
- , Alex Menter
- , Dilip Patel
- , Donald Richards
- , William Solomon
- , Robert Anderson
- , Sumeet Chandra
- , Miguel Conde
- , Saulias Girnius
- , May Matkiwsky
- , Isabel Krsnik
- , Shaji Kumar
- , Albert Oriol
- , Paula Rodríguez
- , Vivek Roy
- , Shanti Srinivas
- & Ronald Steis
Contributions
D.A., J.C., S.L., K.C.A. and J.J.K. conceptualized the project. S.S., D.P., A.W.C., S.N., M.B., S.K., K.S., D.C., W.S.L. and J.J.K. developed the methodology. S.S., D.P., A.W.C., S.N., J.L.A., C.L., B.B., C.M., B.T., A.K., M.W., V.Y., M.T., K.M., D.C. and J.J.K. conducted software analysis. S.S., A.W.C., M.T., K.M. and J.K. performed validation. S.S., D.P., A.W.C., S.N., J.L.A., C.L., B.B., C.M., B.T., A.K., M.W., V.Y. and J.J.K. carried out the formal analysis. A.C., S.J., D.S.S., R.V., G.O., A.J., R.N., D.L., J.B., M.L., J.W., S.Z.U., The MMRF CoMMpass Network, J.R.A., L.C., M.B., A.H., S.K., J.M., R.R., K.S., E.T., A.B., B.L., M.K., M.D.A., M.G., K.C., J.S., P.K., A.D., B.Z., M.T., K.M., S.D.J., H.J.C., S.L. and J.J.K. conducted the investigation. A.C., S.J., D.S.S., R.V., G.O., A.J., R.N., D.L., J.B., M.L., J.W., S.Z.U., The MMRF CoMMpass Network, H.J.C. and S.L. arranged the resources. S.S., D.P., A.W.C., S.N., J.L.A., C.L., D.C.R., M.D.A., M.G., A.D., B.Z., M.T., K.M., M.D., J.R., S.D.J., H.J.C., D.A. and J.J.K. curated the data. S.S. and J.J.K. wrote the original draft. S.S., A.C., D.S.S., S.Z.U., B.B., N.C.G., K.C.A., H.J.C., S.L. and J.J.K. did the writing, reviewing and editing of the manuscript. S.S., D.P., A.W.C., S.N., J.L.A. and J.J.K. handled visualization. S.S., K.C., J.S., P.K., J.R., D.C., W.S.L., D.A., S.D.J., J.C., S.L. and J.J.K. provided supervision. D.C.R., K.C., A.D., B.Z., K.M., M.D., J.R., N.C.G., S.D.J., J.C., D.A., S.L. and J.J.K. managed project administration. P.K., S.D.J., J.C., S.L. and J.J.K. secured funding.
Corresponding authors
Correspondence to Sagar Lonial or Jonathan J. Keats .
Ethics declarations
Competing interests.
S.S. is currently employed by Janssen. A.C. has received research funding from Amgen, BMS, Janssen, Seattle Genetics and Takeda, and is a speaker/consultant/advisory board member for Abbvie, Amgen, Antengene, BMS, Genentech, GSK, Janssen, Karyopharm, Sanofi, Seattle Genetics, Secura Bio, Shattuck Labs and Takeda. S.J. is a speaker/consultant/advisory board member for BMS, Caribou Biosciences, DMC, Janssen, Regeneron, Sanofi and Takeda. D.S.S. has received research funding from Amgen, BMS, Janssen, Karyopharm, Novartis and Takeda, and is a speaker/consultant/advisory board member for Amgen, BMS, Celularity Scientific, Janssen, Karyopharm, Novartis and Takeda. R.V. has received research funding from BMS, Sanofi and Takeda, and is a speaker/consultant/advisory board member for BMS, Harpoon, Janssen Karyopharm, Legend Biotech, Pfizer, Sanofi and Takeda. A.J. is a speaker/consultant/advisory board member for Abbvie, BMS, Amgen, GSK, Janssen and Sanofi. R.N. has received research funding from BMS, GSK, Janssen, Karyopharm, Pfizer, Regeneron and Takeda, and is a speaker/consultant/advisory board member for BMS, GSK, Janssen, Karyopharm, Pfizer, Regeneron, Sanofi and Takeda. D.L. has received research funding from Annexon Biosciences, Alpine Immune, Abbvie, Astex Pharmaceuticals, Baxalta, BeiGene, Bioverativ, CSL Behring, BMS, Delta-Fly Pharma, Exact Sciences, Forma Therapeutics, Global Blood Therapeutics, Immunovant, Incycte, Janssen, NeoImmuneTech, Novartis, Novo Nordisk, Partner Therapeutics, Pharm-Olam, Principia Biopharma, Salix Pharmaceuticals, Sanofi, Takeda and Vifor Pharma. J.B. has received research funding from AbbVie, Acetylon, Amgen, BMS, C4 Therapeutics, CARSgen, Cartesian, Celularity, CRISPR Therapeutics, EMD Serono, Fate Therapeutics, Genentech, GSK, Ichnos Sciences, Incyte, Janssen, Karyopharm, Lilly, Novartis, Poseida, Sanofi, Takeda, Teva and 2seventy bio, and is a speaker/consultant/advisory board member for BMS, CRISPR Therapeutics, Janssen, Kite Pharma, Legend Biotech, Roche and Takeda. M.L. is a speaker/consultant/advisory