Thank you for visiting nature.com. You are using a browser version with limited support for CSS. To obtain the best experience, we recommend you use a more up to date browser (or turn off compatibility mode in Internet Explorer). In the meantime, to ensure continued support, we are displaying the site without styles and JavaScript.
- View all journals
- Explore content
- About the journal
- Publish with us
- Sign up for alerts
- Review Article
- Open access
- Published: 20 November 2023
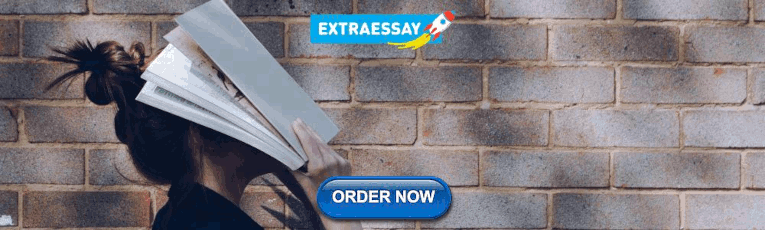
Behavioural biases in real estate investment: a literature review and future research agenda
- Akshita Singh 1 ,
- Shailendra Kumar 1 ,
- Utkarsh Goel 1 &
- Amar Johri 2
Humanities and Social Sciences Communications volume 10 , Article number: 846 ( 2023 ) Cite this article
5204 Accesses
1 Citations
2 Altmetric
Metrics details
Psychological aspects of human nature cause behavioural biases and can lead to decisions that differ from what is expected based solely on rational analysis. The effects of behavioural biases on financial markets like stocks and mutual funds have been studied previously, but real estate has yet to receive much attention. The existing works in the real estate domain have focused on different biases, but no study has examined the works already done to provide concise documentation of these past works. Thus, this article is an earnest attempt to fill that gap. This paper reviews the articles which were sourced from Scopus and the Web of Science database, published between 1980 and 2022. The PRISMA model led to the inclusion of 86 articles for the review. Analysis revealed that anchoring bias, loss aversion, and herding bias have been studied extensively. On the other hand, biases like gambler’s fallacy, familiarity bias, framing bias, home bias, confirmation bias and mental accounting have been less explored. The paper identifies the substantial gaps in the existing studies, giving avenues for future exploration. The key ones are, firstly only a few biases have been studied extensively and many biases are less explored, particularly using primary data. This provides a vast available space for future work. Secondly, studies in developing countries are fewer, which needs to be addressed. Lastly, studies need to explore the interplay of different biases to create a more robust model that can explain the effect of these biases. The paper gives a conceptual understanding of different biases and what factors affect them. Also, it will help policymakers strategize their business and mitigate the negative effects of biases.
Similar content being viewed by others
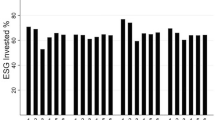
Optimistic framing increases responsible investment of investment professionals
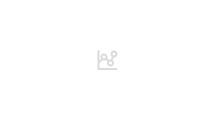
Earnings management and financial distress or soundness determining CEOs’ future over- and under-investment decisions
Economic policy uncertainty and cash dividend policy: evidence from china, introduction.
The need to better understand the factors and processes that influence investment decisions has led to a greater investigation into the investment decision-making process (Kengatharan and Kengatharan, 2014 ; Chaudary, 2019 ). Numerous principles and theories assist traditional finance asserting that markets are efficient and systematic; these include “the Markowitz portfolio theory”, “the Miller and Modigliani arbitrage theory”, “the CAPM model of Sharpe, Linter & Black”, and “the option pricing theory of Black, Scholes and Merton” (Merton, 1985 ; Zain-ul-Abdin et al., 2019 ). The assumption that becomes the founding pillar for the efficient market hypothesis (EMH) is that investors are rational and the estimation of financial asset prices includes the incorporation of all the available information (Fama, 1970 ; Weixiang et al., 2022 ). The efficient utility theory (EUH) states that investors face the challenge of choosing among various alternatives amidst a world crowded with uncertainties during decision-making, and investors make rational judgements by weighing all available options according to their benefits and the risks they pose. Thus, they make balanced decisions (Kumar and Goyal, 2015 ; Jain et al., 2022 ).
The “Prospect theory,” which exhibits conflicting outcomes with EMH and EUH, was brought to the public’s notice by the investigations conducted by Kahneman and Tversky ( 1979 ). The theory questioned the idea of rationality and fundamentally altered how investment decision-making was studied. The economists Meir Statman, Richard Thaler, Hersh Shefrin, David Bell, Robert Shiller, and others continued this path-breaking research finding. Thus, how do investors behave? Furthermore, why do they behave in a particular manner? To answer these questions, a new concept of behavioural finance emerged in 1980. Behavioural finance discusses the irrationality in decision-making and the psychological elements driving it. The investor’s decision usually deviates from rationality and leans toward irrationality due to behavioural biases (Baker and Ricciardi, 2015 ; Kumar and Goyal, 2015 ). Behavioural bias is an inclination towards error (Shefrin and Statman, 1985 ). Amidst this ongoing debate between rational and irrational decision-making, Lo ( 2012 ) suggested that investor behaviour is not hard-lined towards either side of rationality but is a situational adaptive behaviour where investors behave more rationally during stable market conditions and tend to become irrational during turbulent or volatile market condition (Apau and Jeke, 2022 ; Muzindutsi et al., 2023 ).
Various behavioural biases influence investors when making investment decisions (Dervishaj, 2021 ; Mittal, 2022 ; Bihari et al., 2023 ; Sinha and Shunmugasundaram, 2023 ). The commonly discussed biases in different investment avenues, i.e., Stock market, mutual fund, and real estate investments, are overconfidence, disposition effect, anchoring bias, availability bias, loss aversion, regret aversion, herding, representativeness, mental accounting, etc. While the research has mainly focused on stock markets, studies on behavioural biases have substantially improved our understanding of investor actions in financial markets. In this area of research, real estate investing needs to receive more attention. Investment in real estate is the most significant financial transaction undertaken by the average household (Beracha and Wintoki, 2013 ; Seay et al., 2018 ; D’Lima and Schultz, 2021 ). Despite several studies demonstrating an integrated relationship between real estate and stock returns, real estate markets differ in liquidity, transparency, and regional heterogeneity (Bao et al., 2021 ; van Dijk et al., 2022 ). The key difference between investment avenues, like the stock market or mutual funds, and real estate is that while the former avenues are available over the counter in a well-defined systematic market, the latter is more of an unorganized market where people invest in purchases and sales on personal levels and not on any systematic trading platform. Real estate frequently displays behaviour difficult for typical asset market models to account for (Engelhardt, 2003 ; Beracha and Skiba, 2014 ). For instance, they show sharp price fluctuations (Li and Wan, 2021 ). Buyers frequently offer varying prices for properties that are almost identical, which may be due to information asymmetries in real estate markets (Zhou et al., 2015 ). This information asymmetry and difficulty in getting systematic data easily may have caused reduced interest by researchers in studying this field. The data from stock markets are quickly and transparently available due to a systematic market worldwide. This transparency is lacking in the real estate sector (Schulte et al., 2005 ; Newell, 2016 ). Also, the data is not readily available, which naturally deflects the researchers as data availability is a significant issue while performing research (Krause and Lipscomb, 2016 ). Such issues cause less interest by researchers in this area of study.
In real estate literature, few studies have focused on behavioural anomalies (Arbel et al., 2014 ). Despite this transaction’s high stakes and deliberate nature, prior research has yet to offer conclusive answers. Understanding how real estate investors behave is essential because real estate is becoming a more popular asset class in portfolios (Kumar and Vergara-Alert, 2020 ). The recent trends show that the Indian real estate sector is set to grow at a tremendous rate according to a report by India Investment Grid (IIG). With the increasing popularity of easy investment vehicles like REITs, this sector will require a thorough understanding which provides motivation for this study. This study aims to delve into one of the crucial facets of behavioural research: behavioural biases that influence individual decision-making in real estate investment. This study is unique in the manner that no study exists to the best of the authors’ awareness that reviews existing research regarding the investment behaviour of real estate investors. This paper looks forward to compile and examine the existing research works that study behavioural biases in the real estate sector to provide a concise summary and address the following research questions:
RQ1: What are the study trends in behavioural biases in real estate investing in terms of year of study, sample country, journal of publication, statistical approach, data type, and the number of papers on a particular bias?
RQ2: What does content analysis reveal about the knowledge imparted by previous research works for different behavioural biases?
RQ3: What research gaps are still present and possible future research agenda in the context of behavioural biases in the real estate investment sector?
The flow of the paper is as follows: The paper begins with “Introduction” which introduces the work. In this, Fig. 1 represents the pictorial presentation of how this paper has been organized. Section “A prelude to behavioural biases in real estate investment” briefly talks about different behavioural biases that exists in real estate investment. Up next, “Research method: literature search approach” illustrates the research methodology adopted. In the section “Literature review: classification and analysis of the literature”, the articles are reviewed and analysed, after which, “Content analysis” section discusses each bias in detail. The section “Results and discussion” discusses the findings. In the end, “Conclusion and future scope” concludes the present literature review and gives the future research agenda.
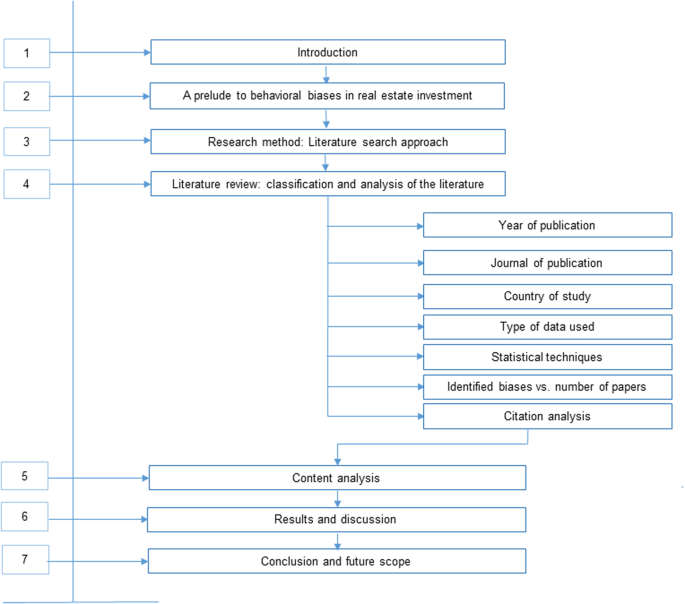
This figure shows the pictorial presentation of how the paper has been organized into various sections.
A prelude to behavioural biases in real estate investment
The term “behavioural bias” describes a pattern of judgmental variation that occurs in particular settings and can occasionally lead to perception alteration, inaccurate judgements, illogical interpretation, or irrationality. Biases are the inclination towards error (Shefrin and Statman, 1985 ). Going by the definition in the dictionary, biases, and flawed cognitive reasoning and thinking draw similarities; nevertheless, it is more accurate to say that they are caused by poor reasoning influenced by feelings or emotions. Understanding the effect of behavioural biases on investment decisions is essential. With its understanding, investors and their advisors may be better equipped to achieve their objectives and enhance economic outcomes. Timely recognition of behavioural biases can help in avoiding potential financial disasters.
