Analytical vs. Descriptive
What's the difference.
Analytical and descriptive are two different approaches used in various fields of study. Analytical refers to the process of breaking down complex ideas or concepts into smaller components to understand their underlying principles or relationships. It involves critical thinking, logical reasoning, and the use of evidence to support arguments or conclusions. On the other hand, descriptive focuses on providing a detailed account or description of a particular phenomenon or event. It aims to present facts, observations, or characteristics without any interpretation or analysis. While analytical aims to uncover the "why" or "how" behind something, descriptive aims to provide a comprehensive picture of what is being studied. Both approaches have their own merits and are often used in combination to gain a deeper understanding of a subject matter.
Attribute | Analytical | Descriptive |
---|---|---|
Definition | Focuses on breaking down complex problems into smaller components and analyzing them individually. | Focuses on describing and summarizing data or phenomena without attempting to explain or analyze them. |
Goal | To understand the underlying causes, relationships, and patterns in data or phenomena. | To provide an accurate and objective description of data or phenomena. |
Approach | Uses logical reasoning, critical thinking, and data analysis techniques. | Relies on observation, measurement, and data collection. |
Focus | Emphasizes on the "why" and "how" questions. | Emphasizes on the "what" questions. |
Subjectivity | Objective approach, minimizing personal bias. | Subjective approach, influenced by personal interpretation. |
Examples | Statistical analysis, data mining, hypothesis testing. | Surveys, observations, case studies. |
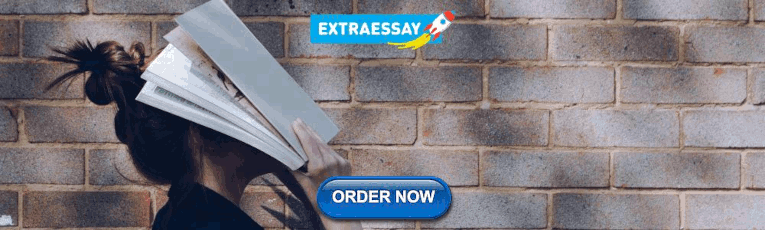
Further Detail
Introduction.
When it comes to research and data analysis, two common approaches are analytical and descriptive methods. Both methods have their own unique attributes and serve different purposes in understanding and interpreting data. In this article, we will explore the characteristics of analytical and descriptive approaches, highlighting their strengths and limitations.
Analytical Approach
The analytical approach focuses on breaking down complex problems or datasets into smaller components to gain a deeper understanding of the underlying patterns and relationships. It involves the use of logical reasoning, critical thinking, and statistical techniques to examine data and draw conclusions. The primary goal of the analytical approach is to uncover insights, identify trends, and make predictions based on the available information.
One of the key attributes of the analytical approach is its emphasis on hypothesis testing. Researchers using this method formulate hypotheses based on existing theories or observations and then collect and analyze data to either support or refute these hypotheses. By systematically testing different variables and their relationships, the analytical approach allows researchers to make evidence-based claims and draw reliable conclusions.
Another important attribute of the analytical approach is its reliance on quantitative data. This method often involves the use of statistical tools and techniques to analyze numerical data, such as surveys, experiments, or large datasets. By quantifying variables and measuring their relationships, the analytical approach provides a rigorous and objective framework for data analysis.
Furthermore, the analytical approach is characterized by its focus on generalizability. Researchers using this method aim to draw conclusions that can be applied to a broader population or context. By using representative samples and statistical inference, the analytical approach allows researchers to make inferences about the larger population based on the analyzed data.
However, it is important to note that the analytical approach has its limitations. It may overlook important contextual factors or qualitative aspects of the data that cannot be easily quantified. Additionally, the analytical approach requires a strong understanding of statistical concepts and techniques, making it more suitable for researchers with a background in quantitative analysis.
Descriptive Approach
The descriptive approach, on the other hand, focuses on summarizing and presenting data in a meaningful and informative way. It aims to provide a clear and concise description of the observed phenomena or variables without necessarily seeking to establish causal relationships or make predictions. The primary goal of the descriptive approach is to present data in a manner that is easily understandable and interpretable.
One of the key attributes of the descriptive approach is its emphasis on data visualization. Researchers using this method often employ charts, graphs, and other visual representations to present data in a visually appealing and accessible manner. By using visual aids, the descriptive approach allows for quick and intuitive understanding of the data, making it suitable for a wide range of audiences.
Another important attribute of the descriptive approach is its flexibility in dealing with different types of data. Unlike the analytical approach, which primarily focuses on quantitative data, the descriptive approach can handle both quantitative and qualitative data. This makes it particularly useful in fields where subjective opinions, narratives, or observations play a significant role.
Furthermore, the descriptive approach is characterized by its attention to detail. Researchers using this method often provide comprehensive descriptions of the variables, including their distribution, central tendency, and variability. By presenting detailed summaries, the descriptive approach allows for a thorough understanding of the data, enabling researchers to identify patterns or trends that may not be immediately apparent.
However, it is important to acknowledge that the descriptive approach has its limitations as well. It may lack the rigor and statistical power of the analytical approach, as it does not involve hypothesis testing or inferential statistics. Additionally, the descriptive approach may be more subjective, as the interpretation of the data relies heavily on the researcher's judgment and perspective.
In conclusion, the analytical and descriptive approaches have distinct attributes that make them suitable for different research purposes. The analytical approach emphasizes hypothesis testing, quantitative data analysis, and generalizability, allowing researchers to draw evidence-based conclusions and make predictions. On the other hand, the descriptive approach focuses on data visualization, flexibility in handling different data types, and attention to detail, enabling researchers to present data in a clear and concise manner. Both approaches have their strengths and limitations, and the choice between them depends on the research objectives, available data, and the researcher's expertise. By understanding the attributes of each approach, researchers can make informed decisions and employ the most appropriate method for their specific research needs.
Comparisons may contain inaccurate information about people, places, or facts. Please report any issues.

Critical Writing 101
Descriptive vs analytical vs critical writing.
By: Derek Jansen (MBA) | Expert Reviewed By: Dr Eunice Rautenbach | April 2017
Across the thousands of students we work with , descriptive writing (as opposed to critical or analytical writing) is an incredibly pervasive problem . In fact, it’s probably the biggest killer of marks in dissertations, theses and research papers . So, in this post, we’ll explain the difference between descriptive and analytical writing in straightforward terms, along with plenty of practical examples.
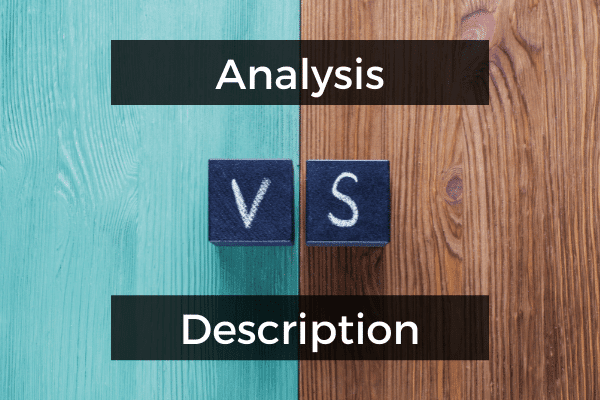
Descriptive vs Analytical Writing
Writing critically is one of the most important skills you’ll need to master for your academic journey, but what exactly does this mean?
Well, when it comes to writing, at least for academic purposes, there are two main types – descriptive writing and critical writing. Critical writing is also sometimes referred to as analytical writing, so we’ll use these two terms interchangeably.
To understand what constitutes critical (or analytical) writing, it’s useful to compare it against its opposite, descriptive writing. At the most basic level, descriptive writing merely communicates the “ what ”, “ where ”, “ when ” or “ who ”. In other words, it describes a thing, place, time or person. It doesn’t consider anything beyond that or explore the situation’s impact, importance or meaning. Here’s an example of a descriptive sentence:
“Yesterday, the president unexpectedly fired the minister of finance.”
As you can see, this sentence just states what happened, when it happened and who was involved. Classic descriptive writing.
Contrasted to this, critical writing takes things a step further and unveils the “ so what? ” – in other words, it explains the impact or consequence of a given situation. Let’s stick with the same event and look at an example of analytical writing:
“The president’s unexpected firing of the well-respected finance minister had an immediate negative impact on investor confidence. This led to a sharp decrease in the value of the local currency, especially against the US dollar. This devaluation means that all dollar-based imports are now expected to rise in cost, thereby raising the cost of living for citizens, and reducing disposable income.”
As you can see in this example, the descriptive version only tells us what happened (the president fired the finance minister), whereas the critical version goes on to discuss some of the impacts of the president’s actions.
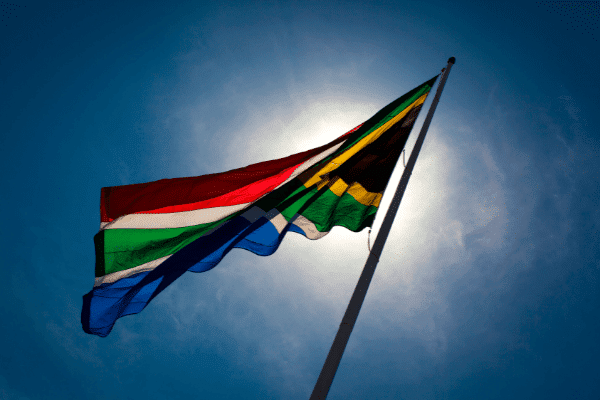
Ideally, critical writing should always link back to the broader objectives of the paper or project, explaining what each thing or event means in relation to those objectives. In a dissertation or thesis, this would involve linking the discussion back to the research aims, objectives and research questions – in other words, the golden thread .
Sounds a bit fluffy and conceptual? Let’s look at an example:
If your research aims involved understanding how the local environment impacts demand for specialty imported vegetables, you would need to explain how the devaluation of the local currency means that the imported vegetables would become more expensive relative to locally farmed options. This in turn would likely have a negative impact on sales, as consumers would turn to cheaper local alternatives.
As you can see, critical (or analytical) writing goes beyond just describing (that’s what descriptive writing covers) and instead focuses on the meaning of things, events or situations, especially in relation to the core research aims and questions.
Need a helping hand?
But wait, there’s more.
This “ what vs so what” distinction is important in understanding the difference between description and analysis, but it is not the only difference – the differences go deeper than this. The table below explains some other key differences between descriptive and analytical writing.
Descriptive Writing | Analytical writing |
---|---|
States what happened (the event). | Explain what the impact of the event was (especially in relation to the research question/s). |
Explains what a theory says. | Explains how this is relevant to the key issue(s) and research question(s). |
Notes the methods used. | Explains whether these methods were relevant or not. |
States what time/date something happened. | Explains why the timing is important/relevant. |
Explains how something works. | Explains whether and why this is positive or negative. |
Provides various pieces of information. | Draws a conclusion in relation to the various pieces of information. |
Should I avoid descriptive writing altogether?
Not quite. For the most part, you’ll need some descriptive writing to lay the foundation for the critical, analytical writing. In other words, you’ll usually need to state the “what” before you can discuss the “so what”. Therefore, description is simply unavoidable and in fact quite essential , but you do want to keep it to a minimum and focus your word count on the analytical side of things.
As you write, a good rule of thumb is to identify every what (in other words, every descriptive point you make) and then check whether it is accompanied by a so what (in other words, a critical conclusion regarding its meaning or impact).
Of course, this won’t always be necessary as some conclusions are fairly obvious and go without saying. But, this basic practice should help you minimise description, maximise analysis, and most importantly, earn you marks!
Let’s recap.
So, the key takeaways for this post are as follows:
- Descriptive writing focuses on the what , while critical/analytical writing focuses on the so what .
- Analytical writing should link the discussion back to the research aims, objectives or research questions (the golden thread).
- Some amount of description will always be needed, but aim to minimise description and maximise analysis to earn higher marks.
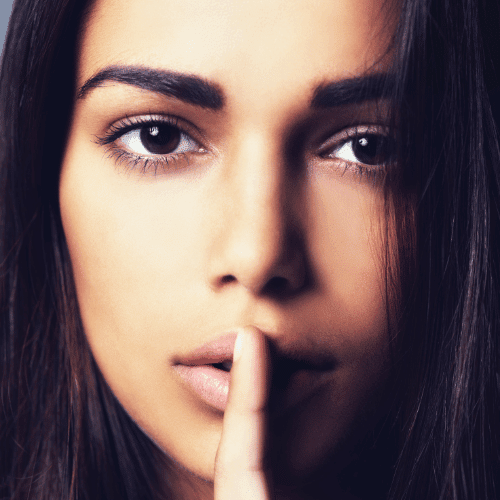
Psst... there’s more!
This post was based on one of our popular Research Bootcamps . If you're working on a research project, you'll definitely want to check this out ...
22 Comments
Thank you so much. This was helpful and a switch from the bad writing habits to the good habits.
Great to hear that, Sarah. Glad you found it useful!
I am currently working on my Masters Thesis and found this extremely informative and helpful. Thank you kindly.
I’m currently a University student and this is so helpful. Thank you.
It really helped me to get the exact meaning of analytical writing. Differences between the two explains it well
Thank you! this was very useful
With much appreciation, I say thank you. Your explanations are down to earth. It has been helpful.
Very helpful towards my theses journey! Many thanks 👍
very helpful
very helpful indeed
Thanks Derek for the useful coaching
Thank you for sharing this. I was stuck on descriptive now I can do my corrections. Thank you.
I was struggling to differentiate between descriptive and analytical writing. I googled and found this as it is so helpful. Thank you for sharing.
I am glad to see this differences of descriptive against analytical writing. This is going to improve my masters dissertation
Thanks in deed. It was helpful
Thank you so much. I’m now better informed
Busy with MBA in South Africa, this is very helpful as most of the writing requires one to expound on the topics. thanks for this, it’s a salvation from watching the blinking cursor for hours while figuring out what to write to hit the 5000 word target 😂
It’s been fantastic and enriching. Thanks a lot, GRAD COACH.
Wonderful explanation of descriptive vs analytic writing with examples. This is going to be greatly helpful for me as I am writing my thesis at the moment. Thank you Grad Coach. I follow your YouTube videos and subscribed and liked every time I watch one.
Very useful piece. thanks
Brilliantly explained – thank you.
Submit a Comment Cancel reply
Your email address will not be published. Required fields are marked *
Save my name, email, and website in this browser for the next time I comment.
- Print Friendly
- En español – ExME
- Em português – EME
An introduction to different types of study design
Posted on 6th April 2021 by Hadi Abbas
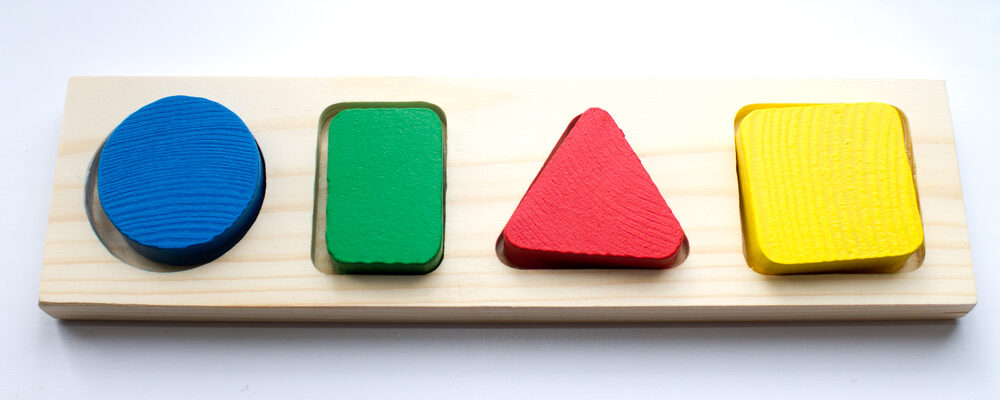
Study designs are the set of methods and procedures used to collect and analyze data in a study.
Broadly speaking, there are 2 types of study designs: descriptive studies and analytical studies.
Descriptive studies
- Describes specific characteristics in a population of interest
- The most common forms are case reports and case series
- In a case report, we discuss our experience with the patient’s symptoms, signs, diagnosis, and treatment
- In a case series, several patients with similar experiences are grouped.
Analytical Studies
Analytical studies are of 2 types: observational and experimental.
Observational studies are studies that we conduct without any intervention or experiment. In those studies, we purely observe the outcomes. On the other hand, in experimental studies, we conduct experiments and interventions.
Observational studies
Observational studies include many subtypes. Below, I will discuss the most common designs.
Cross-sectional study:
- This design is transverse where we take a specific sample at a specific time without any follow-up
- It allows us to calculate the frequency of disease ( p revalence ) or the frequency of a risk factor
- This design is easy to conduct
- For example – if we want to know the prevalence of migraine in a population, we can conduct a cross-sectional study whereby we take a sample from the population and calculate the number of patients with migraine headaches.
Cohort study:
- We conduct this study by comparing two samples from the population: one sample with a risk factor while the other lacks this risk factor
- It shows us the risk of developing the disease in individuals with the risk factor compared to those without the risk factor ( RR = relative risk )
- Prospective : we follow the individuals in the future to know who will develop the disease
- Retrospective : we look to the past to know who developed the disease (e.g. using medical records)
- This design is the strongest among the observational studies
- For example – to find out the relative risk of developing chronic obstructive pulmonary disease (COPD) among smokers, we take a sample including smokers and non-smokers. Then, we calculate the number of individuals with COPD among both.
Case-Control Study:
- We conduct this study by comparing 2 groups: one group with the disease (cases) and another group without the disease (controls)
- This design is always retrospective
- We aim to find out the odds of having a risk factor or an exposure if an individual has a specific disease (Odds ratio)
- Relatively easy to conduct
- For example – we want to study the odds of being a smoker among hypertensive patients compared to normotensive ones. To do so, we choose a group of patients diagnosed with hypertension and another group that serves as the control (normal blood pressure). Then we study their smoking history to find out if there is a correlation.
Experimental Studies
- Also known as interventional studies
- Can involve animals and humans
- Pre-clinical trials involve animals
- Clinical trials are experimental studies involving humans
- In clinical trials, we study the effect of an intervention compared to another intervention or placebo. As an example, I have listed the four phases of a drug trial:
I: We aim to assess the safety of the drug ( is it safe ? )
II: We aim to assess the efficacy of the drug ( does it work ? )
III: We want to know if this drug is better than the old treatment ( is it better ? )
IV: We follow-up to detect long-term side effects ( can it stay in the market ? )
- In randomized controlled trials, one group of participants receives the control, while the other receives the tested drug/intervention. Those studies are the best way to evaluate the efficacy of a treatment.
Finally, the figure below will help you with your understanding of different types of study designs.
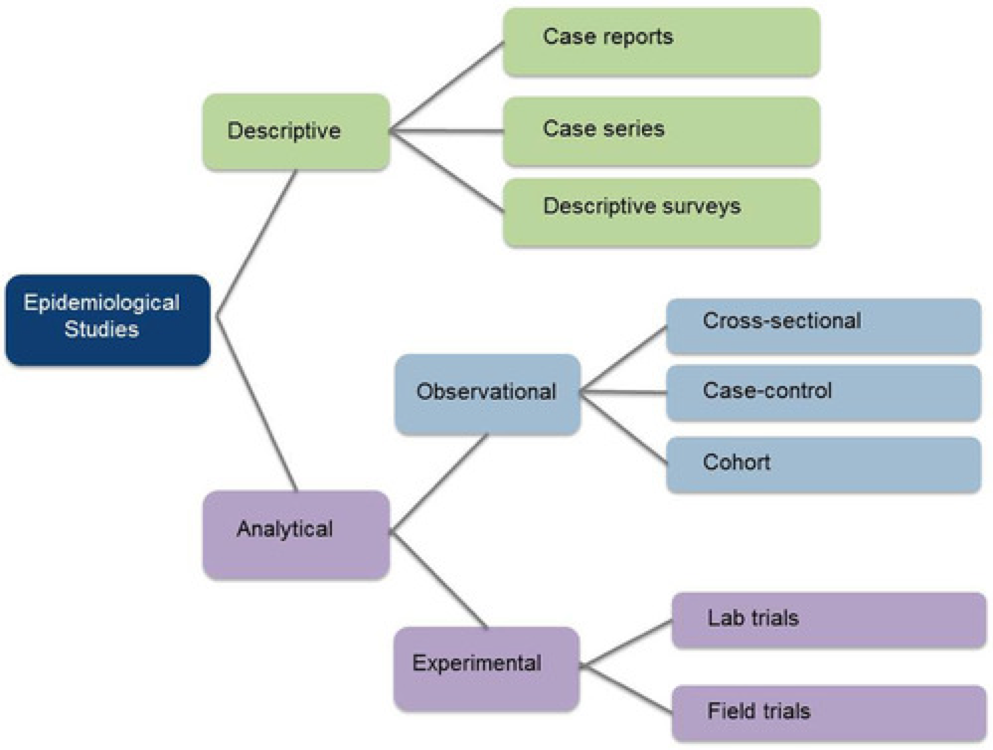
References (pdf)
You may also be interested in the following blogs for further reading:
An introduction to randomized controlled trials
Case-control and cohort studies: a brief overview
Cohort studies: prospective and retrospective designs
Prevalence vs Incidence: what is the difference?
Leave a Reply Cancel reply
Your email address will not be published. Required fields are marked *
Save my name, email, and website in this browser for the next time I comment.
No Comments on An introduction to different types of study design
you are amazing one!! if I get you I’m working with you! I’m student from Ethiopian higher education. health sciences student
Very informative and easy understandable
You are my kind of doctor. Do not lose sight of your objective.
Wow very erll explained and easy to understand
I’m Khamisu Habibu community health officer student from Abubakar Tafawa Balewa university teaching hospital Bauchi, Nigeria, I really appreciate your write up and you have make it clear for the learner. thank you
well understood,thank you so much
Well understood…thanks
Simply explained. Thank You.
Thanks a lot for this nice informative article which help me to understand different study designs that I felt difficult before
That’s lovely to hear, Mona, thank you for letting the author know how useful this was. If there are any other particular topics you think would be useful to you, and are not already on the website, please do let us know.
it is very informative and useful.
thank you statistician
Fabulous to hear, thank you John.
Thanks for this information
Thanks so much for this information….I have clearly known the types of study design Thanks
That’s so good to hear, Mirembe, thank you for letting the author know.
Very helpful article!! U have simplified everything for easy understanding
I’m a health science major currently taking statistics for health care workers…this is a challenging class…thanks for the simified feedback.
That’s good to hear this has helped you. Hopefully you will find some of the other blogs useful too. If you see any topics that are missing from the website, please do let us know!
Hello. I liked your presentation, the fact that you ranked them clearly is very helpful to understand for people like me who is a novelist researcher. However, I was expecting to read much more about the Experimental studies. So please direct me if you already have or will one day. Thank you
Dear Ay. My sincere apologies for not responding to your comment sooner. You may find it useful to filter the blogs by the topic of ‘Study design and research methods’ – here is a link to that filter: https://s4be.cochrane.org/blog/topic/study-design/ This will cover more detail about experimental studies. Or have a look on our library page for further resources there – you’ll find that on the ‘Resources’ drop down from the home page.
However, if there are specific things you feel you would like to learn about experimental studies, that are missing from the website, it would be great if you could let me know too. Thank you, and best of luck. Emma
Great job Mr Hadi. I advise you to prepare and study for the Australian Medical Board Exams as soon as you finish your undergrad study in Lebanon. Good luck and hope we can meet sometime in the future. Regards ;)
You have give a good explaination of what am looking for. However, references am not sure of where to get them from.
Subscribe to our newsletter
You will receive our monthly newsletter and free access to Trip Premium.
Related Articles
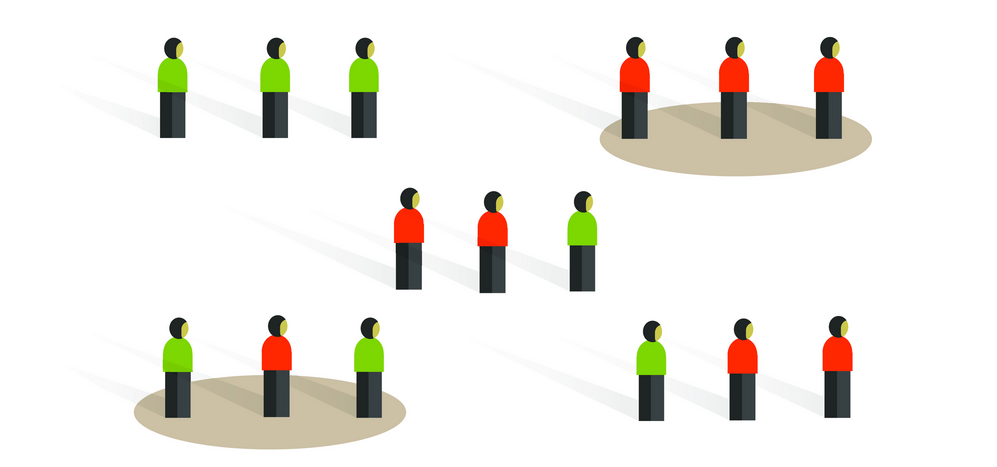
Cluster Randomized Trials: Concepts
This blog summarizes the concepts of cluster randomization, and the logistical and statistical considerations while designing a cluster randomized controlled trial.
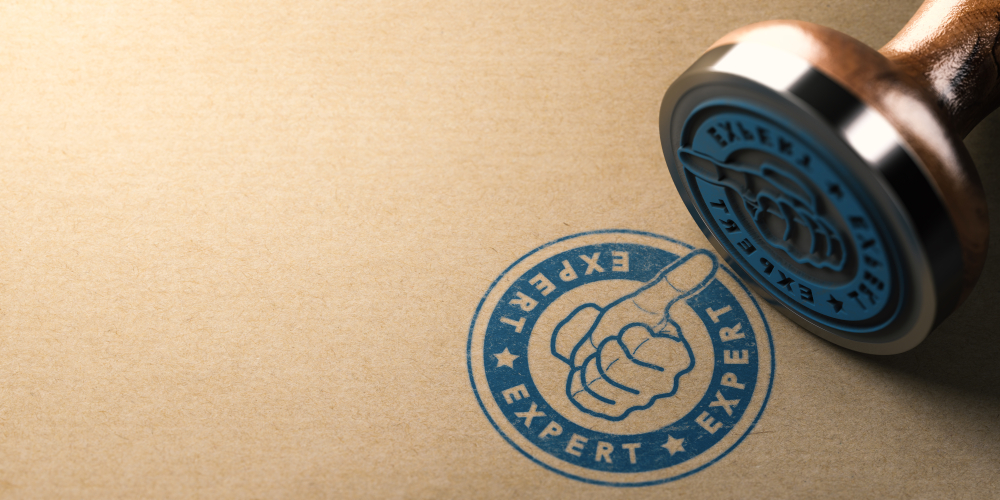
Expertise-based Randomized Controlled Trials
This blog summarizes the concepts of Expertise-based randomized controlled trials with a focus on the advantages and challenges associated with this type of study.
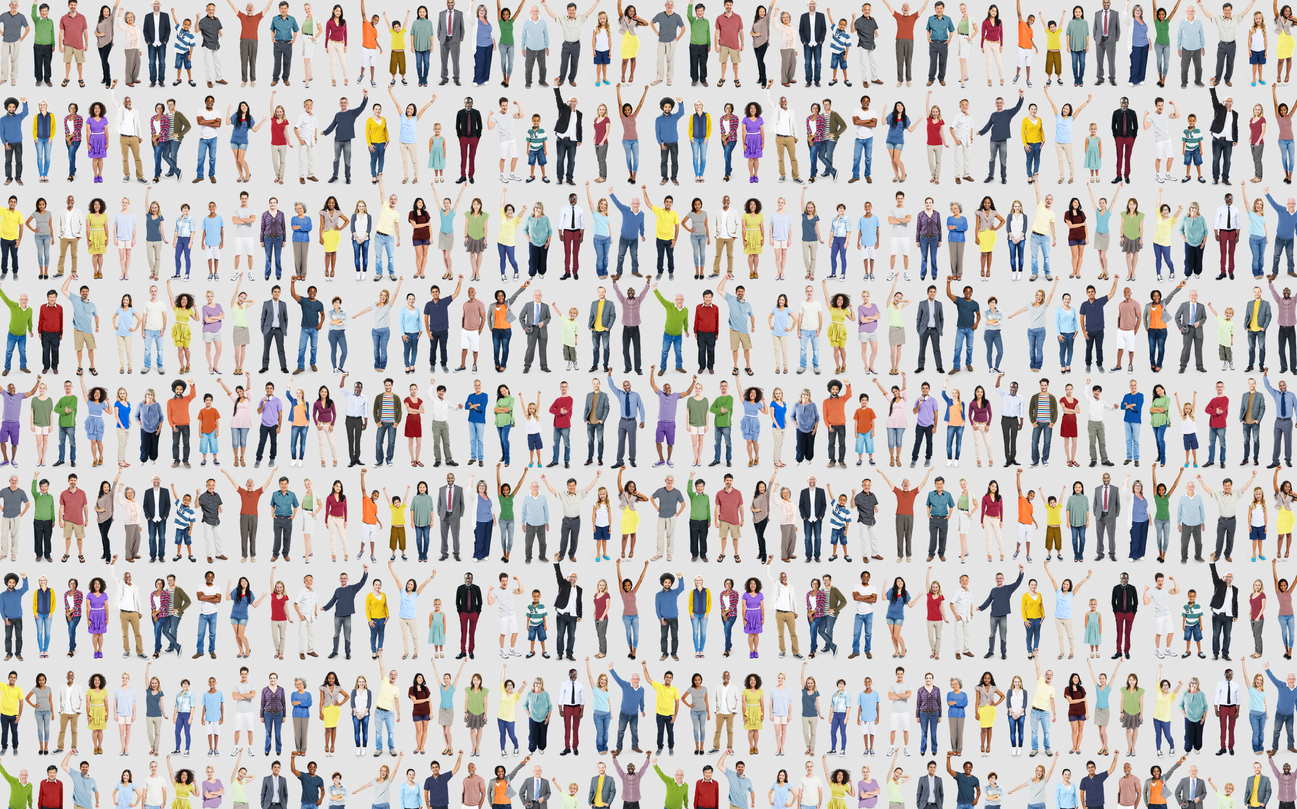
A well-designed cohort study can provide powerful results. This blog introduces prospective and retrospective cohort studies, discussing the advantages, disadvantages and use of these type of study designs.

