- Original Research
- Open access
- Published: 30 January 2018
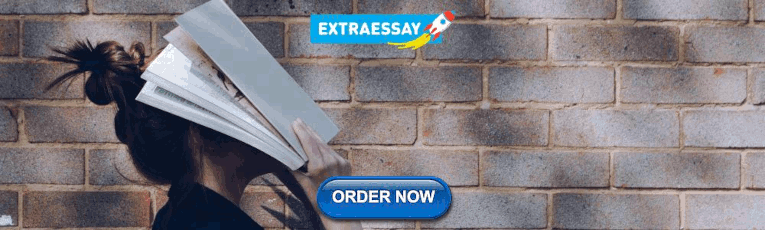
Teaching and learning mathematics through error analysis
- Sheryl J. Rushton 1
Fields Mathematics Education Journal volume 3 , Article number: 4 ( 2018 ) Cite this article
43k Accesses
25 Citations
12 Altmetric
Metrics details
For decades, mathematics education pedagogy has relied most heavily on teachers, demonstrating correctly worked example exercises as models for students to follow while practicing their own exercises. In more recent years, incorrect exercises have been introduced for the purpose of student-conducted error analysis. Combining the use of correctly worked exercises with error analysis has led researchers to posit increased mathematical understanding. Combining the use of correctly worked exercises with error analysis has led researchers to posit increased mathematical understanding.
A mixed method design was used to investigate the use of error analysis in a seventh-grade mathematics unit on equations and inequalities. Quantitative data were used to establish statistical significance of the effectiveness of using error analysis and qualitative methods were used to understand participants’ experience with error analysis.
The results determined that there was no significant difference in posttest scores. However, there was a significant difference in delayed posttest scores.
In general, the teacher and students found the use of error analysis to be beneficial in the learning process.
For decades, mathematics education pedagogy has relied most heavily on teachers demonstrating correctly worked example exercises as models for students to follow while practicing their own exercises [ 3 ]. In more recent years, incorrect exercises have been introduced for the purpose of student-conducted error analysis [ 17 ]. Conducting error analysis aligns with the Standards of Mathematical Practice [ 18 , 19 ] and the Mathematics Teaching Practices [ 18 ]. Researchers posit a result of increased mathematical understanding when these practices are used with a combination of correctly and erroneously worked exercises [ 1 , 4 , 8 , 11 , 15 , 16 , 18 , 19 , 23 ].
Review of literature
Correctly worked examples consist of a problem statement with the steps taken to reach a solution along with the final result and are an effective method for the initial acquisitions of procedural skills and knowledge [ 1 , 11 , 26 ]. Cognitive load theory [ 1 , 11 , 25 ] explains the challenge of stimulating the cognitive process without overloading the student with too much essential and extraneous information that will limit the working memory and leave a restricted capacity for learning. Correctly worked examples focus the student’s attention on the correct solution procedure which helps to avoid the need to search their prior knowledge for solution methods. Correctly worked examples free the students from performance demands and allow them to concentrate on gaining new knowledge [ 1 , 11 , 16 ].
Error analysis is an instructional strategy that holds promise of helping students to retain their learning [ 16 ]. Error analysis consists of being presented a problem statement with the steps taken to reach a solution in which one or more of the steps are incorrect, often called erroneous examples [ 17 ]. Students analyze and explain the errors and then complete the exercise correctly providing reasoning for their own solution. Error analysis leads students to enact two Standards of Mathematical Practice, namely, (a) make sense of problems and persevere in solving them and (b) attend to precision [ 19 ].
Another of the Standards of Mathematical Practice suggests that students learn to construct viable arguments and comment on the reasoning of others [ 19 ]. According to Große and Renkl [ 11 ], students who attempted to establish a rationale for the steps of the solution learned more than those who did not search for an explanation. Teachers can assist in this practice by facilitating meaningful mathematical discourse [ 18 ]. “Arguments do not have to be lengthy, they simply need to be clear, specific, and contain data or reasoning to back up the thinking” [ 20 ]. Those data and reasons could be in the form of charts, diagrams, tables, drawings, examples, or word explanations.
Researchers [ 7 , 21 ] found the process of explaining and justifying solutions for both correct and erroneous examples to be more beneficial for achieving learning outcomes than explaining and justifying solutions to correctly worked examples only. They also found that explaining why an exercise is correct or incorrect fostered transfer and led to better learning outcomes than explaining correct solutions only. According to Silver et al. [ 22 ], students are able to form understanding by classifying procedures into categories of correct examples and erroneous examples. The students then test their initial categories against further correct and erroneous examples to finally generate a set of attributes that defines the concept. Exposing students to both correctly worked examples and error analysis is especially beneficial when a mathematical concept is often done incorrectly or is easily confused [ 11 ].
Große and Renkl [ 11 ] suggested in their study involving university students in Germany that since errors are inherent in human life, introducing errors in the learning process encourages students to reflect on what they know and then be able to create clearer and more complete explanations of the solutions. The presentation of “incorrect knowledge can induce cognitive conflicts which prompt the learner to build up a coherent knowledge structure” [ 11 ]. Presenting a cognitive conflict through erroneously worked exercises triggers learning episodes through reflection and explanations, which leads to deeper understanding [ 29 ]. Error analysis “can foster a deeper and more complete understanding of mathematical content, as well as of the nature of mathematics itself” [ 4 ].
Several studies have been conducted on the use of error analysis in mathematical units [ 1 , 16 , 17 ]. The study conducted for this article differed from these previous studies in mathematical content, number of teachers and students involved in the study, and their use of a computer or online component. The most impactful differences between the error analysis studies conducted in the past and this article’s study are the length of time between the posttest and the delayed posttest and the use of qualitative data to add depth to the findings. The previous studies found students who conducted error analysis work did not perform significantly different on the posttest than students who received a more traditional approach to learning mathematics. However, the students who conducted error analysis outperformed the control group in each of the studies on delayed posttests that were given 1–2 weeks after the initial posttest.
Loibl and Rummel [ 15 ] discovered that high school students became aware of their knowledge gaps in a general manner by attempting an exercise and failing. Instruction comparing the erroneous work with correctly worked exercises filled the learning gaps. Gadgil et al. [ 9 ] conducted a study in which students who compared flawed work to expertly done work were more likely to repair their own errors than students who only explained the expertly done work. This discovery was further supported by other researchers [8, 14, 24]. Each of these researchers found students ranging from elementary mathematics to university undergraduate medical school who, when given correctly worked examples and erroneous examples, learned more than students who only examined correctly worked examples. This was especially true when the erroneous examples were similar to the kinds of errors that they had committed [ 14 ]. Stark et al. [ 24 ] added that it is important for students to receive sufficient scaffolding in correctly worked examples before and alongside of the erroneous examples.
The purpose of this study was to explore whether seventh-grade mathematics students could learn better from the use of both correctly worked examples and error analysis than from the more traditional instructional approach of solving their exercises in which the students are instructed with only correctly worked examples. The study furthered previous research on the subject of learning from the use of both correctly worked examples and error analysis by also investigating the feedback from the teacher’s and students’ experiences with error analysis. The following questions were answered in this study:
What was the difference in mathematical achievement when error analysis was included in students’ lessons and assignments versus a traditional approach of learning through correct examples only?
What kind of benefits or disadvantages did the students and teacher observe when error analysis was included in students’ lessons and assignments versus a traditional approach of learning through correct examples only?
A mixed method design was used to investigate the use of error analysis in a seventh-grade mathematics unit on equations and inequalities. Quantitative data were used to establish statistical significance of the effectiveness of using error analysis and qualitative methods were used to understand participants’ experience with error analysis [ 6 , 27 ].
Participants
Two-seventh-grade mathematics classes at an International Baccalaureate (IB) school in a suburban charter school in Northern Utah made up the control and treatment groups using a convenience grouping. One class of 26 students was the control group and one class of 27 students was the treatment group.
The same teacher taught both the groups, so a comparison could be made from the teacher’s point of view of how the students learned and participated in the two different groups. At the beginning of the study, the teacher was willing to give error analysis a try in her classroom; however, she was not enthusiastic about using this strategy. She could not visualize how error analysis could work on a daily basis. By the end of the study, the teacher became very enthusiastic about using error analysis in her seventh grade mathematics classes.
The total group of participants involved 29 males and 24 females. About 92% of the participants were Caucasian and the other 8% were of varying ethnicities. Seventeen percent of the student body was on free or reduced lunch. Approximately 10% of the students had individual education plans (IEP).
A pretest and posttest were created to contain questions that would test for mathematical understanding on equations and inequalities using Glencoe Math: Your Common Core Edition CCSS [ 5 ] as a resource. The pretest was reused as the delayed posttest. Homework assignments were created for both the control group and the treatment group from the Glencoe Math: Your Common Core Edition CCSS textbook. However, the researcher rewrote two to three of the homework exercises as erroneous examples for the treatment group to find the error and fix the exercise with justifications (see Figs. 1 , 2 ). Students from both groups used an Assignment Time Log to track the amount of time which they spent on their homework assignments.
Example of the rewritten homework exercises as equation erroneous examples
Example of the rewritten homework exercises as inequality erroneous examples
Both the control and the treatment groups were given the same pretest for an equations and inequality unit. The teacher taught both the control and treatment groups the information for the new concepts in the same manner. The majority of the instruction was done using the direct instruction strategy. The students in both groups were allowed to work collaboratively in pairs or small groups to complete the assignments after instruction had been given. During the time she allotted for answering questions from the previous assignment, she would only show the control group the exercises worked correctly. However, for the treatment group, the teacher would write errors which she found in the students’ work on the board. She would then either pair up the students or create small groups and have the student discuss what errors they noticed and how they would fix them. Often, the teacher brought the class together as a whole to discuss what they discovered and how they could learn from it.
The treatment group was given a homework assignment with the same exercises as the control group, but including the erroneous examples. Students in both the control and treatment groups were given the Assignment Time Log to keep a record of how much time was spent completing each homework assignment.
At the end of each week, both groups took the same quiz. The quizzes for the control group received a grade, and the quiz was returned without any further attention. If a student asked how to do an exercise, the teacher only showed the correct example. The teacher graded the quizzes for the treatment group using the strategy found in the Teaching Channel’s video “Highlighting Mistakes: A Grading Strategy” [ 2 ]. She marked the quizzes by highlighting the mistakes; no score was given. The students were allowed time in class or at home to make corrections with justifications.
The same posttest was administered to both groups at the conclusion of the equation and inequality chapter, and a delayed posttest was administered 6 weeks later. The delayed posttest also asked the students in the treatment group to respond to an open-ended request to “Please provide some feedback on your experience”. The test scores were analyzed for significant differences using independent samples t tests. The responses to the open-ended request were coded and analyzed for similarities and differences, and then, used to determine the students’ perceptions of the benefits or disadvantages of using error analysis in their learning.
At the conclusion of gathering data from the assessments, the researcher interviewed the teacher to determine the differences which the teacher observed in the preparation of the lessons and students’ participation in the lessons [ 6 ]. The interview with the teacher contained a variety of open-ended questions. These are the questions asked during the interview: (a) what is your opinion of using error analysis in your classroom at the conclusion of the study versus before the study began? (b) describe a typical classroom discussion in both the control group class and the treatment group class, (c) talk about the amount of time you spent grading, preparing, and teaching both groups, and (d) describe the benefits or disadvantages of using error analysis on a daily basis compared to not using error analysis in the classroom. The responses from the teacher were entered into a computer, coded, and analyzed for thematic content [ 6 , 27 ]. The themes that emerged from coding the teacher’s responses were used to determine the kind of benefits or disadvantages observed when error analysis was included in students’ lessons and assignments versus a traditional approach of learning through correct examples only from the teacher’s point of view.
Findings and discussion
Mathematical achievement.
Preliminary analyses were carried out to evaluate assumptions for the t test. Those assumptions include: (a) the independence, (b) normality tested using the Shapiro–Wilk test, and (c) homogeneity of variance tested using the Levene Statistic. All assumptions were met.
The Levene Statistic for the pretest scores ( p > 0.05) indicated that there was not a significant difference in the groups. Independent samples t tests were conducted to determine the effect error analysis had on student achievement determined by the difference in the means of the pretest and posttest and of the pretest and delayed posttest. There was no significant difference in the scores from the posttest for the control group ( M = 8.23, SD = 5.67) and the treatment group ( M = 9.56, SD = 5.24); t (51) = 0.88, p = 0.381. However, there was a significant difference in the scores from the delayed posttest for the control group ( M = 5.96, SD = 4.90) and the treatment group ( M = 9.41, SD = 4.77); t (51) = 2.60, p = 0.012. These results suggest that students can initially learn mathematical concepts through a variety of methods. Nevertheless, the retention of the mathematical knowledge is significantly increased when error analysis is added to the students’ lessons, assignments, and quizzes. It is interesting to note that the difference between the means from the pretest to the posttest was higher in the treatment group ( M = 9.56) versus the control group ( M = 8.23), implying that even though there was not a significant difference in the means, the treatment group did show a greater improvement.
The Assignment Time Log was completed by only 19% of the students in the treatment group and 38% of the students in the control group. By having such a small percentage of each group participate in tracking the time spent completing homework assignment, the results from the t test analysis cannot be used in any generalization. However, the results from the analysis were interesting. The mean time spent doing the assignments for each group was calculated and analyzed using an independent samples t test. There was no significant difference in the amount of time students which spent on their homework for the control group ( M = 168.30, SD = 77.41) and the treatment group ( M = 165.80, SD = 26.53); t (13) = 0.07, p = 0.946. These results suggest that the amount of time that students spent on their homework was close to the same whether they had to do error analyses (find the errors, fix them, and justify the steps taken) or solve each exercise in a traditional manner of following correctly worked examples. Although the students did not spend a significantly different amount of time outside of class doing homework, the treatment group did spend more time during class working on quiz corrections and discussing error which could attribute to the retention of knowledge.
Feedback from participants
All students participating in the current study submitted a signed informed consent form. Students process mathematical procedures better when they are aware of their own errors and knowledge gaps [ 15 ]. The theoretical model of using errors that students make themselves and errors that are likely due to the typical knowledge gaps can also be found in works by other researchers such as Kawasaki [ 14 ] and VanLehn [ 29 ]. Highlighting errors in the students’ own work and in typical errors made by others allowed the participants in the treatment group the opportunity to experience this theoretical model. From their experiences, the participants were able to give feedback to help the researcher delve deeper into what the thoughts were of the use of error analysis in their mathematics classes than any other study provided [ 1 , 4 , 7 , 8 , 9 , 11 , 14 , 15 , 16 , 17 , 21 , 23 , 24 , 25 , 26 , 29 ]. Overall, the teacher and students found the use of error analysis in the equations and inequalities unit to be beneficial. The teacher pointed out that the discussions in class were deeper in the treatment group’s class. When she tried to facilitate meaningful mathematical discourse [ 18 ] in the control group class, the students were unable to get to the same level of critical thinking as the treatment group discussions. In the open-ended question at the conclusion of the delayed posttest (“Please provide some feedback on your experience.”), the majority (86%) of the participants from the treatment group indicated that the use of erroneous examples integrated into their lessons was beneficial in helping them recognize their own mistakes and understanding how to correct those mistakes. One student reported, “I realized I was doing the same mistakes and now knew how to fix it”. Several (67%) of the students indicated learning through error analysis made the learning process easier for them. A student commented that “When I figure out the mistake then I understand the concept better, and how to do it, and how not to do it”.
When students find and correct the errors in exercises, while justifying themselves, they are being encouraged to learn to construct viable arguments and critique the reasoning of others [ 19 ]. This study found that explaining why an exercise is correct or incorrect fostered transfer and led to better learning outcomes than explaining correct solutions only. However, some of the higher level students struggled with the explanation component. According to the teacher, many of these higher level students who typically do very well on the homework and quizzes scored lower on the unit quizzes and tests than the students expected due to the requirement of explaining the work. In the past, these students had not been justifying their thinking and always got correct answers. Therefore, providing reasons for erroneous examples and justifying their own process were difficult for them.
Often teachers are resistant to the idea of using error analysis in their classroom. Some feel creating erroneous examples and highlighting errors for students to analyze is too time-consuming [ 28 ]. The teacher in this study taught both the control and treatment groups, which allowed her the perspective to compare both methods. She stated, “Grading took about the same amount of time whether I gave a score or just highlighted the mistakes”. She noticed that having the students work on their errors from the quizzes and having them find the errors in the assignments and on the board during class time ultimately meant less work for her and more work for the students.
Another reason behind the reluctance to use error analysis is the fact that teachers are uncertain about exposing errors to their students. They are fearful that the discussion of errors could lead their students to make those same errors and obtain incorrect solutions [ 28 ]. Yet, most of the students’ feedback stated the discussions in class and the error analyses on the assignments and quizzes helped them in working homework exercises correctly. Specifically, they said figuring out what went wrong in the exercise helped them solve that and other exercises. One student said that error analysis helped them “do better in math on the test, and I actually enjoyed it”. Nevertheless, 2 of the 27 participating students in the treatment group had negative comments about learning through error analysis. One student did not feel that correcting mistakes showed them anything, and it did not reinforce the lesson. The other student stated being exposed to error analysis did, indeed, confuse them. The student kept thinking the erroneous example was a correct answer and was unsure about what they were supposed to do to solve the exercise.
When the researcher asked the teacher if there were any benefits or disadvantages to using error analysis in teaching the equations and inequalities unit, she said that she thoroughly enjoyed teaching using the error analysis method and was planning to implement it in all of her classes in the future. In fact, she found that her “hands were tied” while grading the control group quizzes and facilitating the lessons. She said, “I wanted to have the students find their errors and fix them, so we could have a discussion about what they were doing wrong”. The students also found error analysis to have more benefits than disadvantages. Other than one student whose response was eliminated for not being on topic and the two students with negative comments, the other 24 of the students in the treatment group had positive comments about their experience with error analysis. When students had the opportunity to analyze errors in worked exercises (error analysis) through the assignments and quizzes, they were able to get a deeper understanding of the content and, therefore, retained the information longer than those who only learned through correct examples.
Discussions generated in the treatment group’s classroom afforded the students the opportunity to critically reason through the work of others and to develop possible arguments on what had been done in the erroneous exercise and what approaches might be taken to successfully find a solution to the exercise. It may seem surprising that an error as simple as adding a number when it should have been subtracted could prompt a variety of questions and lead to the students suggesting possible ways to solve and check to see if the solution makes sense. In an erroneous exercise presented to the treatment group, the students were provided with the information that two of the three angles of a triangle were 35° and 45°. The task was to write and solve an equation to find the missing measure. The erroneous exercise solver had created the equation: x + 35 + 45 = 180. Next was written x + 80 = 180. The solution was x = 260°. In the discussion, the class had on this exercise, the conclusion was made that the error occurred when 80 was added to 180 to get a sum of 260. However, the discussion progressed finding different equations and steps that could have been taken to discover the missing angle measure to be 100° and why 260° was an unreasonable solution. Another approach discussed by the students was to recognize that to say the missing angle measure was 260° contradicted with the fact that one angle could not be larger than the sum of the angle measures of a triangle. Analyzing the erroneous exercises gave the students the opportunity of engaging in the activity of “explaining” and “fixing” the errors of the presented exercise as well as their own errors, an activity that fostered the students’ learning.
The students participating in both the control and treatment groups from the two-seventh-grade mathematics classes at the IB school in a suburban charter school in Northern Utah initially learned the concepts taught in the equations and inequality unit statistically just as well with both methods of teaching. The control group had the information taught to them with the use of only correctly worked examples. If they had a question about an exercise which they did wrong, the teacher would show them how to do the exercise correctly and have a discussion on the steps required to obtain the correct solutions. On their assignments and quizzes, the control group was expected to complete the work by correctly solving the equations and inequalities in the exercise, get a score on their work, and move on to the next concept. On the other hand, the students participating in the treatment group were given erroneous examples within their assignments and asked to find the errors, explain what had been done wrong, and then correctly solve the exercise with justifications for the steps they chose to use. During lessons, the teacher put erroneous examples from the students’ work on the board and generated paired, small groups, or whole group discussion of what was wrong with the exercise and the different ways to do it correctly. On the quizzes, the teacher highlighted the errors and allowed the students to explain the errors and justify the correct solution.
Both the method of teaching using error analysis and the traditional method of presenting the exercise and having the students solve it proved to be just as successful on the immediate unit summative posttest. However, the delayed posttest given 6 weeks after the posttest showed that the retention of knowledge was significantly higher for the treatment group. It is important to note that the fact that the students in the treatment group were given more time to discuss the exercises in small groups and as a whole class could have influenced the retention of mathematical knowledge just as much or more than the treatment of using error analysis. Researchers have proven academic advantages of group work for students, in large part due to the perception of students having a secure support system, which cannot be obtained when working individually [ 10 , 12 , 13 ].
The findings of this study supported the statistical findings of other researchers [ 1 , 16 , 17 ], suggesting that error analysis may aid in providing a richer learning experience that leads to a deeper understanding of equations and inequalities for long-term knowledge. The findings of this study also investigated the teacher’s and students’ perceptions of using error analysis in their teaching and learning. The students and teacher used for this study were chosen to have the same teacher for both the control and treatment groups. Using the same teacher for both groups, the researcher was able to determine the teacher’s attitude toward the use of error analysis compared to the non-use of error analysis in her instruction. The teacher’s comments during the interview implied that she no longer had an unenthusiastic and skeptical attitude toward the use of error analysis on a daily basis in her classroom. She was “excited to implement the error analysis strategy into the rest of her classes for the rest of the school year”. She observed error analysis to be an effective way to deal with common misconceptions and offer opportunities for students to reflect on their learning from their errors. The process of error analysis assisted the teacher in supporting productive struggle in learning mathematics [ 18 ] and created opportunity for students to have deep discussions about alternative ways to solve exercises. Error analysis also aided in students’ discovery of their own errors and gave them possible ways to correct those errors. Learning through the use of error analysis was enjoyable for many of the participating students.
According to the NCTM [ 18 ], effective teaching of mathematics happens when a teacher implements exercises that will engage students in solving and discussing tasks that promote mathematical reasoning and problem solving. Providing erroneous examples allowed discussion, multiple entry points, and varied solution strategies. Both the teacher and the students participating in the treatment group came to the conclusion that error analysis is a beneficial strategy to use in the teaching and learning of mathematics. Regardless of the two negative student comments about error analysis not being helpful for them, this researcher recommends the use of error analysis in teaching and learning mathematics.
The implications of the treatment of teaching students mathematics through the use of error analysis are that students’ learning could be fostered and retention of content knowledge may be longer. When a teacher is able to have their students’ practice critiquing the reasoning of others and creating viable arguments [ 19 ] by analyzing errors in mathematics, the students not only are able to meet the Standard of Mathematical Practice, but are also creating a lifelong skill of analyzing the effectiveness of “plausible arguments, distinguish correct logic or reasoning from that which is flawed, and—if there is a flaw in an argument—explain what it is” ([ 19 ], p. 7).
Limitations and future research
This study had limitations. The sample size was small to use the same teacher for both groups. Another limitation was the length of the study only encompassed one unit. Using error analysis could have been a novelty and engaged the students more than it would when the novelty wore off. Still another limitation was the study that was conducted at an International Baccalaureate (IB) school in a suburban charter school in Northern Utah, which may limit the generalization of the findings and implications to other schools with different demographics.
This study did not have a separation of conceptual and procedural questions on the assessments. For a future study, the creation of an assessment that would be able to determine if error analysis was more helpful in teaching conceptual mathematics or procedural mathematics could be beneficial to teachers as they plan their lessons. Another suggestion for future research would be to gather more data using several teachers teaching both the treatment group and the control group.
Adams, D.M., McLaren, B.M., Durkin, K., Mayer, R.E., Rittle-Johnson, B., Isotani, S., van Velsen, M.: Using erroneous examples to improve mathematics learning with a web-based tutoring system. Comput. Hum. Behav. 36 , 401–411 (2014)
Article Google Scholar
Alcala, L.: Highlighting mistakes: a grading strategy. The teaching channel. https://www.teachingchannel.org/videos/math-test-grading-tips
Atkinson, R.K., Derry, S.J., Renkl, A., Wortham, D.: Learning from examples: instructional Principles from the worked examples research. Rev. Educ. Res. 70 (2), 181–214 (2000)
Borasi, R.: Exploring mathematics through the analysis of errors. Learn. Math. 7 (3), 2–8 (1987)
Google Scholar
Carter, J.A., Cuevas, G.J., Day, R., Malloy, C., Kersaint, G., Luchin, B.M., Willard, T.: Glencoe math: your common core edition CCSS. Glencoe/McGraw-Hill, Columbus (2013)
Creswell, J.: Research design: qualitative, quantitative, and mixed methods approaches, 4th edn. Sage Publications, Thousand Oaks (2014)
Curry, L. A.: The effects of self-explanations of correct and incorrect solutions on algebra problem-solving performance. In: Proceedings of the 26th annual conference of the cognitive science society, vol. 1548. Erlbaum, Mahwah (2004)
Durkin, K., Rittle-Johnson, B.: The effectiveness of using incorrect examples to support learning about decimal magnitude. Learn. Instr. 22 (3), 206–214 (2012)
Gadgil, S., Nokes-Malach, T.J., Chi, M.T.: Effectiveness of holistic mental model confrontation in driving conceptual change. Learn. Instr. 22 (1), 47–61 (2012)
Gaudet, A.D., Ramer, L.M., Nakonechny, J., Cragg, J.J., Ramer, M.S.: Small-group learning in an upper-level university biology class enhances academic performance and student attitutdes toward group work. Public Libr. Sci. One 5 , 1–9 (2010)
Große, C.S., Renkl, A.: Finding and fixing errors in worked examples: can this foster learning outcomes? Learn. Instr. 17 (6), 612–634 (2007)
Janssen, J., Kirschner, F., Erkens, G., Kirschner, P.A., Paas, F.: Making the black box of collaborative learning transparent: combining process-oriented and cognitive load approaches. Educ. Psychol. Rev. 22 , 139–154 (2010)
Johnson, D.W., Johnson, R.T.: An educational psychology success story: social interdependence theory and cooperative learning. Educ. Res. 38 , 365–379 (2009)
Kawasaki, M.: Learning to solve mathematics problems: the impact of incorrect solutions in fifth grade peers’ presentations. Jpn. J. Dev. Psychol. 21 (1), 12–22 (2010)
Loibl, K., Rummel, N.: Knowing what you don’t know makes failure productive. Learn. Instr. 34 , 74–85 (2014)
McLaren, B.M., Adams, D., Durkin, K., Goguadze, G., Mayer, R.E., Rittle-Johnson, B., Van Velsen, M.: To err is human, to explain and correct is divine: a study of interactive erroneous examples with middle school math students. 21st Century learning for 21st Century skills, pp. 222–235. Springer, Berlin (2012)
Chapter Google Scholar
McLaren, B.M., Adams, D.M., Mayer, R.E.: Delayed learning effects with erroneous examples: a study of learning decimals with a web-based tutor. Int. J. Artif. Intell. Educ. 25 (4), 520–542 (2015)
National Council of Teachers of Mathematics (NCTM): Principles to actions: ensuring mathematical success for all. Author, Reston (2014)
National Governors Association Center for Best Practices & Council of Chief State School Officers (NGA Center and CCSSO): Common core state standards. Authors, Washington, DC (2010)
O’Connell, S., SanGiovanni, J.: Putting the practices into action: Implementing the common core standards for mathematical practice K-8. Heinemann, Portsmouth (2013)
Siegler, R.S.: Microgenetic studies of self-explanation. Microdevelopment: transition processes in development and learning, pp. 31–58. Cambridge University Press, New York (2002)
Silver, H.F., Strong, R.W., Perini, M.J.: The strategic teacher: Selecting the right research-based strategy for every lesson. ASCD, Alexandria (2009)
Sisman, G.T., Aksu, M.: A study on sixth grade students’ misconceptions and errors in spatial measurement: length, area, and volume. Int. J. Sci. Math. Educ. 14 (7), 1293–1319 (2015)
Stark, R., Kopp, V., Fischer, M.R.: Case-based learning with worked examples in complex domains: two experimental studies in undergraduate medical education. Learn. Instr. 21 (1), 22–33 (2011)
Sweller, J.: Cognitive load during problem solving: effects on learning. Cognitive Sci. 12 , 257–285 (1988)
Sweller, J., Cooper, G.A.: The use of worked examples as a substitute for problem solving in learning algebra. Cognit. Instr. 2 (1), 59–89 (1985)
Tashakkori, A., Teddlie, C.: Sage handbook of mixed methods in social & behavioral research, 2nd edn. Sage Publications, Thousand Oaks (2010)
Book Google Scholar
Tsovaltzi, D., Melis, E., McLaren, B.M., Meyer, A.K., Dietrich, M., Goguadze, G.: Learning from erroneous examples: when and how do students benefit from them? Sustaining TEL: from innovation to learning and practice, pp. 357–373. Springer, Berlin (2010)
VanLehn, K.: Rule-learning events in the acquisition of a complex skill: an evaluation of CASCADE. J. Learn. Sci. 8 (1), 71–125 (1999)
Download references
Acknowledgements
Not applicable.
Competing interests
The author declares that no competing interests.
Availability of data and materials
A spreadsheet of the data will be provided as an Additional file 1 : Error analysis data.
Consent for publication
Ethics approval and consent to participate.
All students participating in the current study submitted a signed informed consent form.
Publisher’s Note
Springer Nature remains neutral with regard to jurisdictional claims in published maps and institutional affiliations.
Author information
Authors and affiliations.
Weber State University, 1351 Edvalson St. MC 1304, Ogden, UT, 84408, USA
Sheryl J. Rushton
You can also search for this author in PubMed Google Scholar
Corresponding author
Correspondence to Sheryl J. Rushton .
Additional file
Additional file 1:.
Error analysis data.
Rights and permissions
Open Access This article is distributed under the terms of the Creative Commons Attribution 4.0 International License ( http://creativecommons.org/licenses/by/4.0/ ), which permits unrestricted use, distribution, and reproduction in any medium, provided you give appropriate credit to the original author(s) and the source, provide a link to the Creative Commons license, and indicate if changes were made.
Reprints and permissions
About this article
Cite this article.
Rushton, S.J. Teaching and learning mathematics through error analysis. Fields Math Educ J 3 , 4 (2018). https://doi.org/10.1186/s40928-018-0009-y
Download citation
Received : 07 March 2017
Accepted : 16 January 2018
Published : 30 January 2018
DOI : https://doi.org/10.1186/s40928-018-0009-y
Share this article
Anyone you share the following link with will be able to read this content:
Sorry, a shareable link is not currently available for this article.
Provided by the Springer Nature SharedIt content-sharing initiative
- Error analysis
- Correct and erroneous examples
- Mathematics teaching practices
- Standards of mathematical practices
Error Analysis
- First Online: 17 September 2021
Cite this chapter
- Maurizio Petrelli 2
Part of the book series: Springer Textbooks in Earth Sciences, Geography and Environment ((STEGE))
Chapter 10 is about errors and error propagation. It defines precision, accuracy, standard error, and confidence intervals. Then it demonstrates how to report uncertainties in binary diagrams. Finally, it shows two approaches to propagate the uncertainties: the linearized and Monte Carlo methods.
This is a preview of subscription content, log in via an institution to check access.
Access this chapter
Subscribe and save.
- Get 10 units per month
- Download Article/Chapter or eBook
- 1 Unit = 1 Article or 1 Chapter
- Cancel anytime
- Available as PDF
- Read on any device
- Instant download
- Own it forever
- Available as EPUB and PDF
- Compact, lightweight edition
- Dispatched in 3 to 5 business days
- Free shipping worldwide - see info
- Durable hardcover edition
Tax calculation will be finalised at checkout
Purchases are for personal use only
Institutional subscriptions
https://www.pcg-random.org .
https://numpy.org/doc/stable/reference/random/bit_generators/ .
Author information
Authors and affiliations.
Department of Physics and Geology, University of Perugia, Perugia, Italy
Maurizio Petrelli
You can also search for this author in PubMed Google Scholar
Corresponding author
Correspondence to Maurizio Petrelli .
Rights and permissions
Reprints and permissions
Copyright information
© 2021 The Author(s), under exclusive license to Springer Nature Switzerland AG
About this chapter
Petrelli, M. (2021). Error Analysis. In: Introduction to Python in Earth Science Data Analysis. Springer Textbooks in Earth Sciences, Geography and Environment. Springer, Cham. https://doi.org/10.1007/978-3-030-78055-5_10
Download citation
DOI : https://doi.org/10.1007/978-3-030-78055-5_10
Published : 17 September 2021
Publisher Name : Springer, Cham
Print ISBN : 978-3-030-78054-8
Online ISBN : 978-3-030-78055-5
eBook Packages : Earth and Environmental Science Earth and Environmental Science (R0)
Share this chapter
Anyone you share the following link with will be able to read this content:
Sorry, a shareable link is not currently available for this article.
Provided by the Springer Nature SharedIt content-sharing initiative
- Publish with us
Policies and ethics
- Find a journal
- Track your research
Thank you for visiting nature.com. You are using a browser version with limited support for CSS. To obtain the best experience, we recommend you use a more up to date browser (or turn off compatibility mode in Internet Explorer). In the meantime, to ensure continued support, we are displaying the site without styles and JavaScript.
- View all journals
- Explore content
- About the journal
- Publish with us
- Sign up for alerts
- Open access
- Published: 08 May 2023
Deep fake detection and classification using error-level analysis and deep learning
- Rimsha Rafique 1 ,
- Rahma Gantassi 2 ,
- Rashid Amin 1 , 3 ,
- Jaroslav Frnda 4 , 5 ,
- Aida Mustapha 6 &
- Asma Hassan Alshehri 7
Scientific Reports volume 13 , Article number: 7422 ( 2023 ) Cite this article
22k Accesses
19 Citations
6 Altmetric
Metrics details
- Energy science and technology
- Mathematics and computing
Due to the wide availability of easy-to-access content on social media, along with the advanced tools and inexpensive computing infrastructure, has made it very easy for people to produce deep fakes that can cause to spread disinformation and hoaxes. This rapid advancement can cause panic and chaos as anyone can easily create propaganda using these technologies. Hence, a robust system to differentiate between real and fake content has become crucial in this age of social media. This paper proposes an automated method to classify deep fake images by employing Deep Learning and Machine Learning based methodologies. Traditional Machine Learning (ML) based systems employing handcrafted feature extraction fail to capture more complex patterns that are poorly understood or easily represented using simple features. These systems cannot generalize well to unseen data. Moreover, these systems are sensitive to noise or variations in the data, which can reduce their performance. Hence, these problems can limit their usefulness in real-world applications where the data constantly evolves. The proposed framework initially performs an Error Level Analysis of the image to determine if the image has been modified. This image is then supplied to Convolutional Neural Networks for deep feature extraction. The resultant feature vectors are then classified via Support Vector Machines and K-Nearest Neighbors by performing hyper-parameter optimization. The proposed method achieved the highest accuracy of 89.5% via Residual Network and K-Nearest Neighbor. The results prove the efficiency and robustness of the proposed technique; hence, it can be used to detect deep fake images and reduce the potential threat of slander and propaganda.
Similar content being viewed by others
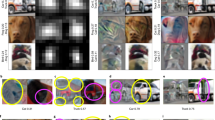
Adversarial explanations for understanding image classification decisions and improved neural network robustness
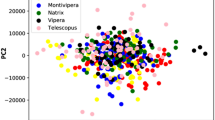
A comparative study on image-based snake identification using machine learning
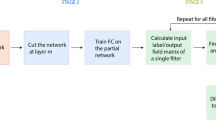
Towards a universal mechanism for successful deep learning
Introduction.