A variety of behavioural biases influence investors while they make investment decisions. The lack of information or inability to interpret it gives rise to several biases. Overconfidence is a cognitive bias propagated by Odean et al. ( 1998 ). In the presence of this bias, people tend to exaggerate their skills, knowledge, and predictions while downplaying the possibilities of future uncertainties (De Bondt and Thaler, 1995 ). Overconfident people feel their judgement is more trustworthy than others (Jain et al., 2015 ). Under the influence of the representativeness heuristic, investors try to associate the past performance or information of a certain market condition or firm with the general notion that the same outcome will happen in the future. In the event of a lack of information, our brain often tries to complete the mental illustration by combining prior knowledge and thinking to deduce the probability of a certain outcome. E.g., when purchasing a home, buyers frequently assess the risk of the property and the potential return on their investment by comparing the price to that of comparable homes in the area (Kahneman and Tversky, 1979 ). Similar to this bias, investors also suffer from Gambler’s fallacy. It is also known as the Monte Carlo fallacy. It occurs when someone mistakenly thinks that the outcome of a prior event or series of events will determine how probable an upcoming random event is to occur (Malik et al., 2021 ). When investors cannot deduce logical reasoning from the market information, they tend to show herding bias. Like sheep walking in herds, investors often blindly follow what other or larger groups of investors are doing without applying logical deduction of those activities (Kahneman and Tversky, 1979 ). It has been noted that investors who suffer from this prejudice frequently consult brokers, friends, and co-workers before making an investment decision. Apart from the information itself and its deduction, the timeline of the information availability and the theme of presenting that information is also important. In availability bias, decision-makers favour the most recent information and events they can access rather than considering other options (Cascão et al., 2023 ). According to framing bias, biased information presentation might alter the investor’s opinions. Investors avoid risk when information is presented positively to ensure profits. However, they will accept the risk to prevent losses when the same information is presented in a negative frame. Lastly, people also tend to make irrational decisions due to mental accounting bias, where they categorize money differently based on subjective standards, frequently prompting illogical spending and financially unwise investment choices (Thaler, 1985 ; Enslin et al., 2022 ).
Humans tend to perceive profits and losses differently. This anomaly gives rise to three behavioural biases: disposition effect, loss aversion and regret aversion. When deciding whether to sell or keep an investment, losing investments are often held onto too long by investors. Rather than holding profitable securities for long periods of time, they often dispose of them prematurely. This is known as the disposition effect (Basana and Tarigan, 2022 ). According to Shefrin and Statman ( 1985 ) winning stocks should be sold, and losing stocks should be retained. Loss aversion was coined by Benartzi and Thaler ( 2000 ). It occurs because people react differently to guaranteed profits and guaranteed losses. People prefer not to take risks when there is a guarantee of profit, but they are willing to take risks if there is a potential of losing. This demonstrates that they value loss certainty more than loss uncertainty. According to the idea of regret aversion, when people regret a choice, it has a bigger impact on subsequent decisions. As a result, investors are either encouraged to take more risks or discouraged from making risky investing decisions. This is done to avert any feelings of regret further (M. J. Seiler et al., 2008 ).
Reluctance to change or relying stubbornly on some information that has been previously received also causes behavioural biases. Anchoring bias is one such bias where investors tend to base their decisions on an illogical price, which is sometimes meaningless. Because of this bias, investors frequently set prices based on previous knowledge of purchasing and selling. This leads to mistiming of the market and results in investors buying or selling securities at exorbitant prices. Investors may also miss out on profits if they fail to meet the price they set to buy or sell securities (Northcraft and Neale, 1987 ). Investors often get affirmation about their decision by seeking information or evidence that supports or confirms it, often from their peer group or friends. This tendency gives rise to confirmation bias (Gallimore, 1996 ).
Investors also tend to favour what is known to them while attempting to shun the unknown under the familiarity bias (Seiler et al., 2013 ; Ambrose and Shen, 2023 ). To this, the endowment effect was coined by Kahneman et al. ( 1990 ). People place too much attention on their current beliefs and are unwilling to modify them. Due to this, they pass up even the most lucrative investment prospects. Investors also tend to prefer investing in securities of domestic countries rather than diversifying it with foreign investments. This is known as home bias (French and Poterba, 1991 ; Lin and Viswanathan, 2016 ; Riff and Yagil, 2016 ; Coën et al., 2021 ).
Research method: literature search approach
The author has systematically reviewed the literature to analyse the articles published on behavioural bias in real estate investment. Systematic literature review (hereafter, SLR) is regarded as the most illuminating and scientific compared to other reviews (Snyder, 2019 ; Paul et al., 2021 ). SLR attempts to address a research question, typically regarding the state of a certain field of study (Kraus et al., 2020 ; Muthuri et al., 2020 ). Another significant aspect of SLR is that it provides solutions to queries that may mislead the findings if only one study is considered (Petticrew and Roberts, 2008 ).
The studies in behavioural finance were initiated in the year 1980. To ensure proper inclusion of the behavioural finance literature, the paper solely focuses on the studies conducted between 1980 and 2022, which have studied one or multiple biases in their paper. The literature was drawn from two leading databases, “Scopus” and “Web of Science”, thereby enabling the analysis of high-quality studies in concerned areas.
The “Preferred Reporting Items for Systematic Reviews and Meta-Analyses” (PRISMA) criteria, which called for the use of keywords to search for pertinent works in the database, were used in the systematic review procedure (Liberati et al., 2009 ; Li et al., 2022 ). The keywords were chosen after carefully examining previous articles. The keywords were searched on both the database on October 12, 2022.
Initially, 16,991 papers were identified relating to keywords searched in the above-mentioned databases. The search criteria entered to limit the literature search in the field: (“Overconfidence” OR “Loss aversion” OR “Regret aversion” OR “Herding” OR “Psychological bias” OR “Cognitive bias” OR “Emotional bias” OR “Representativeness” OR “Anchoring” OR “Mental accounting” OR “Disposition”) AND (“Real Estate” OR “Housing” OR “Property”). Further, we considered the following criteria for the inclusion of the article in our study:
Articles having the search keywords in the article title, abstract and keywords.
Subject: Economics, econometrics and finance; Business, management and accounting; social sciences in Scopus. Management; business; business finance, economics in web of science.
Document type: Article
Articles published in English language only.
Taking these criteria into account, 15,734 papers were excluded through the database search, and 125 duplicate papers were eliminated. Less adequacy of titles and abstracts resulted in the exclusion of 727 and 312 articles, respectively. This resulted in 93 papers being available for full-text analysis, of which 5 papers did not have full-text availability, and 2 papers did not relate to the theme of the study and hence were excluded. Therefore, the final sample counted 86 most relevant articles for the study. The flow chart for the review of the article following the PRISMA framework is given in Fig. 2 .
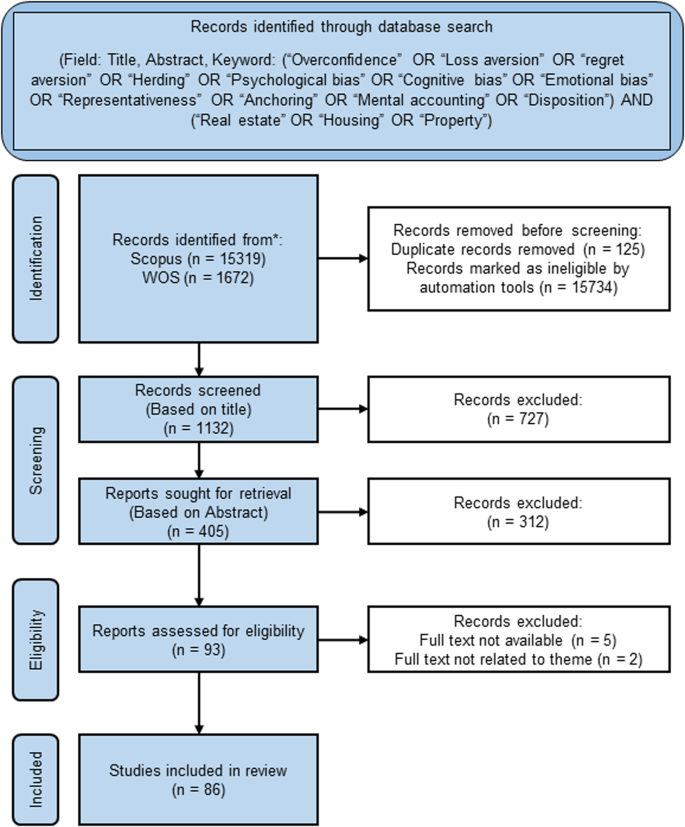
This figure illustrates the PRISMA framework adopted in the study. It shows the count of articles taken up at every stage along with the exclusion criteria and number of excluded articles.
Literature review: classification and analysis of the literature
A total of 86 research papers were examined and categorized based on the year of publication, country of study, source of data collection, statistical techniques used, and citation analysis. This section presents the results of the reviewed literature.
Year of publication
Figure 3 (Table 1 in annexure) represents the distribution of the articles published from 1980 to 2022, according to chronological order, with each year being further divided according to the behavioural biases studied in those years. Studies on behavioural bias in real estate started in 1987, and in recent years studies on this topic have gained momentum. An interesting takeaway from the distribution of articles over time is that the number of articles jumped significantly post-2010. On deeper analysis, it was observed that around 50% of the studies after 2010 were from the USA. This can be attributed to the fact that the housing market crash that occurred in 2008 caused a severe economic recession, and thus it could have led the researchers to delve into the arena of real estate investment so that a better understanding can be offered to minimize such risks in future (Crosby et al., 2018 ). The recent development in the general sentiment of people investing in assets and buying real estate as a means of investment can be seen from the growth in the number of studies. This investment interest, in general, drives the need for a deeper analysis of this avenue. Also, the availability of information for conducting studies has become more accessible due to digital platforms and databases. The graph further shows the distribution of studies according to biases year-wise. This demonstrates that anchoring bias has always been a priority almost every year. Loss aversion has been studied a lot in recent years. Other biases have slowly gained momentum, showing a vast area that still needs to be explored.
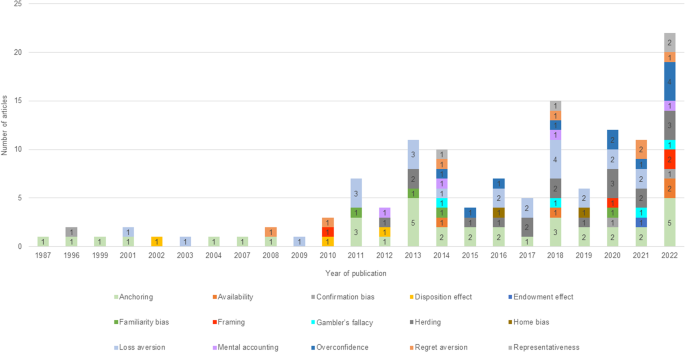
It shows the distribution of articles over the period of 1980–2022. It also highlights the number of articles corresponding to different biases for a particular year.
Journal of publication
This analysis identifies the key journals and publications in this area. Figure 4 (Table 2 in annexure) shows 86 research papers were collected from 45 journals. Out of these, 15 journals had two or more than two publications. Further, it is interesting to note that, Journal of Real Estate Finance and Economics, Journal of Property Research, Real Estate Economics, International Journal of Housing Markets and Analysis and, Journal of Real Estate Research published 32 papers on biases in real estate investment. Journal of Real Estate Finance and Economics emerges as the highest publisher with 9 papers, followed by Journal of Property Research with 7 papers.
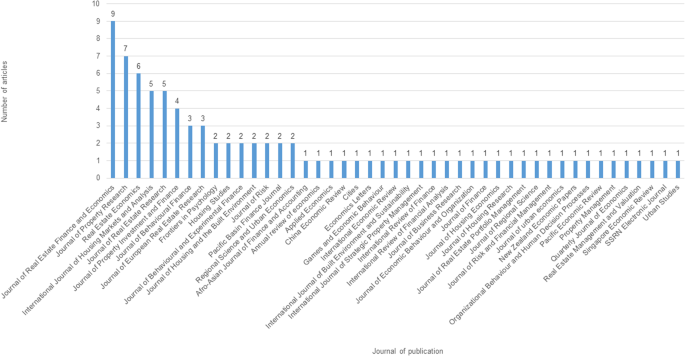
The analysis shown through this figure highlights the key journals in the field of behavioural biases and the number of articles in those journals.