board member for Abbvie, Amgen, AstraZeneca, BeiGene, BMS, Gilead, Genmab, Janssen, Jazz, Karyopharm, Morphosys, Novartis, Seagen, Sellas, Sobi, Sanofi and Takeda. S.Z.U. has received research funding from AbbVie, Amgen, Array Biopharma, BMS, EdoPharma, Gilead Sciences, GSK, Janssen, K36 Therapeutics, Merck, Moderna, Pharmacyclics, Sanofi, Seattle Genetics, SkylineDX and Takeda, and is a speaker/consultant/advisory board member for BMS, Genentech, Gilead Sciences, GSK, Janssen, Novartis, Oncopeptides, Sanofi, Seattle Genetics, Secura Bio, SkylineDX, Takeda and TeneoBio. M.D’A. has received research funding from Janssen and is a speaker/consultant/advisory board member for Adaptive Biotechnology, BMS, GSK, Janssen and Sanofi. M.D. is currently employed by the funder (MMRF). J.R. is currently employed by the funder (MMRF). K.C.A. is a speaker/consultant/advisory board member for Amgen, AstraZeneca, Daewoong, Dynamic Cell Therapies, Janssen, Pfizer, Mana Therapeutics, Oncopep, Precision Biosciences, Starton and Window Therapeutics, and is an equity holder in C4 Therapeutics, Dynamic Cell Therapies, NextRNA, Oncopep, Raqia, Starton and Window Therapeutics. H.J.C. is currently employed by the funder (MMRF) and has received research funding from BMS and Takeda. D.A. was previously employed by the funder (MMRF) and is currently employed by AstraZeneca. S.L. has received research funding from BMS, Janssen, Novartis and Takeda, and is a speaker/consultant/advisory board member for AbbVie, Amgen, BMS, Genentech, GSK, Janssen, Novartis, Pfizer, TG Therapeutics and Takeda. J.J.K. has received research funding from Amgen, Genentech and Janssen, and is a speaker/consultant/advisory board member for Janssen. The other authors declare no competing interests.
Peer review
Peer review information.
Nature Genetics thanks Stephen Nutt, P. Leif Bergsagel and the other, anonymous, reviewer(s) for their contribution to the peer review of this work. Peer reviewer reports are available.
Additional information
Publisher’s note Springer Nature remains neutral with regard to jurisdictional claims in published maps and institutional affiliations.
Extended data
Extended data fig. 1 survival outcomes of the cohort..
Pointwise survival estimates are shown by the respective dark lines, while the 95% confidence interval is shown by the matching shaded bands. ( a ) Time to second line therapy (median 38.1 months, 95% CI = 35.2–40.6 months) and ( b ) overall survival (OS; median 103.6 months, 95% CI = 92.7–not met). The median of the CoMMpass cohort has been met; however, as of the IA22 release, there is insufficient follow-up to accurately report the upper limit of the OS 95% confidence interval. As expected, ISS stage stratified patients into three clinically distinct classes. ( c ) Time to second line therapy outcomes for patients classified as ISSI (53.7 months, 95% CI = 44.9–63.3), ISSII (35.7 months, 95% CI = 31.5–42.2) and ISSIII (24.4 months, 95% CI = 20.6–28.5) at diagnosis are clearly different. Pairwise outcomes were compared by the log-rank test after multiple testing corrections using the Benjamini–Hochberg method. Significant differences in outcomes were observed ISSI vs ISS2 (P = 7.6e−06), ISS1 vs ISS3 (P < 2e−16) and ISS2 vs ISS3 (P = 2.9e−05). ( d ) OS outcomes for patients classified as ISSI (103.9 months, 95% CI = NA–NA), ISSII (median not met, 95% CI = 91.3–NA) and ISSIII (53.9 months, 95% CI = 43.3–59.6) at diagnosis are compared with significant differences in outcomes observed for ISSI vs ISS2 (P = 0.00023), ISS1 vs ISS3 (P < 2e−16) and ISS2 vs ISS3 (P = 2.2e−09).
Extended Data Fig. 2 Copy number consensus clustering matrix.
Consensus clustering matrix with an optimal clustering solution of K = 8. The M3C (Monte Carlo reference-based) consensus clustering algorithm was applied to the CN measurements of 26,771 (100 Kb) intervals across the GRCh37 reference genome for 871 WGS BM-derived baseline samples. Five of the eight subtypes include only samples classified as hyperdiploid.