In the last decade, social media content such as photographs and movies has grown exponentially online due to inexpensive devices such as smartphones, cameras, and computers. The rise in social media applications has enabled people to quickly share this content across the platforms, drastically increasing online content, and providing easy access. At the same time, we have seen enormous progress in complex yet efficient machine learning (ML) and Deep Learning (DL) algorithms that can be deployed for manipulating audiovisual content to disseminate misinformation and damage the reputation of people online. We now live in such times where spreading disinformation can be easily used to sway peoples’ opinions and can be used in election manipulation or defamation of any individual. Deep fake creation has evolved dramatically in recent years, and it might be used to spread disinformation worldwide, posing a serious threat soon. Deep fakes are synthesized audio and video content generated via AI algorithms. Using videos as evidence in legal disputes and criminal court cases is standard practice. The authenticity and integrity of any video submitted as evidence must be established. Especially when deep fake generation becomes more complex, this is anticipated to become a difficult task.
The following categories of deep fake videos exist: face-swap, synthesis, and manipulation of facial features. In face-swap deep fakes, a person's face is swapped with that of the source person to create a fake video to target a person for the activities they have not committed 1 , which can tarnish the reputation of the person 2 . In another type of deep fake called lip-synching, the target person’s lips are manipulated to alter the movements according to a certain audio track. The purpose of lip-syncing is to simulate the victim's attacker's voice by having someone talk in that voice. With puppet-master, deep fakes are produced by imitating the target's facial expressions, eye movements, and head movements. Using fictitious profiles, this is done to propagate false information on social media. Last but not least, deep audio fakes or voice cloning is used to manipulate an individual's voice that associates something with the speaker they haven’t said in actual 1 , 3 .
The importance of discovering the truth in the digital realm has therefore increased. Dealing with deep fakes is significantly more difficult because they are mostly utilized for harmful objectives and virtually anyone can now produce deep fakes utilizing the tools already available. Many different strategies have been put out so far to find deep fakes. Since most are also based on deep learning, a conflict between bad and good deep learning applications has developed 4 . Hence, to solve this problem, the United States Defense Advanced Research Projects Agency (DARPA) launched a media forensics research plan to develop fake digital media detection methods 5 . Moreover, in collaboration with Microsoft, Facebook also announced an AI-based deep fake detection challenge to prevent deep fakes from being used to deceive viewers 6 .
Over the past few years, several researchers have explored Machine Learning and Deep Learning (DL) areas to detect deep fakes from audiovisual media. The ML-based algorithms use labor-intensive and erroneous manual feature extraction before the classification phase. As a result, the performance of these systems is unstable when dealing with bigger databases. However, DL algorithms automatically carry out these tasks, which have proven tremendously helpful in various applications, including deep fake detection. Convolutional neural network (CNN), one of the most prominent DL models, is frequently used due to its state-of-the-art performance that automatically extracts low-level and high-level features from the database. Hence, these methods have drawn the researcher’s interest in scientists across the globe 7 .
Despite substantial research on the subject of deep fakes detection, there is always potential for improvement in terms of efficiency and efficacy. It may be noted that the deep fake generation techniques are improving quickly, thus resulting in increasingly challenging datasets on which previous techniques may not perform effectively. The motivation behind developing automated DL based deep fake detection systems is to mitigate the potential harm caused by deep fake technology. Deep fake content can deceive and manipulate people, leading to serious consequences, such as political unrest, financial fraud, and reputational damage. The development such systems can have significant positive impacts on various industries and fields. These systems also improve the trust and reliability of media and online content. As deep fake technology becomes more sophisticated and accessible, it is important to have reliable tools to distinguish between real and fake content. Hence, developing a robust system to detect deep fakes from media has become very necessary in this age of social media. This paper is a continuation of to study provided by Rimsha et al. 8 . The paper compares the performance of CNN architectures such as AlexNet and VGG16 to detect if the image is real of has been digitally altered. The main contributions of this study are as follows:
In this study, we propose a novel deep fake detection and classification method employing DL and ML-based methods.
The proposed framework preprocesses the image by resizing it according to CNN’s input layer and then performing Error Level Analysis to find any digital manipulation on a pixel level.
The resultant ELA image is supplied to Convolutional Neural Networks, i.e., GoogLeNet, ResNet18 and SqueezeNet, for deep feature extraction.
Extensive experiments are conducted to find the optimal hyper-parameter setting by hyper-parameter tuning.
The performance of the proposed technique is evaluated on the publically available dataset for deep fake detection
Related work
The first ever deep fake was developed in 1860, when a portrait of southern leader John Calhoun was expertly altered for propaganda by swapping his head out for the US President. These manipulations are typically done by splicing, painting, and copy-moving the items inside or between two photos. The appropriate post-processing processes are then used to enhance the visual appeal, scale, and perspective coherence. These steps include scaling, rotating, and color modification 9 , 10 . A range of automated procedures for digital manipulation with improved semantic consistency are now available in addition to these conventional methods of manipulation due to developments in computer graphics and ML/DL techniques. Modifications in digital media have become relatively affordable due to widely available software for developing such content. The manipulation is in digital media is increasing at a very fast pace which requires development of such algorithms to robustly detect and analyze such content to find the difference between right and wrong 11 , 12 , 13 .
Despite being a relatively new technology, deep fake has been the topic of investigation. In recent years, there had been a considerable increase in deep fake articles towards the end of 2020. Due to the advent of ML and DL-based techniques, many researchers have developed automated algorithms to detect deep fakes from audiovisual content. These techniques have helped in finding out the real and fake content easily. Deep learning is well renowned for its ability to represent complicated and high-dimensional data 11 , 14 . Matern et al. 15 employed detected deep fakes from Face Forensics dataset using Multilayered perceptron (MLP) with an AUC of 0.85. However, the study considers facial images with open eyes only. Agarwal et al. 16 extracted features using Open Face 2 toolkit and performed classification via SVM. The system obtained 93% AUC; however, the system provides incorrect results when a person is not facing camera. The authors in Ciftci et al. 17 extracted medical signal features and performed classification via CNN with 97% accuracy. However, the system is computationally complex due to a very large feature vector. In their study, Yang et al. 18 extracted 68-D facial landmarks using DLib and classified these features via SVM. The system obtained 89% ROC. However, the system is not robust to blurred and requires a preprocessing stage. Rossle et al. 19 employed SVM + CNN for feature classification and a Co-Occurrence matrix for feature extraction. The system attained 90.29% accuracy on Face Forensics dataset. However, the system provides poor results on compressed videos. McCloskey et al. 20 developed a deep fake detector by using the dissimilarity of colors between real camera and synthesized and real image samples. The SVM classifier was trained on color based features from the input samples. However, the system may struggle on non-preprocessed and blurry images.
A Hybrid Multitask Learning Framework with a Fire Hawk Optimizer for Arabic Fake News Detection aims to address the issue of identifying fake news in the Arabic language. The study proposes a hybrid approach that leverages the power of multiple tasks to detect fake news more accurately and efficiently. The framework uses a combination of three tasks, namely sentence classification, stance detection, and relevance prediction, to determine the authenticity of the news article. The study also suggests the use of the Fire Hawk Optimizer algorithm, a nature-inspired optimization algorithm, to fine-tune the parameters of the framework. This helps to improve the accuracy of the model and achieve better performance. The Fire Hawk Optimizer is an efficient and robust algorithm that is inspired by the hunting behavior of hawks. It uses a global and local search strategy to search for the optimal solution 21 . The authors in 22 propose a Convolution Vision Transformer (CVT) architecture that differs from CNN in that it relies on a combination of attention mechanisms and convolution operations, making it more effective in recognizing patterns within images.The CVT architecture consists of multi-head self-attention and multi-layer perceptron (MLP) layers. The self-attention layer learns to focus on critical regions of the input image without the need for convolution operations, while the MLP layer helps to extract features from these regions. The extracted features are then forwarded to the output layer to make the final classification decision. However, the system is computationally expensive due to deep architecture. Guarnera et al. 23 identified deep fake images using Expectation Maximization for extracting features and SVM, KNN, LDA as classification methods. However, the system fails in recognizing compressed images. Nguyen et al. 24 proposed a CNN based architecture to detect deep fake content and obtained 83.7% accuracy on Face Forensics dataset. However, the system is unable to generalize well on unseen cases. Khalil et al. 25 employed Local Binary Patterns (LBP) for feature extraction and CNN and Capsule Network for deep fake detection. The models were trained on Deep Fake Detection Challenge-Preview dataset and tested on DFDC-Preview and Celeb- DF datasets. A deep fake approach developed by Afchar et al. 26 employed MesoInception-4 and achieved 81.3% True Positive Rate via Face Forensics dataset.
However, the system requires preprocessing before feature extraction and classification. Hence, results in a low overall performance on low-quality videos. Wang et al. 27 evaluated the performance of Residual Networks on deep fake classification. The authors employed ResNet and ResNeXt, on videos from Face forensics dataset. In another study by Stehouwer et al. 28 , the authors presented a CNN based approach for deep fake content detection that achieved 99% overall accuracy on Diverse Fake Face Dataset. However, the system is computationally expensive due to a very large size feature vector. Despite significant progress, existing DL algorithms are computationally expensive to train and require high-end GPUs or specialized hardware. This can make it difficult for researchers and organizations with limited resources to develop and deploy deep learning models. Moreover, some of the existing DL algorithms are prone to overfitting, which occurs when the model becomes too complex and learns to memorize the training data rather than learning generalizable patterns. This can result in poor performance on new, unseen data. The limitations in the current methodologies prove there is still a need to develop a robust and efficient deep fake detection and classification method using ML and DL based approaches.
Proposed methodology
This section discusses the proposed workflow employed for deep fakes detection. The workflow diagram of our proposed framework is illustrated in Fig. 1 . The proposed system comprises of three core steps (i) image preprocessing by resizing the image according to CNN’s input layer and then generating Error Level Analysis of the image to determine pixel level alterations (ii) deep feature extraction via CNN architectures (iii) classification via SVM and KNN by performing hyper-parameter optimization.
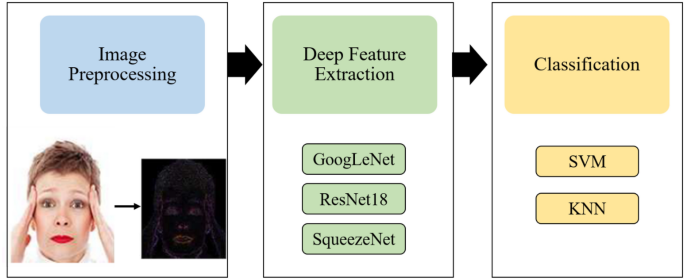
Workflow diagram of the proposed method.
(i) Error level analysis
Error level analysis, also known as ELA, is a forensic technique used to identify image segments with varying compression levels. By measuring these compression levels, the method determines if an image has undergone digital editing. This technique works best on .JPG images as in that case, the entire image pixels should have roughly the same compression levels and may vary in case of tampering 29 , 30 .
JPEG (Joint Photographic Experts Group) is a technique for the lossy compression of digital images. A data compression algorithm discards (loses) some of the data to compress it. The compression level could be used as an acceptable compromise between image size and image quality. Typically, the JPEG compression ratio is 10:1. The JPEG technique uses 8 × 8 pixel image grids independently compressed. Any matrices larger than 8 × 8 are more difficult to manipulate theoretically or are not supported by the hardware, whereas any matrices smaller than 8 × 8 lack sufficient information.
Consequently, the compressed images are of poor quality. All 8 × 8 grids for unaltered images should have a same error level, allowing for the resave of the image. Given that uniformly distributed faults are throughout the image, each square should deteriorate roughly at the same pace. The altered grid in a modified image should have a higher error potential than the rest 31 .
ELA. The image is resaved with 95% error rate, and the difference between the two images is computed. This technique determines if there is any change in cells by checking whether the pixels are at their local minima 8 , 32 . This helps determine whether there is any digital tampering in the database. The ELA is computed on our database, as shown in Fig. 2 .
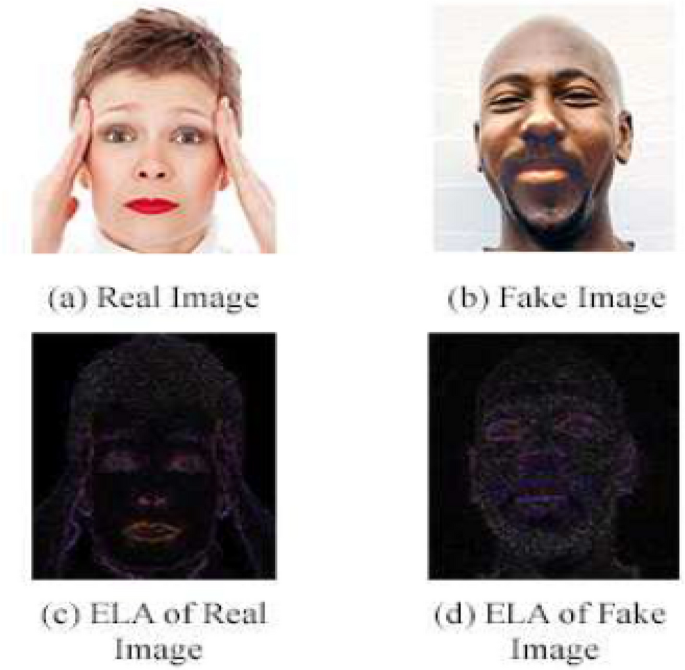
Result of ELA on dataset images.
(ii) Feature extraction using convolutional neural networks
The discovery of CNN has raised its popularity among academics and motivated them to work through difficult problems that they had previously given up on. Researchers have designed several CNN designs in recent years to deal with multiple challenges in various research fields, including deep fake detection. The general architecture of CNN as shown in Fig. 3 , is usually made up of many layers stacked on top of one another. The architecture of CNN consists of a feature extraction module composed of convolutional layers to learn the features and pooling layers reduce image dimensionality. Secondly, it consists of a module comprising a fully connected (FC) layer to classify an image 33 , 34 .
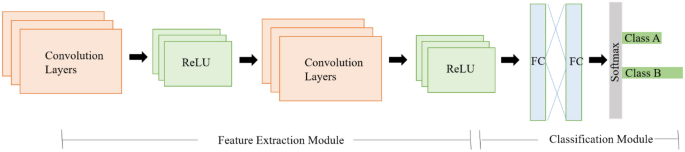
General CNN architecture.
The image is input using the input layer passed down to convolution for deep feature extraction. This layer learns the visual features from the image by preserving the relationship between its pixels. This mathematical calculation is performed on an image matrix using filter/kernel of the specified size 35 . The max-pooling layer reduces the image dimensions. This process helps increase the training speed and reduce the computational load for the next stages 36 . Some networks might include normalization layers, i.e., batch normalization or dropout layer. Batch normalization layer stabilizes the network training performance by performing standardization operations on the input to mini-batches. Whereas, the dropout layer randomly drops some nodes to reduce the network complexity, increasing the network performance 37 , 38 . The last layers of the CNN include an FC layer with a softmax probability function. FC layer stores all the features extracted from the previous phases. These features are then supplied to classifiers for image classification 38 . Since CNN architectures can extract significant features without any human involvement, hence, we used pre-trained CNNs such as GoogLeNet 39 , ResNet18 31 , and SqueezeNet 40 in this study. It may be noted that developing and training a deep learning architecture from scratch is not only a time-consuming task but requires resources for computation; hence we use pre-trained CNN architectures as deep feature extractors in our proposed framework.
Microsoft introduced Residual Network (ResNet) architecture in 2015 that consists of several Convolution Layers of kernel size 3 × 3, an FC layer followed by an additional softmax layer for classification. Because they use shortcut connections that skip one or more levels, residual networks are efficient and low in computational cost 41 . Instead of anticipating that every layer stack will instantly match a specified underlying mapping, the layers fit a residual mapping. As a result of the resulting outputs being added to those of the stacked layers, these fast connections reduce loss of value during training. This functionality also aids in training the algorithm considerably faster than conventional CNNs.
Furthermore, this mapping has no parameters because it transfers the output to the next layer. The ResNet architecture outperformed other CNNs by achieving the lowest top 5% error rate in a classification job, which is 3.57% 31 , 42 . The architecture of ResNet50 is shown in Fig. 4 43 .
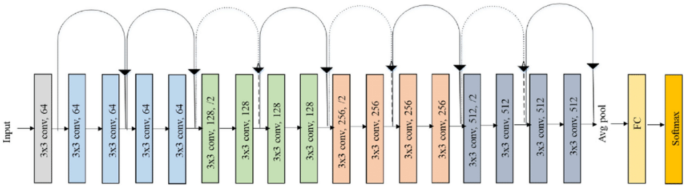
ResNet18 architecture 44 .
SqueezNet was developed by researchers at UC Berkeley and Stanford University that is a very lightweight and small architecture. The smaller CNN architectures are useful as they require less communication across servers in distributed training. Moreover, these CNNs also train faster and require less memory, hence are not computationally expensive compared to conventional deep CNNs. By modifying the architecture, the researchers claim that SqueezeNet can achieve AlexNet level accuracy via a smaller CNN 45 . Because an 1 × 1 filter contains 9× fewer parameters than a 3 × 3 filter, the 3 × 3 filters in these modifications have been replaced with 1 × 1 filters. Furthermore, the number of input channels is reduced to 3 × 3 filters via squeeze layers, which lowers the overall number of parameters.
Last but not least, the downsampling is carried out very late in the network so the convolution layers’ large activation maps which is said to increase classification accuracy 40 . Developed by Google researchers, GoogLeNet is a 22-layer deep convolutional neural network that uses a 1 × 1 convolution filter size, global average pooling and an input size of 224 × 224 × 3. The architecture of GoogLeNet is shown in Fig. 5 . To increase the depth of the network architecture, the convolution filter size is reduced to 1 × 1. Additionally, the network uses global average pooling towards the end of the architecture, which inputs a 7 × 7 feature map and averages it to an 1 × 1 feature map. This helps reduce trainable parameters and enhances the system's performance. A dropout regularization of 0.7 is also used in the architecture, and the features are stored in an FC layer 39 .
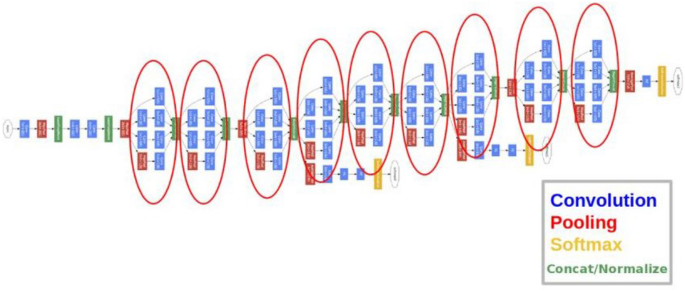
GoogLeNet architecture 46 .
CNNs extract features from images hierarchically using convolutional, pooling, and fully connected layers. The features extracted by CNNs can be broadly classified into two categories: low-level features and high-level features. Low-level features include edges, corners, and intensity variations. CNNs can detect edges by convolving the input image with a filter that highlights the edges in the image. They can also detect corners by convolving the input image with a filter that highlights the corners. Morever, CNNs can extract color features by convolving the input image with filters that highlight specific colors. On the other hand, high-level features include texture, objects, and contextual and hierarchical features. Textures from images are detected by convolving the input image with filters that highlight different textures. The CNNs detect objects by convolving the input image with filters highlighting different shapes. Whereas, contextual features are extracted by considering the relationships between different objects in the image. Finally, the CNNs can learn to extract hierarchical features by stacking multiple convolutional layers on top of each other. The lower layers extract low-level features, while the higher layers extract high-level features.
(iii) Classification via support vector machines and k-nearest neighbors
We classified the deep CNN features via SVM and KNN classifiers in this phase. KNN has gained much popularity in the research community in classification and regression tasks since it outperforms many other existing classifiers due to its simplicity and robustness. KNN calculates the distance between a test sample (k) with its neighbours and then groups the k test sample to its nearest neighbour. The KNN classifier is shown in Fig. 6
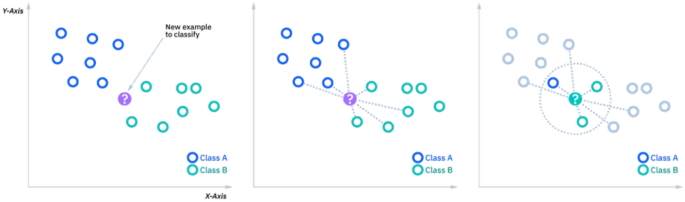
The second classifier used in this study is SVM, a widely popular classifier used frequently in many research fields because of its faster speeds and superior prediction outcomes even on a minimal dataset. The classifier finds the plane with the largest margin that separates the two classes. The wider the margin better is the classification performance of the classifier 30 , 47 . Figure 7 A depicts potential hyperplanes for a particular classification problem, whereas Fig. 7 B depicts the best hyperplane determined by SVM for that problem.
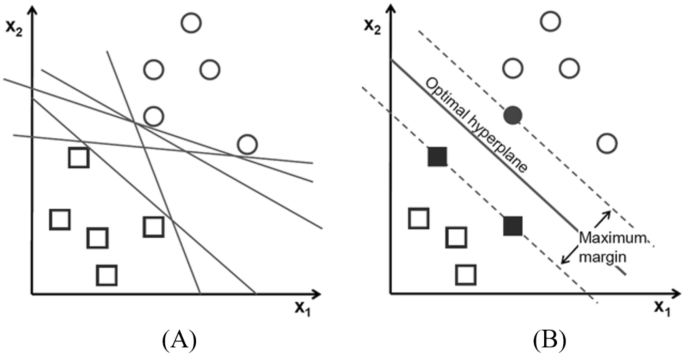
Possible SVM hyperplanes 30 .
Results and discussion
This study uses a publicly accessible dataset compiled by Yonsei University's Computational Intelligence and Photography Lab. The real and fake face database from Yonsei University's Computational Intelligence and Photography Lab is a dataset that contains images of both real and fake human faces. The dataset was designed for use in the research and development of facial recognition and verification systems, particularly those designed to detect fake or manipulated images. Each image in the dataset is labelled as either real or fake, and the dataset also includes additional information about the image, such as the age, gender, and ethnicity of the subject, as well as the manipulation technique used for fake images. Moreover, the images contain different faces, split by the eyes, nose, mouth, or entire face. The manipulated images further subdivided into three categories: easy, mid, and hard images as shown in Fig. 8 48 .
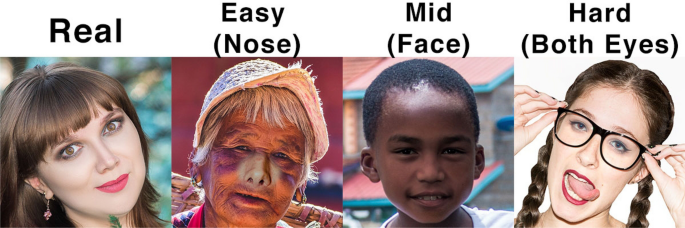
Image samples from the dataset showing real and edited images.
Evaluation metrics
Evaluation metrics are used in machine learning to measure the performance of a model. Machine learning models are designed to learn from data and make predictions or decisions based on that data. It is important to evaluate the performance of a model to understand how well it is performing and to make necessary improvements. One of the most commonly used techniques is a confusion matrix, a table to evaluate the performance of a classification model by comparing the actual and predicted classes for a set of test data. It is a matrix of four values: true positives (TP), false positives (FP), true negatives (TN), and false negatives (FN). The proposed framework is evaluated using accuracy, precision, recall, and f1-score. Even though accuracy is a widely used metric, but is suitable in the case of a balanced dataset; hence, we also evaluated our proposed methods using F1-Score that combines both recall and precision into a single metric. All the evaluation metrics that we used to assess our models are calculated from Eq. ( 1 ) to Eq. ( 4 ).
Proposed method results
The escalating problems with deep fakes have made researchers more interested in media forensics in recent years. Deep fake technology has various applications in the media sector, including lip sync, face swapping, and de-aging humans. Although advances in DL and deep fake technology have various beneficial applications in business, entertainment, and the film industry, they can serve harmful goals and contribute to people's inability to believe what's true 49 , 50 . Hence, finding the difference between real and fake has become vital in this age of social media. Finding deep fake content via the human eye has become more difficult due to progress in deep fake creation technologies. Hence, a robust system must be developed to classify these fake media without human intervention accurately.
In this study, we propose a novel and robust architecture to detect and classify deep fake images using ML and DL-based techniques. The proposed framework employs a preprocessing approach to find ELA. ELA helps find if any portion of the image has been altered by analyzing the image on a pixel level. These images are then supplied to deep CNN architectures (SqueezeNet, ResNet18 & GoogLeNet) to extract deep features. The deep features are then classified via SVM and KNN. The results obtained from ResNet’s confusion matrix and ML classifiers is shown in Fig. 9 . The feature vector achieved highest accuracy of 89.5% via KNN. We tested our various hyper-parameters for both classifiers before reaching the conclusion. The proposed method achieved 89.5% accuracy via KNN on Correlation as a distance metric and total 881 neighbors. SVM achieved 88.6% accuracy on Gaussian Kernel with a 2.3 scale.
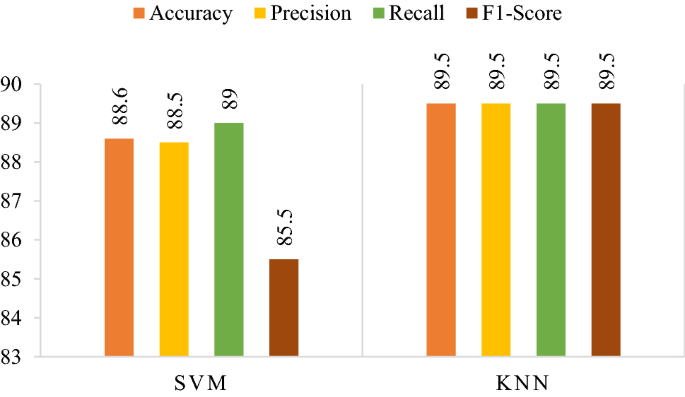
Results obtained from ResNet18's confusion matrix.
Hyperparameter optimization is the process of selecting the best set of hyperparameters for automated algorithms. Optimization is crucial for models because the model's performance depends on the choice of hyperparameters. We optimized parameters such as kernel functions, scale, no. of neighbors, distance metrics, etc., for KNN and SVM. The results obtained from the best parametric settings for different feature vectors are highlighted in bold text and shown in Table 1 . Confusion matrices of both (a) SVM and (b) KNN are illustrated in Fig. 10 .
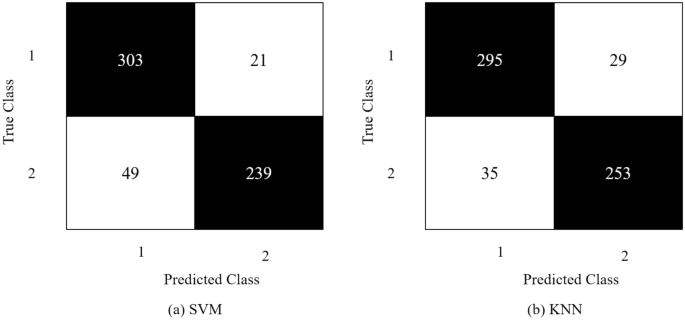
ResNet18's confusion matrix via ( a ) SVM, ( b ) KNN.
Moreover, the feature vector obtained from GoogLeNet’s obtained the highest accuracy of 81% via KNN on Chebyshev as a distance metric with a total number of 154 neighbours. The SVM classified the feature vector with 80.9% accuracy on Gaussian kernel with a 0.41 kernel scale. The tested and optimal metrics (highlighted in bold) are mentioned in Table 2 . Detailed results in other evaluation metrics are mentioned in Fig. 11 , whereas Fig. 12 shows its confusion matrices.
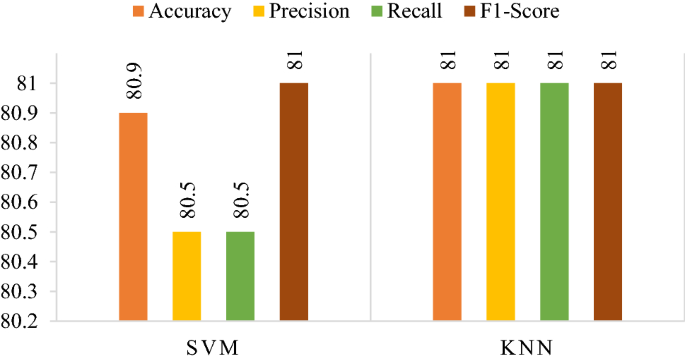
GoogLeNet’s results in terms of ACC, PRE, REC and F1-Score.
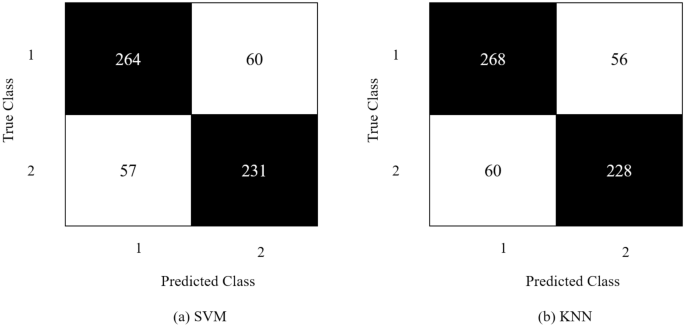
Confusion matrix obtained from GoogLeNet.
SVM and KNN classified the feature vector from SqueezeNet via 69.4% and 68.8%, respectively. The classifiers were evaluated on different parameters, as mentioned in Table 3 and achieved maximum performance on the parameters highlighted in bold text. The results in accuracy, precision, recall and f1-score are mentioned in Fig. 13 . The confusion matrix is shown in Fig. 14 .
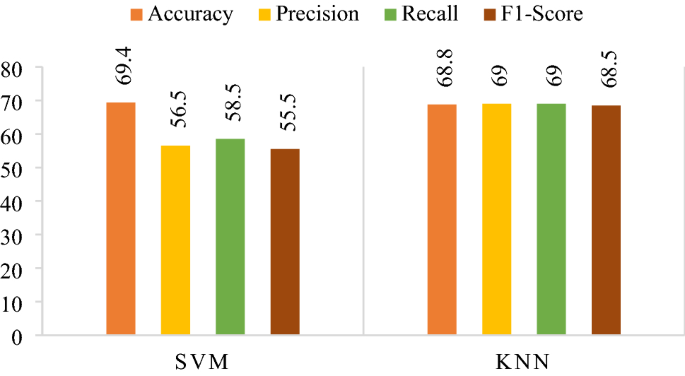
Results obtained from SqueezeNet's confusion matrices.
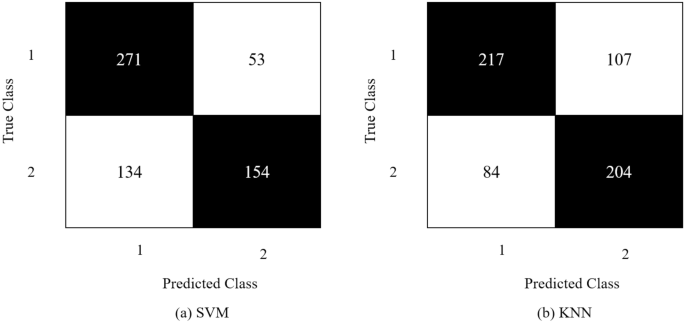
Confusion matrix obtained from SqueezeNet.
Comparison with state-of-the-art methods
This paper proposes a novel architecture to detect and classify deep fake images via DL and ML-based techniques. The proposed framework initially preprocesses the image to generate ELA, which helps determine if the image has been digitally manipulated. The resultant ELA image is then fed to CNN architectures such as GoogLeNet, ResNet18 and ShuffleNet for deep feature extraction. The classification is then performed via SVM and KNN. The proposed method achieved highest accuracy of 89.5% via ResNet18 and KNN. Residual Networks are very efficient and lightweight and perform much better than many other traditional classifiers due to their robust feature extraction and classification techniques. The detailed comparison is shown in Table 4 . Mittal et al. 51 employed Alex Net for deepfake detection. However, the study resulted in a very poor performance. Chandani et al. 50 used a residual network framework to detect deep fake images. Similary, MLP and Meso Inception 4 by Matern et al. 15 and Afchar et al. 26 obtained more than 80% accuracy respectively. Despite being a deep CNN, Residual Networks perform much faster due to their shortcut connections which also aids in boosting the system’s performance. Hence, the proposed method performed much better on the features extracted from ResNet18.
Deep faking is a new technique widely deployed to spread disinformation and hoaxes amongst the people. Even while not all deep fake contents are malevolent, they need to be found because some threaten the world. The main goal of this research was to discover a trustworthy method for identifying deep fake images. Many researchers have been working tirelessly to detect deep fake content using a variety of approaches. However, the importance of this study lies in its use of DL and ML based methods to obtain good results. This study presents a novel framework to detect and classify deep fake images more accurately than many existing systems. The proposed method employs ELA to preprocess images and detect manipulation on a pixel level. The ELA generated images are then supplied to CNNs for feature extraction. These deep features are finally classified using SVM and KNN. The proposed technique achieved highest accuracy of 89.5% via ResNet18’s feature vector & SVM classifier. The results prove the robustness of the proposed method; hence, the system can detect deep fake images in real time. However, the proposed method is developed using image based data. In the future, we will investigate several other CNN architectures on video-based deep fake datasets. We also aim to acquire real life deep fake dataset from the people in our community and use ML and DL techniques to distinguish between deep fake images and regular images to make it more useful and robust. It is worth mentioning that the ground-breaking work will have a significant influence on our society. Using this technology, fake victims can rapidly assess whether the images are real or fake. People will continue to be cautious since our work will enable them to recognize the deep fake image.
Data availability
The datasets used and/or analysed during the current study are available from the corresponding author on reasonable request.
Boylan, J. F. Will Deep-Fake Technology Destroy Democracy (The New York Times, 2018).
Google Scholar
Harwell, D. Scarlett Johansson on fake AI-generated sex videos:‘Nothing can stop someone from cutting and pasting my image’. J. Washigton Post 31 , 12 (2018).
Masood, M. et al. Deepfakes generation and detection: State-of-the-art, open challenges, countermeasures, and way forward. Appl. Intell. 53 , 1–53 (2022).
Amin, R., Al Ghamdi, M. A., Almotiri, S. H. & Alruily, M. Healthcare techniques through deep learning: Issues, challenges and opportunities. IEEE Access 9 , 98523–98541 (2021).
Article Google Scholar
Turek, M.J. Defense Advanced Research Projects Agency . https://www.darpa.mil/program/media-forensics . Media Forensics (MediFor). Vol. 10 (2019).
Schroepfer, M. J. F. Creating a data set and a challenge for deepfakes. Artif. Intell. 5 , 263 (2019).
Kibriya, H. et al. A Novel and Effective Brain Tumor Classification Model Using Deep Feature Fusion and Famous Machine Learning Classifiers . Vol. 2022 (2022).
Rafique, R., Nawaz, M., Kibriya, H. & Masood, M. DeepFake detection using error level analysis and deep learning. in 2021 4th International Conference on Computing & Information Sciences (ICCIS) . 1–4 (IEEE, 2021).
Güera, D. & Delp, E.J. Deepfake video detection using recurrent neural networks. in 2018 15th IEEE International Conference on Advanced Video and Signal Based Surveillance (AVSS) . 1–6 (IEEE, 2018).
Aleem, S. et al. Machine learning algorithms for depression: Diagnosis, insights, and research directions. Electronics 11 (7), 1111 (2022).
Pavan Kumar, M. & Jayagopal, P. Generative adversarial networks: A survey on applications and challenges. Int. J. Multimed. Inf. 10 (1), 1–24 (2021).
Mansoor, M. et al. A machine learning approach for non-invasive fall detection using Kinect. Multimed. Tools Appl. 81 (11), 15491–15519 (2022).
Thies, J., Zollhofer, M., Stamminger, M., Theobalt, C. & Nießner, M. Face2face: Real-time face capture and reenactment of rgb videos. in Proceedings of the IEEE Conference on Computer Vision and Pattern Recognition . 2387–2395 (2016).
Shad, H.S. et al. Comparative Analysis of Deepfake Image Detection Method Using Convolutional Neural Network. Vol. 2021 (2021).
Matern, F., Riess, C. & Stamminger, M. Exploiting visual artifacts to expose deepfakes and face manipulations. in 2019 IEEE Winter Applications of Computer Vision Workshops (WACVW) . 83–92 (IEEE, 2019).
Agarwal, S., Farid, H., Gu, Y., He, M., Nagano, K. & Li, H. Protecting world leaders against deep fakes. in CVPR Workshops . Vol. 1. 38 (2019).
Ciftci, U. A., Demir, I. & Yin, L. Fakecatcher: Detection of Synthetic Portrait Videos Using Biological Signals (Google Patents, 2021).
Yang, X., Li, Y. & Lyu, S. Exposing deep fakes using inconsistent head poses. in ICASSP 2019–2019 IEEE International Conference on Acoustics, Speech and Signal Processing (ICASSP) 8261–8265. (IEEE, 2019).
Rossler, A., Cozzolino, D., Verdoliva, L., Riess, C., Thies, J. & Nießner, M. Faceforensics++: Learning to detect manipulated facial images. in Proceedings of the IEEE/CVF International Conference on Computer Vision . 1–11 (2019).
McCloskey, S. & Albright, M. Detecting GAN-generated imagery using saturation cues. in 2019 IEEE International Conference on Image Processing (ICIP) . 4584–4588. (IEEE, 2019).
Abd Elaziz, M., Dahou, A., Orabi, D.A., Alshathri, S., Soliman, E.M. & Ewees, A.A.J.M. A Hybrid Multitask Learning Framework with a Fire Hawk Optimizer for Arabic Fake News Detection. Vol. 11(2). 258 (2023).
Wodajo, D. & Atnafu, S.J.A.P.A. Deepfake Video Detection Using Convolutional Vision Transformer (2021).
Guarnera, L., Giudice, O. & Battiato, S. Deepfake detection by analyzing convolutional traces. in Proceedings of the IEEE/CVF Conference on Computer Vision and Pattern Recognition Workshops . 666–667 (2020).
Nguyen, H.H., Fang, F., Yamagishi, J. & Echizen, I. Multi-task learning for detecting and segmenting manipulated facial images and videos. in 2019 IEEE 10th International Conference on Biometrics Theory, Applications and Systems (BTAS) . 1–8. (IEEE, 2019).
Khalil, S.S., Youssef, S.M. & Saleh, S.N.J.F.I. iCaps-Dfake: An Integrated Capsule-Based Model for Deepfake Image and Video Detection . Vol. 13(4). 93 (2021).
Afchar, D., Nozick, V., Yamagishi, J. & Echizen, I. Mesonet: A compact facial video forgery detection network. in 2018 IEEE International Workshop on Information Forensics and Security (WIFS) . 1–7 (IEEE, 2018).
Wang, Y. & Dantcheva, A. A video is worth more than 1000 lies. Comparing 3DCNN approaches for detecting deepfakes. in 2020 15th IEEE International Conference on Automatic Face and Gesture Recognition (FG 2020) . 515–519. (IEEE, 2020).
Cozzolino, D., Thies, J., Rössler, A., Riess, C., Nießner, M. & Verdoliva, L.J.A.P.A. Forensictransfer: Weakly-Supervised Domain Adaptation for Forgery Detection (2018).
Huang, G., Liu, Z., Van Der Maaten, L., & Weinberger, K.Q. Densely connected convolutional networks. in Proceedings of the IEEE Conference on Computer Vision and Pattern Recognition . 4700–4708 (2017).
LeCun, Y., Bengio, Y. & Hinton, G. Deep learning. Nature 521 (7553), 436–444 (2015).
Article ADS CAS PubMed Google Scholar
He, K., Zhang, X., Ren, S. & Sun, J. Deep residual learning for image recognition. in Proceedings of the IEEE Conference on Computer Vision and Pattern Recognition . 770–778 (2016).
Nida, N., Irtaza, A. & Ilyas, N. Forged face detection using ELA and deep learning techniques. in 2021 International Bhurban Conference on Applied Sciences and Technologies (IBCAST) . 271–275 (IEEE, 2021).
Kibriya, H., Masood, M., Nawaz, M., Rafique, R. & Rehman, S. Multiclass brain tumor classification using convolutional neural network and support vector machine. in 2021 Mohammad Ali Jinnah University International Conference on Computing (MAJICC) . 1–4 (IEEE, 2021).
Kibriya, H., Masood, M., Nawaz, M. & Nazir, T. J. M. T. Multiclass classification of brain tumors using a novel CNN architecture. Multimed. Tool Appl. 81 , 1–17 (2022).
Salman, F. M. & Abu-Naser, S. S. Classification of real and fake human faces using deep learning. IJAER 6 (3), 1–14 (2022).
Anaraki, A. K., Ayati, M. & Kazemi, F. J. Magnetic resonance imaging-based brain tumor grades classification and grading via convolutional neural networks and genetic algorithms. Information 39 (1), 63–74 (2019).
Albawi, S., Mohammed, T.A. & Al-Zawi, S. Understanding of a convolutional neural network. in 2017 International Conference on Engineering and Technology (ICET) . 1–6 (IEEE, 2017).
O'Shea, K. & Nash, R. J. An Introduction to Convolutional Neural Networks (2015).
Szegedy, C. et al. Going deeper with convolutions. in Proceedings of the IEEE Conference on Computer Vision and Pattern Recognition . 1–9 (2015).
Iandola, F.N., Han, S., Moskewicz, M.W., Ashraf, K., Dally, W.J. & Keutzer, K.J. SqueezeNet : AlexNet-Level Accuracy with 50× Fewer Parameters and< 0.5 MB Model Size (2016).
He, K., Zhang, X., Ren, S. & Sun, J. Deep residual learning for image recognition. in Proceedings of the IEEE Conference on Computer Vision and Pattern Recognition , Las Vegas, USA. 770–778 (2016).
Introduction to Residual Networks . https://www.geeksforgeeks.org/introduction-to-residual-networks/ (2020).
Ali, L. et al. Performance evaluation of deep CNN-based crack detection and localization techniques for concrete structures. Sensors 21 (5), 1688 (2021).
Article ADS PubMed PubMed Central Google Scholar
Ramzan, F. et al. A deep learning approach for automated diagnosis and multi-class classification of Alzheimer’s disease stages using resting-state fMRI and residual neural networks. J. Med. Syst. 44 (2), 1–16 (2020).
Article MathSciNet Google Scholar
Mancini, M., Costante, G., Valigi, P. & Ciarfuglia, T.A. Fast robust monocular depth estimation for obstacle detection with fully convolutional networks. in 2016 IEEE/RSJ International Conference on Intelligent Robots and Systems (IROS) . 4296–4303 (IEEE, 2016).
Kasim, N., Rahman, N., Ibrahim, Z. & Mangshor, N. A. Celebrity face recognition using deep learning. Indonesian J. Electr. Eng. Comput. Sci. 12 (2), 476–481 (2018).
Rezgui, D. & Lachiri, Z. ECG biometric recognition using SVM-based approach. IEEJ Trans. Electr. Electron. Eng. 11 , S94–S100 (2016).
Y. U. Computational Intelligence and Photography Lab. Real-and-Fake-Face-Detection (2019).
Tolosana, R., Romero-Tapiador, S., Fierrez, J. & Vera-Rodriguez, R. Deepfakes evolution: Analysis of facial regions and fake detection performance. in International Conference on Pattern Recognition . 442–456 (Springer, 2016).
Mehra, A. Deepfake Detection Using Capsule Networks with Long Short-Term Memory Networks (University of Twente, 2020).
Mittal, H., Saraswat, M., Bansal, J.C. & Nagar, A. Fake-face image classification using improved quantum-inspired evolutionary-based feature selection method. in 2020 IEEE Symposium Series on Computational Intelligence (SSCI) . 989–995 (IEEE, 2020).
Chandani, K. & Arora, M. Automatic facial forgery detection using deep neural networks. in Advances in Interdisciplinary Engineering . 205–214 (Springer, 2021).
Lee, S., Tariq, S., Shin, Y. & Woo, S. S. Detecting handcrafted facial image manipulations and GAN-generated facial images using Shallow-FakeFaceNet. Appl. Soft Comput. 105 , 107256 (2021).
Download references
This research was supported by the Ministry of Education, Youth and Sports of the Czech Republic under the grant SP2023/007 conducted by VSB—Technical University of Ostrava.
Author information
Authors and affiliations.
Department of Computer Science, University of Engineering and Technology, Taxila, Pakistan, 47050
Rimsha Rafique & Rashid Amin
Department of Electrical Engineering, Chonnam National University, Gwangju, 61186, South Korea
Rahma Gantassi
Department of Computer Science, University of Chakwal, Chakwal, 48800, Pakistan
Rashid Amin
Department of Quantitative Methods and Economic Informatics, Faculty of Operation and Economics of Transport and Communications, University of Zilina, 01026, Zilina, Slovakia
Jaroslav Frnda
Department of Telecommunications, Faculty of Electrical Engineering and Computer Science, VSB Technical University of Ostrava, 70800, Ostrava, Czech Republic
Faculty of Applied Sciences and Technology, Universiti Tun Hussein Onn Malaysia, KM1 Jalan Pagoh, 84600, Pagoh, Johor, Malaysia
Aida Mustapha
Durma College of Science and Humanities, Shaqra University, Shaqra, 11961, Saudi Arabia
Asma Hassan Alshehri
You can also search for this author in PubMed Google Scholar
Contributions
All the authors contributed equally.
Corresponding author
Correspondence to Rashid Amin .
Ethics declarations
Competing interests.
The authors declare no competing interests.
Additional information
Publisher's note.
Springer Nature remains neutral with regard to jurisdictional claims in published maps and institutional affiliations.
Rights and permissions
Open Access This article is licensed under a Creative Commons Attribution 4.0 International License, which permits use, sharing, adaptation, distribution and reproduction in any medium or format, as long as you give appropriate credit to the original author(s) and the source, provide a link to the Creative Commons licence, and indicate if changes were made. The images or other third party material in this article are included in the article's Creative Commons licence, unless indicated otherwise in a credit line to the material. If material is not included in the article's Creative Commons licence and your intended use is not permitted by statutory regulation or exceeds the permitted use, you will need to obtain permission directly from the copyright holder. To view a copy of this licence, visit http://creativecommons.org/licenses/by/4.0/ .
Reprints and permissions
About this article
Cite this article.
Rafique, R., Gantassi, R., Amin, R. et al. Deep fake detection and classification using error-level analysis and deep learning. Sci Rep 13 , 7422 (2023). https://doi.org/10.1038/s41598-023-34629-3
Download citation
Received : 26 December 2022
Accepted : 04 May 2023
Published : 08 May 2023
DOI : https://doi.org/10.1038/s41598-023-34629-3
Share this article
Anyone you share the following link with will be able to read this content:
Sorry, a shareable link is not currently available for this article.
Provided by the Springer Nature SharedIt content-sharing initiative
This article is cited by
Fake and propaganda images detection using automated adaptive gaining sharing knowledge algorithm with densenet121.
- A. Muthukumar
- M. Thanga Raj
- P. Kaleeswari
Journal of Ambient Intelligence and Humanized Computing (2024)
Deepfake detection using convolutional vision transformers and convolutional neural networks
- Ahmed Hatem Soudy
- Omnia Sayed
- Salwa O. Slim
Neural Computing and Applications (2024)
Image forgery detection: comprehensive review of digital forensics approaches
- Satyendra Singh
- Rajesh Kumar
Journal of Computational Social Science (2024)
By submitting a comment you agree to abide by our Terms and Community Guidelines . If you find something abusive or that does not comply with our terms or guidelines please flag it as inappropriate.
Quick links
- Explore articles by subject
- Guide to authors
- Editorial policies
Sign up for the Nature Briefing: AI and Robotics newsletter — what matters in AI and robotics research, free to your inbox weekly.