Country of study
Figure 5 (Table 3 in annexure) shows the bifurcation of articles based on the country where the authors have collected the data or chosen the geographic domain for the studies. The visualization shows that developed countries like the USA and the UK have been the primary locations for most studies. On the other hand, developing countries have been targeted less, resulting in fewer studies. The primary reason for such an anomalous distribution of study is that in developed countries, real estate transaction records are readily available in the public domain, and most transactions are institutional, resulting in more accessible data collection. Moreover, the large number of such transactions results in an abundance of information for statistical analysis. In developing countries like India, most real estate transactions are on a personal level, with agents working independently, resulting in less publicly available information. Another possible factor is a more generalized one regarding research works. The developed countries have much more funding availability and understanding of the long-term effects of proper research work in these sectors. Developing or low-income countries have a comparative shortage of funds for research and other glaring issues to deal with rather than understanding the long-term benefits of research. This causes an abundance of fruitful research in developed countries while shortage in their counterpart countries.
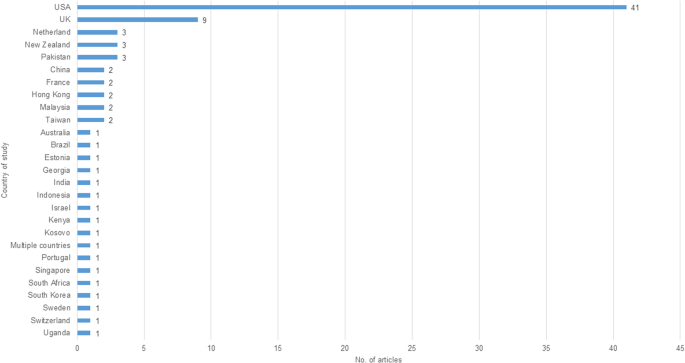
The figure shows how the articles are divided based on the country where the authors have collected the data or have chosen the geographical domain for their study.
Type of data used
Analysis of the literature on the type of data helps us identify the focus of past research. Figure 6 (Table 4 in the annexure) shows the distribution of the study according to the type of data collected for each bias. Out of 86 studied papers, some articles discussed multiple biases, which made an addition to the primary and secondary list. This table shows that while anchoring bias has a healthy distribution between primary and secondary data, most of the other biases either have more primary and less secondary data-based articles or vice versa. We see that herding and loss aversion have a large number of secondary data-based articles and very less studies have used primary data. The importance of primary data-based articles is that they take the real opinions of the target population and hence are closer to real-life scenarios while secondary data-based articles are necessary to test the models and hypotheses on a large sample size to prove the robustness.
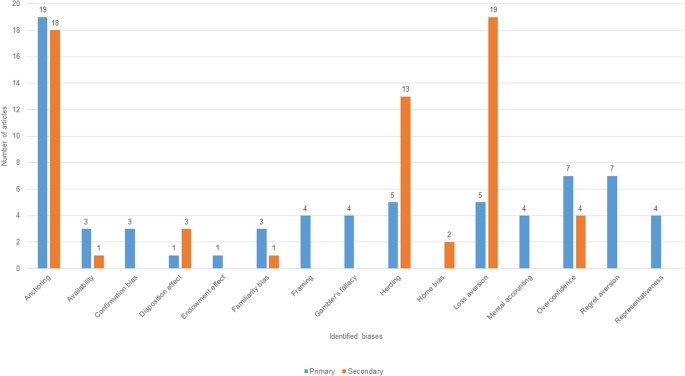
The figure illustrates the distribution of articles based on the type of data used (primary or secondary).
Statistical techniques
This section explores the bifurcation of the studied articles according to the statistical technique employed in them. This exploration is essential in showcasing this avenue’s most sought-after and expansively used method. Figure 7 (Table 5 in annexure) shows the number of articles for various statistical techniques found in the studies and highlights that Regression analysis has been used most times (23 out of 86 selected papers). Different types of regression, such as logit regression, probit regression, linear regression, ordinary least squares, and multiple regression, were used. Only some studies have used multiple techniques such as correlation, chi-square, factor analysis, etc. Articles were not excluded or accepted based on statistical technique; however, during analysis, it was found that only three relevant articles were based on qualitative techniques, and the rest were based on quantitative techniques.
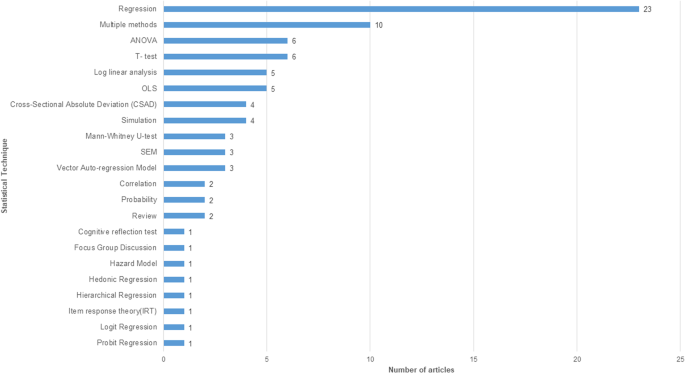
The figure illustrates the different statistical techniques used by the researchers to study behavioural biases.
Figure 8 shows the distribution of biases according to the statistical techniques used in the articles. For almost all biases, the majority of the studies are regression-based. Regression is preferred in predictor-outcome-based model studies due to its simplicity in application and numerous statistical software providing easy regression analysis ranging from MS Excel to IBM SPSS (Fumo and Rafe Biswas, 2015 ). The studies have used multiple linear regression with more than one predictor variable. The regression models give easy access to isolate those predictors that cause problems to the model and find a mathematical relationship that can be used in future works (Rencher, 2005 ). They are also well-proven for hypothesis testing, which gives insights into whether or not what a researcher thinks is correct (Goldstein et al., 1979 ). We can see that more rigorous techniques like SEM are used in a minimal number of articles, and fewer articles have used unique methods like IRT or vector auto-regression.
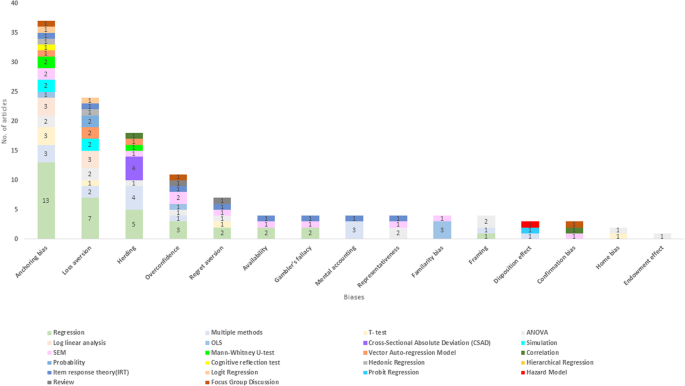
The figure illustrates the distribution of articles according to the bias studied and the statistical technique used in them.
Another interesting thing to note from this analysis is that articles on qualitative methods are much fewer. As explained in the work of Rust et al. ( 2017 ), there is a widespread misbelief that quantitative analysis is more critical due to clear statistical outcomes than qualitative methods. Qualitative methods are essential because they help in gaining a deeper understanding of the theories involved in some particular area of research so that we are well prepared before applying statistical techniques (Gutmann, 2014 ). Ignoring the qualitative approach is like ignoring the theory classes before going for practical. While the quantitative approach works towards establishing the robustness and validity of the model, qualitative methods help in establishing the credibility and logic of the model. We can thus infer that there is a need to focus more on theory development and qualitative work with regard to biases so that a balance between validity and credibility is achieved (Rust and Hughes, 2018 ).
Identified biases vs. number of papers
Behavioural biases have been examined in this section through the research findings of the chosen papers. Figure 9 (Table 6 in annexure) shows that 37 papers are available on anchoring bias, 24 on loss aversion, 18 on herding, and 11 on overconfidence bias. However, a dearth of studies on the home bias, familiarity, framing, endowment effect, and disposition effect in the real estate context gives an inconclusive finding.
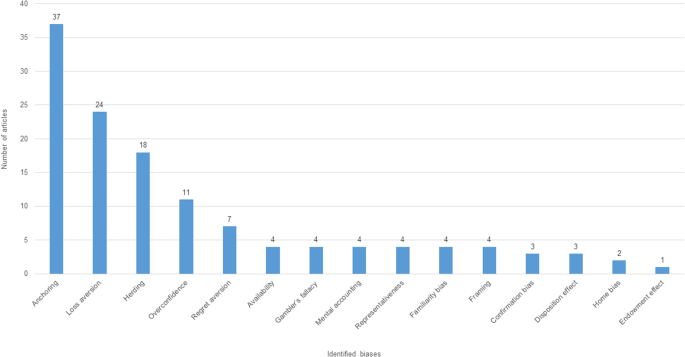
The figure shows the number of identified articles talking about a particular bias.
Citation analysis
In this section, we assessed various articles according to their citations for different biases to find the most pertinent and significant papers available. The citation was located using Google Scholar. We identified that 80 of the 86 selected articles are cited elsewhere. This confirms the reviewed article’s validity, suggesting that other researchers have deemed their work useful. We did not find any citations for 6 articles published recently. Table 7 (in the annexure) shows the articles on various biases with their citation counts. The authors have included only papers with more than 25 citations to avoid a long list of articles.
Content analysis
A research method for the objective, methodical, and quantitative description of communications’ manifest content is called content analysis (Burns and Berelson, 1952 ). The empirical results of earlier research investigations are disclosed using content analysis. In this section, the authors have systematically done the content analysis of different behavioural biases identified in the real estate investment literature. The biases having <5 papers are studied collectively under the “Other biases”.
Anchoring bias
Anchoring bias is a cognitive bias present in the real estate sector (Bao et al., 2021 ; Diaz et al., 1999 ) where people hold on to a reference point in the form of some information about the investment and act biased due to it (Yan and Bao, 2018 ). The initial listing price has been considered an important anchor where investors judge a property value based on the asking price first quotes. Such biasing is not only present with a novice like students (Silva et al., 2019 ) but also with seasoned professionals (Northcraft and Neale, 1987 ) and property fund managers (Lowies et al., 2016 ). Such asking price is also used to judge the property value and arrive at a price; however, anchoring barricades buyers to arrive at a fair price (Bao and Saunders, 2021 ; Bokhari and Geltner, 2011 ; Bucchianeri and Minson, 2013 ). Also, such anchors affect the decision-making of real estate investors (Chang et al., 2016 ; Hoxha and Hasani, 2022 ; Pandey and Jessica, 2018 ; Waweru et al., 2014 ) and negotiations that play in the transaction (Black and Diaz, 1996 ). Such negotiations are affected by round numbers, i.e., prices ending with more zeros. Buyers tend to form an anchor around a round number (Pope et al., 2015 ). The effect is persistent when buyers consider the previous purchase price as an anchor (Leung and Tsang, 2013b ). This anchor around the previous purchase price is also evident when owners have a property whose current value is less than the purchase price, causing them not to list the property and hold on to it (Lane et al., 2011 ). Along with the previous purchase price, sellers sometimes want to achieve break-even and thus form an anchor around it (Seiler et al., 2012 ). Also, this anchoring bias affects risk perception, which in turn affects the investor’s decision-making (Zhang et al., 2022 ).
Apart from pricing, other factors like age, annual income, gender, and information accessibility have been studied in relation to anchoring bias. Younger people have been identified to show higher levels of anchoring bias along with lower-income earning groups. Women also tend to offer more anchoring bias than men (M. J. Seiler et al., 2008 ; Hjalmarsson and Österholm, 2021 ). The availability of information on property value plays an essential role in decision-making. Buyers tend to form anchors when they get information or reference points; sellers can use this tendency to mould buyers in their favour (Paraschiv and Chenavaz, 2011 ). Investors with price uncertainty are thus more likely to fall prey to anchoring bias (Chang et al., 2016 ). Searching the internet prolifically can also cause investors to rely deeply on information received and form and anchor around it (Beracha and Wintoki, 2013 ). However, investors also sometimes tend to anchor around previously or easily available information (Cascão et al., 2023 ). Sellers also seem to consider local fundamentals during the first sale and anchor the initial price in accordance with it (Clapp et al., 2020 ).