Extended Data Fig. 3 Survival outcomes for patients with gain(1q21) and del(13q14).
( a ) Time to second line therapy, and ( b ) OS outcomes for CoMMpass patients with gain(1q21) and del(13q14) (both), gain(1q21) alone, del(13q14) alone and those with neither event. gain(1q21) was defined as a gain of 1 or more copies of 1q21, whereas del(13q14) was defined as a loss of one copy of 13q14. There is a significant difference in time to second line therapy and OS for all groups compared to the group with neither event (p < 0.05); however, there is no significant difference between the groups with gain(1q21) and/or del(13q14). The median time to second line therapy for gain(1q21), del(13q14) patients was 29.3 months (95% CI = 24.4–33.7), while gain(1q21) was 35.3 months (95% CI = 23.6–49.2), del(13q14) was 35.7 months (95% CI = 31.4–40.6), and for those with neither, it was 51.4 months (95% CI = 42.0–55.9). The median OS for gain(1q21), del(13q14) patients was 69.2 months (95% CI = 55.7–97.4), while gain(1q21) was 83.2 months (95% CI = 56.0–not met), del(13q14) was 92.7 months (95% CI = 72.7–not met), conversely the median has not been reached for those with neither. ( c ) In a univariate Cox proportional hazards model, both gain(1q21) (P = 8.2e−05) and del(13q14) (P = 0.0022) were found to significantly impact OS outcome using a Wald test. ( d ) In a multivariate model, both gain(1q21) (n = 307) and del(13q14) (n = 453) were found to have a significant impact on outcome within the full cohort of patients with CN data (n = 871). The box represents the hazard ratio, and the error bars represent the 95% confidence interval.
Extended Data Fig. 4 RNA-seq consensus clustering matrix.
Consensus matrix showing the consistency of class assignment for K = 12 clustering of RNA-seq data derived from 714 BM baseline samples and 4811 feature-selected genes.
Extended Data Fig. 5 RNA subtypes and association with copy number.
Copy number states for patients by RNA subtype are shown. Diploid copy number is represented as 2 (white), copy loss is shaded in blue and copy gain is shaded in red. Rare copy number values exceeding 4 are represented as a copy number value of 4 to maintain uniformity in the heatmap scales for gain and loss.
Extended Data Fig. 6 Relationship between proliferation index and RNA subtypes.
The association with an RNA-seq-defined proliferation index and CoMMpass subtypes is shown (n = 714). The Bergsagel proliferation index 27 for each sample was determined by calculating the geometric mean expression of 12 genes ( TYMS, TK1, CCNB1, MKI67, KIAA101, KIAA0186, CKS1B, TOP2A, UBE2C, ZWINT, TRIP13 and KIF11 ). The PR subtype had the highest median proliferation index score. The index range is shown as a boxplot with the upper and lower bounds of the box representing the 25th and 75th percentile, while the center line indicates the median and whiskers represent the highest and lowest value within 1.5 (IQR).
Extended Data Fig. 7 NF-kB index distribution by RNA subtype.
The association with an RNA-seq-defined NF-kB index and the CoMMpass subtypes is shown (n = 714). The NFKB(11) index for each sample was determined by calculating the geometric mean expression of 11 genes ( BIRC3 , TNFAIP3 , NFKB2 , IL2RG , NFKB1 , RELB , NFKBIA , CD74 , PLEK , MALT1 and WNT10A ) 33 , 34 . The index range is shown as a boxplot with the upper and lower bounds of the box representing the 25th and 75th percentile, while the center line indicates the median and whiskers represent the highest and lowest value within 1.5 (IQR).
Extended Data Fig. 8 Low-purity RNA subtype association with low-purity metrics.
The low-purity RNA subtype was defined based on an association of the samples in this category with multiple independent measures of sample purity. ( a ) An index associated with genes expressed in non-B-cell tissues was used to identify samples with contamination of non-B lineage cells in the CD138 + -enriched cell fractions (n = 714). ( b ) Tumor purity was estimated from the exome copy number or mutation data based on the absolute allele frequency of constitutional variants in deletion regions or somatic SNV allele frequency in diploid regions of the genome when no usable deletions were detected in the tumor (n = 593). The range of estimated contamination ( a ) and purity ( b ) is shown as a boxplot with the upper and lower bounds of the box representing the 25th and 75th percentile, while the center line indicates the median and whiskers represent the highest and lowest value within 1.5 (IQR). ( c ) The full distribution of observed somatic SNV allele frequencies (n = 593) is shown as a violin plot, where the median is indicated by the horizontal line and the population frequency of the value is indicated by the width of the plot.
Extended Data Fig. 9 Change in RNA subtype probabilities over time.
RNA subtype probabilities for the 71 serial patients with RNA-seq data at two or more time points. All patients classified in the low-purity subtype at baseline have a discernable RNA subtype other than low purity at progression, supporting the observation that this subtype is driven by sample purity. Shifts from a non-PR baseline subtype to a largely PR subtype or partial population of PR cells are evident.