Error Analysis
Introduction, significant figures, the idea of error, classification of error, a. mean value, b. measuring error, maximum error, probable error, average deviation, standard deviation, c. standard deviation, propagation of errors.
Grab your spot at the free arXiv Accessibility Forum
Help | Advanced Search
Quantum Physics
Title: closed-loop designed open-loop control of quantum systems: an error analysis.
Abstract: Quantum Lyapunov control, an important class of quantum control methods, aims at generating converging dynamics guided by Lyapunov-based theoretical tools. However, unlike the case of classical systems, disturbance caused by quantum measurement hinders direct and exact realization of the theoretical feedback dynamics designed with Lyapunov theory. Regarding this issue, the idea of closed-loop designed open-loop control has been mentioned in literature, which means to design the closed-loop dynamics theoretically, simulate the closed-loop system, generate control pulses based on simulation and apply them to the real plant in an open-loop fashion. Based on bilinear quantum control model, we analyze in this article the error, i.e., difference between the theoretical and real systems' time-evolved states, incurred by the procedures of closed-loop designed open-loop control. It is proved that the error at an arbitrary time converges to a unitary transformation of initialization error as the number of simulation grids between 0 and that time tends to infinity. Moreover, it is found that once the simulation accuracy reaches a certain level, adopting more accurate (thus possibly more expensive) numerical simulation methods does not efficiently improve convergence. We also present an upper bound on the error norm and an example to illustrate our results.
Subjects: | Quantum Physics (quant-ph) |
Cite as: | [quant-ph] |
(or [quant-ph] for this version) | |
Focus to learn more arXiv-issued DOI via DataCite |
Submission history
Access paper:.
- HTML (experimental)
- Other Formats
References & Citations
- INSPIRE HEP
- Google Scholar
- Semantic Scholar
BibTeX formatted citation