The research by Cheung et al. ( 2022 ) revealed that the capital value (hereafter, CV) evaluation in pricing provided by governments in several nations has some influence on the transaction price (TP). For used properties, TP and CV demonstrated a bi-directional, lead-lag connection. Moreover, the study by Ali et al., ( 2020 ) and Klamer et al. ( 2017 ) showcased that property evaluators suffer from anchoring bias, and tend to form their anchor around the previously transacted price. The impact of these government laws and initiatives on the time required to accept such offers was examined, and it was discovered that if sellers and agents effectively utilize them, the time needed to finalize deals is reduced (Arbel et al., 2014 ). However, Diaz and Hansz ( 2001 ) claim that as long as the appraisers are working in a field, they are comfortable with, an anchor (anonymous expert value) does not affect real estate valuations. However, when appraisers value the property in unfamiliar geographical areas, anonymous expert opinion affects their valuation (Diaz and Hansz, 2001 ). Sometimes, the clients and customers have thinking about the price or value of a property and thus the evaluators get under pressure forming an anchor around that price. Thus the customer influence also causes anchoring bias (Lee et al., 2022 ; Nwuba et al., 2015 ).
Researchers have also examined the property location as a factor for anchoring bias. They postulated that the distance of the property from the buyer’s location may significantly impact the price paid and thus support the anchor in the form of location (Clauretie and Thistle, 2007 ). Buyers who look into a property far away from them have to spend considerable time looking for information and rely on secondary sources since they are not present in that geographic area. This search constraint resulted in paying higher prices than local buyers (Lambson et al., 2004 ). This evidence is further strengthened by an expansive study by Ling et al., ( 2018 ) who used a large sample of 114,588 sales transactions to see this effect. Differentiating the benefits that local buyers get over distant buyers, it is seen that local buyers have an advantage of easily available information and access to options open in the market in the near vicinity. Moreover, people hailing from high-priced property areas will tend to negotiate in and around that reference price point resulting in paying more than what would have been paid by a person hailing from a lower-priced area. This confirms that location acts as an anchor causing buyers to spend more or less with respect being far or near to the target property (Zhou et al., 2015 ).
Various anchors have been studied to determine their effect on the housing market, including the initial listing price of the property, its distance from investors or buyers, government valuations, and public information. However, after analysing these anchors separately, it is important to analyse the real-world picture of real estate investment. A variety of factors influences real-world housing prices intermixed together. It may be worthwhile to explore the combined effect of these anchors in the future and see which anchors tend to dominate the projecting of real estate prices. Despite the complexity of future work, it should yield a more accurate model and promote a multi-directional approach in the housing market.
Loss aversion
Loss aversion arises when people have different perceptions of similar amounts of profit or loss in transactions (Bao et al., 2021 ; Yan and Bao, 2018 ; Mayer, 2011 ). Genesove and Mayer ( 2001 ) studied loss aversion as a behavioural bias for the first time. They explained that homeowners treat gains and losses differently and are averse to the potential nominal loss. They will tend to wait for a potentially high-paying customer to avoid the nominal loss they may have experienced earlier or anticipated (Waweru et al., 2014 ). Owners expecting loss set higher listing prices (Anenberg, 2016 ; Liu et al., 2018 ) and such houses that were expected to sell at a loss sold at an increased price (Greenaway-McGrevy and Haworth, 2020 ). Also, the prices are affected if sellers have prior loss experience (You, 2020 ). This effect of loss aversion is more predominant when considering commercial real estate and more seasoned investors. The effect in commercial real estate has been adjudged to be more predominant because there are ample amounts of transactions happening and thus, investors and sellers have more reference points to compare prices and, thus, high loss aversion bias (Li and Wan, 2021 ). Even in commercial real estate, the effect is more for more sophisticated and experienced investors because their experience of transactions helps them to be more averse to potential losses (Bokhari et al., 2011 ).
This behavioural bias has also been an antecedent in housing mobility, wherein people move to newer locations to avoid nominal loss (Engelhardt, 2003a ; Steegmans and Hassink, 2018 ). The asymmetric preference for loss and gain makes loss aversion an important factor and helps predict housing prices (Anenberg, 2011 ; Leung and Tsang, 2013a , 2013b ; Hayunga and Pace, 2017 ). It is also found that housing loan borrowers are affected by loss aversion and sometimes willingly default to repay (Bhutta et al., 2017 ). However, Pandey and Jessica ( 2018 ) presented a contradicting view and said that loss aversion did not influence real estate investors.
Further studies have tried to find the factors influencing loss aversion like time dependency, experience, owners’ race, and usage of agents. Buisson ( 2016 ) suggests time horizon to be an important factor in determining the degree of loss aversion. When the time horizon is short, sellers tend to be more risk-averse and are willing to accept lower prices in order to mitigate the loss that they perceive to be impending. However, when the time horizon is long enough, they tend to wait for a higher price or may exit the market and are less prone to this bias. In addition, the race of owners or usage of agents in transactions does not seem to affect the asking price, but past experiences of loss do tend to have a significant impact (Hayunga and Pace, 2017 ).
While sellers and owners tend to show this bias and have been studied extensively, the effect of loss aversion on selling prices is more magnified when it is associated with real estate developers (Bao et al., 2021 ). Real estate developers tend to be risk averse in selling new real estate, and the increase in price gets delivered down to the ultimate price that homeowners will have to pay to acquire the property. To differentiate the effect, private firms tend to have a greater effect than state-owned firms, and unlisted firms have a greater effect than listed firms (Bao et al., 2021 ). Loss-averse sellers tend to deter sales during the downfall of the market (Tu et al., 2009 ) and negotiate to reduce the risk of loss rather than maximizing profit (Zillante et al., 2019 ). This causes the difference between the price sellers are willing to accept and the price buyers are willing to pay (Gong et al., 2019 ). It also causes many sales to expire (Carrillo, 2013 ).
Despite the presence of loss aversion in commercial real estate (Li and Wan, 2021 ), more data on commercial transactions is necessary to fine-tune the findings. This still needs to be approached and thus demands future work. Another interesting study would be to exploit the time horizon effect suggested by Buisson ( 2016 ) on private firms since they tend to show a higher degree of loss aversion (Bao et al., 2021 ). This behavioural bias has been studied and affirmed to be present with both investors and developers. However, a comparative study between investors and developers can be done to see the comparative effect on real estate prices, which shall give insights into the comparative importance of behavioural bias of investors and developers or buyers and initial sellers.
Regret aversion
Regret is one of the most common feelings that is experienced in the investment sector, where investors often feel regret after they fail to capitalize on probable profit or incur a significant loss. It is human nature that they try to avoid regret by using any comforting means or thinking. Investors try to comfort themselves after a loss by applying a different way of thinking. This, however, has been shown to have detrimental effects afterwards where they tend to hold on to bad investments and amplify the loss later (M. J. Seiler et al., 2008 ).
The studies of Waweru et al. ( 2014 ), Pandey and Jessica ( 2018 ) and Hoxha and Hasani ( 2022 ) confirm the presence of regret aversion bias and how it affects investor decision-making. However, real estate prices do not seem to be impacted by regret aversion bias (Malik et al., 2021 ). A different viewpoint is given by Seiler and Seiler ( 2010 ) who studied regret aversion in the real estate market and concluded that a large majority of people do not show regret aversion bias and perceive a forgone profit as a larger issue than unawareness about a potential gain. They also present that single women tend to show higher regret aversion. Wangzhou et al. ( 2021 ) suggested that regret aversion bias affects risk perception, and financial literacy weakens the relationship between regret aversion and investment decision-making, indicating that people who tend to be financially more proficient can overcome the regret they feel and make more informed decisions rationally.
There has been limited research on regret aversion, and the modelling and analysis techniques have yet to be developed. Therefore, a more extensive sample study could be conducted to investigate this behavioural bias. Despite regret aversion being confirmed by some researchers when it comes to housing decisions, its effect on housing prices has only been studied once with a negative outcome. More research will likely be beneficial since housing decisions usually result in price predictions.
Herding behaviour is a widespread phenomenon across investment regimes where many investors seem to follow other investors leading to poorer investment decisions and mass trading in one direction, causing market instability (Kinatta et al., 2022 ). Such behaviour exists in the housing market (Cascão et al., 2023 ) and the mortgage loan market (Martins et al., 2020 ). Housing prices have been said to be much more volatile like stock prices. Herding bias has been adjudged as a possible explanation for fluctuations in house prices (Hott, 2012 ) across 7 OECD countries. One possible factor behind herding behaviour is the adverse sentiment (Philippas et al., 2013 ) and social and normative influences which cause home buyers to herd (Susanto and Njo, 2020 ).
The importance of housing investment has been explained by Tan ( 2022 ) and Talpsepp et al. ( 2021 ). While studies could not find any correlation between gender and herding behaviour, people of younger age and older people had moderate to low levels of herding bias. Also, people of a commerce background tend to show low herding behaviour (Talpsepp and Tänav, 2021 ). Herding behaviour has been studied for different categories of people, and institutional investors have also been discovered to show herding behaviour (Lantushenko and Nelling, 2017 ). This behaviour is also demonstrated among real estate evaluators who seem to trust and move toward the valuations done by their peer group (Ali et al., 2020 ). An important finding is that the occurrence of such behaviour is not on the organization level but according to property type. Fund managers, however, do not exhibit this behaviour due to a highly volatile and performance-pressure market which causes them to be highly orthodox in their decision-making approach leading them away from herding behaviour (Lowies et al., 2016 ). Herding is observed to be on the lower side, with institutional investors and low uncertainty of asset value (Ngene and Gupta, 2022 ). The authors further suggest that since herding induces market volatility and instability, governments should implement policies and regulations to curtail this behaviour.
Herding is said to be present and varies across regimes, geographical regions, and market conditions (Ngene et al., 2017 ). Many researchers have tried to study this behaviour along with different market conditions to see the effect. Philippas et al. ( 2013 ) started the study in this direction using RIET and concluded that investors tend to show herding behaviour during a turbulent market, with it becoming stronger in a declining market (Zhou and Anderson, 2013 ). Further, the market crash caused strong herding behaviour. Such market conditions further caused positive and negative herding, where negative herding was observed during volatile markets and positive during the market crash regime (Babalos et al., 2015 ). This observation is confirmed by Akinsomi et al. ( 2018 ) who studied the same across three market regimes which yielded that herding behaviour was substantial during the low volatility regime, shifting from anti-herding during high volatility to low volatility. Ro et al. ( 2019 ) also confirm that herding is more robust in up than down market. A different viewpoint is suggested by Lin et al. ( 2018 ) who say that spurious herding behaviour is present during a rising market which is contradictory to previous works.
While herding bias has been studied for market investors, such a study has to be extended to other market players like developers, agents, and fund managers. Such a study could further pave the way for determining the effect of herding bias on the pricing of properties. Another future direction could be to exploit the findings that suggest that herding is related to the education level of investors to find the relationship with property type to see if the level of education causes any moderation. In contrast, investors are segregated based on the property type they are dealing in. Martins et al. ( 2020 ) suggest that Disaster Myopia is observed in some European countries, so it can be intriguing to study whether such short-sightedness for favourable market conditions has changed before and after the pandemic. Two more avenues for future work in this area could be to see the effect of financial literacy on herding behaviour as Talpsepp et al. ( 2021 ) suggest that people with commerce education tended to be less prone to herding due to their knowledge of finance and assessing the relation of herding with a population of the region concerned in order to explore the possibility as suggested by Ngene and Gupta ( 2022 ).