Extended Data Fig. 10 Deletion of CDKN2C in patients who transitioned to PR.
Two patients that transitioned to the PR subtype at progression acquired complete loss of function of CDKN2C due to overlapping deletion. Panels show long-insert WGS reads from tumor samples for patients MMRF_2523 ( a ) and MMRF_1269 ( b ) at baseline (non-PR) and progression (PR). ( a ) At baseline, patient MMRF_2523 was diploid (log 2 CN = −0.0747) with no evidence of a deletion spanning CDKN2C; however, at progression, the patient had a 2-copy deletion of CDKN2C (blue bar, log 2 CN = −3.3505) due to two unique deletions (red bars) spanning CDKN2C (green box). ( b ) At baseline, patient MMRF_1269 had a 1 copy loss of CDKN2C (light blue bar, log 2 CN = −0.3511) due to a larger deletion on chr1. There is also read evidence supporting a deletion involving CDKN2C/FAF1, suggesting that a subclonal population with complete loss of CDKN2C was present at diagnosis in this patient. At progression, when the patient transitioned to PR, the patient’s tumor had a 2-copy deletion of CDKN2C (dark blue bar, log 2 CN = −4.6212). In this patient, the minor clone harboring the CDKN2C deletion at baseline constitutes the bulk of the tumor population at progression.
Supplementary information
Supplementary information.
Supplementary Figs. 1–16 and Supplementary Note.
Reporting Summary
Peer review file, supplementary table 1.
Individual patient features.
Supplementary Table 2
Observed somatic SNV and INDEL mutations.
Supplementary Table 3
Observed somatic structural events (deletions, insertions, duplications, inversions and translocations).
Supplementary Table 4
Per specimen gene expression matrix and inframe fusion events.
Supplementary Table 5
Per specimen copy number and alternate allele frequency matrices.
Supplementary Table 6
Per specimen gene level LOF and GOF status.
Supplementary Table 7
Gene expression subtype classifier results and model coefficients.
Rights and permissions
Open Access This article is licensed under a Creative Commons Attribution-NonCommercial-NoDerivatives 4.0 International License, which permits any non-commercial use, sharing, distribution and reproduction in any medium or format, as long as you give appropriate credit to the original author(s) and the source, provide a link to the Creative Commons licence, and indicate if you modified the licensed material. You do not have permission under this licence to share adapted material derived from this article or parts of it. The images or other third party material in this article are included in the article’s Creative Commons licence, unless indicated otherwise in a credit line to the material. If material is not included in the article’s Creative Commons licence and your intended use is not permitted by statutory regulation or exceeds the permitted use, you will need to obtain permission directly from the copyright holder. To view a copy of this licence, visit http://creativecommons.org/licenses/by-nc-nd/4.0/ .
Reprints and permissions
About this article
Cite this article.
Skerget, S., Penaherrera, D., Chari, A. et al. Comprehensive molecular profiling of multiple myeloma identifies refined copy number and expression subtypes. Nat Genet (2024). https://doi.org/10.1038/s41588-024-01853-0
Download citation
Received : 09 July 2021
Accepted : 28 June 2024
Published : 19 August 2024
DOI : https://doi.org/10.1038/s41588-024-01853-0
Share this article
Anyone you share the following link with will be able to read this content:
Sorry, a shareable link is not currently available for this article.
Provided by the Springer Nature SharedIt content-sharing initiative
Quick links
- Explore articles by subject
- Guide to authors
- Editorial policies
Sign up for the Nature Briefing newsletter — what matters in science, free to your inbox daily.

Office of Neuroscience Research
VIRTUAL Genomics in Medicine Seminar: Felicia Gomez (WashU Medicine) – “Molecular Biology/Genetics Refresher”
Hosted by the Clinical Research Training Center (CRTC)
The Genomics in Medicine Seminar Series offers attendees a practical background in molecular biology and genetics, an introduction to genomics technologies and research, and an understanding of the clinical application of genomics knowledge.
The series is designed for clinicians and other interested persons, including basic science researchers, students, residents, fellows, post-doctoral scholars, and anyone else who wishes to learn more about these topics from WUSM faculty experts. All professionals from ICTS Partner Institutions are welcome to participate.
The lectures will take place on Wednesdays at 4pm via Zoom.
Students interested in enrolling in the seminar series for credit can earn up to two units of academic credit.
For inquiries contact Karlee Kreienkamp .
This seminar series is a collaboration between the Departments of Genetics , Medicine , Pathology & Immunology , Pediatrics , and Becker Medical Library . The series is sponsored by the Washington University Institute of Clinical and Translational Sciences , NIH award UL1TR002345.