Descriptive vs. Analytical Research in Sociology: A Comparative Study

Table of Contents
When we delve into the world of research, particularly in fields like sociology , we encounter a myriad of methods designed to uncover the layers of human society and behavior. Two of the most fundamental research methods are descriptive and analytical research . Each plays a crucial role in understanding our world, but they do so in distinctly different ways. So, what exactly are these methods, and how do they compare when applied in the realm of social studies? Let’s embark on a comparative journey to understand these methodologies better.
Understanding Descriptive Research
Descriptive research is akin to the meticulous work of an artist attempting to capture the intricate details of a landscape. It aims to accurately describe the characteristics of a particular population or phenomenon. By painting a picture of the ‘what’ aspect, this method helps researchers to understand the prevalence of certain attributes, behaviors, or issues within a group.
Key Features of Descriptive Research
- Snapshot in time: It often involves studying a single point or period, providing a snapshot rather than a motion picture.
- Surveys and observations : Common tools include surveys , observations, and case studies .
- Quantitative data: It leans heavily on quantitative data to present findings in numerical format.
- No hypothesis testing: Unlike other research types, it doesn’t typically involve hypothesis testing.
When to Use Descriptive Research
- Establishing a baseline : When there’s a need to set a reference point for future studies or track changes over time.
- Exploratory purposes: When little is known about a topic and there’s a need to gather initial information that could inform future research.
- Policy-making: When organizations or government bodies need factual data to inform decisions and policies.
Exploring Analytical Research
On the flip side, analytical research steps beyond mere description to explore the ‘why’ and ‘how’. It’s like a detective piecing together clues to not just recount events, but to understand the relationships and causations behind them. Analytical researchers critically evaluate information to draw conclusions and generalizations that extend beyond the immediate data.
Key Characteristics of Analytical Research
- Critical evaluation: It involves a deep analysis of the available information to form judgments.
- Qualitative and quantitative data: Uses both numerical data and non-numerical data for a more comprehensive analysis.
- Hypothesis-driven: This method often starts with a hypothesis that the research is designed to test.
- Seeking patterns : Aims to identify patterns, relationships, and causations.
When to Opt for Analytical Research
- Understanding complexities: When the research question is complex and requires understanding the interplay of various factors.
- Building upon previous research: When expanding on existing knowledge or challenging prevailing theories.
- Recommendations for action: When research is aimed at providing actionable insights or solutions to problems.
Comparing Descriptive and Analytical Research in Real-World Scenarios
Imagine a sociologist aiming to tackle a pressing social issue, such as the dynamics of homelessness in urban areas. Descriptive research would enable them to establish the scale and scope of homelessness, identifying key demographics and patterns. Analytical research, however, would take these findings and probe deeper into the causes, examining the social, economic, and political factors that contribute to the situation and what can be done to alleviate it.
Advantages and Limitations
Each research type has its own set of strengths and weaknesses. Descriptive research is powerful for mapping out the landscape but may fall short in explaining the underlying reasons for observed phenomena. Analytical research, with its depth, can provide those explanations, but it may be more time-consuming and complex to conduct.
Choosing the Right Approach
Deciding between descriptive and analytical research often comes down to the specific objectives of the study. It’s not uncommon for researchers to employ both methods within the same broader research project to maximize their understanding of a topic.
In conclusion, descriptive and analytical research are two sides of the same coin, offering different lenses through which we can view and interpret the intricacies of social phenomena. By understanding their distinctions and applications, researchers can better design studies that yield rich, actionable insights into the fabric of society.
What do you think? Could a blend of both descriptive and analytical research provide a more holistic understanding of social issues? Are there situations where one method is clearly preferable over the other?
Submit a Comment Cancel reply
Your email address will not be published. Required fields are marked *
Save my name, email, and website in this browser for the next time I comment.
Submit Comment
Research Methodologies & Methods
1 Logic of Inquiry in Social Research
- A Science of Society
- Comte’s Ideas on the Nature of Sociology
- Observation in Social Sciences
- Logical Understanding of Social Reality
2 Empirical Approach
- Empirical Approach
- Rules of Data Collection
- Cultural Relativism
- Problems Encountered in Data Collection
- Difference between Common Sense and Science
- What is Ethical?
- What is Normal?
- Understanding the Data Collected
- Managing Diversities in Social Research
- Problematising the Object of Study
- Conclusion: Return to Good Old Empirical Approach
3 Diverse Logic of Theory Building
- Concern with Theory in Sociology
- Concepts: Basic Elements of Theories
- Why Do We Need Theory?
- Hypothesis Description and Experimentation
- Controlled Experiment
- Designing an Experiment
- How to Test a Hypothesis
- Sensitivity to Alternative Explanations
- Rival Hypothesis Construction
- The Use and Scope of Social Science Theory
- Theory Building and Researcher’s Values
4 Theoretical Analysis
- Premises of Evolutionary and Functional Theories
- Critique of Evolutionary and Functional Theories
- Turning away from Functionalism
- What after Functionalism
- Post-modernism
- Trends other than Post-modernism
5 Issues of Epistemology
- Some Major Concerns of Epistemology
- Rationalism
- Phenomenology: Bracketing Experience
6 Philosophy of Social Science
- Foundations of Science
- Science, Modernity, and Sociology
- Rethinking Science
- Crisis in Foundation
7 Positivism and its Critique
- Heroic Science and Origin of Positivism
- Early Positivism
- Consolidation of Positivism
- Critiques of Positivism
8 Hermeneutics
- Methodological Disputes in the Social Sciences
- Tracing the History of Hermeneutics
- Hermeneutics and Sociology
- Philosophical Hermeneutics
- The Hermeneutics of Suspicion
- Phenomenology and Hermeneutics
9 Comparative Method
- Relationship with Common Sense; Interrogating Ideological Location
- The Historical Context
- Elements of the Comparative Approach
10 Feminist Approach
- Features of the Feminist Method
- Feminist Methods adopt the Reflexive Stance
- Feminist Discourse in India
11 Participatory Method
- Delineation of Key Features
12 Types of Research
- Basic and Applied Research
- Descriptive and Analytical Research
- Empirical and Exploratory Research
- Quantitative and Qualitative Research
- Explanatory (Causal) and Longitudinal Research
- Experimental and Evaluative Research
- Participatory Action Research
13 Methods of Research
- Evolutionary Method
- Comparative Method
- Historical Method
- Personal Documents
14 Elements of Research Design
- Structuring the Research Process
15 Sampling Methods and Estimation of Sample Size
- Classification of Sampling Methods
- Sample Size
16 Measures of Central Tendency
- Relationship between Mean, Mode, and Median
- Choosing a Measure of Central Tendency
17 Measures of Dispersion and Variability
- The Variance
- The Standard Deviation
- Coefficient of Variation
18 Statistical Inference- Tests of Hypothesis
- Statistical Inference
- Tests of Significance
19 Correlation and Regression
- Correlation
- Method of Calculating Correlation of Ungrouped Data
- Method Of Calculating Correlation Of Grouped Data
20 Survey Method
- Rationale of Survey Research Method
- History of Survey Research
- Defining Survey Research
- Sampling and Survey Techniques
- Operationalising Survey Research Tools
- Advantages and Weaknesses of Survey Research
21 Survey Design
- Preliminary Considerations
- Stages / Phases in Survey Research
- Formulation of Research Question
- Survey Research Designs
- Sampling Design
22 Survey Instrumentation
- Techniques/Instruments for Data Collection
- Questionnaire Construction
- Issues in Designing a Survey Instrument
23 Survey Execution and Data Analysis
- Problems and Issues in Executing Survey Research
- Data Analysis
- Ethical Issues in Survey Research
24 Field Research – I
- History of Field Research
- Ethnography
- Theme Selection
- Gaining Entry in the Field
- Key Informants
- Participant Observation
25 Field Research – II
- Interview its Types and Process
- Feminist and Postmodernist Perspectives on Interviewing
- Narrative Analysis
- Interpretation
- Case Study and its Types
- Life Histories
- Oral History
- PRA and RRA Techniques
26 Reliability, Validity and Triangulation
- Concepts of Reliability and Validity
- Three Types of “Reliability”
- Working Towards Reliability
- Procedural Validity
- Field Research as a Validity Check
- Method Appropriate Criteria
- Triangulation
- Ethical Considerations in Qualitative Research
27 Qualitative Data Formatting and Processing
- Qualitative Data Processing and Analysis
- Description
- Classification
- Making Connections
- Theoretical Coding
- Qualitative Content Analysis
28 Writing up Qualitative Data
- Problems of Writing Up
- Grasp and Then Render
- “Writing Down” and “Writing Up”
- Write Early
- Writing Styles
- First Draft
29 Using Internet and Word Processor
- What is Internet and How Does it Work?
- Internet Services
- Searching on the Web: Search Engines
- Accessing and Using Online Information
- Online Journals and Texts
- Statistical Reference Sites
- Data Sources
- Uses of E-mail Services in Research
30 Using SPSS for Data Analysis Contents
- Introduction
- Starting and Exiting SPSS
- Creating a Data File
- Univariate Analysis
- Bivariate Analysis
31 Using SPSS in Report Writing
- Why to Use SPSS
- Working with SPSS Output
- Copying SPSS Output to MS Word Document
32 Tabulation and Graphic Presentation- Case Studies
- Structure for Presentation of Research Findings
- Data Presentation: Editing, Coding, and Transcribing
- Case Studies
- Qualitative Data Analysis and Presentation through Software
- Types of ICT used for Research
33 Guidelines to Research Project Assignment
- Overview of Research Methodologies and Methods (MSO 002)
- Research Project Objectives
- Preparation for Research Project
- Stages of the Research Project
- Supervision During the Research Project
- Submission of Research Project
- Methodology for Evaluating Research Project
Share on Mastodon
Educational resources and simple solutions for your research journey
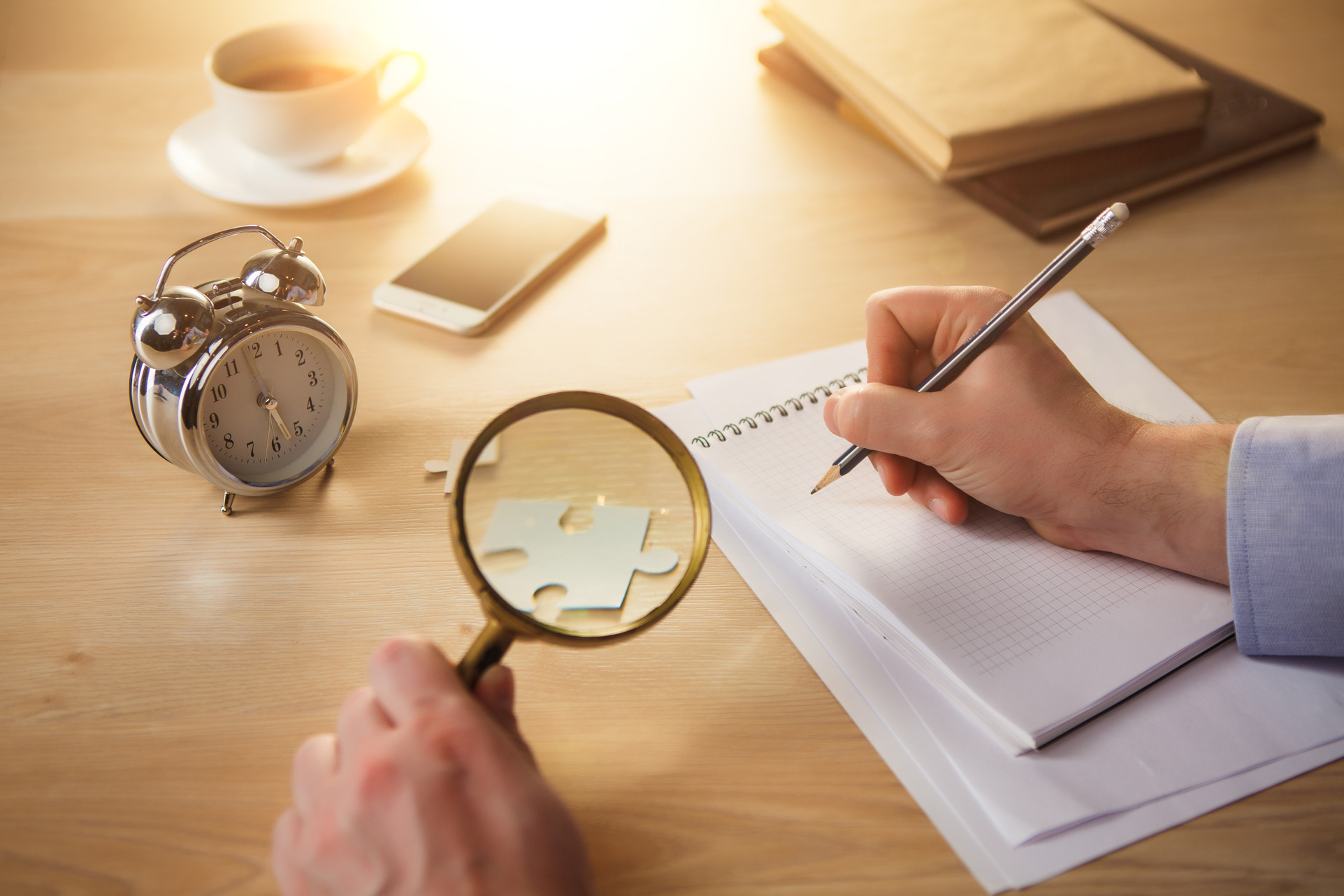
What is Descriptive Research? Definition, Methods, Types and Examples
Descriptive research is a methodological approach that seeks to depict the characteristics of a phenomenon or subject under investigation. In scientific inquiry, it serves as a foundational tool for researchers aiming to observe, record, and analyze the intricate details of a particular topic. This method provides a rich and detailed account that aids in understanding, categorizing, and interpreting the subject matter.
Descriptive research design is widely employed across diverse fields, and its primary objective is to systematically observe and document all variables and conditions influencing the phenomenon.
After this descriptive research definition, let’s look at this example. Consider a researcher working on climate change adaptation, who wants to understand water management trends in an arid village in a specific study area. She must conduct a demographic survey of the region, gather population data, and then conduct descriptive research on this demographic segment. The study will then uncover details on “what are the water management practices and trends in village X.” Note, however, that it will not cover any investigative information about “why” the patterns exist.
Table of Contents
What is descriptive research?
If you’ve been wondering “What is descriptive research,” we’ve got you covered in this post! In a nutshell, descriptive research is an exploratory research method that helps a researcher describe a population, circumstance, or phenomenon. It can help answer what , where , when and how questions, but not why questions. In other words, it does not involve changing the study variables and does not seek to establish cause-and-effect relationships.