Overconfidence
Investors often judge their abilities to be superior or more capable than they are, which affects investment intentions and often leads to bad transactions and losses (Hoxha and Hasani, 2022 ). This cognitive bias has thus been studied to determine the effect on real estate investment decisions. Many researchers have used REIT instead of real estate transactions to study this factor. Eichholtz and Yönder ( 2015 ) have studied this bias for the first time, exploring the effect of overconfidence on trading activity and investments in real estate. They considered investor trading and holding decisions on behalf of the study objectives and concluded that overconfident CEOs tend to invest more than their counterparts and sell less. In the investment arena, it is important that investors can predict the return they expect, and thus different methods are used to do that. On comparing dividend growth theory with overconfidence and self-attribution bias, it was found that the latter affects return predictability more than the former (Chen et al., 2022 ). Not only investors but property evaluators also tend to show more than required confidence in their abilities when evaluating the price of a property (Ali et al., 2020 ). This also causes variations in the valuations done by different evaluators for a similar property (Lee et al., 2022 ).
Housing prices have been another important area that has been studied in relation to overconfidence bias to see the cause–effect relationship. Hwang et al. ( 2020 ) confirm that overconfidence in UK households is present with significant evidence. Malik et al. ( 2021 ) affirm the effect of investors’ overconfidence in real estate prices. Income has been studied as an important demographic variable that affects overconfidence, and results show that higher income causes higher levels of overconfidence among investors (Cascão et al., 2023 ).
While most of the studies have been unanimous on the fact that overconfidence has some effect on investment attributes, Pandey and Jessica ( 2018 ) differ and conclude that it does not significantly influence property investment decisions. Overconfidence bias has been challenging to study for real estate due to the requirement of many high-frequency transactions, which are not easy to find in this sector. Also, these conditions cannot be simulated in laboratories. These things have contributed to the barriers in studies for this bias (Bao and Li, 2016 ).
Education and annual income of investors have been studied and affirmed to be important factors in behavioural biases. It can thus be interesting to see the effect of these two factors on overconfidence. Further, the overconfidence factors like overestimation and over placement apart from over precision, can be included in the proposed model (Hwang et al., 2020 ). To provide some direction to the work of Pandey and Jessica ( 2018 ) a larger sample could be used to test the IRT model since, for more robust findings through IRT, a sample size of 500–1000 is recommended (Finch and French, 2018 ) and the considered sample size in their work is 543 which is on the lower threshold. This can be interesting because their work contradicts other works’ findings, suggesting that overconfidence has a significant influence.
Other biases
Apart from the biases discussed above, the rest of the 10 biases have been studied less and thus have very little research with respect to real estate investments. Availability bias is another bias that is related to information availability. It explains that people tend to give weight to the most easily and readily available information for making decisions. Investment decisions in real estate are also not diverged from this bias, and they do get influenced by availability heuristics (Cascão et al., 2023 ). However, Cascão et al. ( 2023 ) concluded in their study that the effect is not fully confirmed due to the low variance of the model they hypothesized. Another interesting finding of their work was that women tend to display higher availability heuristics. Such dependency on readily available information causes people to be more sensitive towards price. Along with women, investors dealing in local properties also showed higher levels of availability bias (Waweru et al., 2014 ). The effect of time was considered in accordance with availability bias and while comparing the government-supported appraised prices (Capital Value) and actual sales price (Transacted Price), it was found that the effect of information diminished over time which supported the fact that availability bias exists and readily available information is preferred (Cheung et al., 2022 ). Such dependency on easily available information represents the myopic behaviour of investors which means that they are short-sighted in decision-making and consider only what is available to them within easy reach (M. Seiler et al., 2008 ; Pandey and Jessica, 2018 ).
Investors also tend to display confirmation bias wherein they want confirmation from their friends or colleagues over the fair price they have adjudged. They then tend to form an anchor or reference point around that price (Ali et al., 2020 ; Lee et al., 2022 ). However, this bias was said to be negligible among the professional property surveyors in a study conducted in the UK (Gallimore, 1996 ).
The presentation of information to investors also plays a crucial role in decision-making and investment intention in the form of framing bias (Hoxha and Hasani, 2022 ). It has been said that investors seem to perceive a similar piece of information differently if it is presented in a positive or in a negative way. Jin and Gallimore ( 2010 ) and Levy et al. ( 2020 ) established in their study that investors perceive a decrease in price to be more and a similar increase in price to be less if the information is presented to them in a negative or positive manner, respectively. They postulated that such asymmetry got exaggerated based on the property’s nearness to the investor. Kinatta et al. ( 2022 ) gave a different perspective on framing bias and said that investors who tend to analyse and iterate given information in different ways, such as to get different perspectives, tend to make better judgements and decisions.
Investors often tend to predict the outcome of certain events and expect higher than actual returns. They also tend to predict the outcomes based on the probability of return of prices from higher to lower and vice versa. This expectation of higher-than-normal returns affects the decision-making of real estate investors (Malik et al., 2021 ). However, this bias has also been studied less in the real estate investment environment. Apart from the work of Malik et al. ( 2021 ), other researchers have not found very conclusive evidence of gambler’s fallacy to be of importance. While Waweru et al. ( 2014 ) found that the gambler’s fallacy has a shallow impact, other works did not find any significant impact on the decision-making of real estate investors (Pandey and Jessica, 2018 ; Cascão et al., 2023 ).
Representativeness is a similar kind of behavioural bias where investors again try to predict the outcome of a certain event or investment based on the outcome of any event of a similar nature that has happened in the past. It is usually related to overstressing the good outcome of a past event and believing that another event of similar nature will also result in a good outcome (Cascão et al., 2023 ). This factor has been found to be one of the most dominant factors that affect the real estate decision-making process (Waweru et al., 2014 ; Pandey and Jessica, 2018 ; Hoxha and Hasani, 2022 ).
People often tend to compartmentalize their minds to treat different assets and investments differently and individually. Such compartmentalization can lead them to make improper judgements and losses. This happens when people allocate resources to specific assets without comparing or studying the interrelation with other options. It has been found to be negatively but significantly related to decision-making in real estate investment (Kinatta et al., 2022 ). This confirms the findings (Seiler et al., 2012 ; Waweru et al., 2014 ). The only divergence found is in the study of Pandey and Jessica ( 2018 ) who conclude that mental accounting does not have a significant impact.
Sellers try to sell their assets at places and times when return expectancy is high. This has been described as a disposition effect in behavioural biases. Quan ( 2002 ) posits that sellers will flock to places where they are most likely to get good buyers. He examined the difference between an auction and direct sales and found that buyers with higher search costs preferred auctions, while buyers with lower costs preferred direct sales. Thus sellers who desired better payoffs went to auctions. While most people tend to sell their property when returns are high, some sellers are willing to sell the property at the break-even point (Seiler et al., 2012 ). This also cascades down to investors’ willingness to sell a property based on the return. They tend to sell those properties that bring in more return but do not like selling those that have not performed well (Crane and Hartzell, 2010 ).
Some biases are linked to the psychological closeness of the investor with the property. People who look for investment or purchase a house intended for their stay make decisions differently due to emotional attachments. The same goes for sellers who attach value to the property if they stay there. This effect is known as the endowment effect (Tan, 2022 ). Investors also tend to invest in properties or areas known to them and avoid venturing into the unknown, thus displaying familiarity bias (Seiler et al., 2013 ). The limitation of the market thus causes hurdles in diversification and causes an increase in property prices in an area (Henneberry and Mouzakis, 2014 ). The owners who have properties with less current value as compared to past purchase prices suffer from familiarity bias and do not want to list them for sale at lower prices because they feel they are more familiar with the market (Lane et al., 2011 ). Also, as discussed for framing bias asymmetry in the perception of information based on the way of presenting it, this bias gets exaggerated if the investor is more familiar with the market, thus showing familiarity bias (Levy et al., 2020 ). This familiarity bias has sometimes been diffused into home bias, where investors prefer local markets. As seen in the study of Gibilaro and Mattarocci ( 2016 ), African and Asian countries tend to show higher levels of home bias and thus the least diversified portfolio. However, home bias does not affect returns to a great extent (Gaar et al., 2022 ). More experienced investors, however, may go to global markets or leave local markets when they perform poorly (Wright and Yanotti, 2019 ; Florentsen et al., 2020 ).
These biases, however, have been studied less, and therefore much future work can be done for better understanding. One such avenue is to explore different investor types, like institutional investors, developers, etc., so that a wider perspective is received. Also, such studies can be introduced again from time to time for the same sample so that the change in behaviour over time can be recorded and analysed. The latest and advanced statistical techniques can be evaluated with present study designs to validate the findings even more. In totality, these biases are yet open for expansive studies and explorations.
Results and discussion
The literature review of 86 studies examined behavioural biases in real estate investment. This section presents a few findings from the study that give a broad overview of the characteristics of existing literature in this field. There are also certain technological difficulties and research voids that call for additional contributions from the researchers.
Following an examination of the articles, it was found that there has been a significant increase in the number of articles looking at behavioural biases in real estate investment. Real estate has recently gained much attention as an investment sector. There could be various reasons attributed to this, wiz. It provides much diversity in an investment portfolio and is a physical asset. Also, behavioural analysis is important since real estate deals do not have tools, analysis charts, or statistical indicators in abundance like the stock market for example. Moreover, factors like emotional attachment to a dwelling place and housing being a roof over the head play a crucial role in decision-making.
Furthermore, the number of real estate investors has significantly increased in emerging economies in recent years. According to the India Investment Grid (IIG), the Indian real estate industry is expected to reach USD 1 trillion by 2030. Given a steep rise in real estate investment, there is a need to examine the behavioural biases of real estate investors in these countries. However, it is imperative to note that the aforementioned survey of the literature demonstrates that in the past, the majority of research work was undertaken to examine the behavioural biases in real estate investment, focused on developed countries. A possible explanation for this might be that the real estate market of developed countries is more organized, and data is easily available for research.
Upon analysing the number of articles for the biases identified, we see that anchoring bias has the most number of studies (37), followed by loss aversion (24) and herding behaviour (18). The rest of the biases have a very low number of studies to their name. While many studies on some biases have helped a great deal in deeper understanding, future works should target the unexplored biases and their interplay with the other deeper studied ones. Regression has been one of the most widely used statistical techniques in the examined studies and it has been time and again used extensively for establishing the relationships between two variables of interest. However, integrating real-life scenarios often leads to the formation of complex models due to the deeper interplay of many variables. Such complexities could be handled better using newer statistical techniques like PLS-SEM or IRT, as has been used in some studies. Therefore, future studies may use these techniques to give a more robust model that fits closer to real-life situations.
The factors that contribute to anchoring bias are initial listing price, government-influenced appraisals of properties, and the distance of the property from the investor (Cheung et al., 2022 ; Clauretie and Thistle, 2007 ; Northcraft and Neale, 1987 ; Pandey and Jessica, 2018 ). Such factors sway the bias of an investor towards a specific decision, and thus, anchoring heuristics influences both decision-making and property prices. Among the demographics, the age of investors, income group, and gender (women) tend to influence the anchoring bias (Hjalmarsson and Österholm, 2021 ; Kucharska-Stasiak, 2018 ). Future research should examine these factors in interrelation with each other to get a more robust and closer-to-real-world scheme of things.
Investors often treat loss and gains separately and thus hold on to poorly performing assets to mitigate the losses. Such effect was found to be more predominant for commercial real estate and with real estate developers (BAO and LI, 2016 ; Li and Wan, 2021 ). Loss aversion bias also gets affected by time horizon, and when investors have ample time to liquidate or sell an asset, they tend to wait longer, amplifying their losses (Buisson, 2016 ). Future studies could focus on exploiting this time horizon effect on different investor types and private firms which show higher loss aversion behaviour (Bao et al., 2021 ). The interconnection between investors and developers should also give interesting insights since the biased decision-making of developers magnifies the effect due to control over listing prices.