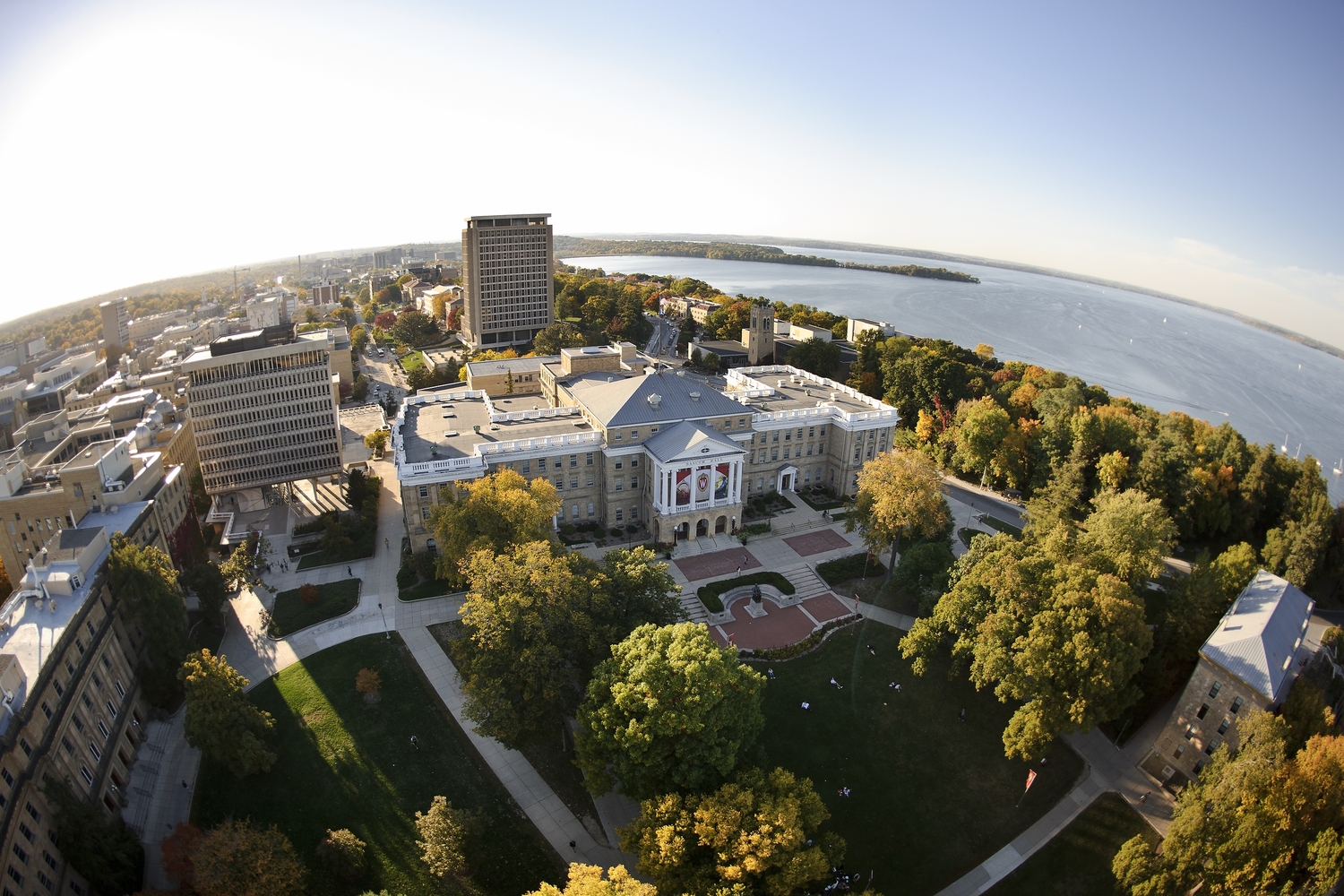
Research Specialist - Genetics
- Madison, Wisconsin
- SCHOOL OF MEDICINE AND PUBLIC HEALTH/PEDIATRICS-GEN
- Staff-Full Time
- Opening at: Aug 20 2024 at 14:50 CDT
- Closing at: Sep 3 2024 at 23:55 CDT
Job Summary:
The Webb laboratory in the Division of Genetics and Metabolism in the Department of Pediatrics is seeking a Researcher to come join us. Our lab focuses on studying the genetics of rare disease. Rare diseases collectively affect ~350 million people globally, and ~80% of rare diseases are genetic in origin, about half affect children, many are fatal, and very few have cures. We focus on further study of rare genetic diseases where Dr. Webb identified the molecular etiology. Ongoing projects in the lab include studying mitochondrial disorders and a newly described pediatric ataxia disorder.