Bibliographic and Citation Tools
Code, data and media associated with this article, recommenders and search tools.
- Institution
arXivLabs: experimental projects with community collaborators
arXivLabs is a framework that allows collaborators to develop and share new arXiv features directly on our website.
Both individuals and organizations that work with arXivLabs have embraced and accepted our values of openness, community, excellence, and user data privacy. arXiv is committed to these values and only works with partners that adhere to them.
Have an idea for a project that will add value for arXiv's community? Learn more about arXivLabs .
Featured Topics
Featured series.
A series of random questions answered by Harvard experts.
Explore the Gazette
Read the latest.

Women who follow Mediterranean diet live longer
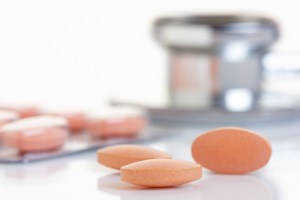
Harvard-led study IDs statin that may block pathway to some cancers
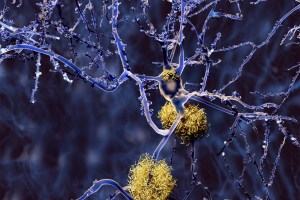
New Alzheimer’s study suggests genetic cause of specific form of disease
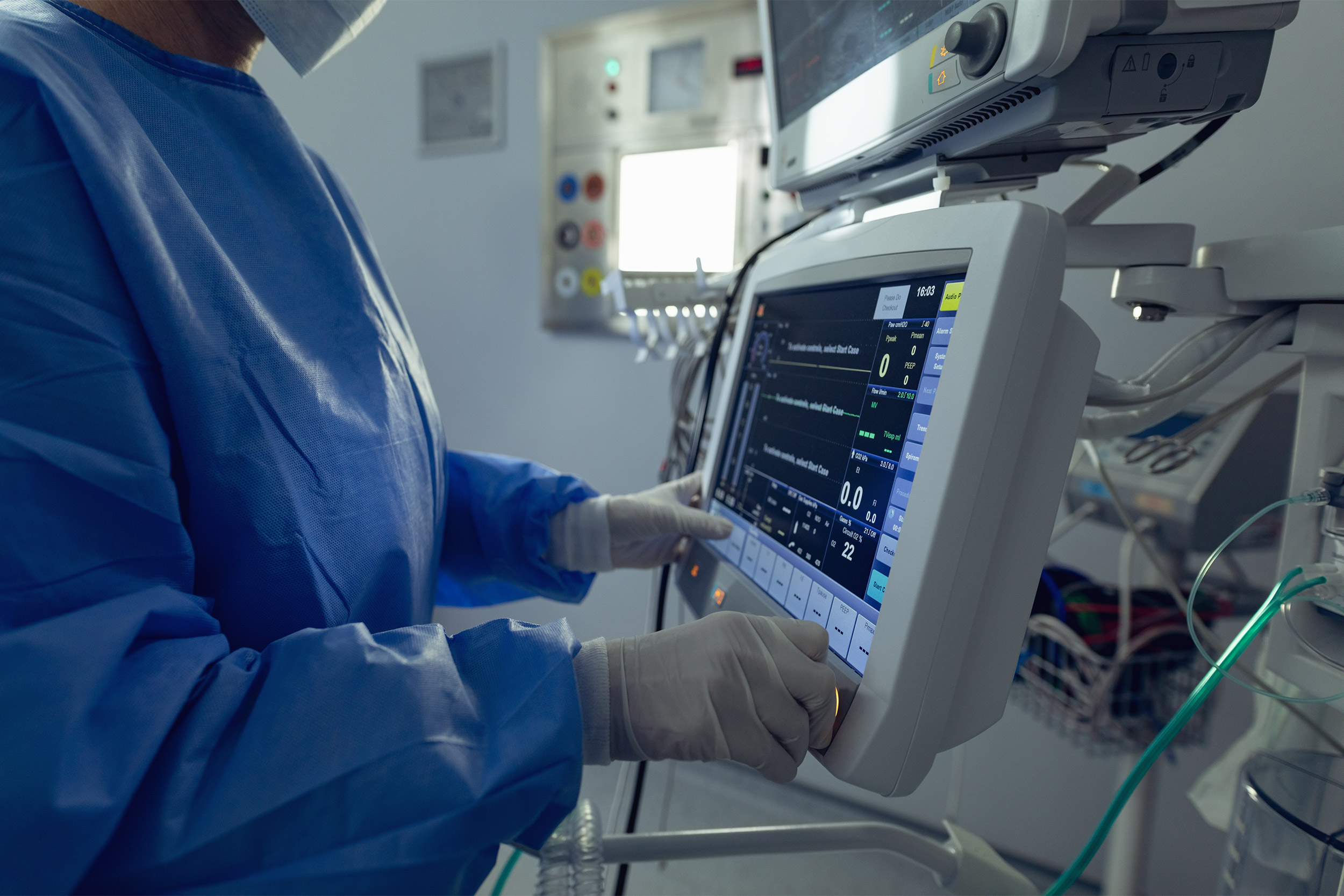
High rate of diagnostic error found in ICU
Nationwide study pinpoints testing mistakes as most common cause.
A new study from Harvard-affiliated Brigham and Women’s Hospital, in collaboration with researchers at the University of California San Francisco, has shed light on the rate and impact of diagnostic errors in hospital settings.
In an analysis of electronic health records from 29 hospitals across the country of 2,428 patients who had either been transferred to an intensive care unit (ICU) or died in the hospital, the researchers found 550 patients (23 percent) experienced a diagnostic error, the majority of which were harmful to the patient. The researchers also determined the most common causes of diagnostic errors.
The study was published Monday in the journal JAMA Internal Medicine.
“We know diagnostic errors are dangerous and hospitals are obviously interested in reducing their frequency, but it’s much harder to do this when we don’t know what’s causing these errors or what their direct impact is on individual patients,” said senior author Jeffrey L. Schnipper of the Brigham’s Division of General Internal Medicine and Primary Care . “We found that diagnostic errors can largely be attributed to either errors in testing, or errors in assessing patients, and this knowledge gives us new opportunities to solve these problems.”
“It appears to be that only a minority of deaths in hospitals are linked to diagnostic errors, but even a single patient death that might have been prevented with a better diagnostic process is one death too many.” Jeffrey L. Schnipper, Women and Brigham’s Hospital
Diagnostic errors are defined in medicine as any failure to either accurately explain a patient’s health problem or a failure to communicate that information to the patient. Some national efforts are currently underway to detect and address their causes, including DECODE , a Diagnostic Centers of Excellence program at the Brigham that focuses on decreasing diagnostic errors in medical imaging by implementing and evaluating a highly resilient system for care planning and coordination, as well as a peer learning system for clinical providers. Other projects underway that involve BWH researchers will address cases and causes of delayed diagnosis of cancer , explore how electronic health records contribute to diagnostic errors , and more.
To date, few studies have quantified the prevalence of diagnostic errors in hospitals or their most common underlying causes. In this study, cases were assessed for diagnostic errors by teams of two physicians who received extensive training in error adjudication and utilized multiple quality control steps. They found that 550 of the patients in their cohort (23 percent) experienced a diagnostic error in the hospital. Of these, 486 (17 percent of all patients) experienced some form of harm because of these errors. Of the 1,863 patients who died, the researchers judged that a diagnostic error was a contributing factor in 121 cases (6.6 percent).
“It appears to be that only a minority of deaths in hospitals are linked to diagnostic errors, but even a single patient death that might have been prevented with a better diagnostic process is one death too many,” said Schnipper.
The researchers found that most errors were attributable to errors in assessing patients, or errors in ordering and interpreting diagnostic tests.
“These two parts of the diagnostic process feed directly into each other,” said Schnipper. “If you don’t think of the correct possible diagnosis during your assessment of a patient, you’re not going to order the right tests. And if you order the wrong test or order the right test but misinterpret the result, this will inevitably change how you then assess a patient.”
While the research demonstrates the dangers that diagnostic errors can pose to patients, the researchers maintain that the rate of diagnostic errors in their specific population of patients, who all had experienced bad outcomes, does not represent the general rate across hospitals. The researchers are next exploring how health systems can implement surveillance systems to catch diagnostic errors as they occur, compare results across hospitals, and start pilot testing possible solutions.
“Our study does not tell us the overall frequency of diagnostic errors in the hospital, but it does tell us that there’s more we can be doing to prevent these types of errors from occurring,” said Schnipper.
Authors of the study include Andrew D. Auerbach, Tiffany M. Lee, Colin C. Hubbard, Sumant R. Ranji, Katie Raffel, Gilmer Valdes, John Boscardin, Anuj K. Dalal (BWH), Alyssa Harris, and Ellen Flynn for the UPSIDE Research Group. The authors declared no competing interests.
This study was supported by the Agency for Healthcare Research and Quality (R01HS027369).
Share this article
You might like.
Large study shows benefits against cancer, cardiovascular mortality, also identifies likely biological drivers of better health

Cholesterol-lowering drug suppresses chronic inflammation that creates dangerous cascade

Findings eventually could pave way to earlier diagnosis, treatment, and affect search for new therapies
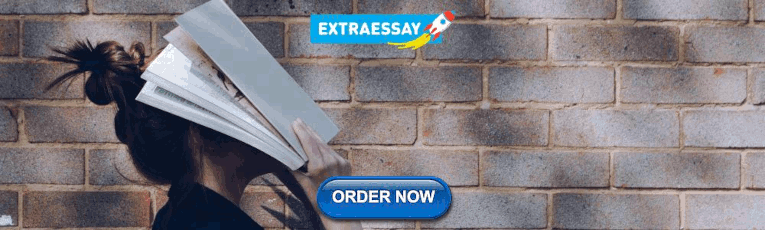
When should Harvard speak out?
Institutional Voice Working Group provides a roadmap in new report
Had a bad experience meditating? You're not alone.
Altered states of consciousness through yoga, mindfulness more common than thought and mostly beneficial, study finds — though clinicians ill-equipped to help those who struggle
Finding right mix on campus speech policies
Legal, political scholars discuss balancing personal safety, constitutional rights, academic freedom amid roiling protests, cultural shifts
Academia.edu no longer supports Internet Explorer.
To browse Academia.edu and the wider internet faster and more securely, please take a few seconds to upgrade your browser .
Enter the email address you signed up with and we'll email you a reset link.
- We're Hiring!
- Help Center
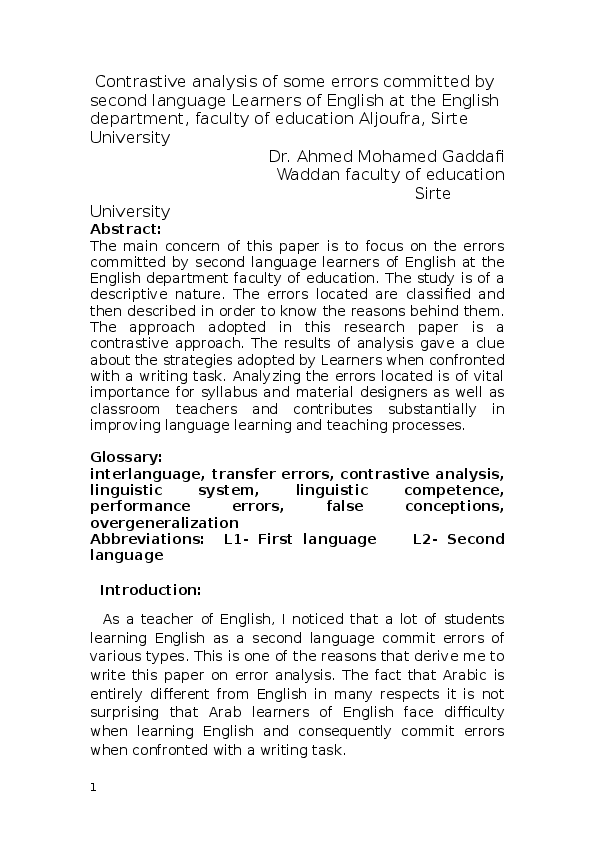
Research paper on error analysis