Importance of descriptive research
Now, let’s delve into the importance of descriptive research. This research method acts as the cornerstone for various academic and applied disciplines. Its primary significance lies in its ability to provide a comprehensive overview of a phenomenon, enabling researchers to gain a nuanced understanding of the variables at play. This method aids in forming hypotheses, generating insights, and laying the groundwork for further in-depth investigations. The following points further illustrate its importance:
Provides insights into a population or phenomenon: Descriptive research furnishes a comprehensive overview of the characteristics and behaviors of a specific population or phenomenon, thereby guiding and shaping the research project.
Offers baseline data: The data acquired through this type of research acts as a reference for subsequent investigations, laying the groundwork for further studies.
Allows validation of sampling methods: Descriptive research validates sampling methods, aiding in the selection of the most effective approach for the study.
Helps reduce time and costs: It is cost-effective and time-efficient, making this an economical means of gathering information about a specific population or phenomenon.
Ensures replicability: Descriptive research is easily replicable, ensuring a reliable way to collect and compare information from various sources.
When to use descriptive research design?
Determining when to use descriptive research depends on the nature of the research question. Before diving into the reasons behind an occurrence, understanding the how, when, and where aspects is essential. Descriptive research design is a suitable option when the research objective is to discern characteristics, frequencies, trends, and categories without manipulating variables. It is therefore often employed in the initial stages of a study before progressing to more complex research designs. To put it in another way, descriptive research precedes the hypotheses of explanatory research. It is particularly valuable when there is limited existing knowledge about the subject.
Some examples are as follows, highlighting that these questions would arise before a clear outline of the research plan is established:
- In the last two decades, what changes have occurred in patterns of urban gardening in Mumbai?
- What are the differences in climate change perceptions of farmers in coastal versus inland villages in the Philippines?
Characteristics of descriptive research
Coming to the characteristics of descriptive research, this approach is characterized by its focus on observing and documenting the features of a subject. Specific characteristics are as below.
- Quantitative nature: Some descriptive research types involve quantitative research methods to gather quantifiable information for statistical analysis of the population sample.
- Qualitative nature: Some descriptive research examples include those using the qualitative research method to describe or explain the research problem.
- Observational nature: This approach is non-invasive and observational because the study variables remain untouched. Researchers merely observe and report, without introducing interventions that could impact the subject(s).
- Cross-sectional nature: In descriptive research, different sections belonging to the same group are studied, providing a “snapshot” of sorts.
- Springboard for further research: The data collected are further studied and analyzed using different research techniques. This approach helps guide the suitable research methods to be employed.
Types of descriptive research
There are various descriptive research types, each suited to different research objectives. Take a look at the different types below.
- Surveys: This involves collecting data through questionnaires or interviews to gather qualitative and quantitative data.
- Observational studies: This involves observing and collecting data on a particular population or phenomenon without influencing the study variables or manipulating the conditions. These may be further divided into cohort studies, case studies, and cross-sectional studies:
- Cohort studies: Also known as longitudinal studies, these studies involve the collection of data over an extended period, allowing researchers to track changes and trends.
- Case studies: These deal with a single individual, group, or event, which might be rare or unusual.
- Cross-sectional studies : A researcher collects data at a single point in time, in order to obtain a snapshot of a specific moment.
- Focus groups: In this approach, a small group of people are brought together to discuss a topic. The researcher moderates and records the group discussion. This can also be considered a “participatory” observational method.
- Descriptive classification: Relevant to the biological sciences, this type of approach may be used to classify living organisms.
Descriptive research methods
Several descriptive research methods can be employed, and these are more or less similar to the types of approaches mentioned above.
- Surveys: This method involves the collection of data through questionnaires or interviews. Surveys may be done online or offline, and the target subjects might be hyper-local, regional, or global.
- Observational studies: These entail the direct observation of subjects in their natural environment. These include case studies, dealing with a single case or individual, as well as cross-sectional and longitudinal studies, for a glimpse into a population or changes in trends over time, respectively. Participatory observational studies such as focus group discussions may also fall under this method.
Researchers must carefully consider descriptive research methods, types, and examples to harness their full potential in contributing to scientific knowledge.
Examples of descriptive research
Now, let’s consider some descriptive research examples.
- In social sciences, an example could be a study analyzing the demographics of a specific community to understand its socio-economic characteristics.
- In business, a market research survey aiming to describe consumer preferences would be a descriptive study.
- In ecology, a researcher might undertake a survey of all the types of monocots naturally occurring in a region and classify them up to species level.
These examples showcase the versatility of descriptive research across diverse fields.
Advantages of descriptive research
There are several advantages to this approach, which every researcher must be aware of. These are as follows:
- Owing to the numerous descriptive research methods and types, primary data can be obtained in diverse ways and be used for developing a research hypothesis .
- It is a versatile research method and allows flexibility.
- Detailed and comprehensive information can be obtained because the data collected can be qualitative or quantitative.
- It is carried out in the natural environment, which greatly minimizes certain types of bias and ethical concerns.
- It is an inexpensive and efficient approach, even with large sample sizes
Disadvantages of descriptive research
On the other hand, this design has some drawbacks as well:
- It is limited in its scope as it does not determine cause-and-effect relationships.
- The approach does not generate new information and simply depends on existing data.
- Study variables are not manipulated or controlled, and this limits the conclusions to be drawn.
- Descriptive research findings may not be generalizable to other populations.
- Finally, it offers a preliminary understanding rather than an in-depth understanding.
To reiterate, the advantages of descriptive research lie in its ability to provide a comprehensive overview, aid hypothesis generation, and serve as a preliminary step in the research process. However, its limitations include a potential lack of depth, inability to establish cause-and-effect relationships, and susceptibility to bias.
Frequently asked questions
When should researchers conduct descriptive research.
Descriptive research is most appropriate when researchers aim to portray and understand the characteristics of a phenomenon without manipulating variables. It is particularly valuable in the early stages of a study.
What is the difference between descriptive and exploratory research?
Descriptive research focuses on providing a detailed depiction of a phenomenon, while exploratory research aims to explore and generate insights into an issue where little is known.
What is the difference between descriptive and experimental research?
Descriptive research observes and documents without manipulating variables, whereas experimental research involves intentional interventions to establish cause-and-effect relationships.
Is descriptive research only for social sciences?
No, various descriptive research types may be applicable to all fields of study, including social science, humanities, physical science, and biological science.
How important is descriptive research?
The importance of descriptive research lies in its ability to provide a glimpse of the current state of a phenomenon, offering valuable insights and establishing a basic understanding. Further, the advantages of descriptive research include its capacity to offer a straightforward depiction of a situation or phenomenon, facilitate the identification of patterns or trends, and serve as a useful starting point for more in-depth investigations. Additionally, descriptive research can contribute to the development of hypotheses and guide the formulation of research questions for subsequent studies.
Editage All Access is a subscription-based platform that unifies the best AI tools and services designed to speed up, simplify, and streamline every step of a researcher’s journey. The Editage All Access Pack is a one-of-a-kind subscription that unlocks full access to an AI writing assistant, literature recommender, journal finder, scientific illustration tool, and exclusive discounts on professional publication services from Editage.
Based on 22+ years of experience in academia, Editage All Access empowers researchers to put their best research forward and move closer to success. Explore our top AI Tools pack, AI Tools + Publication Services pack, or Build Your Own Plan. Find everything a researcher needs to succeed, all in one place – Get All Access now starting at just $14 a month !
Related Posts

Join Us for Peer Review Week 2024

How Editage All Access is Boosting Productivity for Academics in India
Have a language expert improve your writing
Run a free plagiarism check in 10 minutes, generate accurate citations for free.
- Knowledge Base
Methodology
- Descriptive Research | Definition, Types, Methods & Examples
Descriptive Research | Definition, Types, Methods & Examples
Published on May 15, 2019 by Shona McCombes . Revised on June 22, 2023.
Descriptive research aims to accurately and systematically describe a population, situation or phenomenon. It can answer what , where , when and how questions , but not why questions.
A descriptive research design can use a wide variety of research methods to investigate one or more variables . Unlike in experimental research , the researcher does not control or manipulate any of the variables, but only observes and measures them.
Table of contents
When to use a descriptive research design, descriptive research methods, other interesting articles.
Descriptive research is an appropriate choice when the research aim is to identify characteristics, frequencies, trends, and categories.
It is useful when not much is known yet about the topic or problem. Before you can research why something happens, you need to understand how, when and where it happens.
Descriptive research question examples
- How has the Amsterdam housing market changed over the past 20 years?
- Do customers of company X prefer product X or product Y?
- What are the main genetic, behavioural and morphological differences between European wildcats and domestic cats?
- What are the most popular online news sources among under-18s?
- How prevalent is disease A in population B?
Receive feedback on language, structure, and formatting
Professional editors proofread and edit your paper by focusing on:
- Academic style
- Vague sentences
- Style consistency
See an example
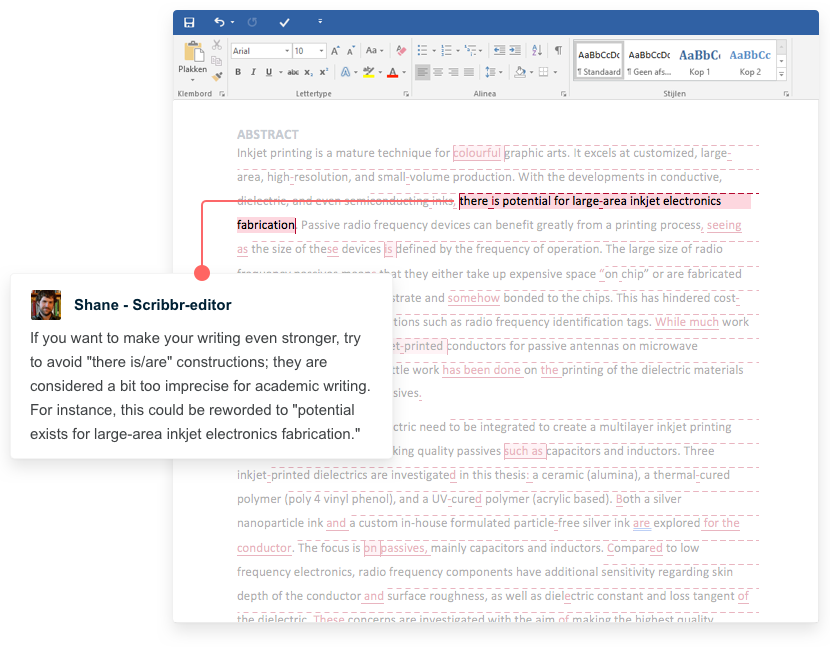
Descriptive research is usually defined as a type of quantitative research , though qualitative research can also be used for descriptive purposes. The research design should be carefully developed to ensure that the results are valid and reliable .
Survey research allows you to gather large volumes of data that can be analyzed for frequencies, averages and patterns. Common uses of surveys include:
- Describing the demographics of a country or region
- Gauging public opinion on political and social topics
- Evaluating satisfaction with a company’s products or an organization’s services
Observations
Observations allow you to gather data on behaviours and phenomena without having to rely on the honesty and accuracy of respondents. This method is often used by psychological, social and market researchers to understand how people act in real-life situations.
Observation of physical entities and phenomena is also an important part of research in the natural sciences. Before you can develop testable hypotheses , models or theories, it’s necessary to observe and systematically describe the subject under investigation.
Case studies
A case study can be used to describe the characteristics of a specific subject (such as a person, group, event or organization). Instead of gathering a large volume of data to identify patterns across time or location, case studies gather detailed data to identify the characteristics of a narrowly defined subject.
Rather than aiming to describe generalizable facts, case studies often focus on unusual or interesting cases that challenge assumptions, add complexity, or reveal something new about a research problem .
If you want to know more about statistics , methodology , or research bias , make sure to check out some of our other articles with explanations and examples.
- Normal distribution
- Degrees of freedom
- Null hypothesis
- Discourse analysis
- Control groups
- Mixed methods research
- Non-probability sampling
- Quantitative research
- Ecological validity
Research bias
- Rosenthal effect
- Implicit bias
- Cognitive bias
- Selection bias
- Negativity bias
- Status quo bias
Cite this Scribbr article
If you want to cite this source, you can copy and paste the citation or click the “Cite this Scribbr article” button to automatically add the citation to our free Citation Generator.
McCombes, S. (2023, June 22). Descriptive Research | Definition, Types, Methods & Examples. Scribbr. Retrieved September 14, 2024, from https://www.scribbr.com/methodology/descriptive-research/
Is this article helpful?
Shona McCombes
Other students also liked, what is quantitative research | definition, uses & methods, correlational research | when & how to use, descriptive statistics | definitions, types, examples, "i thought ai proofreading was useless but..".
I've been using Scribbr for years now and I know it's a service that won't disappoint. It does a good job spotting mistakes”
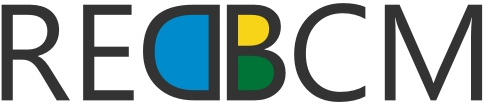
Guilherme Mazui
- What is the Difference Between Analytical and Descriptive?
The main difference between analytical and descriptive research lies in their purpose and approach. Here are the key differences between the two:
- Descriptive research aims to describe a situation, problem, or phenomenon accurately, providing a snapshot of specific phenomena at a particular point in time.
- Analytical research goes beyond description to analyze and interpret data, unearth insights, understand underlying relationships, or solve problems.
- Descriptive research collects data to portray or snapshot the subject matter accurately, classifying, describing, comparing, and measuring data.
- Analytical research uses data to conduct deeper analysis, identify patterns, and explore cause-effect relationships, focusing on cause and effect.
- Descriptive research provides a clear and detailed picture of the situation or issue.
- Analytical research offers insights, explanations, or solutions based on a thorough analysis.
- Complexity :
- Descriptive research is generally more straightforward, as it only describes the existing state of affairs.
- Analytical research is more complex, involving critically examining data to draw meaningful conclusions.
In summary, descriptive research focuses on accurately portraying the current state of variables or conditions, while analytical research delves deeper to understand, interpret, or explain why and how certain phenomena occur, aiming to uncover underlying relationships and causality between variables.
Comparative Table: Analytical vs Descriptive
The main difference between analytical and descriptive writing lies in their focus and purpose. Here is a table comparing the two:
Descriptive Writing | Analytical Writing |
---|---|
States what happened (the event) | Explains the impact of the event, especially in relation to the research question(s) |
Explains what a theory says | Explains how the theory is relevant to the key issue(s) and research question(s) |
Notes the methods used | Explains whether these methods were relevant or not |
States what time/date something happened | Explains why the timing is important/relevant |
Explains how something works | Provides various pieces of information |
- | Draws a conclusion in relation to the various pieces of information |
Descriptive writing focuses on providing clear descriptions of facts or things that have happened, while analytical writing evaluates information and draws conclusions based on the data and context. In most cases, both types of writing are used in combination to effectively communicate ideas and findings.
- Descriptive vs Analytic Epidemiology
- Prescriptive vs Descriptive
- Descriptive vs Correlational Research
- Descriptive vs Exploratory Research
- Narrative vs Descriptive Essay
- Descriptive vs Inferential Statistics
- Descriptive vs Experimental Research
- Case Study vs Descriptive Approach to Research
- Summary vs Analysis
- Description vs Definition
- Qualitative Analysis vs Quantitative Analysis
- Explain vs Describe
- Job Analysis vs Job Description
- Analysis vs Synthesis
- Narration vs Description
- Analysis vs Analyses
- Quantitative vs Qualitative
- Prescriptivism vs Descriptivism
- Predictive vs Prescriptive Analytics
- Skip to main content
- Skip to primary sidebar
- Skip to footer
- QuestionPro

- Solutions Industries Gaming Automotive Sports and events Education Government Travel & Hospitality Financial Services Healthcare Cannabis Technology Use Case AskWhy Communities Audience Contactless surveys Mobile LivePolls Member Experience GDPR Positive People Science 360 Feedback Surveys
- Resources Blog eBooks Survey Templates Case Studies Training Help center