While it is suggested that regret aversion is an important factor in decision-making (Waweru et al., 2014 ; Pandey and Jessica, 2018 ), many researchers could not find any significant influence over decision-making and real estate prices (Malik et al., 2021 ; Seiler and Seiler, 2010 ). The review finds that regret aversion is studied quite less, and thus, very few models are available to conclude the effects. Future studies with larger samples and more factors could give a higher understanding and generalized results.
Herding behaviour has been an important bias not only in the real estate sector but more predominantly in stock markets. It has been confirmed to cause price fluctuations (Hott, 2012 ) with the bias being more prevalent during volatile or turbulent market conditions (Zhou and Anderson, 2013 ). Even institutional investors tend to show herding behaviour (Lantushenko and Nelling, 2017 ; Ngene and Gupta, 2022 ). Such behaviour can be attributed to people’s tendency to lose confidence in their understanding when conditions are adverse and prone to follow others. This is justified by the finding that people in commerce tend to herd less due to a higher understanding of financial situations. Future suggested works could thus see the effect of financial literacy and understanding on herding behaviour along with different market players like fund managers, developers, and buyers.
Investors tend to be overconfident in their knowledge or intuition of any transaction or investment which, often leads to losses. Firm owners with higher levels of overconfidence were found to sell less and buy more (Eichholtz and Yönder, 2015 ). Also, people with higher incomes tend to show higher levels of overconfidence (Cascão et al., 2023 ). Such behaviour could be explained by the fact that people tend to believe that their investments will reap larger than actual benefits, which causes them to liquidate their assets less and hold on to bad investments. Going by the effect of education as concluded in other biases, it could be interesting to see in future studies how education affects overconfidence along with the level of income.
Apart from the above-discussed biases, other biases have been studied less, and thus findings are yet to become conclusive without backing from more independent studies. Regarding availability bias, researchers have demonstrated that it affects investment decisions because it shows investors’ short-sightedness when they focus on easily available information (Pandey and Jessica, 2018 ). Moreover, the bias gets affected by time since the information is available and whether the investors are dealing in local or non-local properties (Waweru et al., 2014 ; Cheung et al., 2022 ). The assurance that investors get from asking their friends or colleagues also takes the shape of confirmation bias, which also facilitates anchoring bias (Ali et al., 2020 ). Gambler’s fallacy also has not been supported by many researchers (Waweru et al., 2014 ; Pandey and Jessica, 2018 ; Cascão et al., 2023 ) with only one study confirming the presence (Malik et al., 2021 ). Mental accounting has been shown to affect decision-making negatively (Kinatta et al., 2022 ). The three biases, namely, familiarity bias, the endowment effect, and home bias, are related to the closeness of the property to the investor, both emotionally and physically. The limited works in these avenues indicate that investors tend to be more interested in local properties (Seiler et al., 2013 ; Wright and Yanotti, 2019 ). Such findings can be attributed to the fact that people are more familiar with what they are close to regularly, and also, they are privy to much information that can be difficult for people who are far away. Framing variation has been shown to have positive outcomes (Kinatta et al., 2022 ) owing to the fact that it is always better to analyse a particular situation from different perspectives. Lastly, the disposition effect has been stated to be present where investors are always likely to sell a property when the return is significantly higher (Seiler et al., 2012 ). However, the findings cannot be termed very conclusive due to the lack of more studies. The future arena for these biases is wide open due to the lack of work. These future studies could utilize the interrelationships studied in other more avidly studied biases and venture into the underlying factors. Also, studies can be repeated over time to analyse the change in behaviour and check the time factor in the existing models.
Conclusion and future scope
Real estate investment has significantly gained momentum in recent years. It has been judged as a significant diversification in the portfolio of investors. Moreover, real estate has been emotionally important for people since they consider a roof over their heads necessary. Investors’ decision-making for real estate has been similar to other investments, leading to the study of behaviour and associated biases. Behavioural finance explains that people do not always make rational decisions based on numbers and facts. More often, they make irrational decisions based on their beliefs or understanding, which causes bias towards particular factors. Even though behavioural biases play a crucial role in decision-making, the study related to the effect of such biases in the perspective of real estate is still not abundant. Most of the focus has been on stock markets, and many of the identified biases have very little to negligible studies in their name. This has led the authors to systematically review the existing works related to behavioural biases in real estate investment to provide an excerpt of the works conducted. A systematic review of published research from 1980 to 2022 is presented in this study. Besides reviewing identified papers, the authors provide directions for future research in this area and shed light on the research gap in this area. In order to identify relevant studies, the PRISMA model was used, which resulted in 86 articles being included in the review. Various biases have been studied in detail with an analysis of the factors that influence those biases and their effect on various real estate parameters. The authors have provided explanations for the findings, identified which biases have been studied less, and provided future directions. Anchoring, loss aversion, regret aversion, herding, and overconfidence have been the most studied behavioural biases. In contrast, availability, gambler’s fallacy, representativeness, home bias, endowment effect, familiarity, mental accounting, and framing variation are less studied ones.
The studies identify initial listing price, previous purchase price, information accessibility, gender, age, government appraisals, and property distance from investors to be the most important factors influencing anchoring heuristics. Loss aversion is found to be more predominant in commercial real estate and seasoned investors affecting housing prices drastically if developers show loss aversion. Also, the time horizon is important, and investors with more time tend to be more loss-averse and sell late. Researchers have concluded that regret aversion influences decision-making but does not affect real estate prices. For herding bias, it was found that it has a great impact on housing price fluctuations and is more prominent during turbulent or volatile markets. Also, people having financial knowledge tend to show lesser herding behaviour. Coming to overconfidence, it was found that income affects overconfidence bias, and most of the researchers tended to use REIT to study this since physical real estate transactions are not so frequent to easily get the data. While other biases have also been studied and researchers provide some insights, the studies are too few for the authors to draw any conclusive evidence and make any comments. These biases do tend to show the effect of decision-making but there are yet open for more studies to support these findings.
This paper provides some important academic contributions to understand behavioural biases. It provides a comprehensive yet concise excerpt of the existing research works to give a wholesome understanding of the behavioural biases in real estate investment and the different factors affecting them. It also conceptualizes the effect of those biases on different parameters like housing prices and market conditions. This paper also presents some contributions to policy making. It shall help policymakers and real estate investment institutions strategize effectively to create an encouraging environment for real estate investors. As the study points out, the effect of different factors like closeness of the property from the investor, education level, and motives behind house purchase, investment institutions can develop programs that can help investors understand the rational point of view so that they can overcome the effect of biases to make correct decisions. Policymakers can make better policies like adequate valuation of property and limited time offers on financial instruments so that false reference points that can negatively affect the property price are minimized. Lastly, it can help investors to understand that they should evaluate the market information and rely on it more than their beliefs so that biases like herding behaviour and overconfidence are reduced.
Future researchers have an open area to explore the biases in real estate investment because human behaviour is a never-ending arena. Future works should consider the combined effect of various factors that affect the biases but have been studied individually. The real-life scenario will be much more complex as it will consist of multiple biases. Moreover, future studies should involve a multitude of players in this market like fund managers, actual sellers, buyers, institutional investors, and developers. This has been done in very few biases; the rest are yet to be explored. Apart from the future direction given for deeper analysis with respect to studied behavioural biases, there are a few suggestions that can align with the real estate landscape as a whole and help in a finer understanding of the underlying nuances. Firstly, future studies should concentrate on real estate investment in developing countries like India where there is a higher potential for growth and investors are more inclined towards real estate investment. Secondly, researchers should identify the biases according to the type of property, to get an idea of whether the investors of residential and commercial property act similarly or they are different. Further, future works should utilize more primary data as it is closer to reality and also real-time. While some biases like anchoring bias have a healthy distribution among primary and secondary, some biases like herding and loss aversion have a disproportionately high number of secondary data-based studies. Hence, primary data-based studies for these type of biases is necessary. Finally, besides direct real estate investment, biases of REIT investors should also be identified and they can be compared by the researcher. The rationale for doing such bifurcation is to get an idea of how investors’ behaviour differs when they invest in direct and securitized real estate. These directions should help potential researchers to expand the intellectual universe of behavioural biases and thus offer understanding for decision-making and investments.
Data availability
This is a review paper wherein the authors have analysed various articles by different authors who have been cited at required places. The cited information belongs to the authors mentioned in the reference section. The generated data is included in the paper.
Akinsomi O et al. (2018) Impact of volatility and equity market uncertainty on herd behaviour: evidence from UK REITs. J Eur Real Estate Res 11(2):169–186. https://doi.org/10.1108/JERER-06-2017-0021/FULL/HTML