Responsibilities:
- 70% Conducts research experiments according to established research protocols with moderate impact to the project(s). Collects data and monitors test results
- 10% Operates, cleans, and maintains organization of research equipment and research area. Tracks inventory levels and places replenishment orders
- 5% Reviews, analyzes, and interprets data and/or documents results for presentations and/or reporting to internal and external audiences
- 5% Participates in the development, interpretation, and implementation of research methodology and materials
- 5% Provides operational guidance on day-to-day activities of unit or program staff and/or student workers
- 5% Performs literature reviews and writes reports
Institutional Statement on Diversity:
Diversity is a source of strength, creativity, and innovation for UW-Madison. We value the contributions of each person and respect the profound ways their identity, culture, background, experience, status, abilities, and opinion enrich the university community. We commit ourselves to the pursuit of excellence in teaching, research, outreach, and diversity as inextricably linked goals. The University of Wisconsin-Madison fulfills its public mission by creating a welcoming and inclusive community for people from every background - people who as students, faculty, and staff serve Wisconsin and the world. For more information on diversity and inclusion on campus, please visit: Diversity and Inclusion
Preferred Bachelor's Degree
Qualifications:
Background in genetics, molecular biology, or a related field is required. Prior research experience is required. Experience with molecular biology techniques is required. Experience with cell culture and/or mouse model systems is also preferred.
Full Time: 100% It is anticipated this position requires work be performed in-person, onsite, at a designated campus work location.
Appointment Type, Duration:
Ongoing/Renewable
Minimum $47,000 ANNUAL (12 months) Depending on Qualifications Actual pay will depend on experience and qualifications. Employees in this position can expect to receive benefits such as generous vacation, holidays, and paid time off; competitive insurances and savings accounts; retirement benefits. Benefits information can be found at ( https://hr.wisc.edu/benefits/ ).
Additional Information:
University sponsorship is not available for this position, including transfers of sponsorship. The selected applicant will be responsible for ensuring their continuous eligibility to work in the United States (i.e. a citizen or national of the United States, a lawful permanent resident, a foreign national authorized to work in the United States without the need of an employer sponsorship) on or before the effective date of appointment. This position is an ongoing position that will require continuous work eligibility. UW-Madison is not an E-Verify employer, and therefore, is not eligible to employ F1 STEM OPT Extension participants. If you are selected for this position you must provide proof of work authorization and eligibility to work.
How to Apply:
To apply for this position, please click on the "Apply Now" button. You will be asked to upload a current resume/CV and a cover letter briefly describing your qualifications and experience.
Kelsey Scholtens [email protected] 608-262-0006 Relay Access (WTRS): 7-1-1. See RELAY_SERVICE for further information.
Official Title:
Research Specialist(RE047)
Department(s):
A53-MEDICAL SCHOOL/PEDIATRICS/GENETICS
Employment Class:
Academic Staff-Renewable
Job Number:
The university of wisconsin-madison is an equal opportunity and affirmative action employer..
You will be redirected to the application to launch your career momentarily. Thank you!
Frequently Asked Questions
Applicant Tutorial
Disability Accommodations
Pay Transparency Policy Statement
Refer a Friend
You've sent this job to a friend!
Website feedback, questions or accessibility issues: [email protected] .
Learn more about accessibility at UW–Madison .
© 2016–2024 Board of Regents of the University of Wisconsin System • Privacy Statement
Before You Go..
Would you like to sign-up for job alerts.
Thank you for subscribing to UW–Madison job alerts!
Impact Factor an Index
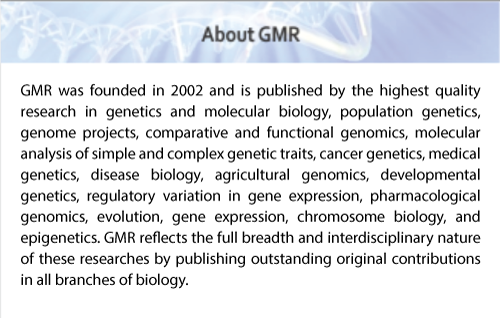
Google scholar citation report
Citations : 56184.