Home Market Research Research Tools and Apps
Analytical Research: What is it, Importance + Examples
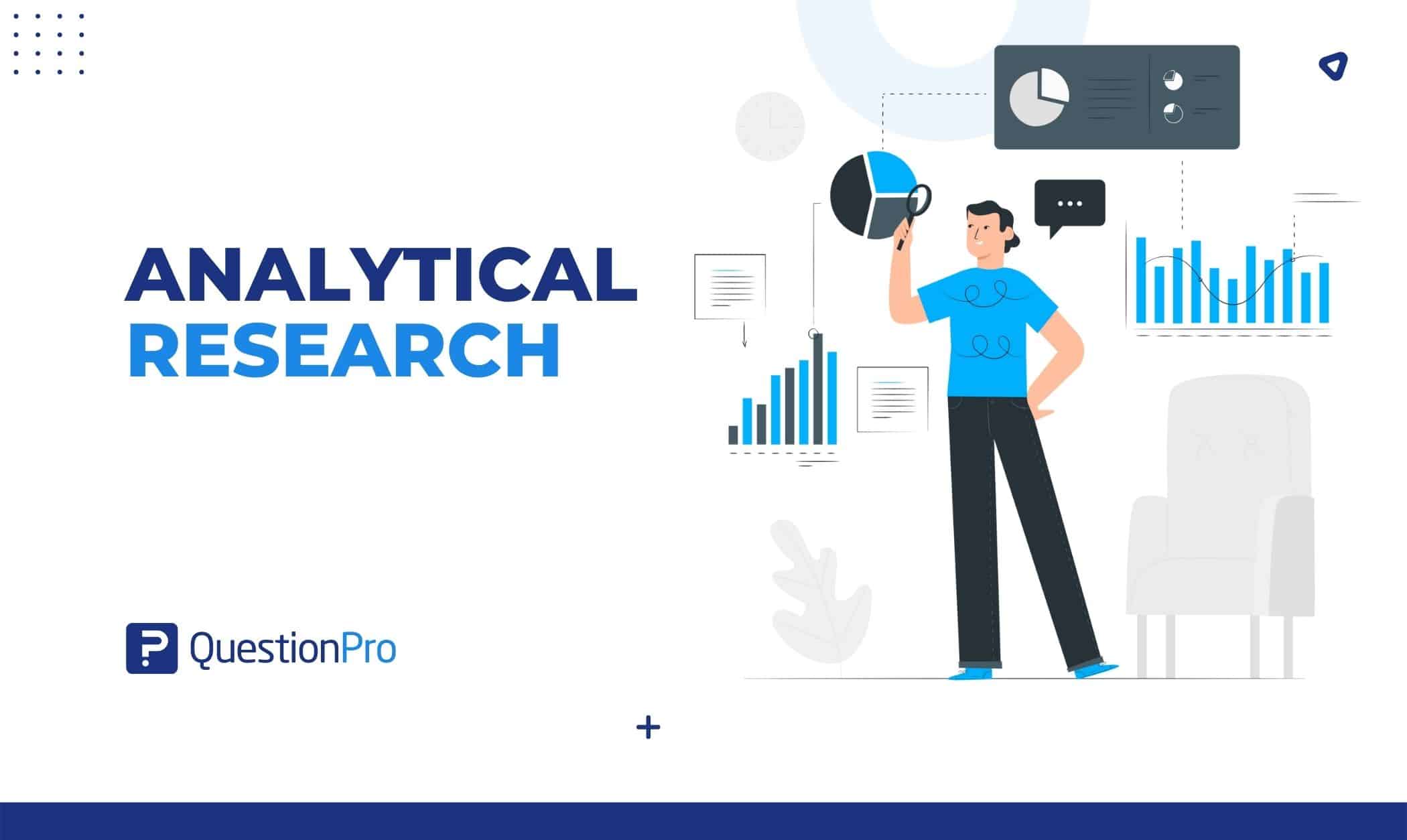
Finding knowledge is a loose translation of the word “research.” It’s a systematic and scientific way of researching a particular subject. As a result, research is a form of scientific investigation that seeks to learn more. Analytical research is one of them.
Any kind of research is a way to learn new things. In this research, data and other pertinent information about a project are assembled; after the information is gathered and assessed, the sources are used to support a notion or prove a hypothesis.
An individual can successfully draw out minor facts to make more significant conclusions about the subject matter by using critical thinking abilities (a technique of thinking that entails identifying a claim or assumption and determining whether it is accurate or untrue).
What is analytical research?
This particular kind of research calls for using critical thinking abilities and assessing data and information pertinent to the project at hand.
Determines the causal connections between two or more variables. The analytical study aims to identify the causes and mechanisms underlying the trade deficit’s movement throughout a given period.
It is used by various professionals, including psychologists, doctors, and students, to identify the most pertinent material during investigations. One learns crucial information from analytical research that helps them contribute fresh concepts to the work they are producing.
Some researchers perform it to uncover information that supports ongoing research to strengthen the validity of their findings. Other scholars engage in analytical research to generate fresh perspectives on the subject.
Various approaches to performing research include literary analysis, Gap analysis , general public surveys, clinical trials, and meta-analysis.
Importance of analytical research
The goal of analytical research is to develop new ideas that are more believable by combining numerous minute details.
The analytical investigation is what explains why a claim should be trusted. Finding out why something occurs is complex. You need to be able to evaluate information critically and think critically.
This kind of information aids in proving the validity of a theory or supporting a hypothesis. It assists in recognizing a claim and determining whether it is true.
Analytical kind of research is valuable to many people, including students, psychologists, marketers, and others. It aids in determining which advertising initiatives within a firm perform best. In the meantime, medical research and research design determine how well a particular treatment does.
Thus, analytical research can help people achieve their goals while saving lives and money.
Methods of Conducting Analytical Research
Analytical research is the process of gathering, analyzing, and interpreting information to make inferences and reach conclusions. Depending on the purpose of the research and the data you have access to, you can conduct analytical research using a variety of methods. Here are a few typical approaches:
Quantitative research
Numerical data are gathered and analyzed using this method. Statistical methods are then used to analyze the information, which is often collected using surveys, experiments, or pre-existing datasets. Results from quantitative research can be measured, compared, and generalized numerically.
Qualitative research
In contrast to quantitative research, qualitative research focuses on collecting non-numerical information. It gathers detailed information using techniques like interviews, focus groups, observations, or content research. Understanding social phenomena, exploring experiences, and revealing underlying meanings and motivations are all goals of qualitative research.
Mixed methods research
This strategy combines quantitative and qualitative methodologies to grasp a research problem thoroughly. Mixed methods research often entails gathering and evaluating both numerical and non-numerical data, integrating the results, and offering a more comprehensive viewpoint on the research issue.
Experimental research
Experimental research is frequently employed in scientific trials and investigations to establish causal links between variables. This approach entails modifying variables in a controlled environment to identify cause-and-effect connections. Researchers randomly divide volunteers into several groups, provide various interventions or treatments, and track the results.
Observational research
With this approach, behaviors or occurrences are observed and methodically recorded without any outside interference or variable data manipulation . Both controlled surroundings and naturalistic settings can be used for observational research . It offers useful insights into behaviors that occur in the actual world and enables researchers to explore events as they naturally occur.
Case study research
This approach entails thorough research of a single case or a small group of related cases. Case-control studies frequently include a variety of information sources, including observations, records, and interviews. They offer rich, in-depth insights and are particularly helpful for researching complex phenomena in practical settings.
Secondary data analysis
Examining secondary information is time and money-efficient, enabling researchers to explore new research issues or confirm prior findings. With this approach, researchers examine previously gathered information for a different reason. Information from earlier cohort studies, accessible databases, or corporate documents may be included in this.
Content analysis
Content research is frequently employed in social sciences, media observational studies, and cross-sectional studies. This approach systematically examines the content of texts, including media, speeches, and written documents. Themes, patterns, or keywords are found and categorized by researchers to make inferences about the content.
Depending on your research objectives, the resources at your disposal, and the type of data you wish to analyze, selecting the most appropriate approach or combination of methodologies is crucial to conducting analytical research.
Examples of analytical research
Analytical research takes a unique measurement. Instead, you would consider the causes and changes to the trade imbalance. Detailed statistics and statistical checks help guarantee that the results are significant.
For example, it can look into why the value of the Japanese Yen has decreased. This is so that an analytical study can consider “how” and “why” questions.
Another example is that someone might conduct analytical research to identify a study’s gap. It presents a fresh perspective on your data. Therefore, it aids in supporting or refuting notions.
Descriptive vs analytical research
Here are the key differences between descriptive research and analytical research:
Aspect | Descriptive Research | Analytical Research |
Objective | Describe and document characteristics or phenomena. | Analyze and interpret data to understand relationships or causality. |
Focus | “What” questions | “Why” and “How” questions |
Data Analysis | Summarizing information | Statistical research, hypothesis testing, qualitative research |
Goal | Provide an accurate and comprehensive description | Gain insights, make inferences, provide explanations or predictions |
Causal Relationships | Not the primary focus | Examining underlying factors, causes, or effects |
Examples | Surveys, observations, case-control study, content analysis | Experiments, statistical research, qualitative analysis |
The study of cause and effect makes extensive use of analytical research. It benefits from numerous academic disciplines, including marketing, health, and psychology, because it offers more conclusive information for addressing research issues.
QuestionPro offers solutions for every issue and industry, making it more than just survey software. For handling data, we also have systems like our InsightsHub research library.
You may make crucial decisions quickly while using QuestionPro to understand your clients and other study subjects better. Make use of the possibilities of the enterprise-grade research suite right away!
LEARN MORE FREE TRIAL
MORE LIKE THIS
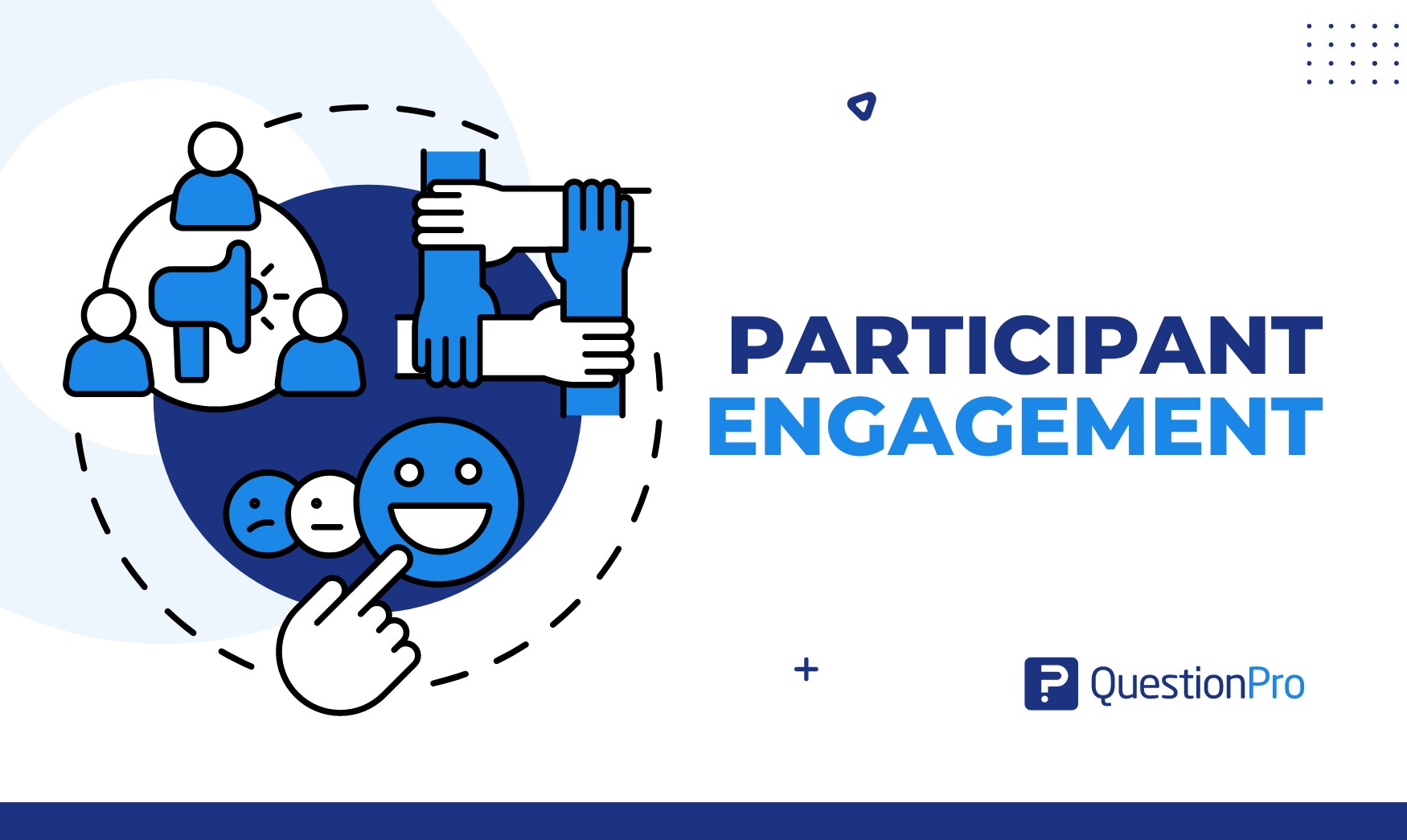
Participant Engagement: Strategies + Improving Interaction
Sep 12, 2024
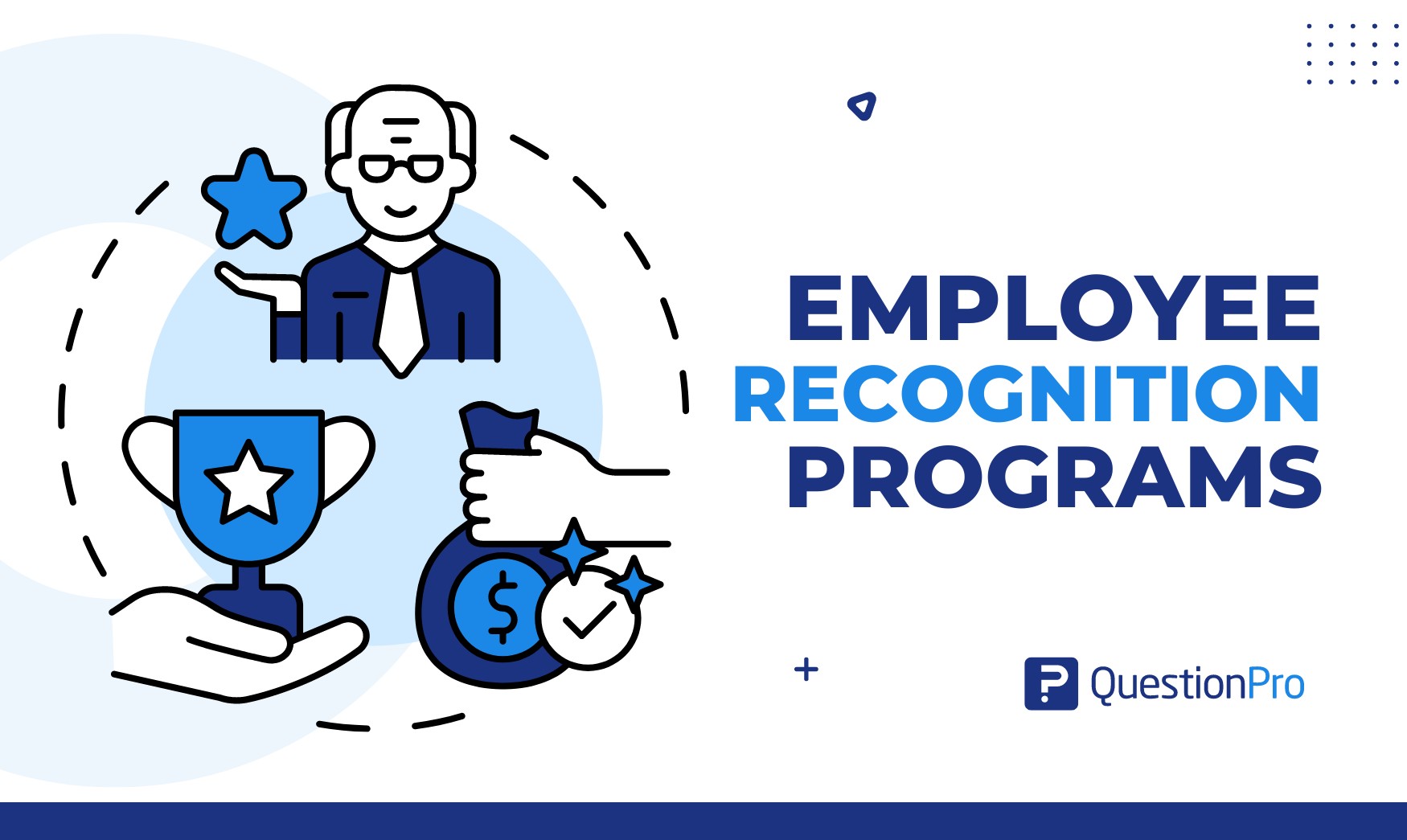
Employee Recognition Programs: A Complete Guide
Sep 11, 2024
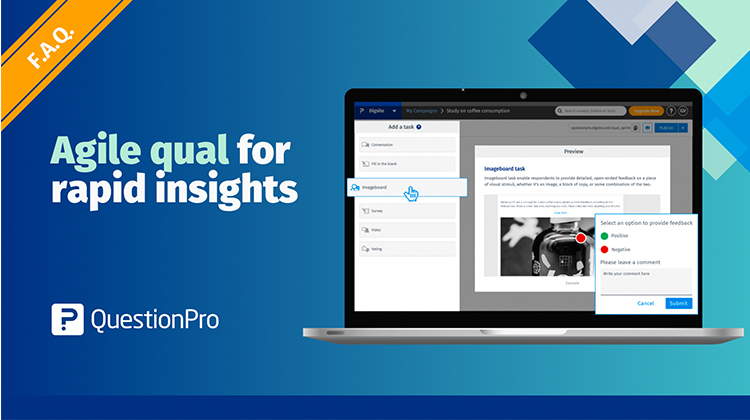
A guide to conducting agile qualitative research for rapid insights with Digsite
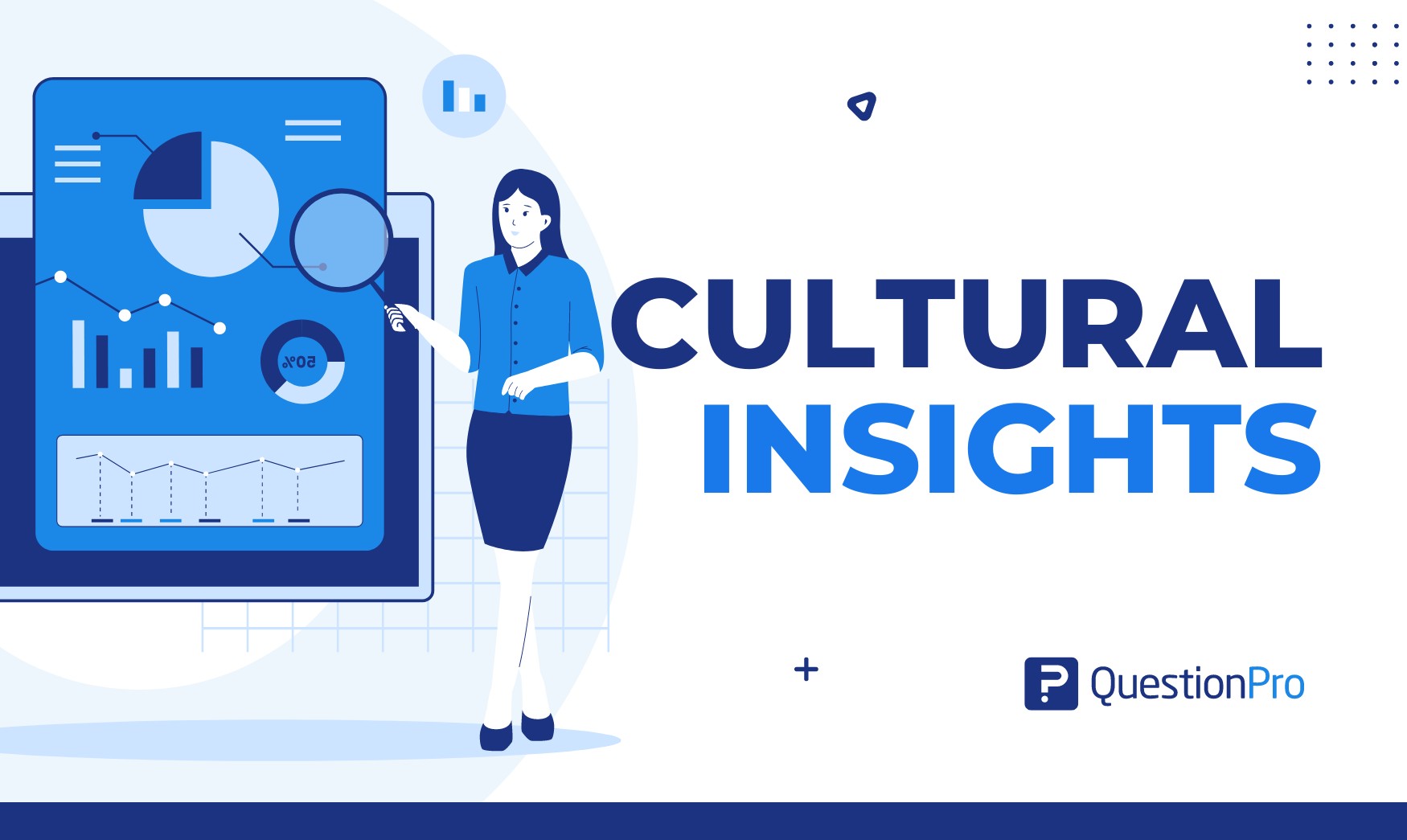
Cultural Insights: What it is, Importance + How to Collect?
Sep 10, 2024
Other categories
- Academic Research
- Artificial Intelligence
- Assessments
- Brand Awareness
- Case Studies
- Communities
- Consumer Insights
- Customer effort score
- Customer Engagement
- Customer Experience
- Customer Loyalty
- Customer Research
- Customer Satisfaction
- Employee Benefits
- Employee Engagement
- Employee Retention
- Friday Five
- General Data Protection Regulation
- Insights Hub
- Life@QuestionPro
- Market Research
- Mobile diaries
- Mobile Surveys
- New Features
- Online Communities
- Question Types
- Questionnaire
- QuestionPro Products
- Release Notes
- Research Tools and Apps
- Revenue at Risk
- Survey Templates
- Training Tips
- Tuesday CX Thoughts (TCXT)
- Uncategorized
- What’s Coming Up
- Workforce Intelligence
What are Analytical Study Designs?
- Research Process
- Peer Review
Analytical study designs can be experimental or observational and each type has its own features. In this article, you'll learn the main types of designs and how to figure out which one you'll need for your study.
Updated on September 19, 2022