The main concern of this paper is to focus on the errors committed by second language learners of English at the English department faculty of education. The study is of a descriptive nature. The errors located are classified and then described in order to know the reasons behind them. The approach adopted in this research paper is a contrastive approach. The results of analysis gave a clue about the strategies adopted by Learners when confronted with a writing task. Analyzing the errors located is of vital importance for syllabus and material designers as well as classroom teachers and contributes substantially in improving language learning and teaching processes.
Related Papers
Rajesh Prakhya
The aim of this paper is to investigate errors made by second and foreign language (L2) learners so as to understand the strategies and techniques used in the process of second and foreign language learning. Error analysis is a very important area of applied linguistics as well as of second and foreign language learning. It is also a systematic method to analyze learners' errors. Errors are not always bad, rather they are crucial parts and aspects in the process of learning a language. They may provide insights into the complicated processes of language development as well as a systematic way for identifying, describing and explaining students' errors. Errors may also help to better understand the process of second and foreign language acquisition. This study tries to investigate why Pakistani ESL and Iranian EFL learners fail to produce grammatically correct sentences in English, in spite of having English as a compulsory subject at all levels in their learning institutions and schools. What are the reasons for their poor English written performance? In the present study, the writing assignments of university students as well as intermediate English learners were analyzed for 53 the purpose of error analysis. Results of the analysis suggest that students lack grammatical accuracy in their writing and are not sure of the grammatical rules that may apply in their writing in English. The study concludes that they are highly influenced by the rules of their first language (L1).
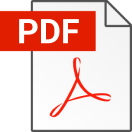
Bahram Kazemian , د. شهباز
The aim of this paper is to investigate errors made by second and foreign language (L2) learners so as to understand the strategies and techniques used in the process of second and foreign language learning. Error analysis is a very important area of applied linguistics as well as of second and foreign language learning. It is also a systematic method to analyze learners' errors. Errors are not always bad, rather they are crucial parts and aspects in the process of learning a language. They may provide insights into the complicated processes of language development as well as a systematic way for identifying, describing and explaining students' errors. Errors may also help to better understand the process of second and foreign language acquisition. This study tries to investigate why Pakistani ESL and Iranian EFL learners fail to produce grammatically correct sentences in English, in spite of having English as a compulsory subject at all levels in their learning institutions and schools. What are the reasons for their poor English written performance? In the present study, the writing assignments of university students as well as intermediate English learners were analyzed for the purpose of error analysis. Results of the analysis suggest that students lack grammatical accuracy in their writing and are not sure of the grammatical rules that may apply in their writing in English. The study concludes that they are highly influenced by the rules of their first language (L1).
Rida Sarfraz
Langkawi: Journal of The Association for Arabic and English
Fahmy Imaniar
Dana Ferris
Mediterranean Journal of Social Sciences
Julija Jaramaz
IJAERS Journal
Of all the language skills, writing is the most difficult skill for the students who learn English as a second language because they have less experience with written expression. In this research paper, efforts have been made to prove the relevance of error analysis in the English writing skills of the Intermediate Level students. To conduct the research, a random written sample of a Class XII student of Jawahar Navodaya Vidyalaya, Navsari, Gujarat, has been taken up. Errors in the written text have been categorized using the theory provided by Dulay, Burt, and Krashen (1982, pp.146). The need of this research arose after looking into the writing errors made by the JNV students of Intermediate Level, where English is taught and learned as a Second Language. The intent is to prove how error analysis can help to possibly identify sources of errors in learners' writing; categorize the errors, and try to present a method for correction and improve the writing skills of the students. This study can be of great help to the English teachers of JNVs that were established in 1986 to bring out the best of Indian rural talent; to improve upon the writing skills of their students.
Journal of Research on English and Language Learning (J-REaLL)
Writing is one of the important English skills. The process to make good writing is difficult. There were errors in the writing process. Therefore, the researcher was interested in analyzing the kinds of errors in writing. The problem of this study is to identify the errors made by the third-semester students of English education department at Universitas PGRI Madiun in the academic year 2021/2022. A descriptive qualitative method is used to analyze this research. The researcher analyzed subject-verb agreement errors, verb tense errors, verb form errors, singular/plural noun ending errors, and word form errors. The steps to finding the data are: collecting the sources of the data, understanding the content of the writing, selecting the test which contains errors, analyzing the collected data, and drawing conclusions. The result of this research, from the lowest to the highest, is as follows: singular/plural noun ending errors (3.40%), subject-verb agreement errors (12.24%), verb for...
EXPOSURE : JURNAL PENDIDIKAN BAHASA INGGRIS
dedi aprianto
Writing, the language skill three, is a part of the productive skill of the four intergrated language skills. Error Analysis as the language learning approach to study about the problematic English written product. It is the mostly-complecated skill than the others. This current study aims at analyzing the grammatical errors made of and investigating the levels of English grammatical errors produced. This study was carried out by using a descriptive quantitative research method by using a test, a guided writing test by letting the students write the patterned texts by answering the questions given, as the instrument to obtain the data on the error types made and the levels of English grammatical errors produced. This study shows that the non-syntactical errors found are spellings (44%), punctuations (19%), Capitalizations LCL (14%), and the selection of words (23%). Whereas the syntactical errors are the use of articles (5.5%), parts of speech (8%), subject-verbs agreement (11%), us...
Loading Preview
Sorry, preview is currently unavailable. You can download the paper by clicking the button above.
RELATED PAPERS
International Journal of Professional Business Review
Marites Catabay
Andrew D Cohen
Danny Iswantoro
Journal of English Language Teaching and Applied Linguistics
Mohamed Seddik
Nisar A Koka
Panchanan Mohanty
Akram Nayernia
Pakistan Languages and Humanities Review
Atta Ur Rehamn Jadoon
Ana Ibanez Moreno
Lia Nurmalia
Ferdinand Cortez
Dr. Sudha Mishra
Nikita Suthar
Natu Clemente
lamine ndiaye
Language and Language Teaching Journal
amelia estrelita
Setyo Cahyono
Datokarama English Education Journal
Yuni Amelia
Journal of Emerging Technologies and Innovative Research (JETIR)
Premchand Nagdeote
Jose Tummers
Published article
Sahar M . Alzahrani
- We're Hiring!
- Help Center
- Find new research papers in:
- Health Sciences
- Earth Sciences
- Cognitive Science
- Mathematics
- Computer Science
- Academia ©2024
- DOI: 10.47637/griyacendikia.v9i2.1490
- Corpus ID: 271828747
Analysis of Errors in Students' Writing of Analytical Exposition Text at the Eleventh Grade of SMA Negeri 2 Kotabumi Academic Year 2023/2024
- Universitas Muhammadiyah Kotabumi , Desi Lusiana , +4 authors Eksposisi Analitik
- Published in Griya Cendikia 8 August 2024
9 References
Error analysis, an analysis of students’ difficulties in writing descriptive text, instrumen pengumpulan data, an analysis of students’ errors in writing descriptive texts, students' perceptions: using writing process approach in efl writing class, related papers.
Showing 1 through 3 of 0 Related Papers
Biostatistics Graduate Program
Siwei zhang is first author of jamia paper.
Posted by duthip1 on Tuesday, August 13, 2024 in News .
Congratulations to PhD candidate Siwei Zhang , alumnus Nicholas Strayer (PhD 2020; now at Posit), senior biostatistician Yajing Li , and assistant professor Yaomin Xu on the publication of “ PheMIME: an interactive web app and knowledge base for phenome-wide, multi-institutional multimorbidity analysis ” in the Journal of the American Medical Informatics Association on August 10. As stated in the abstract, “PheMIME provides an extensive multimorbidity knowledge base that consolidates data from three EHR systems, and it is a novel interactive tool designed to analyze and visualize multimorbidities across multiple EHR datasets. It stands out as the first of its kind to offer extensive multimorbidity knowledge integration with substantial support for efficient online analysis and interactive visualization.” Collaborators on the paper include members of Vanderbilt’s Division of Genetic Medicine, Department of Biomedical Informatics, Department of Urology, Department of Obstetrics and Gynecology, Division of Hematology and Oncology, VICTR , Department of Pharmacology, Center for Drug Safety and Immunology, and Department of Psychiatry and Behavioral Sciences, as well as colleagues at Massachusetts General Hospital, North Carolina State University, Murdoch University (Australia), and the Broad Institute. Dr. Xu is corresponding author.
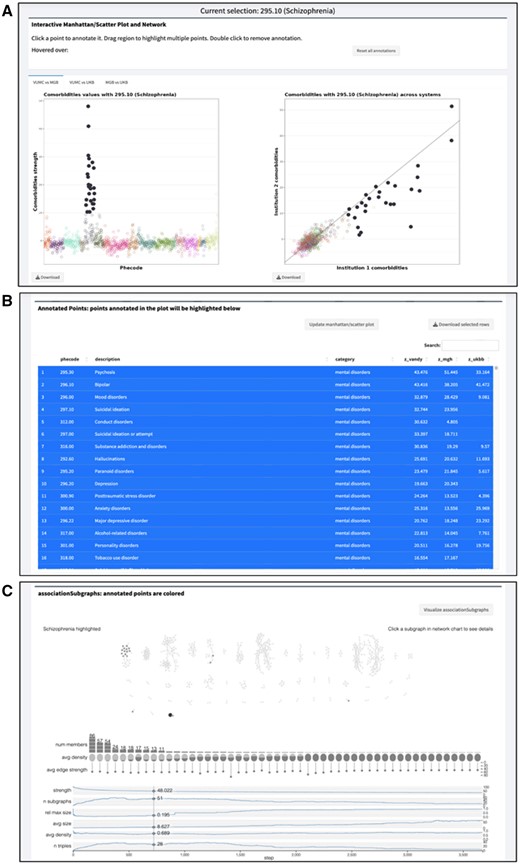
Tags: cloud computing , EHR , methods , network analysis , R , schizophrenia , Shiny
Leave a Response
You must be logged in to post a comment

- Working Papers
- Center for Financial Research
- Researchers
- Research Fellows
- Senior Advisor
- Special Advisor
- 2023 Fellows
- 2022 Fellows
- Visiting Scholar
- 23rd Bank Research Conference
- Conference Videos
- Speaker Information
- Poster Videos
- Poster Session Videos
- Previous Conference Programs
- Other Conferences
- 2021-2022 FDIC Academic Challenge
- 2020-2021 FDIC Academic Challenge
- Prior Years
- Research Assistants
- Internships
- Visiting Scholars
Working Papers presented as PDF files on this page reference Portable Document Format (PDF) files. Adobe Acrobat, a reader available for free on the Internet, is required to display or print PDF files. ( PDF Help )
Most Recently Published Working Papers |
---|
FDIC Center for Financial Research Working Paper No. 2024-03 |
FDIC Center for Financial Research Working Paper No. 2024-02 |
FDIC Center for Financial Research Working Paper No. 2024-01 |
FDIC Center for Financial Research Working Paper No. 2023-03 |
FDIC Center for Financial Research Working Paper No. 2023-02 |
The Center for Financial Research (CFR) Working Paper Series allows CFR staff and their coauthors to circulate preliminary research findings to stimulate discussion and critical comment. Views and opinions expressed in CFR Working Papers reflect those of the authors and do not necessarily reflect those of the FDIC or the United States. Comments and suggestions are welcome and should be directed to the authors. References should cite this research as a “FDIC CFR Working Paper” and should note that findings and conclusions in working papers may be preliminary and subject to revision.
Last Updated: August 4, 2024
Log in using your username and password
- Search More Search for this keyword Advanced search
- Latest content
- Current issue
- BMJ Journals
You are here
- Volume 78, Issue 9
- Estimated changes in free sugar consumption one year after the UK soft drinks industry levy came into force: controlled interrupted time series analysis of the National Diet and Nutrition Survey (2011–2019)
- Article Text
- Article info
- Citation Tools
- Rapid Responses
- Article metrics