Ali HM et al. (2020) Effects of behavioural uncertainties in property valuation. Int J Built Environ Sustain 7(3):69–79. https://doi.org/10.11113/IJBES.V7.N3.557
Article Google Scholar
Ambrose BW, Shen L (2023) Past experiences and investment decisions: evidence from real estate markets. J Real Estate Finance Econ 66(2):300–326. https://doi.org/10.1007/S11146-021-09844-2
Anenberg E (2011) Loss aversion, equity constraints and seller behavior in the real estate market. Reg Sci Urban Econ 41(1):67–76. https://doi.org/10.1016/J.REGSCIURBECO.2010.08.003
Anenberg E (2016) Information frictions and housing market dynamics. Int Econ Rev 57(4):1449–1479. https://doi.org/10.1111/IERE.12204
Article MathSciNet MATH Google Scholar
Apau R, Jeke L (2022) The effect of performance manipulation on fund flows under different market conditions in South Africa. Invest Manag Financ Innov 19(3):203–214. https://doi.org/10.21511/IMFI.19(3).2022.17
Arbel Y, Ben-Shahar D, Gabriel S (2014) Anchoring and housing choice: results of a natural policy experiment. Reg Sci Urban Econ 49:68–83. https://doi.org/10.1016/j.regsciurbeco.2014.07.004
Babalos V, Balcilar M, Gupta R (2015) Herding behavior in real estate markets: novel evidence from a Markov-switching model. J Behav Exp Finance 8:40–43. https://doi.org/10.1016/j.jbef.2015.10.004
Baker H, Ricciardi V (2015) How biases affect investor behaviour. Eur Financ Rev 7–10. https://papers.ssrn.com/sol3/papers.cfm?abstract_id=2457425 . Accessed 1 Sept 2023
Bao HXH, Li SH (2016) Overconfidence and real estate research: a survey of the literature. Singap Econ Rev 61(04):1650015. https://doi.org/10.1080/02673037.2021.1935767
Bao HXH, Saunders R (2021) Reference dependence in the UK housing market. Hous Stud 38(7):1191–1219. https://doi.org/10.1080/02673037.2021.1935767
Bao HXH, Meng CC, Wu J (2021) Reference dependence, loss aversion and residential property development decisions. J Hous Built Environ 36(4):1535–1562. https://doi.org/10.1007/S10901-020-09803-Y/TABLES/7
Basana SR, Tarigan ZJH (2022) The effect of essential information and disposition effect on shifting decision investment. Accounting 8(2):227–234. https://doi.org/10.5267/j.ac.2021.6.015
Benartzi S, Thaler RH (1995) Myopic Loss Aversion and the Equity Premium Puzzle. Q J Econ 110(1):73–92. https://doi.org/10.2307/2118511
Beracha E, Skiba H (2014) Real Estate Investment Decision Making in Behavioral Finance. In Investor Behavior (pp. 555–572). John Wiley & Sons, Ltd. https://doi.org/10.1002/9781118813454.ch30
Beracha E, Wintoki MB (2013) Forecasting residential real estate price changes from online search activity. J Real Estate Res 35(3):283–312. https://doi.org/10.1080/10835547.2013.12091364
Bhutta N, Dokko J, Shan H (2017) Consumer ruthlessness and mortgage default during the 2007 to 2009 housing bust. J Finance 72(6):2433–2466. https://doi.org/10.1111/JOFI.12523
Bihari A et al. (2023) Does cognitive biased knowledge influence investor decisions? An empirical investigation using machine learning and artificial neural network. VINE J Inf Knowl Manag Syst https://doi.org/10.1108/VJIKMS-08-2022-0253/FULL/PDF
Black R, Diaz J (1996) The use of information versus asking price in the real property negotiation process. J Prop Res 13(4):13–287. https://doi.org/10.1080/095999196368808
Bokhari S, Geltner D (2011) Loss aversion and anchoring in commercial real estate pricing: empirical evidence and price index implications. Real Estate Econ 39(4):635–670. https://doi.org/10.1111/j.1540-6229.2011.00308.x
Bucchianeri GW, Minson JA (2013) A homeowner’s dilemma: anchoring in residential real estate transactions. J Econ Behav Organ 89:76–92. https://doi.org/10.1016/J.JEBO.2013.01.010
Buisson F (2016) Prospect theory and loss aversion in the housing market. J Real Estate Res 38(2):229–249. https://doi.org/10.1080/10835547.2016.12091444
Burns T, Berelson B (1952) Content analysis in communication research. British J Sociol 4(1). https://doi.org/10.2307/587175
Carrillo PE (2013) To sell or not to sell: measuring the heat of the housing market. Real Estate Econ 41(2):310–346. https://doi.org/10.1111/REEC.12003
Cascão A, Quelhas AP, Cunha AM (2023) Heuristics and cognitive biases in the housing investment market. Int J Hous Mark Anal 16(5):991–1006. https://doi.org/10.1108/IJHMA-05-2022-0073
Chang C-C, Chao C-H, Yeh J-H (2016) The role of buy-side anchoring bias: evidence from the real estate market. Pac-Basin Finance J 38:34–58. https://doi.org/10.1016/j.pacfin.2016.02.008
Chaudary S (2019) Does salience matter in investment decision?: differences between individual and professional investors. Kybernetes 48(8):1894–1912. https://doi.org/10.1108/K-09-2018-0490/FULL/HTML
Chen TY, Huang GY, Wu ZX (2022) Overreaction-based momentum in the real estate investment trust market. Int Rev Finance 22(3):453–471. https://doi.org/10.1111/IRFI.12358
Cheung K, Yiu C, Gaun Y (2022) Homebuyer purchase decisions: are they anchoring to appraisal values or market prices? J Risk Financ Manag 15(4) https://doi.org/10.3390/jrfm15040159
Clapp JM, Lu-Andrews R, Zhou T (2020) Anchoring to purchase price and fundamentals: application of salience theory to housing cycle diagnosis. Real Estate Econ 48(4):1274–1317. https://doi.org/10.1111/1540-6229.12259
Clauretie TM, Thistle PD (2007) The effect of time-on-market and location on search costs and anchoring: the case of single-family properties. J Real Estate Finance Econ 35(2):181–196. https://doi.org/10.1007/S11146-007-9034-X
Coën A, Simon A, Zaiter S (2021) Why is there a home bias? An analysis of US REITs geographic concentration. Finance 42(1):111–154. https://doi.org/10.3917/fina.421.0111
Crane AD, Hartzell JC (2010) Is there a disposition effect in corporate investment decisions? Evidence from real estate investment trusts. SSRN Electron J https://doi.org/10.2139/ssrn.1031010
Crosby N et al. (2018) Can institutional investors bias real estate portfolio appraisals? Evidence from the market downturn. J Bus Ethics 147(3):651–667. https://doi.org/10.1007/S10551-015-2953-1/TABLES/6
D’Lima W, Schultz P (2021) Residential real estate investments and investor characteristics. J Real Estate Finance Econ 63(3):354–393. https://doi.org/10.1007/S11146-020-09771-8
De Bondt, WFM, Thaler, RH (1995) Financial Decision-Making in Markets and Firms: A Behavioral Perspective. In Handbooks in Operations Research and Management Science (Vol. 9, Issue C, pp. 385–410). Elsevier. https://doi.org/10.1016/S0927-0507(05)80057-X
Dervishaj B (2021) Psychological biases, main factors of financial behaviour—a literature review. Eur J Med Nat Sci 4(1):27–44 https://revistia.com/index.php/ejmn/article/view/5038
Google Scholar
Diaz J, Hansz J (2001) The use of reference points in valuation judgment. J Prop Res 18(2):141–148. https://doi.org/10.1080/09599910110039897
Diaz J, Zhao R, Black R (1999) Does contingent reward reduce negotiation anchoring?. J Prop Invest Finance 17(4):374–379. https://doi.org/10.1108/14635789910271764/FULL/HTML
van Dijk DW, Geltner DM, van de Minne AM (2022) The dynamics of liquidity in commercial property markets: revisiting supply and demand indexes in real estate. J Real Estate Finance Econ 64(3):327–360. https://doi.org/10.1007/S11146-020-09782-5
Eichholtz P, Yönder E (2015) CEO overconfidence, REIT investment activity and performance. Real Estate Econ 43(1):139–162. https://doi.org/10.1111/1540-6229.12054
Engelhardt GV (2003a) Nominal loss aversion, housing equity constraints, and household mobility: evidence from the United States. J Urban Econ 53(1):171–195. https://doi.org/10.1016/S0094-1190(02)00511-9
Engelhardt GV (2003b) Nominal loss aversion, housing equity constraints, and household mobility: evidence from the United States. J Urban Econ 53(1):171–195. https://doi.org/10.1016/S0094-1190(02)00511-9
Enslin Z, Hall J, du Toit E (2022) The susceptibility of management accountants to framing bias. Meditari Account Res 31(7):133–155. https://doi.org/10.1108/MEDAR-02-2021-1185/FULL/ZACK.ENSLIN
Fama (1970) Efficient capital markets: a review of theory and empirical work. J Finance 25(2). https://doi.org/10.2307/2325488
Finch WH, French BF (2018) Introduction to educational and psychological measurement. In: Educational and psychological measurement. Routledge, pp. 1–10
Florentsen B et al. (2020) Turning local: home-bias dynamics of relocating foreigners. J Empir Finance 58:436–452. https://doi.org/10.1016/j.jempfin.2020.07.006
French K, Poterba J (1991) Investor diversification and international equity markets. Amn Econ Rev 81:222–226. https://doi.org/10.3386/w3609
Fumo N, Rafe Biswas MA (2015) Regression analysis for prediction of residential energy consumption. Renew Sustain Energy Rev 47:332–343. https://doi.org/10.1016/j.rser.2015.03.035
Gaar E, Scherer D, Schiereck D (2022) The home bias and the local bias: a survey. Manag Rev Q 72(1):21–57. https://doi.org/10.1007/S11301-020-00203-8
Gallimore P (1996) Confirmation bias in the valuation process: a test for corroborating evidence. J Prop Res 13(4):261–273. https://doi.org/10.1080/095999196368781
Genesove D, Mayer C (2001) Loss aversion and seller behavior: evidence from the housing market. Q J Econ 116(4):1233–1260. https://doi.org/10.1162/003355301753265561
Article MATH Google Scholar
Gibilaro L, Mattarocci G (2016) Are home-biased REITs worthwhile? J Real Estate Portf Manag 22(1):19–30. https://doi.org/10.1080/10835547.2016.12089977
Goldstein M, Chatterjee S, Price B (1979) Regression analysis by example J R Stat Soc Ser A (General) 142(4):512. https://doi.org/10.2307/2982566
Gong C, Lizieri C, Bao H (2019) Smarter information, smarter consumers”? Insights into the housing market. J Bus Res 97:51–64. https://doi.org/10.1016/j.jbusres.2018.12.036
Greenaway-McGrevy R, Haworth C (2020) Loss aversion in New Zealand housing. N Z Econ Pap 54(2):138–160. https://doi.org/10.1080/00779954.2019.1631877 . pp
Gutmann J (2014) Qualitative research practice: a guide for social science students and researchers (2nd edn) Int J Market Res 56(3):407
Hayunga DK, Pace RK (2017) List prices in the US housing market. J Real Estate Finance Econ 55(2):155–184. https://doi.org/10.1007/S11146-016-9555-2
Henneberry J, Mouzakis F (2014) Familiarity and the determination of yields for regional office property investments in the UK. Reg Stud 48(3):530–546. https://doi.org/10.1080/00343404.2013.765556
Hjalmarsson E, Österholm P (2021) Anchoring in surveys of household expectations Econ Lett 198:109687. https://doi.org/10.1016/j.econlet.2020.109687
Hott C (2012) The influence of herding behaviour on house prices. J Eur Real Estate Res 5(3):177–198. https://doi.org/10.1108/17539261211282046/FULL/HTML
Hoxha V, Hasani I (2022) Decision-making biases in property investments in Prishtina, Kosovo. J Prop Invest Finance 41(2):155–181. https://doi.org/10.1108/JPIF-04-2022-0031/FULL/
Hwang S, Cho Y, Shin J (2020) The impact of UK household overconfidence in public information on house prices. J Prop Res 37(4):360–389. https://doi.org/10.1080/09599916.2020.1790631
Jain J et al. (2022) Behavioural biases affecting investors’ decision-making process: a scale development approach. Manag Res Rev 45(8):1079–1098. https://doi.org/10.1108/MRR-02-2021-0139/FULL/HTML
Jain R, Jain P, Jain C (2015) Behavioral biases in the decision making of individual investors. IUP J Manag Res 14(3) https://search.ebscohost.com/login.aspx?direct=true&profile=ehost&scope=site&authtype=crawler&jrnl=09729216&AN=108710890&h=YDtHlqOtZTF7Ar5Z9p5sTMp7H%2BzwbxWy8n%2F7kRaXqx508FcwnThbs%2F4ANFTIlsN6OzvAE8LBFt53tYsVcyiA4Q%3D%3D&crl=c
Jin C, Gallimore P (2010) The effects of information presentation on real estate market perceptions. J Prop Res 27(3):239–246. https://doi.org/10.1080/09599916.2010.518404
Kahneman D, Tversky A (1979) On the interpretation of intuitive probability: a reply to Jonathan Cohen. Cognition 7(4):409–411. https://doi.org/10.1016/0010-0277(79)90024-6
Kahneman D, Knetsch JL, Thaler RH (1990) Experimental tests of the endowment effect and the coase theorem. J Political Econ 98(6):1325–1348. https://doi.org/10.1086/261737