A study design is critical to your research study because it determines exactly how you will collect and analyze your data. If your study aims to study the relationship between two variables, then an analytical study design is the right choice.
But how do you know which type of analytical study design is best for your specific research question? It's necessary to have a clear plan before you begin data collection. Lots of researchers, sadly, speed through this or don't do it at all.
When are analytical study designs used?
A study design is a systematic plan, developed so you can carry out your research study effectively and efficiently. Having a design is important because it will determine the right methodologies for your study. Using the right study design makes your results more credible, valid, and coherent.
Descriptive vs. analytical studies
Study designs can be broadly divided into either descriptive or analytical.
Descriptive studies describe characteristics such as patterns or trends. They answer the questions of what, who, where, and when, and they generate hypotheses. They include case reports and qualitative studies.
Analytical study designs quantify a relationship between different variables. They answer the questions of why and how. They're used to test hypotheses and make predictions.
Experimental and observational
Analytical study designs can be either experimental or observational. In experimental studies, researchers manipulate something in a population of interest and examine its effects. These designs are used to establish a causal link between two variables.
In observational studies, in contrast, researchers observe the effects of a treatment or intervention without manipulating anything. Observational studies are most often used to study larger patterns over longer periods.
Experimental study designs
Experimental study designs are when a researcher introduces a change in one group and not in another. Typically, these are used when researchers are interested in the effects of this change on some outcome. It's important to try to ensure that both groups are equivalent at baseline to make sure that any differences that arise are from any introduced change.
In one study, Reiner and colleagues studied the effects of a mindfulness intervention on pain perception . The researchers randomly assigned participants into an experimental group that received a mindfulness training program for two weeks. The rest of the participants were placed in a control group that did not receive the intervention.
Experimental studies help us establish causality. This is critical in science because we want to know whether one variable leads to a change, or causes another. Establishing causality leads to higher internal validity and makes results reproducible.
Experimental designs include randomized control trials (RCTs), nonrandomized control trials (non-RCTs), and crossover designs. Read on to learn the differences.
Randomized control trials
In an RCT, one group of individuals receives an intervention or a treatment, while another does not. It's then possible to investigate what happens to the participants in each group.
Another important feature of RCTs is that participants are randomly assigned to study groups. This helps to limit certain biases and retain better control. Randomization also lets researchers pinpoint any differences in outcomes to the intervention received during the trial. RTCs are considered the gold standard in biomedical research and are considered to provide the best kind of evidence.
For example, one RCT looked at whether an exercise intervention impacts depression . Researchers randomly placed patients with depressive symptoms into intervention groups containing different types of exercise (i.e., light, moderate, or strong). Another group received usual medications or no exercise interventions.
Results showed that after the 12-week trial, patients in all exercise groups had decreased depression levels compared to the control group. This means that by using an RCT design, researchers can now safely assume that the exercise variable has a positive impact on depression.
However, RCTs are not without drawbacks. In the example above, we don't know if exercise still has a positive impact on depression in the long term. This is because it's not feasible to keep people under these controlled settings for a long time.
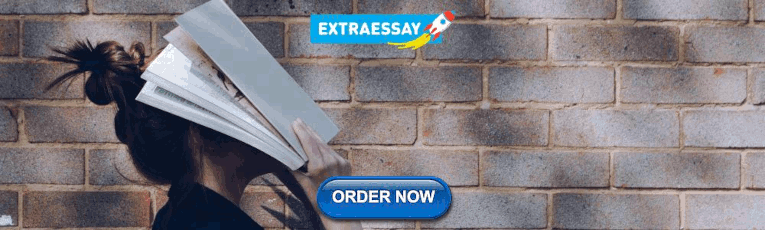
Advantages of RCTs
- It is possible to infer causality
- Everything is properly controlled, so very little is left to chance or bias
- Can be certain that any difference is coming from the intervention
Disadvantages of RCTs
- Expensive and can be time-consuming
- Can take years for results to be available
- Cannot be done for certain types of questions due to ethical reasons, such as asking participants to undergo harmful treatment
- Limited in how many participants researchers can adequately manage in one study or trial
- Not feasible for people to live under controlled conditions for a long time
Nonrandomized controlled trials
Nonrandomized controlled trials are a type of nonrandomized controlled studies (NRS) where the allocation of participants to intervention groups is not done randomly . Here, researchers purposely assign some participants to one group and others to another group based on certain features. Alternatively, participants can sometimes also decide which group they want to be in.
For example, in one study, clinicians were interested in the impact of stroke recovery after being in an enriched versus non-enriched hospital environment . Patients were selected for the trial if they fulfilled certain requirements common to stroke recovery. Then, the intervention group was given access to an enriched environment (i.e. internet access, reading, going outside), and another group was not. Results showed that the enriched group performed better on cognitive tasks.
NRS are useful in medical research because they help study phenomena that would be difficult to measure with an RCT. However, one of their major drawbacks is that we cannot be sure if the intervention leads to the outcome. In the above example, we can't say for certain whether those patients improved after stroke because they were in the enriched environment or whether there were other variables at play.
Advantages of NRS's
- Good option when randomized control trials are not feasible
- More flexible than RCTs
Disadvantages of NRS's
- Can't be sure if the groups have underlying differences
- Introduces risk of bias and confounds
Crossover study
In a crossover design, each participant receives a sequence of different treatments. Crossover designs can be applied to RCTs, in which each participant is randomly assigned to different study groups.
For example, one study looked at the effects of replacing butter with margarine on lipoproteins levels in individuals with cholesterol . Patients were randomly assigned to a 6-week butter diet, followed by a 6-week margarine diet. In between both diets, participants ate a normal diet for 5 weeks.
These designs are helpful because they reduce bias. In the example above, each participant completed both interventions, making them serve as their own control. However, we don't know if eating butter or margarine first leads to certain results in some subjects.
Advantages of crossover studies
- Each participant serves as their own control, reducing confounding variables
- Require fewer participants, so they have better statistical power
Disadvantages of crossover studies
- Susceptible to order effects, meaning the order in which a treatment was given may have an effect
- Carry-over effects between treatments
Observational studies
In observational studies, researchers watch (observe) the effects of a treatment or intervention without trying to change anything in the population. Observational studies help us establish broad trends and patterns in large-scale datasets or populations. They are also a great alternative when an experimental study is not an option.
Unlike experimental research, observational studies do not help us establish causality. This is because researchers do not actively control any variables. Rather, they investigate statistical relationships between them. Often this is done using a correlational approach.
For example, researchers would like to examine the effects of daily fiber intake on bone density . They conduct a large-scale survey of thousands of individuals to examine correlations of fiber intake with different health measures.
The main observational studies are case-control, cohort, and cross-sectional. Let's take a closer look at each one below.
Case-control study
A case-control is a type of observational design in which researchers identify individuals with an existing health situation (cases) and a similar group without the health issue (controls). The cases and the controls are then compared based on some measurements.
Frequently, data collection in a case-control study is retroactive (i.e., backwards in time). This is because participants have already been exposed to the event in question. Additionally, researchers must go through records and patient files to obtain the records for this study design.
For example, a group of researchers examined whether using sleeping pills puts people at risk of Alzheimer's disease . They selected 1976 individuals that received a dementia diagnosis (“cases”) with 7184 other individuals (“controls”). Cases and controls were matched on specific measures such as sex and age. Patient data was consulted to find out how much sleeping pills were consumed over the course of a certain time.
Case-control is ideal for situations where cases are easy to pick out and compare. For instance, in studying rare diseases or outbreaks.
Advantages of case-control studies
- Feasible for rare diseases
- Cheaper and easier to do than an RCT
Disadvantages of case-control studies
- Relies on patient records, which could be lost or damaged
- Potential recall and selection bias
Cohort study (longitudinal)
A cohort is a group of people who are linked in some way. For instance, a birth year cohort is all people born in a specific year. In cohort studies, researchers compare what happens to individuals in the cohort that have been exposed to some variable compared with those that haven't on different variables. They're also called longitudinal studies.
The cohort is then repeatedly assessed on variables of interest over a period of time. There is no set amount of time required for cohort studies. They can range from a few weeks to many years.
Cohort studies can be prospective. In this case, individuals are followed for some time into the future. They can also be retrospective, where data is collected on a cohort from records.
One of the longest cohort studies today is The Harvard Study of Adult Development . This cohort study has been tracking various health outcomes of 268 Harvard graduates and 456 poor individuals in Boston from 1939 to 2014. Physical screenings, blood samples, brain scans and surveys were collected on this cohort for over 70 years. This study has produced a wealth of knowledge on outcomes throughout life.
A cohort study design is a good option when you have a specific group of people you want to study over time. However, a major drawback is that they take a long time and lack control.
Advantages of cohort studies
- Ethically safe
- Allows you to study multiple outcome variables
- Establish trends and patterns
Disadvantages of cohort studies
- Time consuming and expensive
- Can take many years for results to be revealed
- Too many variables to manage
- Depending on length of study, can have many changes in research personnel
Cross-sectional study
Cross-sectional studies are also known as prevalence studies. They look at the relationship of specific variables in a population in one given time. In cross-sectional studies, the researcher does not try to manipulate any of the variables, just study them using statistical analyses. Cross-sectional studies are also called snapshots of a certain variable or time.
For example, researchers wanted to determine the prevalence of inappropriate antibiotic use to study the growing concern about antibiotic resistance. Participants completed a self-administered questionnaire assessing their knowledge and attitude toward antibiotic use. Then, researchers performed statistical analyses on their responses to determine the relationship between the variables.
Cross-sectional study designs are ideal when gathering initial data on a research question. This data can then be analyzed again later. By knowing the public's general attitudes towards antibiotics, this information can then be relayed to physicians or public health authorities. However, it's often difficult to determine how long these results stay true for.
Advantages of cross-sectional studies
- Fast and inexpensive
- Provides a great deal of information for a given time point
- Leaves room for secondary analysis
Disadvantages of cross-sectional studies
- Requires a large sample to be accurate
- Not clear how long results remain true for
- Do not provide information on causality
- Cannot be used to establish long-term trends because data is only for a given time
So, how about your next study?
Whether it's an RCT, a case-control, or even a qualitative study, AJE has services to help you at every step of the publication process. Get expert guidance and publish your work for the world to see.

The AJE Team
See our "Privacy Policy"
- Privacy Policy

Home » Descriptive Analytics – Methods, Tools and Examples
Descriptive Analytics – Methods, Tools and Examples
Table of Contents

Descriptive Analytics
Definition:
Descriptive analytics focused on describing or summarizing raw data and making it interpretable. This type of analytics provides insight into what has happened in the past. It involves the analysis of historical data to identify patterns, trends, and insights. Descriptive analytics often uses visualization tools to represent the data in a way that is easy to interpret.
Descriptive Analytics in Research
Descriptive analytics plays a crucial role in research, helping investigators understand and describe the data collected in their studies. Here’s how descriptive analytics is typically used in a research setting:
- Descriptive Statistics: In research, descriptive analytics often takes the form of descriptive statistics . This includes calculating measures of central tendency (like mean, median, and mode), measures of dispersion (like range, variance, and standard deviation), and measures of frequency (like count, percent, and frequency). These calculations help researchers summarize and understand their data.
- Visualizing Data: Descriptive analytics also involves creating visual representations of data to better understand and communicate research findings . This might involve creating bar graphs, line graphs, pie charts, scatter plots, box plots, and other visualizations.
- Exploratory Data Analysis: Before conducting any formal statistical tests, researchers often conduct an exploratory data analysis, which is a form of descriptive analytics. This might involve looking at distributions of variables, checking for outliers, and exploring relationships between variables.
- Initial Findings: Descriptive analytics are often reported in the results section of a research study to provide readers with an overview of the data. For example, a researcher might report average scores, demographic breakdowns, or the percentage of participants who endorsed each response on a survey.
- Establishing Patterns and Relationships: Descriptive analytics helps in identifying patterns, trends, or relationships in the data, which can guide subsequent analysis or future research. For instance, researchers might look at the correlation between variables as a part of descriptive analytics.
Descriptive Analytics Techniques
Descriptive analytics involves a variety of techniques to summarize, interpret, and visualize historical data. Some commonly used techniques include:
Statistical Analysis
This includes basic statistical methods like mean, median, mode (central tendency), standard deviation, variance (dispersion), correlation, and regression (relationships between variables).
Data Aggregation
It is the process of compiling and summarizing data to obtain a general perspective. It can involve methods like sum, count, average, min, max, etc., often applied to a group of data.
Data Mining
This involves analyzing large volumes of data to discover patterns, trends, and insights. Techniques used in data mining can include clustering (grouping similar data), classification (assigning data into categories), association rules (finding relationships between variables), and anomaly detection (identifying outliers).
Data Visualization
This involves presenting data in a graphical or pictorial format to provide clear and easy understanding of the data patterns, trends, and insights. Common data visualization methods include bar charts, line graphs, pie charts, scatter plots, histograms, and more complex forms like heat maps and interactive dashboards.
This involves organizing data into informational summaries to monitor how different areas of a business are performing. Reports can be generated manually or automatically and can be presented in tables, graphs, or dashboards.
Cross-tabulation (or Pivot Tables)
It involves displaying the relationship between two or more variables in a tabular form. It can provide a deeper understanding of the data by allowing comparisons and revealing patterns and correlations that may not be readily apparent in raw data.
Descriptive Modeling
Some techniques use complex algorithms to interpret data. Examples include decision tree analysis, which provides a graphical representation of decision-making situations, and neural networks, which are used to identify correlations and patterns in large data sets.
Descriptive Analytics Tools
Some common Descriptive Analytics Tools are as follows:
Excel: Microsoft Excel is a widely used tool that can be used for simple descriptive analytics. It has powerful statistical and data visualization capabilities. Pivot tables are a particularly useful feature for summarizing and analyzing large data sets.
Tableau: Tableau is a data visualization tool that is used to represent data in a graphical or pictorial format. It can handle large data sets and allows for real-time data analysis.
Power BI: Power BI, another product from Microsoft, is a business analytics tool that provides interactive visualizations with self-service business intelligence capabilities.
QlikView: QlikView is a data visualization and discovery tool. It allows users to analyze data and use this data to support decision-making.
SAS: SAS is a software suite that can mine, alter, manage and retrieve data from a variety of sources and perform statistical analysis on it.
SPSS: SPSS (Statistical Package for the Social Sciences) is a software package used for statistical analysis. It’s widely used in social sciences research but also in other industries.
Google Analytics: For web data, Google Analytics is a popular tool. It allows businesses to analyze in-depth detail about the visitors on their website, providing valuable insights that can help shape the success strategy of a business.
R and Python: Both are programming languages that have robust capabilities for statistical analysis and data visualization. With packages like pandas, matplotlib, seaborn in Python and ggplot2, dplyr in R, these languages are powerful tools for descriptive analytics.
Looker: Looker is a modern data platform that can take data from any database and let you start exploring and visualizing.
When to use Descriptive Analytics
Descriptive analytics forms the base of the data analysis workflow and is typically the first step in understanding your business or organization’s data. Here are some situations when you might use descriptive analytics:
Understanding Past Behavior: Descriptive analytics is essential for understanding what has happened in the past. If you need to understand past sales trends, customer behavior, or operational performance, descriptive analytics is the tool you’d use.
Reporting Key Metrics: Descriptive analytics is used to establish and report key performance indicators (KPIs). It can help in tracking and presenting these KPIs in dashboards or regular reports.
Identifying Patterns and Trends: If you need to identify patterns or trends in your data, descriptive analytics can provide these insights. This might include identifying seasonality in sales data, understanding peak operational times, or spotting trends in customer behavior.
Informing Business Decisions: The insights provided by descriptive analytics can inform business strategy and decision-making. By understanding what has happened in the past, you can make more informed decisions about what steps to take in the future.
Benchmarking Performance: Descriptive analytics can be used to compare current performance against historical data. This can be used for benchmarking and setting performance goals.
Auditing and Regulatory Compliance: In sectors where compliance and auditing are essential, descriptive analytics can provide the necessary data and trends over specific periods.
Initial Data Exploration: When you first acquire a dataset, descriptive analytics is useful to understand the structure of the data, the relationships between variables, and any apparent anomalies or outliers.
Examples of Descriptive Analytics
Examples of Descriptive Analytics are as follows:
Retail Industry: A retail company might use descriptive analytics to analyze sales data from the past year. They could break down sales by month to identify any seasonality trends. For example, they might find that sales increase in November and December due to holiday shopping. They could also break down sales by product to identify which items are the most popular. This analysis could inform their purchasing and stocking decisions for the next year. Additionally, data on customer demographics could be analyzed to understand who their primary customers are, guiding their marketing strategies.
Healthcare Industry: In healthcare, descriptive analytics could be used to analyze patient data over time. For instance, a hospital might analyze data on patient admissions to identify trends in admission rates. They might find that admissions for certain conditions are higher at certain times of the year. This could help them allocate resources more effectively. Also, analyzing patient outcomes data can help identify the most effective treatments or highlight areas where improvement is needed.
Finance Industry: A financial firm might use descriptive analytics to analyze historical market data. They could look at trends in stock prices, trading volume, or economic indicators to inform their investment decisions. For example, analyzing the price-earnings ratios of stocks in a certain sector over time could reveal patterns that suggest whether the sector is currently overvalued or undervalued. Similarly, credit card companies can analyze transaction data to detect any unusual patterns, which could be signs of fraud.
Advantages of Descriptive Analytics
Descriptive analytics plays a vital role in the world of data analysis, providing numerous advantages:
- Understanding the Past: Descriptive analytics provides an understanding of what has happened in the past, offering valuable context for future decision-making.
- Data Summarization: Descriptive analytics is used to simplify and summarize complex datasets, which can make the information more understandable and accessible.
- Identifying Patterns and Trends: With descriptive analytics, organizations can identify patterns, trends, and correlations in their data, which can provide valuable insights.
- Inform Decision-Making: The insights generated through descriptive analytics can inform strategic decisions and help organizations to react more quickly to events or changes in behavior.
- Basis for Further Analysis: Descriptive analytics lays the groundwork for further analytical activities. It’s the first necessary step before moving on to more advanced forms of analytics like predictive analytics (forecasting future events) or prescriptive analytics (advising on possible outcomes).
- Performance Evaluation: It allows organizations to evaluate their performance by comparing current results with past results, enabling them to see where improvements have been made and where further improvements can be targeted.
- Enhanced Reporting and Dashboards: Through the use of visualization techniques, descriptive analytics can improve the quality of reports and dashboards, making the data more understandable and easier to interpret for stakeholders at all levels of the organization.
- Immediate Value: Unlike some other types of analytics, descriptive analytics can provide immediate insights, as it doesn’t require complex models or deep analytical capabilities to provide value.
Disadvantages of Descriptive Analytics
While descriptive analytics offers numerous benefits, it also has certain limitations or disadvantages. Here are a few to consider:
- Limited to Past Data: Descriptive analytics primarily deals with historical data and provides insights about past events. It does not predict future events or trends and can’t help you understand possible future outcomes on its own.
- Lack of Deep Insights: While descriptive analytics helps in identifying what happened, it does not answer why it happened. For deeper insights, you would need to use diagnostic analytics, which analyzes data to understand the root cause of a particular outcome.
- Can Be Misleading: If not properly executed, descriptive analytics can sometimes lead to incorrect conclusions. For example, correlation does not imply causation, but descriptive analytics might tempt one to make such an inference.
- Data Quality Issues: The accuracy and usefulness of descriptive analytics are heavily reliant on the quality of the underlying data. If the data is incomplete, incorrect, or biased, the results of the descriptive analytics will be too.
- Over-reliance on Descriptive Analytics: Businesses may rely too much on descriptive analytics and not enough on predictive and prescriptive analytics. While understanding past and present data is important, it’s equally vital to forecast future trends and make data-driven decisions based on those predictions.
- Doesn’t Provide Actionable Insights: Descriptive analytics is used to interpret historical data and identify patterns and trends, but it doesn’t provide recommendations or courses of action. For that, prescriptive analytics is needed.
About the author
Muhammad Hassan
Researcher, Academic Writer, Web developer
You may also like

Sentiment Analysis – Tools, Techniques and...

What is Data Science? Components, Process and Tools

Emerging Research Methods – Types and Examples

Digital Ethnography – Types, Methods and Examples

What is Big Data? Types, Tools and Examples

Blockchain Research – Methods, Types and Examples

Home » Health » What is the Difference Between Descriptive and Analytic Epidemiology
What is the Difference Between Descriptive and Analytic Epidemiology
The main difference between descriptive and analytical epidemiology is that descriptive epidemiology generates hypotheses on risk factors and causes of disease, whereas analytical epidemiology tests hypotheses by assessing the determinants of diseases, focusing on risk factors and causes as well as, analyzing the distribution of exposures and diseases. Furthermore, descriptive epidemiology is comparatively a small and less complex study area, while analytical epidemiology is a larger and more complex study area.
Descriptive and analytical epidemiology are two main areas of epidemiology that studies the distribution, patterns, and determinants of health and diseases in defined populations .
Key Areas Covered
1. What is Descriptive Epidemiology – Definition, Features, Importance 2. What is Analytical Epidemiology – Definition, Features, Importance 3. What are the Similarities Between Descriptive and Analytical Epidemiology – Outline of Common Features 4. What is the Difference Between Descriptive and Analytical Epidemiology – Comparison of Key Differences
Key Terms
Analytical Epidemiology, Descriptive Epidemiology, Making Hypotheses, Occurrence of Diseases, Testing Hypotheses

What is Descriptive Epidemiology
Descriptive epidemiology is one of the two main areas of epidemiological studies. It is responsible for the determination of the patterns of disease occurrence, focusing on clinical information, person, place, and time. Here, the clinical information includes the signs and symptoms of the disease, laboratory results, data on hospitalization, and live or dead numbers. Besides, it uses demographic information, including age, sex, material status, personal habits, etc. Also , it studies socioeconomic information such as education, occupation, income, residence, place of work, etc. Furthermore, cultural information, including ethnicity, dietary habits, and religious preferences, also have an effect on causing diseases.