- http://orcid.org/0000-0003-1857-2122 Nina Trivedy Rogers 1 ,
- http://orcid.org/0000-0002-3957-4357 Steven Cummins 2 ,
- Catrin P Jones 1 ,
- Oliver Mytton 3 ,
- Mike Rayner 4 ,
- Harry Rutter 5 ,
- Martin White 1 ,
- Jean Adams 1
- 1 MRC Epidemiology Unit, University of Cambridge School of Clinical Medicine, Institute of Metabolic Science, Cambridge Biomedical Campus , University of Cambridge , Cambridge , UK
- 2 Department of Public Health, Environments & Society , London School of Hygiene & Tropical Medicine , London , UK
- 3 Great Ormond Street Institute of Child Health , University College London , London , UK
- 4 Nuffield Department of Population Health , University of Oxford , Oxford , UK
- 5 Department of Social and Policy Sciences , , University of Bath , Bath , UK
- Correspondence to Dr Nina Trivedy Rogers, MRC Epidemiology Unit, University of Cambridge School of Clinical Medicine, Institute of Metabolic Science, Cambridge Biomedical Campus, University of Cambridge, Cambridge, CB2 1TN, UK; nina.rogers{at}mrc-epid.cam.ac.uk
Background The UK soft drinks industry levy (SDIL) was announced in March 2016 and implemented in April 2018, encouraging manufacturers to reduce the sugar content of soft drinks. This is the first study to investigate changes in individual-level consumption of free sugars in relation to the SDIL.
Methods We used controlled interrupted time series (2011–2019) to explore changes in the consumption of free sugars in the whole diet and from soft drinks alone 11 months after SDIL implementation in a nationally representative sample of adults (>18 years; n=7999) and children (1.5–19 years; n=7656) drawn from the UK National Diet and Nutrition Survey. Estimates were based on differences between observed data and a counterfactual scenario of no SDIL announcement/implementation. Models included protein consumption (control) and accounted for autocorrelation.
Results Accounting for trends prior to the SDIL announcement, there were absolute reductions in the daily consumption of free sugars from the whole diet in children and adults of 4.8 g (95% CI 0.6 to 9.1) and 10.9 g (95% CI 7.8 to 13.9), respectively. Comparable reductions in free sugar consumption from drinks alone were 3.0 g (95% CI 0.1 to 5.8) and 5.2 g (95% CI 4.2 to 6.1). The percentage of total dietary energy from free sugars declined over the study period but was not significantly different from the counterfactual.
Conclusion The SDIL led to significant reductions in dietary free sugar consumption in children and adults. Energy from free sugar as a percentage of total energy did not change relative to the counterfactual, which could be due to simultaneous reductions in total energy intake associated with reductions in dietary free sugar.
- PUBLIC HEALTH
Data availability statement
Data are available in a public, open access repository. Data from the National Diet and Nutrition Survey years 1–11 (2008–09 to 2018–19) can be accessed on the UK Data Service ( https://ukdataservice.ac.uk/ ).
This is an open access article distributed in accordance with the Creative Commons Attribution 4.0 Unported (CC BY 4.0) license, which permits others to copy, redistribute, remix, transform and build upon this work for any purpose, provided the original work is properly cited, a link to the licence is given, and indication of whether changes were made. See: https://creativecommons.org/licenses/by/4.0/ .
https://doi.org/10.1136/jech-2023-221051
Statistics from Altmetric.com
Request permissions.
If you wish to reuse any or all of this article please use the link below which will take you to the Copyright Clearance Center’s RightsLink service. You will be able to get a quick price and instant permission to reuse the content in many different ways.
WHAT IS ALREADY KNOWN ON THIS TOPIC
High intakes of free sugars are associated with a range of non-communicable diseases. Sugar sweetened beverages constitute a major source of dietary free sugars in children and adults.
The UK soft drink industry levy (SDIL) led to a reduction in the sugar content in many sugar sweetened beverages and a reduction in household purchasing of sugar from drinks.
No previous study has examined the impact of the SDIL on total dietary consumption of free sugars at the individual level.
WHAT THIS STUDY ADDS
There were declining trends in the intake of dietary free sugar in adults and children prior to the UK SDIL.
Accounting for prior trends, 1 year after the UK SDIL came into force children and adults further reduced their free sugar intake from food and drink by approximately 5 g/day and 11 g/day, respectively. Children and adults reduced their daily free sugar intake from soft drinks alone by approximately 3 g/day and approximately 5 g/day, respectively.
Energy intake from free sugars as a proportion of total energy consumed did not change significantly following the UK SDIL, indicating energy intake from free sugar was reducing simultaneously with overall total energy intake.
HOW THIS STUDY MIGHT AFFECT RESEARCH, PRACTICE OR POLICY
The UK SDIL was associated with significant reductions in consumption of free sugars from soft drinks and across the whole diet and reinforces previous research indicating a reduction in purchasing. This evidence should be used to inform policy when extending or considering other sugar reduction strategies.
Energy intake from free sugars has been falling but levels remain higher than the 5% recommendation set by the WHO. Reductions in dietary sugar in relation to the SDIL may have driven significant reductions in overall energy.
Introduction
High consumption of free sugars is associated with non-communicable diseases. 1 Guidelines from the World Health Organization (WHO) and the UK Scientific Advisory Committee on Nutrition (SACN) suggest limiting free sugar consumption to below 5% of total energy intake to achieve maximum health benefits, 1 2 equivalent to daily maximum amounts of 30 g for adults, 24 g for children (7–10 years) and 19 g for young children (4–6 years). In the UK, consumption of free sugar is well above the recommended daily maximum, although levels have fallen over the last decade. 3 For example, adolescents consume approximately 70 g/day 4 and obtain 12.3% of their energy from free sugars. 3 Sugar sweetened beverages (SSBs) constitute a major source of free sugar in the UK diet, 2 5 and are the largest single source for children aged 11–18 years where they make up approximately one-third of their daily sugar intake. 6 A growing body of evidence has shown a link between consumption of SSBs and higher risk of weight gain, type 2 diabetes, coronary heart disease and premature mortality, 7 such that the WHO recommends taxation of SSBs in order to reduce over-consumption of free sugars and to improve health. 8 To date, >50 countries have introduced taxation on SSBs, which has been associated with a reduction in sales and dietary intake of free sugar from SSBs. 9 Reductions in the prevalence of childhood obesity 10 11 and improvements in dental health outcomes 12 13 have also been reported.
In March 2016 the UK government announced the UK soft drink industry levy (SDIL), a two-tier levy on manufacturers, importers and bottlers of soft drinks which would come into force in March 2018. 14 The levy was designed to incentivise manufacturers to reformulate and reduce the free sugar content of SSBs (see details in online supplemental text 1 ).
Supplemental material
One year after the UK SDIL was implemented there was evidence of a reduction in the sugar content of soft drinks 15 and households on average reduced the amount of sugar purchased from soft drinks by 8 g/week with no evidence of substitution with confectionary or alcohol. 16 However, lack of available data meant it was not possible to examine substitution of purchasing other sugary foods and drinks, which has previously been suggested in some but not all studies. 17 18 Household purchasing only approximates individual consumption because it captures only those products brought into the home, products may be shared unequally between household members, and it does not account for waste.
To examine the effects of the SDIL on total sugar intake at the individual level, in this study we used surveillance data collected using 3- or 4-day food diaries as part of the UK National Diet and Nutrition Survey (NDNS). We aimed to examine changes in absolute and relative consumption of free sugars from soft drinks alone and from both food and drinks (allowing us to consider possible substitutions with other sugary food items), following the announcement and implementation of the UK SDIL.
Data source
We used 11 years of data (2008–2019) from the NDNS. Data collection, sampling design and information on response is described in full elsewhere. 19 In brief, NDNS is a continuous national cross-sectional survey capturing information on food consumption, nutritional status and nutrient intake inside and outside of the home in a representative annual sample of approximately 500 adults and 500 children (1.5–18 years) living in private households in the UK. Participants are sampled throughout the year, such that in a typical month about 40 adults and 40 children participate (further details are shown in online supplemental text 2 ).
Outcomes of interest
Outcomes of interest were absolute and relative changes in the total intake of dietary free sugar from (1) all food and soft drinks combined and (2) from soft drinks alone. A definition of free sugar is given in online supplemental text 3 . Drink categories examined were those that fell within the following NDNS categories: soft drinks – not low calorie; soft drinks – low calorie; semi-skimmed milk; whole milk; skimmed milk; fruit juice, 1% fat milk and other milk and cream. Additionally, we examined absolute and relative changes in percentage energy from free sugar in (1) food and soft drinks and (2) soft drinks alone. While examination of changes in sugar consumption and percentage energy from sugar across the whole diet (food and drink) captures overall substitutions with other sugar-containing products following the UK SDIL, examination of sugar consumption from soft drinks alone provides a higher level of specificity to the SDIL.
Protein intake was selected as a non-equivalent dependent control. It was not a nutritional component specifically targeted by the intervention or other government interventions and therefore is unlikely to be affected by the SDIL but could still be affected by confounding factors such as increases in food prices 20 (see online supplemental text 4 ).
Statistical analysis
Controlled interrupted time series (ITS) analyses were performed to examine changes in the outcomes in relation to the UK SDIL separately in adults and children. We analysed data at the quarterly level over 11 years with the first data point representing dates from April to June 2008 and the last representing dates from January to March 2019. Model specifications are shown in online supplemental text 5 . Where diary date entries extended over two quarters, the earlier quarter was designated as the time point for analysis. Generalised least squares models were used. Autocorrelation in the time series was determined using Durbin–Watson tests and from visualisations of autocorrelation and partial correlation plots. Autocorrelation-moving average correlation structure with order (p) and moving average (q) parameters were used and selected to minimise the Akaike information criterion in each model. Trends in free sugar consumption prior to the announcement of SDIL in April 2016 were used to estimate counterfactual scenarios of what would have happened if the SDIL had not been announced or come into force. Thus, the interruption point was the 3-month period beginning April 2016. Absolute and relative differences in consumption of free sugars/person/day were estimated by calculating the difference between the observed and counterfactual values at quarterly time point 45. To account for non-response and to ensure the sample distribution represented the UK distribution of females and males and age profile, weights provided by NDNS were used and adapted for analysis of adults and children separately. 21 A study protocol has been published 22 and the study is registered ( ISRCTN18042742 ). For changes to the original protocol see online supplemental text 6 . All statistical analyses were performed in R version 4.1.0.
Data from 7999 adults and 7656 children were included across 11 years representing approximately 40 children and 40 adults each month. Table 1 gives descriptive values for the outcomes of interest. Compared with the pre-announcement period, free sugars consumed from all soft drinks reduced by around one-half in children and one-third in adults in the post-announcement period. Total dietary free sugar consumption and percentage of total dietary energy derived from free sugars also declined. Mean protein consumption was relatively stable over both periods in children and adults. The age and sex of the children and adults were very similar in the pre- and post-announcement periods.
- View inline
Mean amount of free sugar (g) consumed in children and adults per day during the study period before and after the announcement of the soft drinks industry levy (SDIL)
All estimates of change in free sugar consumption referred to below are based on g/individual/day in the 3-month period beginning January 2019 and compared with the counterfactual scenario of no UK SDIL announcement and implementation.
Change in free sugar consumption (soft drinks only)
In children, consumption of free sugars from soft drinks was approximately 27 g/day at the start of the study period but fell steeply throughout. By the end of the study period mean sugar consumption from soft drinks was approximately 10 g/day ( figure 1 ). Overall, relative to the counterfactual scenario, there was an absolute reduction in daily free sugar consumption from soft drinks of 3.0 g (95% CI 0.1 to 5.8) or a relative reduction of 23.5% (95% CI 46.0% to 0.9%) in children ( table 2 ). In adults, free sugar consumption at the beginning of the study was lower than that of children (approximately 17 g/day) and was declining prior to the SDIL announcement, although less steeply ( figure 1 ). Following the SDIL announcement, free sugar consumption from soft drinks appeared to decline even more steeply. There was an absolute reduction in free sugar consumption from soft drinks of 5.2 g (95% CI 4.2 to 6.1) or a relative reduction of 40.4% (95% CI 32.9% to 48.0%) in adults relative to the counterfactual ( figure 1 , table 2 ).
- Download figure
- Open in new tab
- Download powerpoint
Observed and modelled daily consumption (g) of free sugar from drink products per adult/child from April 2008 to March 2019. Red points show observed data and solid red lines (with light red shadows) show modelled data (and 95% CIs) of free sugar consumed from drinks. The dashed red line indicates the counterfactual line based on pre-announcement trends and if the announcement and implementation had not happened. Modelled protein consumption from drinks (control group) was removed from the graph to include resolution but is available in the supplementary section. The first and second dashed lines indicate the announcement and implementation of the soft drinks industry levy (SDIL), respectively.
Change in free sugar consumption in food and drink and energy from free sugar as a proportion of total energy compared with the counterfactual scenario of no announcement and implementation of the UK soft drinks industry levy (SDIL)
Change in total dietary free sugar consumption (food and soft drinks combined)
Consumption of total dietary free sugars in children was approximately 70 g/day at the beginning of the study but this fell to approximately 45 g/day by the end of the study ( figure 2 ). Relative to the counterfactual scenario, there was an absolute reduction in total dietary free sugar consumption of 4.8 g (95% CI 0.6 to 9.1) or relative reduction of 9.7% (95% CI 18.2% to 1.2%) in children ( figure 2 ; table 2 ). In adults, consumption of total dietary free sugar consumption at the beginning of the study was approximately 60 g/day falling to approximately 45 g/day by the end of the study ( figure 2 ). Relative to the counterfactual scenario there was an absolute reduction in total dietary free sugar consumption in adults of 10.9 g (95% CI 7.8 to 13.9) or a relative reduction of 19.8% (95% CI 25.4% to 14.2%). Online supplemental figures show that, relative to the counterfactual, dietary protein consumption and energy from protein was more or less stable across the study period (see online supplemental figures S3–S6 ).
Observed and modelled daily consumption (g) of free sugar from food and drink products per adult/child from April 2008 to March 2019. Red points show observed data and solid red lines (with light red shadows) show modelled data (and 95% CIs) of free sugar consumed from food and drinks. The dashed red line indicates the counterfactual line based on pre-announcement trends and if the announcement and implementation had not happened. Modelled protein consumption from food and drinks (control group) was removed from the graph to include resolution but is available in the supplementary section. The first and second dashed lines indicate the announcement and implementation of the soft drinks industry levy (SDIL), respectively.
Change in energy from free sugar as a proportion of total energy
The percentage of energy from total dietary free sugar decreased across the study period but did not change significantly relative to the counterfactual scenario in children or adults, with relative changes in free sugar consumption of −7.6 g (95% CI −41.7 to 26.5) and −24.3 g (95% CI −54.0 to 5.4), respectively (see online supplemental figure S1 and table 2 ). Energy from free sugar in soft drinks as a proportion of total energy from soft drinks also decreased across the study period but did not change significantly relative to the counterfactual (see online supplemental figure S2 ).
Summary of main findings
This study is the first to examine individual level consumption of free sugars in the total diet (and in soft drinks only) in relation to the UK SDIL. Using nationally representative population samples, we found that approximately 1 year following the UK SDIL came into force there was a reduction in total dietary free sugar consumed by children and adults compared with what would have been expected if the SDIL had not been announced and implemented. In children this was equivalent to a reduction of 4.8 g of free sugars/day from food and soft drinks, of which 3 g/day came from soft drinks alone, suggesting that the reduction of sugar in the diet was primarily due to a reduction of sugar from soft drinks. In adults, reductions in dietary sugar appeared to come equally from food and drink with an 11 g reduction in food and drink combined, of which 5.2 g was from soft drinks only. There was no significant reduction compared with the counterfactual in the percentage of energy intake from free sugars in the total diet or from soft drinks alone in both children and adults, suggesting that energy intake from free sugar was reducing simultaneously with overall total energy intake.
Comparison with other studies and interpretation of results
Our finding of a reduction in consumption of free sugars from soft drinks after accounting for pre-SDIL announcement trends is supported by previous research showing a large reduction in the proportion of available soft drinks with over 5 g of sugar/100 mL, the threshold at which soft drinks become levy liable. 15 Furthermore, efforts of the soft drink industry to reformulate soft drinks were found to have led to significant reductions in the volume and per capita sales of sugar from these soft drinks. 23
Our findings are consistent with recent research showing reductions in purchasing of sugar from soft drinks of approximately 8 g/household/week (equivalent to approximately 3 g/person/week or approximately 0.5 g/person/day) 1 year after the SDIL came into force. 16 The estimates from the current study suggest larger reductions in consumption (eg, 3 g free sugar/day from soft drinks in children) than previously reported for purchasing. Methodological differences may explain these differences in estimated effect sizes. Most importantly, the previous study used data on soft drink purchases that were for consumption in the home only. In contrast, we captured information on consumption (rather than purchasing) in and out of the home. Consumption of food and particularly soft drinks outside of the home in young people (1–21 years) increases with age and makes a substantial contribution to total free sugar intakes, highlighting the importance of recording both in home and out of home sugar consumption. 4 Purchasing and consumption data also treat waste differently; purchase data record what comes into the home and therefore include waste, whereas consumption data specifically aim to capture leftovers and waste and exclude it from consumption estimates. While both studies use weights to make the population samples representative of the UK, there may be differences in the study participant characteristics in the two studies, which may contribute to the different estimates.
Consistent with other studies, 24 we found that across the 11-year study period we observed a downward trend in free sugar and energy intake in adults and children. 3 A decline in consumption of free sugars was observed in the whole diet rather than just soft drinks, suggesting that consumption of free sugar from food was also declining from as early as 2008. One reason might be the steady transition from sugar in the diet to low-calorie artificial sweeteners, which globally have had an annual growth of approximately 5.1% between 2008 and 2015. 25
Public health signalling around the time of the announcement of the levy may also have contributed to the changes we observed. Public acceptability and perceived effectiveness of the SDIL was reported to be high 4 months before and approximately 20 months after the levy came into force. 26 Furthermore, awareness of the SDIL was found to be high among parents of children living in the UK, with most supporting the levy and intending to reduce purchases of SSBs as a result. 27 Health signalling was also found following the implementation of the SSB tax in Mexico, with one study reporting that most adults (65%) were aware of the tax and that those aware of the tax were more likely to think the tax would reduce purchases of SSBs, 28 although a separate study found that adolescents in Mexico were mostly unaware of the tax, 29 suggesting that public health signalling may differ according to age.
In 2016 the UK government announced a voluntary sugar reduction programme as part of its childhood obesity plan (which also included SDIL) with the aim of reducing sugar sold by industry by 5% no later than 2018 and by 20% in time for 2020 through both reformulation and portion size reduction. 30 While the programme only managed to achieve overall sugar reductions of approximately 3.5%, this did include higher reductions in specific products such as yoghurts (−17%) and cereals (−13%) by 2018 which may have contributed to some of the observed reductions in total sugar consumption (particularly from foods) around the time of the SDIL. While there is strong evidence that the UK SDIL led to significant reformulation 15 and reductions in purchases of sugar from soft drinks, 16 the products targeted by the sugar reduction programme were voluntary with no taxes or penalties if targets were not met, possibly leading to less incentive for manufacturers to reformulate products that were high in sugar. The 5-year duration of the voluntary sugar reduction programme also makes it challenging to attribute overall reductions using interruption points that we assigned to the ITS to align with the date of the SDIL announcement. The soft drinks categories in our study included levy liable and non-levy liable drinks because we wanted to examine whether individuals were likely to substitute levy liable drinks for high sugar non-liable options. The decline in sugar consumed overall and in soft drinks in relation to the levy suggests that individuals did not change their diets substantially by substituting more sugary foods and drinks. This is consistent with findings from a previous study that found no changes in relation to the levy in sugar purchased from fruit juice, powder used to make drinks or confectionery. 16
Consistent with previous analyses, 3 our findings showed that there was a downward trend in energy intake from sugar as a proportion of total energy across the duration of the study. While there was no reduction compared with the counterfactual scenario (which was also decreasing), our estimates suggest that, by 2019, on average energy from sugar as a proportion of all energy appears to be in line with the WHO recommendation of 10% but not the more recent guidelines of 5% which may bring additional health benefits. 1 31 This finding may suggest that reductions in energy intake from sugar were reducing in concert with overall energy intake and indeed may have been driving it. However, the magnitude of calories associated with the reduction in free sugars, compared with the counterfactual scenario in both adults and children, was modest and thus potentially too small to reflect significant changes in the percentage of energy from sugar. In children, a daily reduction of 4.8 g sugar equates to approximately 19.2 kilocalories out of an approximate daily intake of approximately 2000 kilocalories which is equivalent to approximately 1% reduction in energy intake. Furthermore, overall measures of dietary energy are also likely to involve a degree of error reducing the level of precision in any estimates.
Our estimates of changes in sugar consumption in relation to SDIL suggest that adults may have experienced a greater absolute reduction in sugar than children, which is not consistent with estimates of the distributional impact of the policy. 32 However, our understanding may be aided by the visualisations afforded by graphical depictions of our ITS graphs. Children’s consumption of sugar at the beginning of the study period, particularly in soft drinks, was higher than in adults but reducing at a steeper trajectory, which will have influenced our estimated counterfactual scenario of what would have happened without the SDIL. This steep downward trajectory could not have continued indefinitely as there is a lower limit for sugar consumption. No account for this potential ‘floor effect’ was made in the counterfactual. Adults had a lower baseline of sugar consumption, but their trajectory of sugar consumption decreased at a gentler trajectory, potentially allowing more scope for improvement over the longer run.
Reductions in the levels of sugar in food and drink may have also impacted different age groups and children and adults differently. For example, the largest single contributor to free sugars in younger children aged 4–10 years is cereal and cereal products, followed by soft drinks and fruit juice. By the age of 11–18 years, soft drinks provide the largest single source (29%) of dietary free sugar. For adults the largest source of free sugars is sugar, preserves and confectionery, followed by non-alcoholic beverages. 5
Strengths and limitations
The main strengths of the study include the use of nationally representative data on individual consumption of food and drink in and out of the home using consistent food diary assessment over a 4-day period, setting it apart from other surveys which have used food frequency questionnaires, 24 hour recall, shortened dietary instruments or a mixture of these approaches across different survey years. 33 The continual collection of data using consistent methods enabled us to analyse dietary sugar consumption and energy quarterly over 11 years (or 45 time points) including the announcement and implementation period of the SDIL. Information on participant age allowed us to examine changes in sugar consumption in adults and children separately. Limited sample sizes restricted our use of weekly or monthly data and prevented us from examining differences between sociodemographic groups. At each time point we used protein consumption in food and drink as a non-equivalent control category, strengthening our ability to adjust for time-varying confounders such as contemporaneous events. The trends in counterfactual scenarios of sugar consumption and energy from free sugar as part of total energy were based on trends from April 2008 to the announcement of the UK SDIL (March 2016); however, it is possible that the direction of sugar consumption may have changed course. Ascribing changes in free sugar consumption to the SDIL should include exploration of other possible interventions that might have led to a reduction in sugar across the population. We are only aware of the wider UK government’s voluntary sugar reduction programme implemented across overlapping timelines (2015–2020) and leading to reductions in sugar consumption that were well below the targets set. 30 In turn, under-reporting of portion sizes and high energy foods, which may be increasingly seen as less socially acceptable, has been suggested as a common error in self-reported dietary intake with some groups including older teenagers and females, especially those who are living with obesity, more likely to underestimate energy intake. 34 35 However, there is no evidence to suggest this would have changed as a direct result of the SDIL. 36
Conclusions
Our findings indicate that the UK SDIL led to reductions in consumption of dietary free sugars in adults and children 1 year after it came into force. Energy from free sugar as a proportion of overall energy intake was falling prior to the UK SDIL but did not change in relation to the SDIL, suggesting that a reduction in sugar may have driven a simultaneous reduction in overall energy intake.
Ethics statements
Patient consent for publication.
Not applicable.
Ethics approval
For NDNS 2008–2013, ethical approval was obtained from the Oxfordshire A Research Ethics Committee (Reference number: 07/H0604/113). For NDNS 2014–2017, ethical approval was given from the Cambridge South NRES Committee (Reference number: 13/EE/0016). Participants gave informed consent to participate in the study before taking part.
- World Health Organization
- Scientific Advisory Committee on Nutrition
- Griffith R ,
- O’Connell M ,
- Smith K , et al
- Roberts C ,
- Maplethorpe N , et al
- Tedstone A ,
- Targett V ,
- Mizdrak A , et al
- Rogers NT ,
- Cummins S ,
- Forde H , et al
- Gracner T ,
- Marquez-Padilla F ,
- Hernandez-Cortes D
- Petimar J ,
- Gibson LA ,
- Wolff MS , et al
- Conway DI ,
- Mytton O , et al
- Scarborough P ,
- Adhikari V ,
- Harrington RA , et al
- Mytton OT , et al
- Powell LM ,
- Chriqui JF ,
- Khan T , et al
- Lawman HG ,
- Bleich SN , et al
- Venables MC ,
- Nicholson S , et al
- Lopez Bernal J ,
- Gasparrini A
- Public Health England
- Briggs A , et al
- Marriott BP ,
- Malek AM , et al
- Sylvetsky AC ,
- Penney TL , et al
- Gillison F ,
- Álvarez-Sánchez C ,
- Contento I ,
- Jiménez-Aguilar A , et al
- Ortega-Avila AG ,
- Papadaki A ,
- Briggs ADM ,
- Mytton OT ,
- Kehlbacher A , et al
- Campbell M ,
- Baird J , et al
- Prentice AM ,
- Goldberg GR , et al
- Hebert JR ,
- Pbert L , et al
- Page P , et al
Supplementary materials
Supplementary data.
This web only file has been produced by the BMJ Publishing Group from an electronic file supplied by the author(s) and has not been edited for content.
- Data supplement 1
X @stevencjcummins
Contributors OM, SC, MR, HR, MW and JA conceptualised and acquired funding for the study. NTR carried out statistical analyses. NTR and JA drafted the manuscript. All authors contributed to the article and approved the submitted version.
As the guarantor, NTR had access to the data, controlled the decision to publish and accepts full responsibility for the work and the conduct of the study.
Funding NTR, OM, MW and JA were supported by the Medical Research Council (grant Nos MC_UU_00006/7). This project was funded by the NIHR Public Health Research programme (grant nos 16/49/01 and 16/130/01) to MW. The views expressed are those of the authors and not necessarily those of the National Health Service, the NIHR, or the Department of Health and Social Care, UK. The funders had no role in study design, data collection and analysis, decision to publish or preparation of the manuscript.
Competing interests None declared.
Provenance and peer review Not commissioned; externally peer reviewed.
Supplemental material This content has been supplied by the author(s). It has not been vetted by BMJ Publishing Group Limited (BMJ) and may not have been peer-reviewed. Any opinions or recommendations discussed are solely those of the author(s) and are not endorsed by BMJ. BMJ disclaims all liability and responsibility arising from any reliance placed on the content. Where the content includes any translated material, BMJ does not warrant the accuracy and reliability of the translations (including but not limited to local regulations, clinical guidelines, terminology, drug names and drug dosages), and is not responsible for any error and/or omissions arising from translation and adaptation or otherwise.
Read the full text or download the PDF:
- Language Teaching
- English Language Studies
Error Analysis in ESL Writing: A Case Study at Academic English Program at Saint Michael's College, VT, USA

- Institut Parahikma Indonesia
Discover the world's research
- 25+ million members
- 160+ million publication pages
- 2.3+ billion citations

- Muh. Agus Wijaya

- Kadek Heni Oktarina Wisudayanti

- Shamimah Mohideen
- Assis Lecturer

- Chittima Kaweera

- Poopak Bahmani
- Farahman Farrokhi

- Keith Folse
- Hsiao-ping Wu
- Esther V. Garza
- Elizabeth B. Bernhardt
- Pooneh Heydari
- Mohammad S Bagheri
- Recruit researchers
- Join for free
- Login Email Tip: Most researchers use their institutional email address as their ResearchGate login Password Forgot password? Keep me logged in Log in or Continue with Google Welcome back! Please log in. Email · Hint Tip: Most researchers use their institutional email address as their ResearchGate login Password Forgot password? Keep me logged in Log in or Continue with Google No account? Sign up
This paper is in the following e-collection/theme issue:
Published on 14.8.2024 in Vol 26 (2024)
Cancer Prevention and Treatment on Chinese Social Media: Machine Learning–Based Content Analysis Study
Authors of this article:

Original Paper
- Keyang Zhao 1 * , DPhil ;
- Xiaojing Li 1, 2 * , Prof Dr ;
- Jingyang Li 3 , DPhil
1 School of Media & Communication, Shanghai Jiao Tong University, Shanghai, China
2 Institute of Psychology and Behavioral Science, Shanghai Jiao Tong University, Shanghai, China
3 School of Software, Shanghai Jiao Tong University, Shanghai, China
*these authors contributed equally
Corresponding Author:
Xiaojing Li, Prof Dr
School of Media & Communication
Shanghai Jiao Tong University
800 Dongchuan Rd.
Minhang District
Shanghai, 200240
Phone: 86 13918611103
Fax:86 21 34207088
Email: [email protected]
Background: Nowadays, social media plays a crucial role in disseminating information about cancer prevention and treatment. A growing body of research has focused on assessing access and communication effects of cancer information on social media. However, there remains a limited understanding of the comprehensive presentation of cancer prevention and treatment methods across social media platforms. Furthermore, research comparing the differences between medical social media (MSM) and common social media (CSM) is also lacking.
Objective: Using big data analytics, this study aims to comprehensively map the characteristics of cancer treatment and prevention information on MSM and CSM. This approach promises to enhance cancer coverage and assist patients in making informed treatment decisions.
Methods: We collected all posts (N=60,843) from 4 medical WeChat official accounts (accounts with professional medical backgrounds, classified as MSM in this paper) and 5 health and lifestyle WeChat official accounts (accounts with nonprofessional medical backgrounds, classified as CSM in this paper). We applied latent Dirichlet allocation topic modeling to extract cancer-related posts (N=8427) and identified 6 cancer themes separately in CSM and MSM. After manually labeling posts according to our codebook, we used a neural-based method for automated labeling. Specifically, we framed our task as a multilabel task and utilized different pretrained models, such as Bidirectional Encoder Representations from Transformers (BERT) and Global Vectors for Word Representation (GloVe), to learn document-level semantic representations for labeling.
Results: We analyzed a total of 4479 articles from MSM and 3948 articles from CSM related to cancer. Among these, 35.52% (2993/8427) contained prevention information and 44.43% (3744/8427) contained treatment information. Themes in CSM were predominantly related to lifestyle, whereas MSM focused more on medical aspects. The most frequently mentioned prevention measures were early screening and testing, healthy diet, and physical exercise. MSM mentioned vaccinations for cancer prevention more frequently compared with CSM. Both types of media provided limited coverage of radiation prevention (including sun protection) and breastfeeding. The most mentioned treatment measures were surgery, chemotherapy, and radiotherapy. Compared with MSM (1137/8427, 13.49%), CSM (2993/8427, 35.52%) focused more on prevention.
Conclusions: The information about cancer prevention and treatment on social media revealed a lack of balance. The focus was primarily limited to a few aspects, indicating a need for broader coverage of prevention measures and treatments in social media. Additionally, the study’s findings underscored the potential of applying machine learning to content analysis as a promising research approach for mapping key dimensions of cancer information on social media. These findings hold methodological and practical significance for future studies and health promotion.
Introduction
In 2020, 4.57 million new cancer cases were reported in China, accounting for 23.7% of the world’s total [ 1 ]. Many of these cancers, however, can be prevented [ 2 , 3 ]. According to the World Health Organization (WHO), 30%-50% of cancers could be avoided through early detection and by reducing exposure to known lifestyle and environmental risks [ 4 ]. This underscores the imperative to advance education on cancer prevention and treatment.
Mass media serves not only as a primary channel for disseminating cancer information but also as a potent force in shaping the public health agenda [ 5 , 6 ]. Previous studies have underscored the necessity of understanding how specific cancer-related content is presented in the media. For example, the specific cancer types frequently mentioned in news reports have the potential to influence the public’s perception of the actual incidence of cancer [ 7 ].
Nowadays, social media plays an essential role in disseminating health information, coordinating resources, and promoting health campaigns aimed at educating individuals about prevention measures [ 8 ]. Additionally, it influences patients’ decision-making processes regarding treatment [ 9 ]. A study revealed that social media use correlates with increased awareness of cancer screening in the general population [ 10 ]. In recent years, there has been a notable surge in studies evaluating cancer-related content on social media. However, previous studies often focused on specific cancer types [ 11 ] and limited aspects of cancer-related issues [ 12 ]. The most recent comprehensive systematic content analysis of cancer coverage, conducted in 2013, indicated that cancer news coverage has heavily focused on treatment, while devoting very little attention to prevention, detection, or coping [ 13 ].
Evaluating cancer prevention information on social media is crucial for future efforts by health educators and cancer control organizations. Moreover, providing reliable medical information to individuals helps alleviate feelings of fear and uncertainty [ 14 ]. Specifically, patients often seek information online when making critical treatment decisions, such as chemotherapy [ 15 ]. Therefore, it is significant to comprehensively evaluate the types of treatment information available on social media.
Although many studies have explored cancer-related posts from the perspectives of patients with cancer [ 16 ] and caregivers [ 17 ], the analysis of posts from medical professionals has been found to be inadequate [ 18 ]. This paradox arises from the expectation that medical professionals, given their professional advantages, should take the lead in providing cancer education on social media. Nevertheless, a significant number of studies have highlighted the prevalence of unreliable medical information on social media [ 19 ]. A Japanese study highlighted a concerning phenomenon: despite efforts by medical professionals to promote cancer screening online, a significant number of antiscreening activists disseminated contradictory messages on the internet, potentially undermining the effectiveness of cancer education initiatives [ 20 ]. Hence, there is an urgent need for the accurate dissemination of health information on social media, with greater involvement from scientists or professional institutions, to combat the spread of misinformation [ 21 ]. Despite efforts to study professional medical websites [ 22 ] and apps [ 23 ], there remains a lack of comprehensive understanding of the content posted on medical social media (MSM). Further study is thus needed to compare the differences between cancer information on social media from professional medical sources and nonprofessional sources to enhance cancer education.
For this study, we defined social media as internet-based platforms characterized by social interactive functions such as reading, commenting, retweeting, and timely interaction [ 24 ]. Based on this definition, we further classified 2 types of media based on ownership, content, and contributors: common social media (CSM) and MSM. MSM refers to social media platforms owned by professional medical institutions or organizations. It primarily provides medical and health information by medical professionals, including medical-focused accounts on social media and mobile health apps. CSM refers to social media owned or managed by individuals without medical backgrounds. It mainly provides health and lifestyle content.
Similar to Facebook (Meta Platforms, Inc.), WeChat (Tencent Holdings Limited) is the most popular social media platform in China, installed on more than 90% of smartphones. Zhang et al [ 25 ] has indicated that 63.26% of people prefer to obtain health information from WeChat. Unlike other Chinese social media platforms, WeChat has a broader user base that spans various age groups [ 26 ]. WeChat Public Accounts (WPAs) operate within the WeChat platform, offering services and information to the public. Many hospitals and primary care institutions in China have increasingly registered WPAs to provide health care services, medical information, health education, and more [ 27 ]. Therefore, this study selected WPA as the focus of research.
Based on big data analytics, this study aims to comprehensively map the characteristics of cancer treatment and prevention information on MSM and CSM, which could significantly enhance cancer coverage and assist patients in treatment decision-making. To address the aforementioned research gaps, 2 research questions were formulated.
- Research question 1: What are the characteristics of cancer prevention information discussed on social media? What are the differences between MSM and CSM?
- Research question 2: What are the characteristics of cancer treatment information discussed on social media? What are the differences between MSM and CSM?
Data Collection and Processing
We selected representative WPAs based on the reports from the “Ranking of Influential Health WeChat Public Accounts” [ 28 ] and the “2021 National Rankings of Best Hospitals by Specialty” [ 29 ]. In this study, we focused on 4 medical WPAs within MSM: Doctor Dingxiang (丁香医生), 91Huayi (华医网), The Cancer Hospital of Chinese Academy of Medical Sciences (中国医学科学院肿瘤医院), and Fudan University Shanghai Cancer Center (复旦大学附属肿瘤医院). We also selected 5 health and lifestyle WeChat Official Accounts classified as CSM for this study: Health Times (健康时报), Family Doctor (家庭医生), CCTV Lifestyle (CCTV 生活圈), Road to Health (健康之路), and Life Times (生命时报).
We implemented a Python-based (Python Foundation) crawler to retrieve posts from the aforementioned WPAs. Subsequently, we implemented a filtration process to eliminate noisy and unreliable data. Note that our focus is on WPAs that provide substantial information, defined as containing no fewer than a certain number of characters. We have deleted documents that contain less than 100 Chinese characters. Furthermore, we have removed figures and videos from the remaining documents. Eventually, we conducted an analysis at the paragraph level. According to our findings from random sampling, noise in articles from WPAs mostly originates from advertisements, which are typically found in specific paragraphs. Therefore, we retained only paragraphs that did not contain advertising keywords. In total, we collected 60,843 posts from these WPAs, comprising 20,654 articles from MSM and 40,189 articles from CSM.
The workflow chart in Figure 1 depicts all procedures following data collection and preprocessing. After obtaining meaningful raw documents, we performed word-level segmentation on the texts. We then removed insignificant stopwords and replaced specific types of cancers with a general term to facilitate coarse-grained latent Dirichlet allocation (LDA)–based filtering. Subsequently, we conducted fine-grained LDA topic modeling on the filtered documents without replacing keywords to visualize the topics extracted from the WPAs. Furthermore, we utilized a manually labeled codebook to train a long short-term memory (LSTM) network for document classification into various categories. Finally, we performed data analysis using both the topic distribution derived from fine-grained LDA and the classified documents.