Genetics and Molecular Research received 56184 citations as per google scholar report
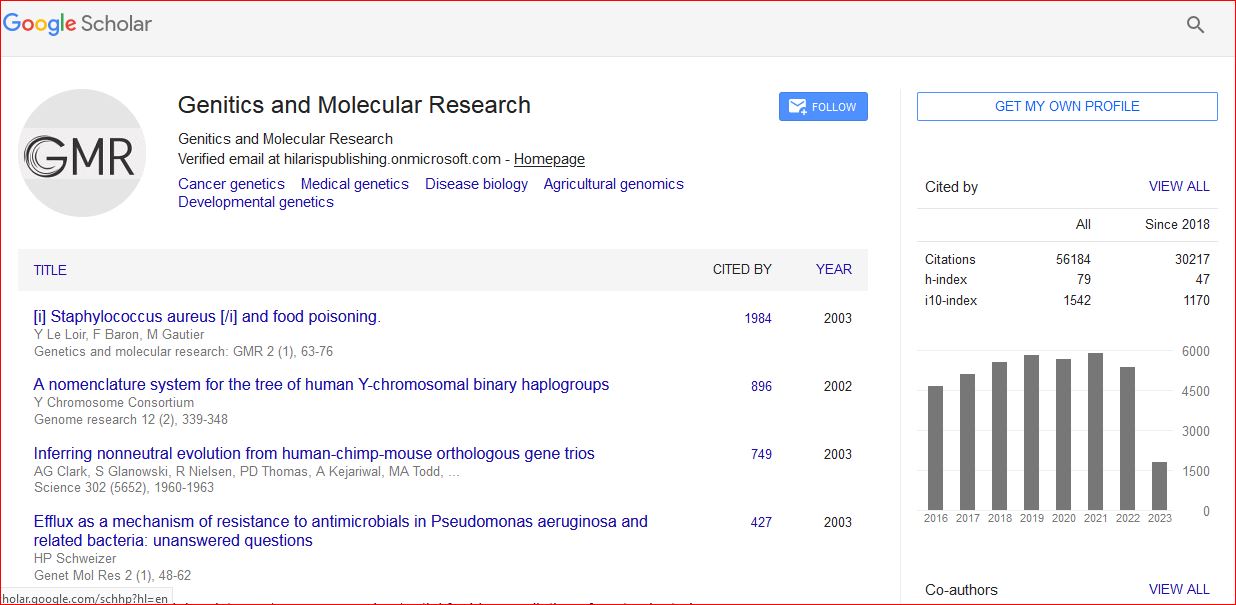
Stay in touch
Publish with gmr.
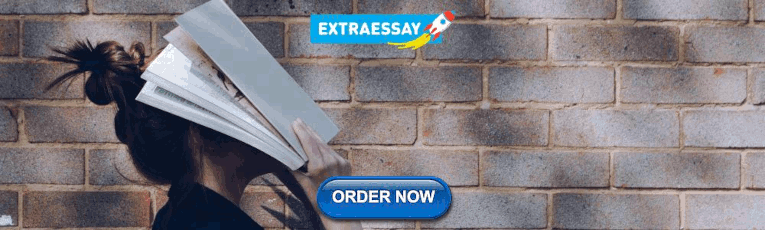
COMMENTS
Genetics and Molecular Research is a peer-reviewed, all-electronic journal that publishes articles on genetics and molecular research. It offers a fast editorial execution and review process (FEE-Review Process) with an additional prepayment of $99.
About the Journal. Genetics and Molecular Research (GMR) publishes high quality research in genetics and molecular biology. GMR reflects the full breadth and interdisciplinary nature of this research by publishing outstanding original contributions in all areas of biology. GMR publishes human studies, as well as research on model organisms ...
Research Article Vol 23, Issue 1, 2024 A Systematic Review of the Relationship between Psychological Stress Protocols and Non-linear Heart Rate Variability E.T. AttarPublished: February 28, 2024Genet.
GMR is a free online journal that publishes original research papers in the areas of genetics, molecular biology, and evolution. Learn about its aims, scope, benefits, indexing, and citation formats.
A peer-reviewed journal covering all areas of genetics and genomics, spanning experimental, theoretical, and synthetic approaches. Publishes research that provides new insights into the fundamental mechanisms underlying genetic and genomic processes, with no publication charges except for special services.
In 1987, the New York Times Magazine characterized the Human Genome Project as the "biggest, costliest, most provocative biomedical research project in history." 2 But in the years between the ...
The Genetics and Molecular Research Journal is a peer-reviewed open-access scientific journal in the fields of biology and medicine, edited and published monthly in the fields of genetics, molecular biology, proteomics, genomics and evolution. The journal was listed on Beall's list before it was taken down in 2017. [1]
Genetics and Molecular Research (GMR) publishes high-quality research in genetics and molecular biology across all areas of biology. GMR covers topics such as gene discovery, function, expression, regulation, evolution, and disease, as well as human and model organism studies.
Research Article Vol 23, Issue 2, 2024 Exploring the Roles of Mitochondrial-Associated MicroRNAs of Head and Neck Cancer Stem Cells in Drug Resistance and Tumor Recurrence Xiaoning Zhu1,2, Sethu Thakachy Subha3, Voon Hoong Fong3, Wee Lin Tan1 and Yoke Kqueen Cheah1,4*Published June 27, 2024Genet.
In celebration of the 20th anniversary of Nature Reviews Genetics, we asked 12 leading researchers to reflect on the key challenges and opportunities faced by the field of genetics and genomics.
Genetics and Molecular Research publishes high quality research in genetics and molecular biology, covering various topics and organisms. It is a peer-reviewed journal maintained by the Research Foundation of Ribeirão Preto, with a low SJR ranking and a high H-index.
VC is a Research Assistant Professor in the Department of Biochemistry and Molecular Genetics at the University of Illinois at Chicago. His expertise in Biochemistry, Molecular Biology, Genetics, Oncology, and Cancer Biology is extensive. He is an invited reviewer for more than fourteen international peer review journals and is the author of ...