Figure 1: Bar Graph of the Incidence of Mild Traumatic Brain Injury by Age Range
Generally, descriptive epidemiologists collect relatively accessible data used for program planning , generating hypotheses, and suggesting ideas for further studies. Moreover, the hypotheses produced by descriptive epidemiological studies are confirmed by the analytical epidemiology. Furthermore, the three main types of descriptive epidemiology are the case report, case studies, and incidence. Case reports describe the person, place, and time of a specific case while case series describes the person, place, and time of a group of cases. Incidence studies, on the other hand, describe the number of new cases during a specific time.
What is Analytical Epidemiology
Analytical epidemiology is the second area of epidemiology, and it is a more complex and broader area than descriptive epidemiology. It is responsible for testing the hypotheses built in descriptive epidemiology. Therefore, the main objective of analytical epidemiology is to assess the determinants of diseases, risk factors and causes, as well as, to analyze the distribution of diseases and their exposures. Additionally, the key feature of analytical epidemiology is that it uses comparison groups.

Figure 2: Table of Comparison of Prostate Screening Results Globally
Moreover, the two main types of analytical epidemiology are the experimental epidemiology and observational epidemiology. In experimental epidemiology , a randomized selection process based on chance is used to study different study groups. Sometimes, it can be clinical procedures, which study new drugs to prevent a particular disease in a community. In contrast, observational epidemiology is based on non-randomized studies. Moreover, they mainly study patterns of exposure. Furthermore, the four types of analytical epidemiology studies are cohort, case-control, cross-sectional, and ecologic.
Similarities Between Descriptive and Analytical Epidemiology
- Descriptive and analytical epidemiology are two main study areas of epidemiology.
- Moreover, both study the distribution, patterns, and determinants of health and diseases in defined populations.
- Also, their main goals are to identify who is at risk and to provide clues to the cause of diseases.
- Therefore, they are a type of important activities in public health authorities.
Difference Between Descriptive and Analytical Epidemiology
Definition .
Descriptive epidemiology refers to the area of epidemiology that focuses on describing disease distribution by characteristics relating to time, place, and people, while analytical epidemiology refers to the area of epidemiology, which measures the association between a particular exposure and a disease, using information collected from individuals, rather than from the aggregate population.
Importance
While descriptive epidemiology generates hypotheses on risk factors and causes of disease, analytical epidemiology tests hypotheses by assessing the determinants of diseases focusing on risk factors and causes as well as, analyzing the distribution of exposures and diseases. Thus, this is the main difference between descriptive and analytical epidemiology.
Focuses on
Another difference between descriptive and analytical epidemiology is that descriptive epidemiology focuses on what, who, when, and where disease can occur, while analytical epidemiology focuses on why and how disease occurs.
Significance
Furthermore, descriptive epidemiology is comparatively a small and less complex study area, while analytical epidemiology is a larger and more complex study area.
Broadness
Descriptive epidemiology uses individuals or a group of individuals to make hypotheses, while analytical epidemiology uses comparison groups to test hypotheses. Hence, this is also a difference between descriptive and analytical epidemiology.
Moreover, descriptive epidemiology includes case reports, case series, and incidence, while analytical epidemiology includes observational studies and experimental studies.
As an example, descriptive epidemiology examines case series using person, place, and time of first 100 patients with SARS, while analytical epidemiology measures risk factors for SARS such as contact with animals and infected people.
Conclusion
Descriptive epidemiology is one of the two main areas of epidemiology that produces hypotheses about the risk factors and causes of diseases. Analytical epidemiology, on the other hand, is the area of epidemiology which tests the above hypotheses. Moreover, it assesses the risk factors and analyzes the distribution of diseases. Therefore, the main difference between descriptive and analytical epidemiology is the type of study.
References:
1. Kobayashi, John. “Study Types in Epidemiology.” Nwcphp.org , Northwest Center for Public Health Practice. Available Here .
Image Courtesy:
1. “MTBI incidince bar graph” By self – Own work ( CC BY-SA 3.0 ) via Commons Wikimedia 2. “ Prostate cancer global epidemiology ” By US govt (Public Domain) via Commons Wikimedia
About the Author: Lakna
Lakna, a graduate in Molecular Biology and Biochemistry, is a Molecular Biologist and has a broad and keen interest in the discovery of nature related things. She has a keen interest in writing articles regarding science.
You May Also Like These
Leave a reply cancel reply.
- Translators
- Graphic Designers
Please enter the email address you used for your account. Your sign in information will be sent to your email address after it has been verified.
Analytical vs. Descriptive Writing: Definitions and Examples
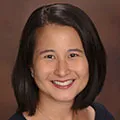
Scholars at all levels are expected to write. People who are not students or scholars often engage in writing for work, or to communicate with friends, family, and strangers through email, text messages, and social media. Academia recognizes two major types of writing—descriptive writing and analytical writing—which are both used in non-academic situations as well. As you might expect, descriptive writing focuses on clear descriptions of facts or things that have happened, while analytical writing provides additional analysis.
Descriptive writing is the most straightforward type of academic writing. It provides accurate information about "who", "what", "where", and "when". Examples of descriptive writing include:
- Summarizing an article (without offering additional insight)
- Stating the results of an experiment (without analyzing the implications)
- Describing a newsworthy event (without discussing possible long-term consequences)
High school students and undergraduates are most commonly asked to write descriptively, to show that they understand the key points of a specific topic (e.g. the major causes of World War II).
Analytical writing goes beyond summarizing information and instead provides evaluation, comparison, and possible conclusions. It addresses the questions of "why?", "so what?", and "what next?". Examples of analytical writing include:
- The discussion section of research papers
- Opinion pieces about the likely consequences of newsworthy events and the steps that should be taken in response.
High school students and undergraduates are sometimes asked to write analytically to "stretch their thinking". Possible topics might include "Could World War II have been avoided?" and "How can CRISPR-Cas9 technology improve human health?". The value of any such analysis is entirely dependent on the writer's ability to understand and clearly explain relevant information, which would be explained through descriptive writing. For graduate students and professional researchers, the quality of their work is at least partially based on the quality of their analysis.
The following table from The Study Skills Handbook by Stella Cottrell (2013, 4th edition, Palgrave Macmillan, page 198) is commonly used to summarize the differences between descriptive writing and analytical writing.
Descriptive Writing | Critical Analytical Writing |
---|---|
States what happened | Identifies the significance |
States what something is like | Evaluates strengths and weaknesses |
Gives the story so far | Weighs one piece of information against another |
Outlines the order in which things happened | Makes reasoned judgements |
Instructs how to do something | Argues a case according to the evidence |
List the main elements of a theory | Shows why something is relevant or suitable |
Outlines how something works | Indicates why something will work (best) |
Notes the method used | Identifies whether something is appropriate or suitable |
States when something occurred | Identifies why the timing is of importance |
States the different components | Weighs the importance of component parts |
States options | Gives reasons for selecting each option |
Lists details | Evaluates the relative significance of details |
Lists in any order | Structures information in order of importance |
States links between items | Shows the relevance of links between pieces of information |
Gives information or reports findings | Evaluates information and draws conclusions |
Description and analysis are also used in spoken communication such as presentations and conversations, and in visual communication such as diagrams and memes. In all of these cases, it is important to communicate clearly and effectively, and to use reliable sources of information.
Descriptive writing and analytical writing are often used in combination. In job application cover letters and essays for university admission, adding analytical text can provide context for otherwise unremarkable statements.
- Descriptive text: "I graduated from Bear University in 2020 with a B.S. in Chemistry and a cumulative GPA of 3.056."
- Analytical text: "While I struggled with some of my introductory courses, I proactively sought help to fill gaps in my understanding, and earned an "A" grade for all five of my senior year science courses. Therefore, I believe I am a strong candidate for . . ."
Combining description and analysis can also be very effective when discussing the significance of research results.
- Descriptive text: "Our study found significant (>2 ug/L) concentrations of polyfluoroalkyl substances (PFAS) in blood samples from all 5,478 study participants."
- Analytical text: "These results are alarming because the sample population included people who range in age from 1 month old to 98 years old, who live on five different continents, who reside in extremely rural areas and in urban areas, and who have little to no direct contact with products containing PFAS. PFAS are called "forever chemicals" because they are estimated to take hundreds or thousands of years to degrade. According to the US Centers for Disease Control (CDC), PFAS can move through soils to contaminate drinking water, and bioaccumulate in animals. Further research is urgently needed to better understand the adverse effects that PFAS have on human health, to identify the source of PFAS in rural communities, and to develop a method to sequester or destroy PFAS that have already entered the environment."
In both of the examples above, the analytical text includes additional facts (e.g. "A" grade for senior science courses; 1 month old to 98 years old) that help strengthen the argument. The student's transcript and the research paper's results section would contain these same facts—along with many others—written descriptively or presented in graphs, tables, or lists. For the analytical text, the author is trying to persuade the reader, and has therefore selected relevant facts to support their argument.
In the example about PFAS, the author's argument is further strengthened by citing additional information from a reputable source (the CDC). In reports where the author is supposed to be unbiased (e.g. a journalist writing descriptively), a similar effect can be obtained by quoting reputable sources. For example, "Professor of environmental science Kim Lee explains that PFAS are. . ." In these situations, it is often appropriate to present opposing views, as long as they come from reputable sources. This strategy of quoting or citing reputable sources can also be effective for students and professionals who do not have strong credentials in the topic under discussion.
Analytical writing supports a point of view
People cannot choose their own facts, but the same facts can be used to support very different points of view. Let's consider some different points of view that can be supported by the PFAS example from above.
- Scientific point of view: "Further research is urgently needed to better understand the adverse effects that PFAS have on human health, to identify the source of PFAS in rural communities, and to develop a method to sequester or destroy PFAS that have already entered the environment."
- Policy point of view: "Legislative action is urgently needed to ban the use of all PFAS, instead of banning new PFAS one at a time. Abundant and reliable data strongly indicates that all PFAS have similar effects, even if they have small differences in chemical composition. Given such evidence, the impetus must be on the chemical industry to prove safety, rather than on the general public to prove harm."
- Legal point of view: "Chemical companies have known about the danger of PFAS for years, but hid the evidence and continued to use these chemicals. Therefore, individuals and communities who have been harmed have the right to sue for damages."
These three points of view focus on three different fields (science, policy, and law), but all have a negative view of PFAS. The next example shows how the same factual information can be used to support opposing views.
- Descriptive text: " According to Data USA , the average fast food worker in 2019 was 26.1 years old, and earned a salary of $12,294 a year."
- Point of view #1: "These data show why raising the minimum wage is unnecessary. Most fast food workers are young, with many being teenagers who are making extra money while living with their parents. The majority will eventually transition to jobs that require more skills, and that are rewarded with higher pay. If we mandate that companies pay low-skill workers more than required by the free market, then more highly skilled workers will also demand a pay raise. This will hurt businesses, contribute to inflation, and have no net benefit."
- Point of view #2: "These data show why raising the minimum wage is so important. On average, for every 16-year-old working in fast food for extra money, there is a 36-year-old trying to make ends meet. As factory jobs have moved overseas, employees without specialized skills have turned to fast food for steady employment. According to the UC Berkeley Labor Center , for families with someone working full-time (40 hours/week) in fast food, more than half are enrolled in public assistance programs. These include Medicaid, food stamps, and the Earned Income Tax Credit. Therefore, taxpayers are subsidizing companies that pay poverty wages, so that their employees can have access to basic necessities like food and healthcare."
A primary purpose of analytical writing is to show how facts (explained through descriptive writing) support a particular conclusion or a particular path forward. This often requires explaining why an alternative interpretation is less satisfactory. This is how scholarly work—and good discussions in less formal situations—contribute to our collective understanding of the world.
Descriptive Research and Qualitative Research
Cite this chapter.
- Eunsook T. Koh 2 &
- Willis L. Owen 2
1037 Accesses
19 Citations
Descriptive research is a study of status and is widely used in education, nutrition, epidemiology, and the behavioral sciences. Its value is based on the premise that problems can be solved and practices improved through observation, analysis, and description. The most common descriptive research method is the survey, which includes questionnaires, personal interviews, phone surveys, and normative surveys. Developmental research is also descriptive. Through cross-sectional and longitudinal studies, researchers investigate the interaction of diet (e.g., fat and its sources, fiber and its sources, etc.) and life styles (e.g., smoking, alcohol drinking, etc.) and of disease (e.g., cancer, coronary heart disease) development. Observational research and correlational studies constitute other forms of descriptive research. Correlational studies determine and analyze relationships between variables as well as generate predictions. Descriptive research generates data, both qualitative and quantitative, that define the state of nature at a point in time. This chapter discusses some characteristics and basic procedures of the various types of descriptive research.
This is a preview of subscription content, log in via an institution to check access.
Access this chapter
Subscribe and save.
- Get 10 units per month
- Download Article/Chapter or eBook
- 1 Unit = 1 Article or 1 Chapter
- Cancel anytime
- Available as PDF
- Read on any device
- Instant download
- Own it forever
- Compact, lightweight edition
- Dispatched in 3 to 5 business days
- Free shipping worldwide - see info
- Durable hardcover edition
Tax calculation will be finalised at checkout
Purchases are for personal use only
Institutional subscriptions
Unable to display preview. Download preview PDF.
Achterberg C. Qualitative methods in nutritional education evaluation research. J Nutr Educ 1988; 20: 244
Article Google Scholar
Agar, M.H. The Professional Stranger: an Informal Introduction to Ethnography. Academic Press, New York: Academic Press 1980.
Google Scholar
Amstrong B., Doll R. Environmental factors and cancer incidence and mortality in different countries, with special reference to dietary practices. Int J Cancer, 1975; 15:617–631
Bang H.O., Dyerberg J., Hjorne, N. The composition of food consumed by Greenland Eskimos. Acta Med Scand 1976; 200: 69–75
Article CAS Google Scholar
Bailey, K.D. Methods of Social Research. New York: Free Press, Macmillan Publishing Co, Inc, 1978.
Beal V.A. The nutritional history in longitudinal research. J Am Dietet A 1967; 51: 526–531
Berdie D.R. Questionnaire length and response rate. J Appl Psychol 1973; 58:278–280
Berdie, D.R., Anderson J.F., Niebuhr, M.A. Questionnaires: Design and Use. Metuchen, N.J.: Scarescrow Press, 1986.
Buell P. Changing incidence of breast cancer in Japanese-American women. JNCI 1973; 51:1479–1483
CAS Google Scholar
Dyberg J., Bang H.O., Hjorne N. Fatty acid composition of the plasma lipids in Greenland Eskimos. Am J Clin Nutr 1975; 28: 958–961
Frank G. Life history model of adaptation to disability: the case of a congenital amputee. Soc Sci Med 1984; 19: 639–645
Fetterman, D.L. “A Walk Through the Wilderness: Learning to Find Your Way.” In Experiencing Fieldwork: An Inside View of Qualitative Research. Shaffir, W., Stebbins, R. eds. Newbury Park, CA: Sage, 1991.
Fieldhouse, P. Food & Nutrition: Customs & Culture. New York: Croom Helm, 1986
Fielding, N.G., Fielding, J.L. Linking Data. Beverly Hills, CA: Sage, 1986.
Firestone W.A. Meaning in method: The rhetoric of quantitative and qualitative research. Educational Researcher 1987; 16:16–21
Geertz, C. The interpretation of Cultures. New York: Basic Books, 1973.
Glaser B.G., Strauss, A.L. The Discovery of Grounded Theory: Strategies for Qualitative Research. New York: Aldine Publishing Co, 1967.
Goldberger, J.E. Goldberger on Pellagra. Baton Rouge, LA: Louisiana State University Press, 1964.
Greer J.G. What do open-ended questions measure? Public Opinion Quart 1988; 52:365–371
Gordis, L. Epidemiology. Philadelphia PA: Saunders, 1996.
Hammersley, M., Atkinson, P. Ethnography: Principles in Practice. London: Tavistock, 1983.
Headland, T.N., Pike, K.L., Harris, M. Emics and Etics: The Insider/Outsider Debate. Newbury Park, CA: Sage, 1990.
Hodge, R., Kress, G. Social Semiotics. Ithaca, NY: Cornell University Press, 1988.
Holbrook J.T., Patterson K.Y., Bodner J.E., Douglas L.W., Veillon C., Kelsey J.L. Mertz W, Smith J.C. Sodium and potassium intake and balance in adults consuming self selected diets. Am J Clin Nutr 1984; 40: 786–793
Human Nutrition Information Service, US Department of Agriculture, Food Consumption: Households in the United States, Spring 1977, Washington, D.E.: Government Printing Office, Publication H-1, 1982.
ICNND (International Committee on Nutrition for National Defense) Manual for Nutrition Surveys. Second edition. Superintendent of Documents. Washington, D.C.: U.S. Government Printing Office, 1963
Jacob E. Qualitative research traditions: A review. Rev Educ Res 1987; 57: 1–4
Jelliffe, D.B. The Assessment of the Nutritional Status of the Community. WHO Monograph 53. Geneva: World Health Organization, 1966.
Keys, A. Seven Countries: A Multivariate Analysis of Death and Corornary Heart Disease. Cambridge, MA: Harvard University Press, 1980.
Kittler, P.G., Sucher, K. Food and Culture in America. New York: Reinhold, 1989.
Kirk, J., Miller, M.L. Reliability and Validity in Qualitative Research. Beverly Hills, CA: Sage, 1986.
Koh E.T., Caples V. Nutrient intake of low-income, black families in Southwest Mississippi. J Am Dietet A 1979; 75:665–670
Kolonel, L.N., Hinds, M.W., Hankin, J.H. “Cancer Patterns Among Migrant and Native-Born Japanese in Hawaii in Relation to Smoking, Drinking, and Dietary Habits.” In Genetic and Environmental Factors in Experimental and Human Cancer. Gelboin, H.V. et al. eds. Tokyo, Japan: Science Press, 1980.
Kromann N., Green A. Epidemiological studies in the Upernavik district, Greenland: Incidence of some chronic disease 1950–1974. Acta Med Scand 1980; 401–405
Krueger, R. Focus Groups: A Practical Guide for Applied Rese arch. Newbury Park, CA: Sage, 1988.
Langness, L.L. Frank, G. Lives: An Anthropological Approach to Biography. Novato, CA: Chandler & Sharp, 1981.
Lind, J. (1753) A Treatise on the Scurvy. Reprinted Edinburgh: Edinburgh University Press, 1953.
Lock, L.F. “The Question of Quality in Qualitative Research.” In Proceedings of the 5th Measurement and Evaluation Symposium. Nelson, J.K. ed. Baton Rouge, LA: Louisiana State University Press, 1987.
Lofland, J., Lofland, L. Analyzing Social Settings: A Guide to Qualitative Research. Belmont, CA: Wadsworth, 1984.
Marshall, C; Rossman, G.B. Designing Qualitative Research. Newbury Park, CA: Sage, 1989.
Mauser, J.S., Kramer, S. Epidemiology — An Introductory Text. Philadelphia PA: Saunders, 1985.
McClendon M.J., O’Brien D.J. Question-order effects on the determinants of subjective well-being. Public Opinion Quart 1988; 52:351–364
McCraken, G. The Long Interview. Newbury Park, CA: Sage, 1988.
McMichael A.J., McCall M.G., Hartshorne J.M., Woodings T.L. Patterns of gastro-intestinal cancer in European migrants to Australia: The role of dietary changes. In J Cancer 1980; 25:431–437
Miles, M.B., Huberman, A.M. Qualitative Data Analysis: A Sourcebook of New Methods. (2nd ed.) Thousand Oaks, CA: Sage, 1994.
Montgomery A.C., Crittenden K.S. Improving coding reliability for open-ended questions. Public Opinion Quart 1977; 41: 235–243
National Center for Health Statistics: Plan and Operation of the HANES. Vital and Health Statistics, Series 1, Nos 10a and 10b, DHEW Pub No (HSM) 73–130. Washington, D.C.: U.S. Government Printing Office, 1973.
O’Brien T., Dugdale V. Questionnaire administration by computer. J Market Res Soc, 1978; 20:228–237
Okolo, E.N. Health Research Design and Methodology. Boca Raton, FL: CRC Press 1990.
Parkin, D.M., Muir, C.S., Whelan, S.L., Gao, Y.T., Ferlay, J., Powell, J. Cancer Incidence in Five Continents VI IARC Sci Publ 120, Lyon: International Agency for Research on Cancer, 1992.
Peterkin B.B., Rizek R.L., Tippett K.S. Nationwide food consumption survey, 1987. Nutr Today 1988; 23:18–24
Poe G.S., Seeman I., McLaughlin J., Mehl E., Dietz M. Don’t know boxes in factual questions in a mail questionnaire: Effects on level and quality of response. Public Opinion Quart 1988; 52: 212–222
Poikolainen K., Karkkainen P. Nature of questionnaire options affects estimates of alcohol intake. J Stud Alcohol 1985; 46: 219–222
Potter, J.D. Food, Nutrition and the Prevention of Cancer: A Global Perspective. Washington, D.C.: American Institute for Cancer Research, 1997.
Rose, G.A., Blackburn, H. Cardiovascular Survey Methods. Geneva, Switzerland: World Health Organization 1968.
Runcie, J.F. Experiencing Social Sresearch. Homewood, IL.: Dorsey Press, 1976.
Sandelowski, M. The Problem of Rigor in Qualitative Research. Adv Nurs Sci April 27–37, 1986.
Schutz R.W. Qualitative research: comments and controversies. Research Quarterly Exercise Sport, 1989; 60:30–35
Sheatsley, P.B. “Questionnaire Construction and Item Writing.” In Handbook of Survey Research. Rossi, P.H., Wright, J.D., Anderson, A.B. eds. Orlando, FL: Academic Press, 1983.
Sherry, B. “Epidemiologic Analytical Research.” In Research. Monsen, E.R. Chicago, IL: American Dietetic Association, 1992
Spradley, J. The Ethnographic Interview. Chicago, IL: Holtz, Rinehart & Winston, 1979.
Spradley, J.P. Participant Observation. New York: Holt, Rinehart & Winston, 1980.
Sudman, S., Bradburn, N.M. Asking Questions: A Practical Guide to Questionnaire Design. (2nd ed.) Washington, D.C.: Jossey-Bass Publisher, 1983.
Taylor, S.J., Bogdan, R. Introduction to Qualitative Research Methods. (2nd ed.) New York: Wiley, 1984.
Thomas, J.R. Nelson, J.K. Research Methods in Physical Activity. (3rd ed.), Champaign, IL: Human Kinetics, 1996.
Van Maanen, J. ed. Qualitative Methodology. Newbury Park, CA: Sage, 1983.
Willett, W. Nutritional Epidemiology. (2nd ed.) New York: Oxford University Press, 1998.
Book Google Scholar
Download references
Author information
Authors and affiliations.
University of Oklahoma Health Sciences Center, USA
Eunsook T. Koh & Willis L. Owen
You can also search for this author in PubMed Google Scholar
Rights and permissions
Reprints and permissions