Latent Dirichlet Allocation Topic Modeling
LDA is a generative statistical model that explains sets of observations by latent groups, revealing why some parts of the data are similar [ 30 ]. The LDA algorithm can speculate on the topic distribution of a document.
When comparing LDA with other natural language processing methods such as LSTM-based deep learning, it is worth noting that LDA stands out as an unsupervised learning algorithm. Unlike its counterparts, LDA has the ability to uncover hidden topics without relying on labeled training data. Its strength lies in its capability to automatically identify latent topics within documents by analyzing statistical patterns of word co-occurrences. In addition, LDA provides interpretable outcomes by assigning a probability distribution to each document, representing its association with various topics. Similarly, it assigns a probability distribution to each topic, indicating the prevalence of specific words within that topic. This feature enables researchers to understand the principal themes present in their corpus and the extent to which these themes are manifested in individual documents.
The foundational principle of LDA involves using probabilistic inference to estimate the distribution of topics and word allocations. Specifically, LDA assumes that each document is composed of a mixture of a small number of topics, and each word’s presence can be attributed to one of these topics. This approach allows for overlapping content among documents, rather than strict categorization into separate groups. For a deeper understanding of the technical and theoretical aspects of the LDA algorithm, readers are encouraged to refer to the research conducted by Blei et al [ 30 ]. In this context, our primary focus was on the application of the algorithm to our corpus, and the procedure is outlined in the following sections.
Document Selection
Initially, document selection involves using a methodological approach to sample documents from the corpus, which may include random selection or be guided by predetermined criteria such as document relevance or popularity within the social media context.
Topic Inference
Utilizing LDA or a similar topic modeling technique, we infer the underlying topical structure within each document. This involves modeling documents as mixtures of latent topics represented by a Dirichlet distribution, from which topic proportions are sampled.
Topic Assignment to Words
After determining topic proportions, we proceed to assign topics to individual words in the document. Using a multinomial distribution, each word is probabilistically associated with one of the inferred topics based on the previously derived topic proportions.
Word Distribution Estimation
Each topic is characterized by a distinct distribution over the vocabulary, representing the likelihood of observing specific words within that topic. Using a Dirichlet distribution, we estimate the word distribution for each inferred topic.
Word Generation
Finally, using the multinomial distribution again, we generate words for the document by sampling from the estimated word distribution corresponding to the topic assigned to each word. This iterative process produces synthetic text that mirrors the statistical properties of the original corpus.
To filter out noncancer-related documents in our case, we replaced cancer-related words with “癌症” (cancer or tumor in Chinese) in all documents. We then conducted an LDA analysis to compute the topic distribution of each document and retained documents related to topics where “癌症” appears among the top 10 words.
In our study, we used Python packages such as jieba and gensim for document segmentation and extracting per-topic-per-word probabilities from the model. During segmentation, we applied a stopword dictionary to filter out meaningless words and transformed each document into a cleaned version containing only meaningful words.
During the LDA analysis, to determine the optimal number of topics, our main goal was to compute the topic coherence for various numbers of topics and select the model that yielded the highest coherence score. Coherence measures the interpretability of each topic by assessing whether the words within the same topic are logically associated with each other. The higher the score for a specific number k , the more closely related the words are within that topic. In this phase, we used the Python package pyLDAvis to compare coherence scores with different numbers of topics. Subsequently, we filtered and retained only the documents related to cancer topics, resulting in 4479 articles from MSM and 3948 articles from CSM.
Among the filtered articles, we conducted another LDA analysis to extract topics from the original articles without replacing cancer-related words. Using pyLDAvis, we calculated the coherence score and identified 6 topics for both MSM and CSM articles.
To visualize the topic modeling results, we created bar graphs where the y-axis indicates the top 10 keywords associated with each topic, and the x-axis represents the weight of each keyword (indicating its contribution to the topic). At the bottom of each graph ( Figures 2 and 3 ), we generalized and presented the name of each topic based on the top 10 most relevant keywords.

Manual Content Analysis: Coding Procedure
Based on the codebook, 2 independent coders (KZ and JL) engaged in discussions regarding the coding rules to ensure a shared understanding of the conceptual and operational distinctions among the coding items. To ensure the reliability of the coding process, both coders independently coded 100 randomly selected articles. Upon completion of the pilot coding, any disagreements were resolved through discussion between the 2 coders.
For the subsequent coding phase, each coder was assigned an equitable proportion of articles, with 10% of the cancer-related articles randomly sampled from both MSM samples (450/4479) and CSM samples (394/3948). Manual coding was performed on a total of 844 articles, which served as the training data set for the machine learning model. The operational definitions of each coding variable are detailed in Multimedia Appendix 1 .
Coding Measures
Cancer prevention measures.
Coders identified whether an article mentioned any of the following cancer prevention measures [ 31 - 35 ]: (1) avoid tobacco use, (2) maintain a healthy weight, (3) healthy diet, (4) exercise regularly, (5) limit alcohol use, (6) get vaccinated, (7) reduce exposure to ultraviolet radiation and ionizing radiation, (8) avoid urban air pollution and indoor smoke from household use of solid fuels, (9) early screening and detection, (10) breastfeeding, (11) controlling chronic infections, and (12) other prevention measures.
Cancer Treatment Measures
Coders identified whether an article mentioned any of the following treatments [ 36 ]: (1) surgery (including cryotherapy, lasers, hyperthermia, photodynamic therapy, cuts with scalpels), (2) radiotherapy, (3) chemotherapy, (4) immunotherapy, (5) targeted therapy, (6) hormone therapy, (7) stem cell transplant, (8) precision medicine, (9) cancer biomarker testing, and (10) other treatment measures.
Neural-Based Machine Learning
In this part, we attempted to label each article using a neural network. As mentioned earlier, we manually labeled 450 MSM articles and 394 CSM articles. We divided the labeled data into a training set and a test set with a ratio of 4:1. We adopted the pretrained Bidirectional Encoder Representations from Transformers (BERT) model. As BERT can only accept inputs with fewer than 512 tokens [ 37 ], we segmented each document into pieces of 510 tokens (accounting for BERT’s automatic [CLS] and [SEP] tokens, where [CLS] denotes the start of a sentence or a document, and [SEP] denotes the end of a sentence or a document) with an overlap of 384 tokens between adjacent pieces. We began by utilizing a BERT-based encoder to encode each piece and predict its labels using a multioutput decoder. After predicting labels for each piece, we pooled the outputs for all pieces within the same document and used an LSTM network to predict final labels for each document.
Ethical Considerations
This study did not require institutional research board review as it did not involve interactions with humans or other living entities, private or personally identifiable information, or any pharmaceuticals or medical devices. The data set consists solely of publicly available social media posts.
Cancer Topics on Social Media
Applying LDA, we identified 6 topics each for MSM and CSM articles. The distribution of topics among MSM and CSM is presented in Table 1 , while the keyword weights for each topic are illustrated in Figures 2 and 3 .
Media type and topic number | Topic description | Articles, n (%) | Top 10 keywords | ||||||
Topic 1 | Liver cancer and stomach cancer | 1519 (18.03) | Cancer (癌症), liver cancer (肝癌), stomach cancer (胃癌), factors (因素), food (食物), disease (疾病), (幽门), exercise (运动), patient (患者), and diet (饮食) | ||||||
Topic 2 | Female and cancer | 1611 (19.12) | Breast cancer (乳腺癌), female (女性), patient (患者), lung cancer (肺癌), surgery (手术), tumor (肿瘤), mammary gland (乳腺), expert (专家), ovarian cancer (卵巢癌), and lump (结节) | ||||||
Topic 3 | Breast cancer | 1093 (12.97) | Breast cancer (乳腺癌), surgery (手术), thyroid (甲状腺), lump (结节), breast (乳房), patient (患者), female (女性), screening and testing (检查), mammary gland (乳腺), and tumor (肿瘤) | ||||||
Topic 4 | Cervical cancer | 1019 (12.09) | Vaccine (疫苗), cervical cancer (宫颈癌), virus (病毒), cervix (宫颈), patient (患者), nation (国家), female (女性), nasopharynx cancer (鼻咽癌), medicine (药品), and hospital (医院) | ||||||
Topic 5 | Clinical cancer treatment | 2548 (30.24) | Tumor (肿瘤), patient (患者), screening (检查), chemotherapy (化疗), clinic (临床), symptom (症状), hospital (医院), surgery (手术), medicine (药物), and disease (疾病) | ||||||
Topic 6 | Diet and cancer risk | 1741 (20.66) | Patient (患者), tumor (肿瘤), food (食物), polyp (息肉), professor (教授), nutrition (营养), expert (专家), surgery (手术), cancer (癌症), and disease (疾病) | ||||||
Topic 1 | Cancer-causing substances | 1136 (13.48) | Foods (食物), nutrition (营养), carcinogen (致癌物), food (食品), ingredient (含量), vegetable (蔬菜), cancer (癌症), body (人体), lump (结节), and formaldehyde (甲醛) | ||||||
Topic 2 | Cancer treatment | 1319 (15.65) | Patient (患者), cancer (癌症), hospital (医院), lung cancer (肺癌), tumor (肿瘤), medicine (药物), disease (疾病), professor (教授), surgery (手术), and clinic (临床) | ||||||
Topic 3 | Female and cancer risk | 1599 (18.97) | Screening and testing (检查), female (女性), disease (疾病), breast cancer (乳腺癌), cancer (癌症), lung cancer (肺 癌), patient (患者), body (身体), tumor (肿瘤), and risk (风险) | ||||||
Topic 4 | Exercise, diet, and cancer risk | 1947 (23.10) | Cancer (癌症), exercise (运动), food (食物), risk (风险), body (身体), disease (疾病), suggestion (建议), patient (患者), fat (脂肪), and hospital (医院) | ||||||
Topic 5 | Screening and diagnosis of cancer | 1790 (21.24) | Screening and testing (检查), disease (疾病), hospital (医院), stomach cancer (胃癌), symptom (症状), patient (患者), cancer (癌症), liver cancer (肝癌), female (女性), and suggestion (建议) | ||||||
Topic 6 | Disease and body parts | 869 (10.31) | Disease (疾病), intestine (肠道), food (食物), hospital (医院), oral cavity (口腔), patient (患者), teeth (牙齿), cancer (癌症), ovary (卵巢), and garlic (大蒜) |
a In each article, different topics may appear at the same time. Therefore, the total frequency of each topic did not equate to the total number of 8427 articles.
b To ensure the accuracy of the results, directly translating sampled texts from Chinese into English posed challenges due to differences in semantic elements. In English, cancer screening refers to detecting the possibility of cancer before symptoms appear, while diagnostic tests confirm the presence of cancer after symptoms are observed. However, in Chinese, the term “检查” encompasses both meanings. Therefore, we translated it as both screening and testing.