Molecular biology articles from across Nature Portfolio. ... Genetic variants in RNU4-2, ... Research Open Access 19 Aug 2024 Nature Communications. Volume: 15, P: 7105 ...
Genetics and Molecular Research(GMR) publishes Book Reviews, Brief Notes, Case Reports, Comments, Corrections, Errata, Homages,In Memoriams, Letters to the Editor, Methodologies, Mini-Reviews, Obituaries, Opinions, Points of View, Research Notes, Retractions, Reviews, Review Articles, Short Communications, and Thesis Abstracts, with consideration for publishing the results of original ...
Molecular genetics is a branch of biology that addresses how differences in the structures or expression of DNA molecules manifests as variation among organisms. Molecular genetics often applies an "investigative approach" to determine the structure and/or function of genes in an organism's genome using genetic screens. [1] [2] The field of study is based on the merging of several sub-fields ...
Managed and made available on the open access platform of Genetics and Molecular Research, these article collections stimulate reader interest and citations for your research. All Research Topic articles are available in the Research Topics section ( LINK) and in the normal online journal issues. The number of article views for each publication ...
Researching Life at the Molecular LevelBiophysicists seek to understand the fundamental processes of life by applying the methods of physics and chemistry to biological systems. Understanding these biological systems and their complex processes requires exquisitely detailed knowledge of molecular structures and molecular functions.
The Impact IF 2022 of Genetics and Molecular Research is 0.42, which is computed in 2023 as per its definition. Genetics and Molecular Research IF is decreased by a factor of 0.18 and approximate percentage change is -30% when compared to preceding year 2021, which shows a falling trend.
Research shows our bodies go through rapid changes in our 40s and our 60s ... a professor of genetics and director of the Center for Genomics and Personalized Medicine at Stanford Medicine ...
To understand the impact of tumor genetic profile on patient outcomes and treatment response, the Multiple Myeloma Research Foundation (MMRF) sponsored the Relating Clinical Outcomes in Multiple ...
Genetics and Molecular Research received 56184 citations as per google scholar report. Stay in touch Stay informed on our latest news! Subscribe. Publish with GMR Submission Informations Submission Guidelines Submit Now. GMR About GMR Editoral Board For Authors Indexers. Contacts. -800-888-1175;
Genetics and Molecular Research (GMR) publishes high quality research in genetics and molecular biology. GMR reflects the full breadth and interdisciplinary nature of this research by publishing outstanding original contributions in all areas of biology. GMR publishes human studies, as well as research on model organisms—from mice and flies ...
Molecular Ecology is an international journal for research using molecular genetic techniques to address questions in ecology, evolution, behavior and conservation. Abstract Globalization has led to the frequent movement of species out of their native habitat. Some of these species become highly invasive and capable of profoundly altering ...
Hosted by the Clinical Research Training Center (CRTC). The Genomics in Medicine Seminar Series offers attendees a practical background in molecular biology and genetics, an introduction to genomics technologies and research, and an understanding of the clinical application of genomics knowledge.. The series is designed for clinicians and other interested persons, including basic science ...
RNA molecules play a vital role in biological processes within the cell, with significant implications for science and medicine. Notably, the biological functions exerted by specific RNA molecules are often linked to the RNA conformational ensemble. However, the experimental characterization of such three-dimensional RNA structures is challenged by the structural heterogeneity of RNA and by ...
Genetics and Molecular Research (GMR) publishes Book Review, Brief Note, Case Report, Comment, Correction, Errata, Homage, In Memoriam, Letter to the Editor, Methodology, Mini-Review, Obituary, Opinion, Point of View, Research Note, Retraction, Review, Review Article, Short Communication, and Thesis Abstract, with regard to publish the results of original experimental researches that contibute ...
Headings. Limit manuscript sections and sub-sections to 3 heading levels. Make sure heading levels are clearly indicated in the manuscript text. Layout. Manuscript text should be double-spaced. Do not format text in multiple columns. Page and line numbers. Include page numbers and line numbers in the manuscript file.
Our lab focuses on studying the genetics of rare disease. Rare diseases collectively affect ~350 million people globally, and ~80% of rare diseases are genetic in origin, about half affect children, many are fatal, and very few have cures. We focus on further study of rare genetic diseases where Dr. Webb identified the molecular etiology.
Genetics and Molecular Research received 56184 citations as per google scholar report. Stay in touch Stay informed on our latest news! Subscribe. Publish with GMR Submission Informations Submission Guidelines Submit Now. GMR About GMR Editoral Board For Authors Indexers. Contacts. -800-888-1175;
All authors are aware and approve of submission of the manuscript, the manuscript's content, their authorship, and the order of authorship. You have the following information for all authors: First name, middle name (s)/initial (s), last name. Work affiliation (s) E-mail address. Any conflicts of interest. Financial disclosure/funding ...