Copyright information
© 2000 Springer Science+Business Media New York
About this chapter
Koh, E.T., Owen, W.L. (2000). Descriptive Research and Qualitative Research. In: Introduction to Nutrition and Health Research. Springer, Boston, MA. https://doi.org/10.1007/978-1-4615-1401-5_12
Download citation
DOI : https://doi.org/10.1007/978-1-4615-1401-5_12
Publisher Name : Springer, Boston, MA
Print ISBN : 978-1-4613-5535-9
Online ISBN : 978-1-4615-1401-5
eBook Packages : Springer Book Archive
Share this chapter
Anyone you share the following link with will be able to read this content:
Sorry, a shareable link is not currently available for this article.
Provided by the Springer Nature SharedIt content-sharing initiative
- Publish with us
Policies and ethics
- Find a journal
- Track your research
- Open access
- Published: 11 September 2024
Descriptive study showing the effect of small differences in entry scores on retention and success in six courses in nursing
- Sheila A. Doggrell 1
BMC Nursing volume 23 , Article number: 639 ( 2024 ) Cite this article
49 Accesses
Metrics details
Undergraduate enrolments and completions in nursing are declining at the same time as requirements for registered nurses in the workforce is increasing. One way to increase enrolments is to lower entry requirements into nursing programs. This study determined the effect of a small lowering of entry scores on the retention and success rates. The study was undertaken at Griffith University, where identical bioscience and clinical courses run at three campuses, one with a lower- than normal-entry score and two with the normal-entry requirements.
A comparison of the 62–148 students enrolled in the courses at the lower- than normal- (lesser) entry requiring campus with the 197–288 students enrolled in the courses in one normal-entry requiring campuses. For individual courses (4 bioscience and 2 clinical courses) retention rates were measured and compared between campuses/cohorts. For completing students, we measured success as (i) Academic outcomes; overall marks and marks for the exam and coursework and (ii) Failure rates in the examination and coursework. Data analysis was by Student’s t-test and Odds ratio.
For all courses, there were few significant differences in measures of retention and success between the lower- and normal-entry campuses, and all differences were small.
Comparing the campuses; (a) For all courses, progression rates were similar. (b) For completing students, passing rates were similar in the bioscience courses, but higher in the normal- than lower-entry campus for the clinical courses. (c) In the bioscience courses, the overall marks were similar, but in the clinical courses, the overall mark was slightly higher in the normal- than lower-entry campus. (d) The overall failure rates were similar for the biosciences, but higher for the lower- than normal-entry cohort in the clinical courses. (e) The failure rates in exams were similar between cohorts in all courses.
Conclusions
This study shows that small changes in entry requirements for a nursing program had minimal effects on retention. The differences in entry requirements also did not affect the success of students in the bioscience courses. Decreasing entry requirement for nursing programs may be a way to increase nursing graduates.
Peer Review reports
Introduction
There are presently, and impending increasing, shortages in the nursing workforce in many counties (USA [ 1 ], UK [ 2 ], Australia [ 3 ]). At the same time, enrolments into nursing programs are falling (USA [ 1 ], UK [ 2 ], Australia [ 4 ]). Improving the retention (or decreasing attrition), and increasing success (or decreasing failures), and increasing the enrolments of students in nursing programs are possible ways to increase nursing graduates.
Retention in nursing programs is poor and decreasing in some countries. Discussing retention, the American Association of Colleges of Nursing reported that the completion (retention) rates for nursing programs in the US.A. from 2021 to 2022 decreased [ 1 ]. In the UK, between 2014–2020, 13% of students in nursing did not complete [ 5 ]. The Australian government reported, in the latest university data, that the attrition rates for students in nursing increased from 2020 to 2021, where it was ~ 15%, and the six-year completion rates were the lowest on record in 2022 [ 4 ].
In nursing programs, there is evidence the students may have more problems in passing some courses (including the bioscience courses) than the clinical courses [ 6 ]. The factors contributing to this include the large body of facts that students must learn in the biosciences (anatomy, physiology, and pharmacology), poor study skills, and inappropriate entry requirements for science courses [ 6 ].
Worldwide, entry to university courses is still predominantly based on prior achievement levels/academic performance in educational institutions such as school or technical colleges. These achievement levels are usually higher for the more prestigious or demanding courses. A reason for using prior achievement levels as an entry requirement is that it is generally considered to be a predictor of success at university (e.g., health sciences, [ 7 ]).
Most studies of the predictors of success in nursing have been in programs, with little attention to courses. In reviewing, the predictors of nursing studies success in programs, Mthimunye & Daniels [ 8 ] showed that this included both academic performance measures and non-academic measures (e.g., older students, female gender, English language proficiency etc.). The academic performance measures that predict success include high admission Grade Point Average (GPA), high supplemental application score, high pre-admission science GPA [ 9 ]. In a review limited to the USA, Capponi et al., confirmed that both academic and non-academic program criteria predict success in nursing programs, with GPA being the most used criterium [ 10 ].
In the USA, the Test of Essential Academic Skills (TEAS) is a standardised, multiple-choice exam for entry into nursing, and higher scores predict higher GPAs during the program, and higher rates of graduation [ 11 , 12 ]. The students with higher pre-admission GPAs had a higher chance of graduation [ 11 , 13 ]. Science GPA and scores on the Health Educational Systems Incorporated Admission Assessment anatomy and physiology subscales are also predictors of success in nursing programs in the USA [ 13 ]. For the clinical courses in nursing, the scores in the Nursing Entrance Test (NET) Reading and Math components, remain predictors of success and retention in the USA [ 14 ].
In the UK, the number of General Certificate of Secondary Education (GCSE) Advanced levels obtained predicts success in a nursing degree [ 15 ]. Similarly, in Australia, when the University Admissions Index (UAI) was being used in New South Wales to rank Year 12 students, it was shown to be the best predictor of success in nursing programs [ 16 ]. Previous study of high school biology was associated with an increased bioscience course and overall GPA in nursing programs [ 16 ]. In New Zealand, it was shown that entry requirements for nursing had a higher correlation with academic performance in the first- than second-year bioscience course [ 17 ].
Worldwide there are several initiatives being undertaken to improve nursing student retention, success, and enrolments at university. Retention, success, and enrolments at university should not be considered in isolation, as they are not separate identities. One way to increase student enrolments, which has not been considered in detail, is to reduce the entry requirements. However, major considerations need to be given before doing this, as higher entry scores have been shown to predict more success, but also more completions and lower attrition rates.
Previous studies about retention and success in nursing have predominantly been in programs with little information on predictors in courses. From comparing the outcomes of students with the highest and lowest entry requirements in a program, it has been suggested that entry scores are a major predictor of both retention and success in nursing programs with students with the highest entry requirements having the best outcomes. The implication of this, is that dropping the entry score requirements for nursing students in programs will reduce retention and success.
However, none of the studies have directly considered what would happen to retention and success in program or courses if the entry requirements to programs were reduced. Thus, our research question is: Will dropping the entry score requirements have a major effect on retention and success rates for students in courses in a nursing program? At our Australian university, we have three campuses that run identical nursing programs. The only difference is that the entry requirements are lower in one than the other campuses. Our hypothesis was that the campus with the lower entry requirements would have lower retention and success rates. Thus, for individual courses at the lower- and at the normal-entry campuses, we measured and compared between campuses retention and success. Retention was measured as.
Overall completion rates and completion rates of student passing or failing.
Students who could potentially progress or not from than course. The non-progression rate was defined as the combination of enrolled students who did not complete plus the completing students who failed, and for completing students we measured success.
Academic outcomes; overall marks and marks for the exam and coursework
Failure rates in the examination and coursework
As we wanted to ascertain whether our findings related to a variety of courses, analysis was undertaken for six courses.
Ethics approval and consent to participate
Ethical approval was obtained for this project from the University Human Research Ethics Committee (UHREC) at Griffith University (Gold Coast Campus, Parklands Drive, Southport, QLD 4222, Australia); Ethics Approval Number 2022/944. The UHREC is constituted and operates in accordance the National Statement on Ethical Conduct in Human Research (2007) and registered by the National Health and Medical Research Council (Australia). Under this approval from the UHREC, informed consent from individual students was waived. Student anonymity was achieved by removing names and students’ IDs from the marks data prior to the study. All methods were performed in accordance with the relevant guidelines and regulations of the UHREC.
Entry requirements for enrolment
In Australia, at the completion of secondary education, school leavers are given an Australian Tertiary Admission Rank (ATAR, 0 – 99.95; highest), which determines which courses they are eligible for at university. The ATAR is equivalent to the high school diploma in North America, and the General Certificate of Education (GCE) A Level in England and Wales.
The nursing degree at Griffith University is identical at three campuses. One of the campuses is in a low socio-economic index area (SEIFA) whereas the other two are in high SEIFAs [ 18 ]. To obtain sufficient enrolments to run the program on the campus in the lower SEIFA, it has a ‘lower’ entry requirement than the other two high SEIFA campuses, which had the ‘normal’ entry requirement. Thus, in 2022, the ATAR was 81 in the lower SEIFA (‘lower’ entry requirement) and 86 in the high (‘normal’ entry) and in 2021, 78 vs 82, respectively.
Study design
The design is a descriptive and comparative study of the outcomes for the students in nursing at three campuses with different entrance requirements: two campuses with normal-entry and one campus with lower than normal-entry. As the results from the normal-entry campuses were identical, we chose to only present the data from one campus, which was randomly chosen. To be able to include data from all the students in each course, we obtained a waiver from seeking consent (previous section).
Research setting
The research was undertaken in an Australian university, where students are typically required to achieve an overall mark of 50% to pass a course and passing grades are 4 (overall mark, 50–64%), 5 (65–74%), 6 (75–84%) and 7 (≥ 85%). In all courses, students with < 50% of the overall marks failed, and in the clinical courses, students who did not undertake the clinical placement, also failed.
Participants
Almost all the students undertaking the courses in Anatomy & Physiology (A & P) have entered the Bachelor of Nursing program directly after completing either secondary school education with ATAR scores or tertiary enabling/preparation courses. The tertiary enabling course at Griffith university (EmpowerED University Preparation Program) is for students who do not meet the formal admission requirements. Success in this program, and subject to the English language requirements, students can undertake the Bachelor of Nursing.
Students with a Diploma of Nursing (Enrolled) nurses from Australia and Internationally Qualified Nurses are given credit in recognition of prior learning in the A & P courses, and do not undertake these courses. These students are known as Advanced standing students.
After completion or credit for the A & P course, the students (direct entry and advanced standing entry) undertook two courses in Human Pathophysiology and Pharmacology (Path & Pharm 1 and 2) and clinical courses in Chronic Illness Management (Chronic illness) and Mental Health Nursing Practice (Mental health).
After census date (i.e., last date when students can withdraw without financial penalty), between 62–77 students were enrolled in the courses at the lower entry score campus and between 174–288 were enrolled at the normal entry score campus (Table 2 ).
The courses chosen were to represent different stages of the BNursing program and participants. Thus, the A & P courses were chosen to represent the early stages of the program with only direct entry students. The Path and Pharm courses were chosen to represent the mid-year of study with both direct entry and advanced standing students. The clinical courses were chosen to determine whether there were differences between the findings for Path and Pharm bioscience courses and clinical courses for all students. Details of the courses (semester, pre-requisites, method of teaching, assessment) are given in Table 1 .
Marking was not limited to the staff that taught at each campus. It was distributed among staff from all campuses to help nullify any differences between markers.
Data collection procedures
The author was not involved in the teaching and marking of any of the courses. The coordinators of the nursing courses gave their permission to the Nursing program co-ordinator to provide a copy of the Microsoft Excel sheets of the marks associated with the courses to the author. The data was from semesters 1–4 in 2022 and was the starting point for the following analysis. Mean values, and Student’s unpaired tests were performed using the data analysis function in Microsoft Excel. In the analysis, P ≤ 0.05 was considered significantly different.
Retention in individual courses
For each course, the data at the lower- and normal- entry levels were compared using the Odds ratio. The Odds ratio is the odds that an outcome will occur with a particular exposure (in this case, the lower-entry requirement) compared to the odds of the outcome occurring in the absence of that exposure (normal-entry requirement). The larger the Odds ratio, the more likely there is a difference. When the 95% confidence interval (CI) of the Odds ratio does not include 1.0, the Odds ratio indicates a significant difference between groups. The Odds ratios were calculated using the online Odds ratio calculator, https://www.medcalc.org/calc/odds_ratio.php . [ 19 ], which provides computation notes, and gives the Odds ratio, 95% CI, z statistic, and Significance level ( P value).
Data analysis for students enrolled on census date who withdrew early.
The number and percentage of students enrolled on census date who withdrew early from each course was tabulated.
Students enrolled on census day who completed with passing or failing.
For those who were enrolled on census day, the number/percentage of students who completed and passed or failed were tabulated. Some of students who failed after census day may have done so because they were considered to have completed, as they had not formally withdrawn.
Non-progression rates
The numbers of students who could not progress in, or complete the program, defined as the combination of enrolled students on census day who withdrew early and of students who completed but failed, were also tabulated. These students could not complete the program until they had passed this course. In addition, of the courses studied, students who did not pass the Path & Pharm 1 course could not enrol in the Chronic Illness course (Table 1 ).
Success in courses
The assessment and weighting of the assessment in each course is given in Table 1 . The methods for measuring success in courses (percentage of students who obtained ≥ 5 0% of marks overall) has been described previously [ 20 ].
Success: For the students enrolled on census date who completed, data analysis comparing overall academic performance, and performance in coursework and the exam
The marks for the combined coursework, the components of the coursework, and the exam were totalled, the total expressed as a percentage, and then the percentages were averaged. Mean values ± SD were also determined. For each course, the percentages at the entry levels were compared using Students unpaired t-test.
Success: For completing students, data analysis comparing failure rates in examination and coursework
Students who achieved less than 50% in the coursework, or the exam, were considered to have failed that component. The failure rates for each component were compared by Odds ratio between the entry levels.
Results are from 2022. As the results of the study were identical for the two campuses with normal-entry requirements were identical, the results of only one of these is presented.
In any year, first year school leaver/tertiary enabled students undertake A & P 1 and 2. At the same time, second-year full-time students (school leavers/tertiary enabled in 2021 and advanced standing students in 2022) undertook Path & Pharm 1 and 2, and the clinical courses.
Retention: students enrolled on census date who withdrew early, students enrolled on census date who completed with passing, and non-progression rates
The numbers and percentages of these are given in Table 2 . Between the entry requirement levels, odds-ratio analysis with P values are shown (Table 2 ).
The students enrolled on census date who withdrew early were similar for each course, except the Mental health course. In the Mental health course, the students who withdrew early was significantly higher in the normal- than lower-entry campus by 4% percentage points.
The passing rates for completing students enrolled, on census date, were similar in bioscience courses. For the clinical courses, the passing rates for completing students were significantly higher by 8–11% points in the normal- than lower-entry campus.
The non-progression rates (the combination of enrolled students who withdrew early and of completing students who failed) were similar for all courses.
Success: For the students enrolled on census date who completed
The mean percentage marks ± standard deviation in examination and coursework are given in Table 3 .
Between the entry requirement levels, unpaired t-test comparisons with P values showed that in the bioscience courses (Table 3 ).
The overall marks, combined coursework marks and quiz marks were similar in the bioscience courses.
The marks for the concept maps were similar in both Path and Pharm courses. The written assignment marks were also similar in the A & P 2 courses.
In the A & P 1 course, the written assignment mark was significantly higher in the cohort with lower- than normal-entry requirement by 6.2% points.
Between the entry scores, unpaired t-test comparisons with P values showed that in the clinical courses (Table 3 ).
The overall mark for students in the Chronic illness course, was significantly less in the lower- than normal- entry cohort by 3.8% percentage points. This was due to significantly lesser marks in the coursework marks due to a lesser mark in the clinical simulation component by 10.5% points.
In the exam and written assignment, there were no significant differences in marks.
The marks for the coursework were also similar in the Mental health course.
Success: failure rates for students, enrolled on census date, in overall marks, and its components
Between the entry requirement levels, in the bioscience courses, Odds ratio testing with P values, showed (Table 4 ):
The overall failure rates were similar.
In exams, the failure rates were similar.
In the combined coursework, there were no differences in failure rate for the A & P 1, and Path & Pharm 1 and 2.
The failure rates for the combined coursework were significantly greater for the lower-than normal-entry students in the A & P 2 by 11.6% points. This lower coursework mark was due to the lower-entry cohort having greater failure rates in both the quizzes and the written assignment by 8.2% and 7.8% points, respectively.
Comparison in the clinical courses by Odd-ratio testing showed that failure rates were greater in the lower-than normal entry cohorts, which was either a significance or a trend (Table 4 ). Thus:
The overall failure rates were significantly greater for the lower- than normal- entry cohort in the clinical courses by 11.0% and 7.3% percentage points in the Chronic illness and Mental health courses, respectively.
In the lower- than normal-entry cohort, the failure rates in the exam were greater in the Chronic illness course by 6.7 percentage points but this did not reach significance. The failure rates in the Mental health course were similar (0.8 percentage difference).
In the Chronic illnesses course, the failure rates for the combined coursework were significantly greater for the lower than normal-entry students by 17.5% points. This was due to the lower entry cohort having greater failure rates in both the clinical simulation and written assignment by 17.7% and 11.8% points, respectively.
In the Mental health course, there were no significant differences in failure rates in coursework.
In summary, there are more similarities than differences in retention and success rates between entry levels, and the differences did not always favour the normal- over lower-entry campus:
For five of the six courses the students enrolled on census date who withdrew early were similar. In one clinical course, the early withdrawal rate was higher in the normal- than lower-entry campus.
For all courses, the progression rates were similar.
For the bioscience courses, the passing rates for completing students were similar, whereas in the clinical courses, the passing rates for completing students were higher in the normal- than lower-entry campus.
In the bioscience courses and Mental Health course, the overall marks, combined coursework marks and quiz marks were similar. The marks for the written assignment were higher in the campus with lower- than normal-entry requirements in the A & P 1 course, but similar in the other bioscience courses and Mental Health course.
In the Chronic Illness course, the overall mark was slightly less in the lower- than normal-entry cohort but there were no differences in the exam and written assignment marks.
The overall failure rates were similar for the biosciences, but greater for the lower- than normal-entry cohort in the clinical courses. The failure rates in exams were similar between cohorts in all courses.
This summary shows little support for our hypothesis that the campus with the lower-entry requirements would have lower retention and success rates. Thus, the differences between the campuses with normal- and lower-entry requirements were few and small. Importantly, these differences were not detrimental to the lower-entry cohort in retention measured as early withdrawal rates or non-progression rates in all courses. The differences in entry requirements also did not affect the success of students, measured as either overall marks or overall failure rates in the bioscience courses.
In our study we measured retention and success from individual courses, not from a program. This a major difference from most previous studies, which related entry requirements to measures of retention, and success for the top- and bottom-entry marks from a program, without considering the students with middle-entry requirements. This makes a direct comparison not possible for several reasons, including that we included all students in each course. Thus, in this discussion we initially consider our results as stand alone, and then we make an indirect comparison to previous studies.
In considering students from two campuses in our study, difference in completion rates could potentially have affected the success/ academic outcomes of completing students. For instance, if the completion rates were higher or lower at one campus than the other, this could have biased the sample of the completing students to change the success rates/ academic outcomes of the completing students. Thus, in a course with a lower completing rate, this may be associated with a loss of the less able students and could increase the success rates academic outcomes of the completing students. However, in the present study, the completion rates were similar for all the bioscience courses, and one of the clinical courses. Thus, completion rates could not be a major factor in any differences between success/academic outcomes in the courses with similar completion rates.