Among MSM articles, topic 5 was the most frequent (2548/8427, 30.24%), followed by topic 6 (1741/8427, 20.66%) and topic 2 (1611/8427, 19.12%). Both topics 5 and 6 focused on clinical treatments, with topic 5 specifically emphasizing cancer diagnosis. The keywords in topic 6, such as “polyp,” “tumor,” and “surgery,” emphasized the risk and diagnosis of precancerous lesions. Topic 2 primarily focused on cancer surgeries related to breast cancer, lung cancer, and ovarian cancer. The results indicate that MSM articles concentrated on specific cancers with higher incidence in China, including stomach cancer, liver cancer, lung cancer, breast cancer, and cervical cancer [ 10 ].
On CSM, topic 4 (1947/8427, 23.10%) had the highest proportion, followed by topic 5 (1790/8427, 21.24%) and topic 3 (1599/8427, 18.97%). Topic 6 had the smallest proportion. Topics 1 and 4 were related to lifestyle. Topic 1 particularly focused on cancer-causing substances, with keywords such as “food,” “nutrition,” and “carcinogen” appearing most frequently. Topic 4 was centered around exercise, diet, and their impact on cancer risk. Topics 3 and 5 were oriented toward cancer screening and diagnosis. Topic 3 specifically focused on female-related cancers, with discussions prominently featuring breast cancer screening and testing. Topic 5 emphasized early detection and diagnosis of stomach and lung cancers, highlighting keywords such as “screening” and “symptom.”
Cancer Prevention Information
Our experiment on the test set showed that the machine learning model achieved F 1 -scores above 85 for both prevention and treatment categories in both MSM and CSM. For subclasses within prevention and treatment, we achieved F 1 -scores of at least 70 for dense categories (with an occurrence rate >10%, ie, occurs in >1 of 10 entries) and at least 50 for sparse categories (with an occurrence rate <10%, ie, occurs in <1 of 10 entries). Subsequently, we removed items labeled as “other prevention measures” and “other treatment measures” due to semantic ambiguity.
Table 2 presents the distribution of cancer prevention information across MSM (n=4479) and CSM (n=3948).
Type of cancer prevention measures | Number of articles on MSM (n=4479), n (%) | Number of articles on CSM (n=3948), n (%) |
Articles containing prevention information | 1137 (25.39) | 1856 (47.01) |
Early screening and testing | 737 (16.45) | 1085 (27.48) |
Healthy diet | 278 (6.21) | 598 (15.15) |
Get vaccinated | 261 (5.83) | 113 (2.86) |
Avoid tobacco use | 186 (4.15) | 368 (9.32) |
Exercise regularly | 135 (3.01) | 661 (16.74) |
Limit alcohol use | 128 (2.86) | 281 (7.12) |
Avoid urban air pollution and indoor smoke from household use of solid fuels | 19 (0.42) | 64 (1.62) |
Maintain a healthy weight | 18 (0.40) | 193 (4.89) |
Practice safe sex | 12 (0.27) | 4 (0.10) |
Controlling chronic infections | 3 (0.07) | 32 (0.81) |
Reduce exposure to radiation | 2 (0.04) | 1 (0.03) |
Breastfeeding | 1 (0.02) | 1 (0.03) |
a MSM: medical social media.
b CSM: common social media.
Cancer Prevention Information on MSM
The distribution of cancer prevention information on MSM (n=4479) is as follows: articles discussing prevention measures accounted for 25.39% (1137/4479) of all MSM cancer-related articles. The most frequently mentioned measure was “early screening and testing” (737/4479, 16.45%). The second and third most frequently mentioned prevention measures were “healthy diet” (278/4479, 6.21%) and “get vaccinated” (261/4479, 5.83%). The least mentioned prevention measures were “controlling chronic infections” (3/4479, 0.07%), “reduce exposure to radiation” (2/4479, 0.04%), and “breastfeeding” (1/4479, 0.02%), each appearing in only 1-3 articles.
Cancer Prevention Information on CSM
As many as 1856 out of 3948 (47.01%) articles on CSM referred to cancer prevention information. Among these, “early screening and testing” (1085/3948, 27.48%) was the most commonly mentioned prevention measure. “Exercise regularly” (661/3948, 16.74%) and “healthy diet” (598/3948, 15.15%) were the 2 most frequently mentioned lifestyle-related prevention measures. Additionally, “avoid tobacco use” accounted for 9.32% (368/3948) of mentions. Other lifestyle-related prevention measures were “limit alcohol use” (281/3948, 7.12%) and “maintain a healthy weight” (193/3948, 4.89%). The least mentioned prevention measures were “practice safe sex” (4/3948, 0.10%), “reduce exposure to radiation” (1/3948, 0.03%), and “breastfeeding” (1/3948, 0.03%), each appearing in only 1-4 articles.
Cancer Prevention Information on Social Media
Table 3 presents the overall distribution of cancer prevention information on social media (N=8427). Notably, CSM showed a stronger focus on cancer prevention (1856/3948, 47.01%) compared with MSM (1137/8427, 13.49%). Both platforms highlighted the importance of early screening and testing. However, MSM placed greater emphasis on vaccination as a prevention measure. In addition to lifestyle-related prevention measures, both CSM and MSM showed relatively less emphasis on avoiding exposure to environmental carcinogens, such as air pollution, indoor smoke, and radiation. “Breastfeeding” was the least mentioned prevention measure (2/3948, 0.05%) on both types of social media.
Type of cancer prevention measures | Number of articles on MSM , n (%) | Number of articles on CSM , n (%) | Number of articles overall (N=8427), n (%) |
Articles containing prevention information | 1137 (13.49) | 1856 (22.02) | 2993 (35.52) |
Early screening and testing | 737 (8.75) | 1085 (12.88) | 1822 (21.62) |
Healthy diet | 278 (3.30) | 598 (7.10) | 876 (10.40) |
Get vaccinated | 261 (3.10) | 113 (1.34) | 374 (4.44) |
Avoid tobacco use | 186 (2.21) | 368 (4.37) | 554 (6.57) |
Exercise regularly | 135 (1.60) | 661 (7.84) | 796 (9.45) |
Limit alcohol use | 128 (1.52) | 281 (3.33) | 409 (4.85) |
Avoid urban air pollution and indoor smoke from household use of solid fuels | 19 (0.23) | 64 (0.76) | 83 (0.98) |
Maintain a healthy weight | 18 (0.21) | 193 (2.29) | 211 (2.50) |
Practice safe sex | 12 (0.14) | 4 (0.05) | 16 (0.19) |
Controlling chronic infections | 3 (0.04) | 32 (0.38) | 35 (0.42) |
Reduce exposure to radiation | 2 (0.02) | 1 (0.01) | 3 (0.04) |
Breastfeeding | 1 (0.01) | 1 (0.01) | 2 (0.02) |
Cancer Treatment Information
Table 4 presents the distribution of cancer treatment information on MSM (n=4479) and CSM (n=3948).
Type of cancer treatment measures | Number of articles on MSM (n=4479), n (%) | Number of articles on CSM (n=3948), n (%) |
Articles containing treatment information | 2966 (66.22) | 778 (19.71) |
Surgery | 2045 (45.66) | 419 (10.61) |
Chemotherapy | 1122 (25.05) | 285 (7.22) |
Radiation therapy | 1108 (24.74) | 232 (5.88) |
Cancer biomarker testing | 380 (8.48) | 55 (1.39) |
Targeted therapy | 379 (8.46) | 181 (4.58) |
Immunotherapy | 317 (7.08) | 22 (0.56) |
Hormone therapy | 47 (1.05) | 14 (0.35) |
Stem cell transplantation therapy | 5 (0.11) | 0 (0) |
Cancer Treatment Information on MSM
Cancer treatment information appeared in 66.22% (2966/4479) of MSM posts. “Surgery” was the most frequently mentioned treatment measure (2045/4479, 45.66%), followed by “chemotherapy” (1122/4479, 25.05%) and “radiation therapy” (1108/4479, 24.74%). The proportions of “cancer biomarker testing” (380/4479, 8.48%), “targeted therapy” (379/4479, 8.46%), and “immunotherapy” (317/4479, 7.08%) were comparable. Only a minimal percentage of articles (47/4479, 1.05%) addressed “hormone therapy.” Furthermore, “stem cell transplantation therapy” was mentioned in just 5 out of 4479 (0.11%) articles.
Cancer Treatment Information on CSM
Cancer treatment information accounted for only 19.71% (778/3948) of CSM posts. “Surgery” was the most frequently mentioned treatment measure (419/3948, 10.61%), followed by “chemotherapy” (285/3948, 7.22%) and “radiation therapy” (232/3948, 5.88%). Relatively, the frequency of “targeted therapy” (181/3948, 4.58%) was similar to that of the first 3 types. However, “cancer biomarker testing” (55/3948, 1.39%), “immunotherapy” (22/3948, 0.56%), and “hormone therapy” (14/3948, 0.35%) appeared rarely on CSM. Notably, there were no articles on CSM mentioning stem cell transplantation.
Cancer Treatment Information on Social Media
Table 5 shows the overall distribution of cancer treatment information on social media (N=8427). A total of 44.43% (3744/8427) of articles contained treatment information. MSM (2966/8427, 35.20%) discussed treatment information much more frequently than CSM (778/8427, 9.23%). Furthermore, the frequency of all types of treatment measures mentioned was higher on MSM than on CSM. The 3 most frequently mentioned types of treatment measures were surgery (2464/8427, 29.24%), chemotherapy (1407/8427, 16.70%), and radiation therapy (1340/8427, 15.90%). Relatively, MSM (380/8427, 4.51%) showed a higher focus on cancer biomarker testing compared with CSM (55/8427, 0.65%).
Type of cancer treatment measures | Number of articles on MSM , n (%) | Number of articles on CSM , n (%) | Number of articles overall (N=8427), n (%) |
Articles containing treatment information | 2966 (35.20) | 778 (9.23) | 3744 (44.43) |
Surgery | 2045 (24.27) | 419 (4.97) | 2464 (29.24) |
Radiation therapy | 1108 (13.15) | 232 (2.75) | 1340 (15.90) |
Chemotherapy | 1122 (13.31) | 285 (3.38) | 1407 (16.70) |
Immunotherapy | 317 (3.76) | 22 (0.26) | 339 (4.02) |
Targeted therapy | 379 (4.50) | 181 (2.15) | 560 (6.65) |
Hormone therapy | 47 (0.56) | 14 (0.17) | 61 (0.72) |
Stem cell transplant | 5 (0.06) | 0 (0.00) | 5 (0.06) |
Cancer biomarker testing | 380 (4.51) | 55 (0.65) | 435 (5.16) |
Cancer Topics on MSM and CSM
In MSM, treatment-related topics constituted the largest proportion, featuring keywords related to medical examinations. Conversely, in CSM, the distribution of topics appeared more balanced, with keywords frequently associated with cancer risk and screening. Overall, the distribution of topics on MSM and CSM revealed that CSM placed greater emphasis on lifestyle factors and early screening and testing. Specifically, CSM topics focused more on early cancer screening and addressed cancer types with high incidence rates. By contrast, MSM topics centered more on clinical treatment, medical testing, and the cervical cancer vaccine in cancer prevention. Additionally, MSM focused on types of cancers that are easier to screen and prevent, including liver cancer, stomach cancer, breast cancer, cervical cancer, and colon cancer.
Cancer Prevention Information on MSM and CSM
Through content analysis, it was found that 35.52% (2993/8427) of articles on social media contained prevention information, and 44.43% (3744/8427) contained treatment information. Compared with MSM (1137/8427, 13.49%), CSM (2993/8427, 35.52%) focused more on prevention.
Primary prevention mainly involves adopting healthy behaviors to lower the risk of developing cancer, which has been proven to have long-term effects on cancer prevention. Secondary prevention focuses on inhibiting or reversing carcinogenesis, including early screening and detection, as well as the treatment or removal of precancerous lesions [ 38 ]. Compared with cancer screening and treatment, primary prevention is considered the most cost-effective approach to reducing the cancer burden.
From our results, “early screening and testing” (1822/8427, 21.62%) was the most frequently mentioned prevention measure on both MSM and CSM. According to a cancer study from China, behavioral risk factors were identified as the primary cause of cancer [ 10 ]. However, measures related to primary prevention were not frequently mentioned. Additionally, lifestyle-related measures such as “healthy diet,” “regular exercise,” “avoiding tobacco use,” and “limiting alcohol use” were mentioned much less frequently on MSM compared with CSM.
Furthermore, “avoiding tobacco use” (554/8427, 6.57%) and “limiting alcohol use” (409/8427, 4.85%) were rarely mentioned, despite tobacco and alcohol being the leading causes of cancer. In China, public policies on the production, sale, and consumption of alcohol are weaker compared with Western countries. Notably, traditional Chinese customs often promote the belief that moderate drinking is beneficial for health [ 39 ]. Moreover, studies indicated that the smoking rate among adult men exceeded 50% in 2015. By 2018, 25.6% of Chinese adults aged 18 and above were smokers, totaling approximately 282 million smokers in China (271 million males and 11 million females) [ 40 ]. These statistics align with the consistently high incidence of lung cancer among Chinese men [ 41 ]. Simultaneously, the incidence and mortality of lung cancer in Chinese women were more likely associated with exposure to second-hand smoke or occupation-related risk factors.
Although MSM (261/8427, 3.10%) mentioned vaccination more frequently than CSM (113/8427, 1.34%), vaccination was not widely discussed on social media overall (374/8427, 4.44%). The introduction of human papillomavirus vaccination in China has lagged for more than 10 years compared with Western countries. A bivalent vaccine was approved by the Chinese Food and Drug Administration in 2017 but has not been included in the national immunization schedules up to now [ 42 ].
According to the “European Code Against Cancer” [ 43 ], breastfeeding is recommended as a measure to prevent breast cancer. However, there were no articles mentioning the role of breastfeeding in preventing breast cancer on social media.
One of the least frequently mentioned measures was “radiation protection,” which includes sun protection. Although skin cancer is not as common in China as in Western countries, China has the largest population in the world. A study showed that only 55.2% of Chinese people knew that ultraviolet radiation causes skin cancer [ 33 ]. Additional efforts should be made to enhance public awareness of skin cancer prevention through media campaigns.
Overall, our results indicate that social media, especially MSM, focused more on secondary prevention. The outcomes of primary prevention are challenging to identify in individuals, and studies on cancer education may partly explain why primary prevention was often overlooked [ 44 ].
Cancer Treatment Information on MSM and CSM
Compared with a related content analysis study in the United States, our findings also indicate that the media placed greater emphasis on treatment [ 45 ]. Treatment information on MSM was more diverse than on CSM, with a higher proportion of the 3 most common cancer treatments—surgery, chemotherapy, and radiation therapy—mentioned on MSM compared with CSM. Notably, CSM (232/8427, 2.75%) mentioned radiation therapy less frequently compared with MSM (1108/8427, 13.15%), despite it being one of the most common cancer treatment measures in clinical practice.
In addition to common treatment methods, other approaches such as targeted therapy (560/8427, 6.65%) and immunotherapy (339/8427, 4.02%) were rarely discussed. This could be attributed to the high costs associated with these treatments. A study revealed that each newly diagnosed patient with cancer in China faced out-of-pocket expenses of US $4947, amounting to 57.5% of the family’s annual income, posing an unaffordable economic burden of 77.6% [ 46 ]. In 2017, the Chinese government released the National Health Insurance Coverage (NHIC) policy to improve the accessibility and affordability of innovative anticancer medicines, leading to reduced prices and increased availability and utilization of 15 negotiated drugs. However, a study indicated that the availability of these innovative anticancer drugs remained limited. By 2019, the NHIC policy had benefited 44,600 people, while the number of new cancer cases in China in 2020 was 4.57 million [ 47 ]. The promotion of information on innovative therapies helped patients gain a better understanding of their cancer treatment options [ 48 ].
Practical Implications
This research highlighted that MSM did not fully leverage its professional background in providing comprehensive cancer information to the public. In fact, MSM holds substantial potential for contributing to cancer education. The findings from the content analysis also have practical implications for practitioners. They provide valuable insights for experts to assess the effectiveness of social media, monitor the types of information available to the public and patients with cancer, and guide communication and medical professionals in crafting educational and persuasive messages based on widely covered or less attended content.
Limitations and Future Directions
This study had some limitations. First, we only collected 60,843 articles from 9 WPAs in China. Future research could broaden the scope by collecting data from diverse countries and social media platforms. Second, our manual labeling only extracted 10% (450/4479 for MSM and 394/3948 for CSM) of the samples; the accuracy of the machine learning model could be enhanced by training it with a larger set of labeled articles. Finally, our results only represented the media’s presentation, and the impact of this information on individuals remains unclear. Further work could examine its influence on behavioral intentions or actions related to cancer prevention among the audience.
Conclusions
The analysis of cancer-related information on social media revealed an imbalance between prevention and treatment content. Overall, there was more treatment information than prevention information. Compared with MSM, CSM mentioned more prevention information. On MSM, the proportion of treatment information was greater than prevention information, whereas on CSM, the 2 were equal. The focus on cancer prevention and treatment information was primarily limited to a few aspects, with a predominant emphasis on secondary prevention rather than primary prevention. There is a need for further improvement in the coverage of prevention measures and treatments for cancer on social media. Additionally, the findings underscored the potential of applying machine learning to content analysis as a promising research paradigm for mapping key dimensions of cancer information on social media. These findings offer methodological and practical significance for future studies and health promotion.
Acknowledgments
This study was funded by The Major Program of the Chinese National Foundation of Social Sciences under the project “The Challenge and Governance of Smart Media on News Authenticity” (grant number 23&ZD213).
Conflicts of Interest
None declared.
Definitions and descriptions of coding items.
- International Agency for Research on Cancer (IARC), World Health Organization (WHO). Cancer today: the global cancer observatory. IARC. Geneva, Switzerland. WHO; 2020. URL: https://gco.iarc.who.int/today/en [accessed 2023-12-25]
- Yu S, Yang CS, Li J, You W, Chen J, Cao Y, et al. Cancer prevention research in China. Cancer Prev Res (Phila). Aug 2015;8(8):662-674. [ CrossRef ] [ Medline ]
- Xia C, Dong X, Li H, Cao M, Sun D, He S, et al. Cancer statistics in China and United States, 2022: profiles, trends, and determinants. Chin Med J (Engl). Feb 09, 2022;135(5):584-590. [ FREE Full text ] [ CrossRef ] [ Medline ]
- World Health Organization (WHO). Cancer. WHO. 2023. URL: https://www.who.int/news-room/facts-in-pictures/detail/cancer [accessed 2023-12-27]
- Pagoto S, Waring ME, Xu R. A call for a public health agenda for social media research. J Med Internet Res. Dec 19, 2019;21(12):e16661. [ FREE Full text ] [ CrossRef ] [ Medline ]
- Tekeli-Yesil S, Tanner M. Understanding the contribution of conventional media in earthquake risk communication. J Emerg Manag Disaster Commun. Jun 01, 2024;05(01):111-133. [ CrossRef ]
- Jensen JD, Scherr CL, Brown N, Jones C, Christy K, Hurley RJ. Public estimates of cancer frequency: cancer incidence perceptions mirror distorted media depictions. J Health Commun. 2014;19(5):609-624. [ CrossRef ] [ Medline ]
- Banaye Yazdipour A, Niakan Kalhori SR, Bostan H, Masoorian H, Ataee E, Sajjadi H. Effect of social media interventions on the education and communication among patients with cancer: a systematic review protocol. BMJ Open. Nov 30, 2022;12(11):e066550. [ FREE Full text ] [ CrossRef ] [ Medline ]
- Wallner LP, Martinez KA, Li Y, Jagsi R, Janz NK, Katz SJ, et al. Use of online communication by patients with newly diagnosed breast cancer during the treatment decision process. JAMA Oncol. Dec 01, 2016;2(12):1654-1656. [ FREE Full text ] [ CrossRef ] [ Medline ]
- Sun D, Li H, Cao M, He S, Lei L, Peng J, et al. Cancer burden in China: trends, risk factors and prevention. Cancer Biol Med. Nov 15, 2020;17(4):879-895. [ FREE Full text ] [ CrossRef ] [ Medline ]
- Basch CH, Menafro A, Mongiovi J, Hillyer GC, Basch CE. A content analysis of YouTube videos related to prostate cancer. Am J Mens Health. Jan 2017;11(1):154-157. [ FREE Full text ] [ CrossRef ] [ Medline ]
- Vasconcelos Silva C, Jayasinghe D, Janda M. What can Twitter tell us about skin cancer communication and prevention on social media? Dermatology. 2020;236(2):81-89. [ FREE Full text ] [ CrossRef ] [ Medline ]
- Hurley RJ, Riles JM, Sangalang A. Online cancer news: trends regarding article types, specific cancers, and the cancer continuum. Health Commun. 2014;29(1):41-50. [ CrossRef ] [ Medline ]
- Mishel MH, Germino BB, Lin L, Pruthi RS, Wallen EM, Crandell J, et al. Managing uncertainty about treatment decision making in early stage prostate cancer: a randomized clinical trial. Patient Educ Couns. Dec 2009;77(3):349-359. [ CrossRef ] [ Medline ]
- Brown P, Kwan V, Vallerga M, Obhi HK, Woodhead EL. The use of anecdotal information in a hypothetical lung cancer treatment decision. Health Commun. Jun 2019;34(7):713-719. [ CrossRef ] [ Medline ]
- Crannell WC, Clark E, Jones C, James TA, Moore J. A pattern-matched Twitter analysis of US cancer-patient sentiments. J Surg Res. Dec 2016;206(2):536-542. [ CrossRef ] [ Medline ]
- Gage-Bouchard EA, LaValley S, Mollica M, Beaupin LK. Cancer communication on social media: examining how cancer caregivers use Facebook for cancer-related communication. Cancer Nurs. 2017;40(4):332-338. [ CrossRef ] [ Medline ]
- Reid BB, Rodriguez KN, Thompson MA, Matthews GD. Cancer-specific Twitter conversations among physicians in 2014. JCO. May 20, 2015;33(15_suppl):e17500. [ CrossRef ]
- Warner EL, Waters AR, Cloyes KG, Ellington L, Kirchhoff AC. Young adult cancer caregivers' exposure to cancer misinformation on social media. Cancer. Apr 15, 2021;127(8):1318-1324. [ FREE Full text ] [ CrossRef ] [ Medline ]
- Okuhara T, Ishikawa H, Okada M, Kato M, Kiuchi T. Assertions of Japanese websites for and against cancer screening: a text mining analysis. Asian Pac J Cancer Prev. Apr 01, 2017;18(4):1069-1075. [ FREE Full text ] [ CrossRef ] [ Medline ]
- Qin L, Zhang X, Wu A, Miser JS, Liu Y, Hsu JC, et al. Association between social media use and cancer screening awareness and behavior for people without a cancer diagnosis: matched cohort study. J Med Internet Res. Aug 27, 2021;23(8):e26395. [ FREE Full text ] [ CrossRef ] [ Medline ]
- Denecke K, Nejdl W. How valuable is medical social media data? Content analysis of the medical web. Information Sciences. May 30, 2009;179(12):1870-1880. [ CrossRef ]
- Bender JL, Yue RYK, To MJ, Deacken L, Jadad AR. A lot of action, but not in the right direction: systematic review and content analysis of smartphone applications for the prevention, detection, and management of cancer. J Med Internet Res. Dec 23, 2013;15(12):e287. [ FREE Full text ] [ CrossRef ] [ Medline ]
- Li X, Liu Q. Social media use, eHealth literacy, disease knowledge, and preventive behaviors in the COVID-19 pandemic: cross-sectional study on Chinese netizens. J Med Internet Res. Oct 09, 2020;22(10):e19684. [ FREE Full text ] [ CrossRef ] [ Medline ]
- Zhang X, Wen D, Liang J, Lei J. How the public uses social media wechat to obtain health information in China: a survey study. BMC Med Inform Decis Mak. Jul 05, 2017;17(Suppl 2):66. [ FREE Full text ] [ CrossRef ] [ Medline ]
- Elad B. WeChat statistics by device allocation, active users, country wise traffic, demographics and marketing channels, social media traffic. EnterpriseAppsToday. 2023. URL: https://www.enterpriseappstoday.com/stats/wechat-statistics.html [accessed 2023-12-26]
- Liang X, Yan M, Li H, Deng Z, Lu Y, Lu P, et al. WeChat official accounts' posts on medication use of 251 community healthcare centers in Shanghai, China: content analysis and quality assessment. Front Med (Lausanne). 2023;10:1155428. [ FREE Full text ] [ CrossRef ] [ Medline ]
- NewRank. Ranking of influential health WeChat public accounts(中国健康类微信影响力排行榜). NewRank(新榜). 2018. URL: https://newrank.cn/public/info/rank_detail.html?name=health [accessed 2021-04-30]
- Hospital Management Institute of Fudan University. 2021 National rankings of best hospitals by oncology specialty (2021年度肿瘤科专科声誉排行榜). Hospital Management Institute of Fudan University. 2021. URL: https://rank.cn-healthcare.com/fudan/specialty-reputation/year/2021/sid/2 [accessed 2021-05-01]
- Blei D, Ng A, Jordan M. Latent Dirichlet allocation. Journal of Machine Learning Research. 2003;3:993-1022. [ FREE Full text ]
- World Health Organization (WHO). Health topic: cancer. WHO. URL: https://www.who.int/health-topics/cancer#tab=tab_2 [accessed 2023-12-27]
- Moore SC, Lee I, Weiderpass E, Campbell PT, Sampson JN, Kitahara CM, et al. Association of leisure-time physical activity with risk of 26 types of cancer in 1.44 million adults. JAMA Intern Med. Jun 01, 2016;176(6):816-825. [ FREE Full text ] [ CrossRef ] [ Medline ]
- Stephens P, Martin B, Ghafari G, Luong J, Nahar V, Pham L, et al. Skin cancer knowledge, attitudes, and practices among Chinese population: a narrative review. Dermatol Res Pract. 2018;2018:1965674. [ FREE Full text ] [ CrossRef ] [ Medline ]
- International Agency for Research on Cancer (IARC). Agents classified by the IARC monographs, volumes 1–136. IARC. URL: https://monographs.iarc.who.int/agents-classified-by-the-iarc/ [accessed 2023-12-25]
- Han CJ, Lee YJ, Demiris G. Interventions using social media for cancer prevention and management. Cancer Nurs. 2018;41(6):E19-E31. [ CrossRef ]
- National Institutes of Health (NIH), National Cancer Institute (NCI). Types of cancer treatment. NIH. URL: https://www.cancer.gov/about-cancer/treatment/types [accessed 2021-03-15]
- Cui Y, Che W, Liu T, Qin B, Yang Z. Pre-training with whole word masking for Chinese BERT. IEEE/ACM Trans Audio Speech Lang Process. 2021;29:3504-3514. [ CrossRef ]
- Loomans-Kropp HA, Umar A. Cancer prevention and screening: the next step in the era of precision medicine. NPJ Precis Oncol. 2019;3:3. [ FREE Full text ] [ CrossRef ] [ Medline ]
- Tang Y, Xiang X, Wang X, Cubells JF, Babor TF, Hao W. Alcohol and alcohol-related harm in China: policy changes needed. Bull World Health Organ. Jan 22, 2013;91(4):270-276. [ CrossRef ]
- Zhang M, Yang L, Wang L, Jiang Y, Huang Z, Zhao Z, et al. Trends in smoking prevalence in urban and rural China, 2007 to 2018: findings from 5 consecutive nationally representative cross-sectional surveys. PLoS Med. Aug 2022;19(8):e1004064. [ FREE Full text ] [ CrossRef ] [ Medline ]
- Li J, Wu B, Selbæk G, Krokstad S, Helvik A. Factors associated with consumption of alcohol in older adults - a comparison between two cultures, China and Norway: the CLHLS and the HUNT-study. BMC Geriatr. Jul 31, 2017;17(1):172. [ FREE Full text ] [ CrossRef ] [ Medline ]
- Feng R, Zong Y, Cao S, Xu R. Current cancer situation in China: good or bad news from the 2018 Global Cancer Statistics? Cancer Commun (Lond). Apr 29, 2019;39(1):22. [ FREE Full text ] [ CrossRef ] [ Medline ]
- Scoccianti C, Key TJ, Anderson AS, Armaroli P, Berrino F, Cecchini M, et al. European code against cancer 4th Edition: breastfeeding and cancer. Cancer Epidemiol. Dec 2015;39 Suppl 1:S101-S106. [ FREE Full text ] [ CrossRef ] [ Medline ]
- Espina C, Porta M, Schüz J, Aguado IH, Percival RV, Dora C, et al. Environmental and occupational interventions for primary prevention of cancer: a cross-sectorial policy framework. Environ Health Perspect. Apr 2013;121(4):420-426. [ FREE Full text ] [ CrossRef ] [ Medline ]
- Jensen JD, Moriarty CM, Hurley RJ, Stryker JE. Making sense of cancer news coverage trends: a comparison of three comprehensive content analyses. J Health Commun. Mar 2010;15(2):136-151. [ CrossRef ] [ Medline ]
- Huang H, Shi J, Guo L, Zhu X, Wang L, Liao X, et al. Expenditure and financial burden for common cancers in China: a hospital-based multicentre cross-sectional study. The Lancet. Oct 2016;388:S10. [ CrossRef ]
- People's Daily. 17 Cancer drugs included in medical insurance at reduced prices, reducing medication costs by over 75% (17种抗癌药降价进医保减轻药费负担超75%). People's Daily. 2019. URL: http://www.gov.cn/xinwen/2019-02/13/content_5365211.htm [accessed 2023-12-25]
- Fang W, Xu X, Zhu Y, Dai H, Shang L, Li X. Impact of the National Health Insurance Coverage Policy on the Utilisation and Accessibility of Innovative Anti-cancer Medicines in China: An Interrupted Time-Series Study. Front Public Health. 2021;9:714127. [ FREE Full text ] [ CrossRef ] [ Medline ]
Abbreviations
Bidirectional Encoder Representations from Transformers |
common social media |
Global Vectors for Word Representation |
latent Dirichlet allocation |
long short-term memory |
medical social media |
National Health Insurance Coverage |
World Health Organization |
WeChat public account |
Edited by S Ma; submitted 02.01.24; peer-reviewed by F Yang, D Wawrzuta; comments to author 20.03.24; revised version received 19.04.24; accepted 03.06.24; published 14.08.24.
©Keyang Zhao, Xiaojing Li, Jingyang Li. Originally published in the Journal of Medical Internet Research (https://www.jmir.org), 14.08.2024.
This is an open-access article distributed under the terms of the Creative Commons Attribution License (https://creativecommons.org/licenses/by/4.0/), which permits unrestricted use, distribution, and reproduction in any medium, provided the original work, first published in the Journal of Medical Internet Research (ISSN 1438-8871), is properly cited. The complete bibliographic information, a link to the original publication on https://www.jmir.org/, as well as this copyright and license information must be included.
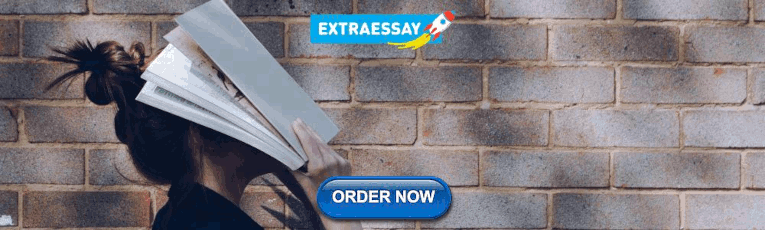
IMAGES
COMMENTS
or. dy − dx. - These errors are much smaller. • In general if different errors are not correlated, are independent, the way to combine them is. dz =. dx2 + dy2. • This is true for random and bias errors. THE CASE OF Z = X - Y. • Suppose Z = X - Y is a number much smaller than X or Y.
The current paper is descriptive qualitative research which aimed at describing grammatical errors of students' writing in English as a Foreign Language (EFL) Class.
The main focus is placed on the explanation of errors, i.e. determining the sources of learners' errors, followed by further analysis of the main causes of both inter lingual and intralingual ...
HERMAN J. C. BERENDSEN is Emeritus Professor of Physical Chemistry the University of Groningen, the Netherlands. His research started in magnetic resonance, but focused later on molecular dynamics simulations systems of biological interest. He is one of the pioneers in this field and, over 37 000 citations, is one of the most quoted authors in ...
Correctly worked examples consist of a problem statement with the steps taken to reach a solution along with the final result and are an effective method for the initial acquisitions of procedural skills and knowledge [1, 11, 26].Cognitive load theory [1, 11, 25] explains the challenge of stimulating the cognitive process without overloading the student with too much essential and extraneous ...
Abstract. Some aspects of science, taken at the broadest level, are universal in empirical research. These include collecting, analyzing, and reporting data. In each of these aspects, errors can and do occur. In this work, we first discuss the importance of focusing on statistical and data errors to continually improve the practice of science.
Get full access to this article. View all access and purchase options for this article.
Abstract. Any data collected will be corrupted by errors; it is important to quantify these errors as the magnitude of the errors will influence the interpretation of the data. Errors arise in all four stages of the experimental process: calibration, acquisition, data analysis, and data combination. The chapter defines and explains how to ...
Specifically, accuracy measures the agreement of our estimates with real values. Typically, the accuracy of an analytical device (LA-ICP-MS in our case) is estimated by evaluating the agreement between the estimates and the accepted values of a reference material.
Hence, to solve this problem, the United States Defense Advanced Research Projects Agency (DARPA) launched a media forensics research plan to develop fake digital media detection methods 5.
This paper presents and discusses a descriptive analysis of the results with respect to three main types of interference: the use of singular and plural nouns, subject-verb agreement, and the ...
1. Systematic Errors: Sometimes called constant errors. These uncertainties can result from errors in calibrating the measuring instruments, constant experimental conditions (e.g. temperature) different from those under which the instruments were calibrated, observational idiosyncracies (e.g. always reading a scale from an angle which is not
Learning a second language and gaining competence in it can only be attained through regular practice and active use of it and during that process learners are expected to commit errors (Brown, 2000).
Data analysis is seldom a straight forward process because of the presence of uncertainties. Data can not be fully understood until the associated uncertainties are unde rstood. g ERROR ANALYSIS The words "error" and "uncertainty" are used to describe the same concept in measurement. It is unfortunate that the term, "error' is the ...
89.332 + 1.1 = 90.432. should be rounded to get 90.4 (the tenths place is the last significant place in 1.1). After multiplication or division, the number of significant figures in the result is determined by the original number with the smallest number of significant figures. For example, (2.80) (4.5039) = 12.61092.
International Journal of Research in Education and Science (IJRES) 611 research adopting it in analyzing written samples of L2 learners at different levels of education. Sermsook et al. (2017) analyzed sentence errors of 26 Thai 2nd year University English major students. 17 types of errors were committed at two different levels.
ISSN 1799-2591 Theory and Practice in Language Studies, Vol. 2, No. 5, pp. 1027-1032, May 2012 © 2012 ACADEMY PUBLISHER Manufactured in Finland.
Quantum Lyapunov control, an important class of quantum control methods, aims at generating converging dynamics guided by Lyapunov-based theoretical tools. However, unlike the case of classical systems, disturbance caused by quantum measurement hinders direct and exact realization of the theoretical feedback dynamics designed with Lyapunov theory. Regarding this issue, the idea of closed-loop ...
In an analysis of electronic health records researchers found errors ... has shed light on the rate and impact of diagnostic errors in hospital settings. In an analysis of electronic health records from 29 hospitals across the country of 2,428 patients who had either been transferred to an intensive care unit (ICU) or died in the hospital, the ...
Contrastive analysis of some errors committed by second language Learners of English at the English department, faculty of education Aljoufra, Sirte University Dr. Ahmed Mohamed Gaddafi Waddan faculty of education Sirte University Abstract: The main concern of this paper is to focus on the errors committed by second language learners of English at the English department faculty of education.
students' writing to know the type of errors the students do most in their writing grammatically to know the causes of errors. After that, the writer describes the number of errors that the students made. The writer describes the errors in each sentence and gives an analysis. For this research, the writer analyses each
data, focusing on the types, frequency, and sources of errors. The findings shed light
Writing is a crucial aspect of English language abilities as a foreign language. Writing is an activity that involves creating thoughts and then deciding how to communicate them and created a set of statements and paragraphs that others could read and understand. The aim of this research is to describe the types of errors in writing text and to describe the dominant errors in writing ...
Siwei Zhang is first author of JAMIA paper. Congratulations to PhD candidate Siwei Zhang, alumnus Nicholas Strayer (PhD 2020; now at Posit), senior biostatistician Yajing Li, and assistant professor Yaomin Xu on the publication of "PheMIME: an interactive web app and knowledge base for phenome-wide, multi-institutional multimorbidity analysis" in the Journal of the American Medical ...
FDIC Center for Financial Research Working Paper No. 2024-02 Ajay Palvia, George Shoukry and Anna-Leigh Stone. Explaining the Life Cycle of Bank-Sponsored Money Market Funds: An Application of the Regulatory Dialectic. FDIC Center for Financial Research Working Paper No. 2024-01 Stefan Jacewitz, Jonathan Pogach, Haluk Unal and Chengjun Wu
Background The UK soft drinks industry levy (SDIL) was announced in March 2016 and implemented in April 2018, encouraging manufacturers to reduce the sugar content of soft drinks. This is the first study to investigate changes in individual-level consumption of free sugars in relation to the SDIL. Methods We used controlled interrupted time series (2011-2019) to explore changes in the ...
To facilitate adoption of the CRA provisions, these requirements need to be translated into the form of harmonised standards, with which manufacturers can comply. In support of the standardisation effort, this study attempt to identify the most relevant existing cybersecurity standards for each CRA requirement, analyses the coverage already offered on the intended scope of the requirement and ...
The present study seeks to explore EFL learners' major writing difficulties by analyzing the nature and distribution of their writing errors and it also investigates whether there is a ...
Background: Nowadays, social media plays a crucial role in disseminating information about cancer prevention and treatment. A growing body of research has focused on assessing access and communication effects of cancer information on social media. However, there remains a limited understanding of the comprehensive presentation of cancer prevention and treatment methods across social media ...
There's research that suggests detectors are biased against nonnative English speakers, and several of the students who shared their experiences were writing in English as a second, or even a ...