However, bias may have been a factor in differences for completing students in one of the clinical courses (Mental Health), where the completion rate was slightly less for the students with the normal- than lower-entry requirements. This could have led to higher passing and lower failure rates, and this was the finding associated for the normal- than lower-entry completing students. However, there was no difference in the overall marks and marks for the exam and coursework for the completing students between the cohorts in this clinical course. This shows, if they did have an effect, that small differences in completion rates did not have major effects. Again, this illustrates very modest difference in retention and success with lower-entry requirements.
In our study, the overall non-completion rates were similar between entry requirements in the bioscience courses, and the Chronic Illness course. In contrast, in the Mental Health course, the overall non-completion rates were higher in the normal- than lower-entry cohort. It is worth noting that the difference in non-completion rates between the normal and lower-entry cohorts in the Mental Health course favours the lower-entry cohort, who had higher completion rates than the normal-entry students. This emphasises that not only did differences occur in a small number of the parameters of retention and success that we measured but, that the differences did not always favour the normal entry requiring campus.
It is possible that entry-requirements (e.g., ATAR scores) are more likely to predict outcomes in the initial than later courses of a program, as these initial outcomes will be prior to exclusion of students who do not complete or failure. In this study, there were several parameters that we measured that were similar between the different entry requirements in the bioscience courses. These included early withdrawal rates, progression rates, overall marks, and failure rates. As these similarities occurred in both A & P courses, which are in the first year of the program, they strongly suggest that the small difference in entry scores between campuses had no effect on effect on the academic performance at this stage of the program.
Although selected for their differences in entry levels, it was noted that the campuses were in different SEIFAs. SEIFA has a scale of 1 to 5, with 5 being the highest social economic index. The lower- than normal-entry level campus was in area 2, whereas the normal-entry was in the highest area (scale = 5, [ 19 ]). This may suggest that lowering entry requirements to a small extent in areas of lower social economic index does not affect outcomes in bioscience courses. Thus, lowering entry levels in area of low social economic index requires further exploration as a method to increase the number of students in nursing.
In contrast, to the results in the initial bioscience courses there were a few parameters that we measured that favoured the normal- over the lower-entry requirements in one or both clinical courses. These differences included completion with passing or failing rates, overall marks, combined coursework marks, and quiz marks. The reason for this is unknown, but it may not be related to entry requirements, as the students were already in their second semester of second year when undertaking the clinical courses (Table 1 ). One possibility is that, as they were students in nursing, they may have had a higher aptitude for clinical than bioscience courses. This possibility is supported by the finding that in the same semester (4th semester), there were few differences between the normal- over the lower-entry requirements in the bioscience course (Path & Pharm 2) but the outcomes were better for the normal- than the lower-entry requirements in the clinical courses. Previous studies have also suggested that students in nursing have better outcomes in clinical than bioscience courses [ 6 ].
Another reason may be that the clinical courses have different populations of students enrolled. The enrolments in the A & P courses were mostly school leavers whereas advanced standing students joined in the Path & Pharm 1 course, and then went on to undertake the clinical courses. As there were no difference in the retention and success rates between entry requirement levels in the Path & Pharm 1 and 2 courses, it seems unlikely the inclusion of advanced standing influenced the outcomes in these courses.
At Griffith University, there are two campuses with normal-entry and one with lower-entry. We did undertake a full analysis of both normal-entry campuses, and there were no differences between them, and consequently we presented data from one of them that was randomly chosen. That there were no differences between the two normal-entry requiring campuses verifies that the program is identical on the two campuses and suggests that the small differences between the normal- and lower-entry campuses presented here are real.
Our results from all the students in individual courses are difficult to compare to results from previous studies comparing highest- and lowest-entry students within courses or in programs discussed in the Introduction. These previous studies have probably not surprising suggested that higher entry scores are clearly associated with better retention and success in either a course or a program. Possible explanations for this difference with our study is that, previous studies have been within one course or one program and have compared the students with the highest and lowest entry marks for retention and success within that course/program and shown the students with the highest entry marks had the best retention/success [ 8 , 15 , 16 ]. Typically, studies have compared the of students in a program from the top-half to the bottom-half of entry qualifications, and consequently, the differences in the entry scores for the groups will be large. If we had done this, we might have confirmed previous results. However, we compared all the students in identical courses at two campuses with only small differences in overall entry requirements, rather than the top and bottom entry students in a single course. A second explanation for the difference between our study is that, not only did the present study use a different protocol to previously, but also looked at much smaller differences in entry requirements: 5 and 4 points on the ATAR scale in 2021 and 2022, respectively. With this small difference, we did not find a clear-cut difference between those students who overall had met a lower- than at the other normal-entry requiring campus.
Implications
The finding of this study has implication for enrolments in nursing, which are falling, while requirement for nurses in increasing. Thus, undergraduate enrolments in the U.S.A. have been declining since 2011/12 [ 1 ]. The American Association of Colleges of Nursing have reported that enrolments in nursing declined by 1.4% or ~ 3,500 students from 2021 to 2022 [ 1 ]. In the future, the increased requirements for nurses will far outstrip the supply [ 1 ]. In the UK, the Nursing Times reported that undergraduate enrolments in nursing are down 12% [ 1 ]. In the UK, the present shortfall in registered nursing in the NHS workforce is over 40,000 and government attempts to recruit more nurses have failed [ 21 ].
In line with overseas trends, the Australian government reported in the latest university enrolment data that the number of enrolled domestic students declined from 2021 to 2022. In nursing, there was a 10% reduction in enrolments between 2018 and 2022, which predominantly occurred between 2021 and 2022. In addition, the attrition rates for nursing students increased from 2020 to 2021, where it was ~ 15%, and the six-year completion rates were the lowest on record in 2022 [ 4 ]. It is projected that Australia will face a shortage of over 100,000 nurses within the next three years [ 3 ].
This study suggests that the entry requirements for students in nursing can be lowered without major effects on retention and success. This may be a way of increasing enrolments and graduates in nursing.
Limitations
As we did not get consent from individual students, our study is not following students as they progress through the program. Rather the data is from all the students in the courses that ran in 2022. This allowed us to include all students in each of the courses, rather than limiting the study to consenting students. The downside of this is that we cannot relate entry requirements for individual students as they progress through the program to their retention and success.
The students in the A & P courses were secondary school leavers or equivalent, who were joined by Advanced standing students for the Path & Pharm and clinical courses. We also did not have information from the students as to whether they were school leavers or advanced standing students. This information may have been a useful addition to the study. Consequently, we asked the University for this information in numbers to fit with the ethics waiver. However, this information is not available for individual courses, only for the programs.
The major strength of this study was that it included courses that were identical between campuses/cohorts, which made it a direct comparison between entry-levels requirements. This contrasts with previous studies that have predominantly compared students with higher and lower-entry qualifications in the same program. Another strength was that the study included both bioscience and clinical courses, whereas most previous studies of courses have been in the biosciences. Finally, the study was investigating differences at course level between entry-level scores in a single program.
This study, which is limited to one program at one school, suggests that small changes in entry requirements for a nursing program had minimal effects on retention. The differences in entry requirements also did not affect the success of students in the bioscience courses. Decreasing entry requirement for nursing programs may be a way to increase nursing graduates.
Availability of data and materials
The datasets used and/or analysed during the current study are available from the corresponding author on reasonable request.
American Association of Colleges of Nursing. New data showing enrollment declines in schools of nursing, raising concerns about the nation’s nursing workforce. https://www.aacnnursing.org/news-data/all-news/new-data-show-enrollment-declines-in-schools-of-nursing-raising-concerns-about-the-nations-nursing-workforce . Accessed 13 Feb 2024.
Nursing Times. Student nurse university starters remain down 12%. https://www.nursingtimes.net/news/education/student-nurse-university-starters-remain-down-22-09-2023/ . Accessed 13 Feb 2024.
Mannix K. The future of Australia’s nursing workforce: COVID-19 and burnout among nurses. December 2021. https://www.unimelb.edu.au/__data/assets/pdf_file/0004/4085194/katelyn_mannix_report.pdf . Accessed 19 Feb 2024.
Australian Government Department of Education. Selected Higher Education Statistics – 2022 Student data https://www.education.gov.au/higher-education-statistics/resources/selected-higher-education-statistics-2022-student-data-key-findings . Accessed 13 Feb 2024.
Nuffield Trust Waste not want not https://www.nuffieldtrust.org.uk/sites/default/files/2023-12/Waste%20not%20want%20not-WEB-pdf.pdf . Last accessed 13 Feb 2024.
McVicar A, Andrew S, Kemble R. The ‘bioscience problem’ for nursing students: an integrative review of published evaluations of Year I bioscience, and proposed directions for curriculum development. Nurs Educ. 2015;35:500–9.
Google Scholar
Alhadlaq A, Alshammari OF, Alsager SM, Neel KAF, Mohamed AG. Ability of admission criteria to predict early academic performance among students of Health Science colleges at King Saud University. Saudi Arabia J Dental Educ. 2015;79:665–70.
Article Google Scholar
Mthimunye K, Daniels FM. Predictors of academic performance, success and retention amongst undergraduate nursing students: a systematic review. S African J High Educ. 2019;33:200–20.
Capponi N, Mason Barber LA. Undergraduate nursing admission criteria: a scoping review of the literature. Nurs Educ Today. 2020;92: 104519.
McCarthy MA, Harris D, Tracz SM. Academic and nursing aptitude and the NCLEX-RN in Baccalaureate programs. J Nurs Educ. 2014;53:151–9.
Article PubMed Google Scholar
Wambuguh O, Eckfield M, and Van Hofwegen L. Examining the importance of admissions criteria in predicting nursing program success. Int J Nurs Educ Scholarship 2016;13: https://doi.org/10.1515/ijnes,2015-0088 /.
Cunningham CJL, Manier A, Anderson A, Sarnosky K. Rational versus empirical prediction of nursing student success. J Prof Nurs. 2014;30:486–92.
Bennett M, Bormann L, Lovan S, Cobb B. Preadmission predictors of student success in a Baccalaurate of Science in nursing program. J Nurs Regulation. 2016;7:11–8.
Herrera C. Quantitative analysis of variables affecting nursing programs completion at Arizona State University. Res in High Educ J. 2013;21:1–18.
Wharrad J, Chapple M, Price N. Predictors of academic success in a Bachelor of Nursing course. Nurse Educ Today. 2003;23:246–54.
Whyte DG, Madigan V, Drinkwater EJ. Predictors of academic performance of nursing and paramedic students in first year bioscience. Nurse Educ Today. 2011;31:849–54.
Van Rooyer P, Dixon Am Dixon G, Wells C. Entry criteria as predictor of performance in the undergraduate nursing degree programme. Nurse Educ Today. 2006;26:593–600.
MedCalc. Free statistical calculators. Odd ratio calculator. https://www.medcalc.org/calc/odds_ratio.php . Accessed 24 June 2024
Queensland Government. Queensland Treasury. SEIFA (Social-Economic Indexes for Areas) https://www.qgso.qld.gov.au/statistics/theme/economy/prices-indexes/seifa-socio-economic-indexes-areas Accessed 19th June, 2024
Doggrell SA. Follow-up descriptive study of how proportioning marks between coursework and examination affects the performance of students in nursing. BMC Nurs. 2023;22(1):135. https://doi.org/10.1186/s12912-023-01286-w .
Article PubMed PubMed Central Google Scholar
Royal College of Nursing. ‘Dangerously inadequate staffing level’: NHS waiting list growing 4 times faster than nurse workforce. https://www.rcn.org.uk/news-and-events/news/uk-inadequate-staffing-levels-nhs-waiting-list-growing-4-times-faster-than-nurse-workforce-161123#:~:text=In%20England%2C%20patient%20demand%20has,the%20number%20of%20nurses%20recruited . Accessed 16 Feb 2024.
Download references
There was no funding of this research.
Author information
Authors and affiliations.
School of Pharmacy and Medical Sciences, Gold Coast Campus, Griffith University, Parklands Dr, Southport, QLD, 4222, Australia
Sheila A. Doggrell
You can also search for this author in PubMed Google Scholar
Contributions
SAD is the author and was responsible for the conception and design of the work, analysis and interpretation of data, and writing of the manuscript. SAD is the only writer of the submitted version and has approved submission and is personally accountable for it. SAD is the corresponding author. There are no other authors.
Corresponding author
Correspondence to Sheila A. Doggrell .
Ethics declarations
Ethical approval was obtained for this project from the University Human Research Ethics Committee (UHREC) at Griffith University (Gold Coast Campus, Parklands Drive, Southport, QLD 4222, Australia); Ethics Approval Number 2022/944. The UHREC is constituted and operates in accordance the National Statement on Ethical Conduct in Human Research (2007) and registered by the National Health and Medical Research Council (Australia). Under this approval from the UHREC, informed consent from individual students was waived.
Consent for publication
Not applicable.
Completing interests
The authors declare no competing interests.
Additional information
Publisher’s note.
Springer Nature remains neutral with regard to jurisdictional claims in published maps and institutional affiliations.
Rights and permissions
Open Access This article is licensed under a Creative Commons Attribution-NonCommercial-NoDerivatives 4.0 International License, which permits any non-commercial use, sharing, distribution and reproduction in any medium or format, as long as you give appropriate credit to the original author(s) and the source, provide a link to the Creative Commons licence, and indicate if you modified the licensed material. You do not have permission under this licence to share adapted material derived from this article or parts of it. The images or other third party material in this article are included in the article’s Creative Commons licence, unless indicated otherwise in a credit line to the material. If material is not included in the article’s Creative Commons licence and your intended use is not permitted by statutory regulation or exceeds the permitted use, you will need to obtain permission directly from the copyright holder. To view a copy of this licence, visit http://creativecommons.org/licenses/by-nc-nd/4.0/ .
Reprints and permissions
About this article
Cite this article.
Doggrell, S.A. Descriptive study showing the effect of small differences in entry scores on retention and success in six courses in nursing. BMC Nurs 23 , 639 (2024). https://doi.org/10.1186/s12912-024-02305-0
Download citation
Received : 06 May 2024
Accepted : 29 August 2024
Published : 11 September 2024
DOI : https://doi.org/10.1186/s12912-024-02305-0
Share this article
Anyone you share the following link with will be able to read this content:
Sorry, a shareable link is not currently available for this article.
Provided by the Springer Nature SharedIt content-sharing initiative
- Academic outcomes
- Bioscience/clinical courses
- Entry requirements
- Retention rates
- Students in nursing
BMC Nursing
ISSN: 1472-6955
- General enquiries: [email protected]
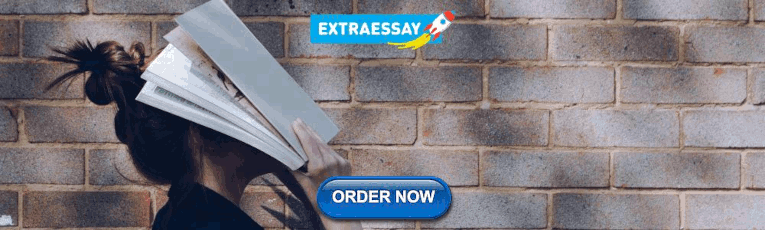
IMAGES
VIDEO
COMMENTS
Descriptive research classifies, describes, compares, and measures data. Meanwhile, analytical research focuses on cause and effect. For example, take numbers on the changing trade deficits between the United States and the rest of the world in 2015-2018. This is descriptive research.
Additionally, the analytical approach requires a strong understanding of statistical concepts and techniques, making it more suitable for researchers with a background in quantitative analysis. Descriptive Approach. The descriptive approach, on the other hand, focuses on summarizing and presenting data in a meaningful and informative way.
Descriptive employs observation and surveys; analytical uses statistical, mathematical, or computational techniques. Descriptive aims to identify patterns or trends, while analytical aims to establish causation. Descriptive research is often qualitative, whereas analytical can be both qualitative and quantitative.
Across the thousands of students we work with, descriptive writing (as opposed to critical or analytical writing) is an incredibly pervasive problem.In fact, it's probably the biggest killer of marks in dissertations, theses and research papers.So, in this post, we'll explain the difference between descriptive and analytical writing in straightforward terms, along with plenty of practical ...
Broadly speaking, there are 2 types of study designs: descriptive studies and analytical studies. Descriptive studies. Describes specific characteristics in a population of interest; The most common forms are case reports and case series; In a case report, we discuss our experience with the patient's symptoms, signs, diagnosis, and treatment ...
In conclusion, descriptive and analytical research are two sides of the same coin, offering different lenses through which we can view and interpret the intricacies of social phenomena. By understanding their distinctions and applications, researchers can better design studies that yield rich, actionable insights into the fabric of society.
Descriptive research is a methodological approach that seeks to depict the characteristics of a phenomenon or subject under investigation. In scientific inquiry, it serves as a foundational tool for researchers aiming to observe, record, and analyze the intricate details of a particular topic. This method provides a rich and detailed account ...
Descriptive research methods. Descriptive research is usually defined as a type of quantitative research, though qualitative research can also be used for descriptive purposes. The research design should be carefully developed to ensure that the results are valid and reliable.. Surveys. Survey research allows you to gather large volumes of data that can be analyzed for frequencies, averages ...
The main difference between analytical and descriptive research lies in their purpose and approach. Here are the key differences between the two: Purpose: Descriptive research aims to describe a situation, problem, or phenomenon accurately, providing a snapshot of specific phenomena at a particular point in time.
Another example is that someone might conduct analytical research to identify a study's gap. It presents a fresh perspective on your data. Therefore, it aids in supporting or refuting notions. Descriptive vs analytical research. Here are the key differences between descriptive research and analytical research:
Descriptive vs. analytical studies. Study designs can be broadly divided into either descriptive or analytical. Descriptive studies describe characteristics such as patterns or trends. They answer the questions of what, who, where, and when, and they generate hypotheses. They include case reports and qualitative studies.
Box 1. Descriptive Analysis Is a Critical Component of Research Box 2. Examples of Using Descriptive Analyses to Diagnose Need and Target Intervention on the Topic of "Summer Melt" Box 3. An Example of Using Descriptive Analysis to Evaluate Plausible Causes and Generate Hypotheses Box 4.
Ranganathan P. Understanding Research Study Designs. Indian J Crit Care Med 2019;23 (Suppl 4):S305-S307. Keywords: Clinical trials as topic, Observational studies as topic, Research designs. We use a variety of research study designs in biomedical research. In this article, the main features of each of these designs are summarized. Go to:
Both descriptive and analytical epidemiology are important for advancing clinical medicine and public health. Descriptive epidemiology assesses the burden and magnitude of health problems in a population, whereas analytical epidemiology identifies the causes and risk factors of health problems. This chapter provides the scopes, designs, data ...
Descriptive and Analytic Studies. Cross-Sectional Study as a Descriptive Study. Purpose: To learn about the characteristics of a population at one point in time (like a photo "snap shot") Design: No comparison group. Population:All members of a small, defined group or a sample from a large group. Results: Produces estimates of the ...
Descriptive Analytics. Definition: Descriptive analytics focused on describing or summarizing raw data and making it interpretable. This type of analytics provides insight into what has happened in the past. It involves the analysis of historical data to identify patterns, trends, and insights. Descriptive analytics often uses visualization ...
INTRODUCTION. In our previous article in this series, [1] we introduced the concept of "study designs"- as "the set of methods and procedures used to collect and analyze data on variables specified in a particular research question.". Study designs are primarily of two types - observational and interventional, with the former being ...
5 min read. The main difference between descriptive and analytical epidemiology is that descriptive epidemiology generates hypotheses on risk factors and causes of disease, whereas analytical epidemiology tests hypotheses by assessing the determinants of diseases, focusing on risk factors and causes as well as, analyzing the distribution of ...
Descriptive studies include case reports, case series reports, cross-sectional studies, surveillance studies, and ecological studies whereas analytical studies are either experimental or observational. Case-control and cohort studies are the type of observational studies out of which the latter is usually the prospective study.
Qualitative research collects data qualitatively, and the method of analysis is also primarily qualitative. This often involves an inductive exploration of the data to identify recurring themes, patterns, or concepts and then describing and interpreting those categories. Of course, in qualitative research, the data collected qualitatively can ...
Examples. Descriptive writing and analytical writing are often used in combination. In job application cover letters and essays for university admission, adding analytical text can provide context for otherwise unremarkable statements. Descriptive text: "I graduated from Bear University in 2020 with a B.S. in Chemistry and a cumulative GPA of 3 ...
There is a difference between Descriptive Research and Analytical Research as discussed here in this lecture ->
Descriptive research is a study of status and is widely used in education, nutrition, epidemiology, and the behavioral sciences. Its value is based on the premise that problems can be solved and practices improved through observation, analysis, and description. The most common descriptive research method is the survey, which includes ...
Background Undergraduate enrolments and completions in nursing are declining at the same time as requirements for registered nurses in the workforce is increasing. One way to increase enrolments is to lower entry requirements into nursing programs. This study determined the effect of a small lowering of entry scores on the retention and success rates. The study was undertaken at Griffith ...