Kengatharan L, Kengatharan N (2014) The influence of behavioral factors in making investment decisions and performance: study on investors of Colombo Stock Exchange, Sri Lanka Asian J Finance Account 6(1):1. https://doi.org/10.5296/ajfa.v6i1.4893
Kinatta MM et al. (2022) Cognitive bias, intuitive attributes and investment decision quality in commercial real estate in Uganda. J Prop Invest Finance 40(2):165–187. https://doi.org/10.1108/JPIF-11-2020-0129
Klamer P, Bakker C, Gruis V (2017) Research bias in judgement bias studies—a systematic review of valuation judgement literature. J Prop Res 34(4):285–304. https://doi.org/10.1080/09599916.2017.1379552
Kraus S, Breier M, Dasí-Rodríguez S (2020) The art of crafting a systematic literature review in entrepreneurship research. Int Entrep Manag J 16(3):1023–1042. https://doi.org/10.1007/S11365-020-00635-4
Krause A, Lipscomb CA (2016) The data preparation process in real estate: guidance and review. J Real Estate Pract Educ 19(1):15–42. https://doi.org/10.1080/10835547.2016.12091756
Kucharska-Stasiak E (2018) 15 Myths about market value. Real Estate Manag Valuat 26(3):113–121. https://doi.org/10.2478/REMAV-2018-0030
Kumar A, Vergara-Alert C (2020) The effect of financial flexibility on payout policy. J Financ Quant Anal 55(1):263–289. https://doi.org/10.1017/S002210901800114X
Kumar S, Goyal N (2015) Behavioural biases in investment decision making—a systematic literature review’. Qual Res Financ Markets 7(1):88–108. https://doi.org/10.1108/QRFM-07-2014-0022/FULL/HTML
Lambson VE, McQueen GR, Slade BA (2004) Do out-of-state buyers pay more for real estate? An examination of anchoring-induced bias and search costs. Real Estate Econ 32(1):85–126. https://doi.org/10.1111/J.1080-8620.2004.00085.X
Lane M, Seiler M, Seiler V (2011) Identifying behavioral explanations for a subset of the real estate shadow market. J Hous Res 20(2):191–210. https://doi.org/10.1080/10835547.2011.12092044
Lantushenko V, Nelling E (2017) Institutional property-type herding in real estate investment trusts. J Real Estate Finance Econ 54(4):459–481. https://doi.org/10.1007/S11146-016-9553-4
Lee C-C et al. (2022) The impacts of task complexity, overconfidence, confirmation bias, customer influence, and anchoring on variations in real estate valuations. Int J Strateg Prop Manag 26(2):141–155. https://doi.org/10.3846/ijspm.2022.16704
Leung TC, Tsang KP (2013b) Can anchoring and loss aversion explain the predictability of housing prices? Pac Econ Rev 18(1):41–59. https://doi.org/10.1111/1468-0106.12009
Leung TC, Tsang KP (2013a) Anchoring and loss aversion in the housing market: implications on price dynamics. China Econ Rev 24(1):42–54. https://doi.org/10.1016/J.CHIECO.2012.10.003
Levy DS, Frethey-Bentham C, Cheung WKS (2020) Asymmetric framing effects and market familiarity: experimental evidence from the real estate market. J Prop Res 37(1):85–104. https://doi.org/10.1080/09599916.2020.1713858
Li L, Wan WX (2021) The effect of expected losses on the Hong Kong property market. J Real Estate Finance Econ https://doi.org/10.1007/s11146-021-09851-3
Li N et al. (2022) Housing safety and health academic and public opinion mining from 1945 to 2021: PRISMA, cluster analysis, and natural language processing approaches. Front Public Health 10. https://doi.org/10.3389/fpubh.2022.902576
Liberati A et al. (2009) The PRISMA statement for reporting systematic reviews and meta-analyses of studies that evaluate health care interventions: explanation and elaboration. J Clin Epidemiol 62(10):e1–e34. https://doi.org/10.1016/J.JCLINEPI.2009.06.006
Article PubMed Google Scholar
Lin M, Viswanathan S (2016) Home bias in online investments: an empirical study of an online crowdfunding market. Manag Sci 62(5):1393–1414. https://doi.org/10.1287/MNSC.2015.2206
Lin W-Y, Wu M-H, Chen M-C (2018) Asymmetry herding behavior of real estate investment trusts: evidence from information demand. J Risk 21(2):99–137. https://doi.org/10.21314/JOR.2018.398
Ling DC, Naranjo A, Petrova MT (2018) Search costs, behavioral biases, and information intermediary effects. J Real Estate Finance Econ 57(1):114–151. https://doi.org/10.1007/S11146-016-9582-Z
Liu X et al. (2018) Listing strategies and housing busts: cutting loss or cutting list price? J Hous Econ 43:102–117. https://doi.org/10.1016/j.jhe.2018.09.006
Lo AW (2012) Adaptive markets and the new world order (corrected May 2012). Financ Anal J 68(2):18–29. https://doi.org/10.2469/faj.v68.n2.6
Lowies GA, Hall JH, Cloete CE (2016) Heuristic-driven bias in property investment decision-making in South Africa. J Prop Invest Finance 34(1):51–67. https://doi.org/10.1108/JPIF-08-2014-0055/FULL/HTML
Malik M et al. (2021) Role of behavioral biases in real estate prices in Pakistan. Real Estate Manag Valuat 29(7):41–53. https://doi.org/10.2478/remav-2021-0005
Martins AM et al. (2020) House price dynamics and bank herding: European empirical evidence. J Real Estate Res 42(3):365–396. https://doi.org/10.1080/08965803.2020.1840897
Mayer C (2011) Housing bubbles: A survey. In Annual Review of Economics (Vol. 3, pp. 559–577). Annual Reviews. https://doi.org/10.1146/annurev.economics.012809.103822
Merton RC (1985) On the Current State of the Stock Market Rationality Hypothesis. In Macroeconomics and Finance: Essays in Honor of Franco Modigliani. https://dspace.mit.edu/bitstream/handle/1721.1/2125/SWP-1717-12868260.pdf
Mittal SK (2022) Behavior biases and investment decision: theoretical and research framework. Qual Res Financ Mark 14(2):213–228. https://doi.org/10.1108/QRFM-09-2017-0085/FULL/HTML
Muthuri RNDK, Senkubuge F, Hongoro C(2020) Determinants of happiness among healthcare professionals between 2009 and 2019: a systematic review Humanit Soc Sci Commun 7(1):1–14. https://doi.org/10.1057/s41599-020-00592-x
Muzindutsi P-F et al. (2023) The impact of investor sentiment on housing prices and the property stock index volatility in South Africa. Real Estate Manag Valuat 31(2):1–17. https://doi.org/10.2478/REMAV-2023-0009
Newell G (2016) The changing real estate market transparency in the European real estate markets. J Prop Invest Finance 34(4):407–420. https://doi.org/10.1108/JPIF-07-2015-0053/FULL/PDF
Ngene GM, Gupta R (2022) Impact of housing price uncertainty on herding behavior: evidence from UK’s regional housing markets. J Hous Built Environ 38(2):931–949. https://doi.org/10.1007/s10901-022-09975-9
Ngene GM, Sohn DP, Hassan MK (2017) Time-varying and spatial herding behavior in the US housing market: evidence from direct housing prices. J Real Estate Finance Econ 54(4):482–514. https://doi.org/10.1007/S11146-016-9552-5
Northcraft G, Neale M (1987) Experts, amateurs, and real estate: an anchoring-and-adjustment perspective on property pricing decisions. Organ Behav Hum Decision Process 39(1). https://doi.org/10.1016/0749-5978(87)90046-X
Nwuba CC, Egwuatu US, Salawu BM (2015) Client influence on valuation: valuers’ motives to succumb. J Prop Res 32(2):147–172. https://doi.org/10.1080/09599916.2015.1005117
Odean T et al. (1998) Are investors reluctant to realize their losses? J Finance 53(5):1775–1798. https://doi.org/10.1111/0022-1082.00072
Pandey R, Jessica VM (2018) Measuring behavioural biases affecting real estate investment decisions in India: using IRT’. Int J Hous Markets Anal 11(4):648–668. https://doi.org/10.1108/IJHMA-12-2017-0103/FULL/HTML
Paraschiv C, Chenavaz R (2011) Sellers’ and buyers’ reference point dynamics in the housing market. Hous Stud 26(3):329–352. https://doi.org/10.1080/02673037.2011.542095
Paul J et al. (2021) Scientific procedures and rationales for systematic literature reviews (SPAR‐4‐SLR). Int J Consum Stud 45(4). https://doi.org/10.1111/ijcs.12695
Petticrew M, Roberts H (2008) Systematic Reviews in the Social Sciences: A Practical Guide. In John Wiley & Sons. https://doi.org/10.1002/9780470754887
Philippas N et al. (2013) Herding behavior in REITs: novel tests and the role of financial crisis. Int Rev Financ Anal 29:166–174. https://doi.org/10.1016/j.irfa.2013.01.004
Pope D, Pope J, Sydnor J (2015) Focal points and bargaining in housing markets. Games Econ Behav 93:89–107. https://doi.org/10.1016/j.geb.2015.07.002
Quan DC (2002) Market mechanism choice and real estate disposition: search versus auction. Real Estate Econ 30(3):365–384. https://doi.org/10.1111/1540-6229.00043
Rencher AC (2005) A review of “methods of multivariate analysis, second edition”. IIE Trans 37(11):1083–1085. https://doi.org/10.1080/07408170500232784
Riff S, Yagil Y (2016) Behavioral factors affecting the home bias phenomenon: experimental tests. J Behav Finance 17(3):267–279. https://doi.org/10.1080/15427560.2016.1203324
Ro SH et al. (2019) Herding behavior among residential developers. J Real Estate Finance Econ 59(2):272–294. https://doi.org/10.1007/S11146-018-9675-Y
Article MathSciNet Google Scholar
Rust NA et al. (2017) Quantity does not always mean quality: the importance of qualitative social science in conservation research. Soc Nat Resour 30(10):1304–1310. https://doi.org/10.1080/08941920.2017.1333661
Rust N A, Hughes C (2018) Social Science Methods to Study Human-Cheetah Interactions. In Cheetahs: Biology and Conservation: Biodiversity of the World: Conservation from Genes to Landscapes (pp. 483–493). https://doi.org/10.1016/B978-0-12-804088-1.00035-6
Schulte KW, Rottke N, Pitschke C (2005) Transparency in the German real estate market. J Prop Investment Finance 23(1):90–108. https://doi.org/10.1108/14635780510575111/FULL/PDF
Seay MC et al. (2018) Characteristics of rental real estate investors during the 2000s. J Financ Couns Plan 29(2):369–382. https://doi.org/10.1891/1052-3073.29.2.369
Seiler M et al. (2008) Familiarity bias and the status quo alternative. J Hous Res 17(2):139–154. https://doi.org/10.1080/10835547.2008.12091988
Seiler MJ et al. (2008) Regret aversion and false reference points in residential real estate. J Real Estate Res 30(4):461–474. https://doi.org/10.1080/10835547.2008.12091229
Seiler MJ et al. (2013) Familiarity bias and perceived future home price movements. J Behav Finance 14(1):9–24. https://doi.org/10.1080/15427560.2012.737389
Seiler MJ, Seiler VL (2010) Mitigating investor risk-seeking behavior in a down real estate market. J Behav Finance 11(3):161–167. https://doi.org/10.1080/15427560.2010.507166
Seiler MJ, Seiler VL, Lane MA (2012) Mental accounting and false reference points in real estate investment decision making. J Behav Finance 13(1):17–26. https://doi.org/10.1080/15427560.2012.653293
Shefrin H, Statman M (1985) The disposition to sell winners too early and ride losers too long: theory and evidence. J Finance 40(3):777–790. https://doi.org/10.1111/j.1540-6261.1985.tb05002.x
Silva SD et al. (2019) Real estate list price anchoring and cognitive ability. Int J Hous Mark Anal 12(4):581–603. https://doi.org/10.1108/IJHMA-08-2018-0060/FULL/HTML
Sinha A, Shunmugasundaram V (2023) Behavioral factors influencing investment decisions: a systematic review using Prisma. Int J Account Finance Rev 14(1):40–52. https://doi.org/10.46281/IJAFR.V14I1.1968
Snyder H (2019) Literature review as a research methodology: an overview and guidelines. J Bus Res 104:333–339. https://doi.org/10.1016/J.JBUSRES.2019.07.039
Steegmans J, Hassink W (2018) Decreasing house prices and household mobility: an empirical study on loss aversion and negative equity. J Reg Sci 58(3):611–634. https://doi.org/10.1111/jors.12380
Susanto SA, Njo A (2020) First-home buyers and herding behavior in Surabaya, Indonesia. Int J Hous Mark Anal 13(3):393–411. https://doi.org/10.1108/IJHMA-04-2019-0041/FULL/HTML
Talpsepp T, Tänav A-L(2021) Do gender, age and education affect herding in the real estate market? J Behav Exp Finance 32:100571
Tan C (2022) A study of boundedly rational behaviour in housing choice: evidence from Malaysia. Int J Hous Mark Anal 15(5):1259–1274. https://doi.org/10.1108/IJHMA-08-2021-0094
Thaler R (1985) Mental accounting and consumer choice. Mark Sci 4(3):199–214. https://doi.org/10.1287/MKSC.4.3.199
Article ADS Google Scholar
Tu Y, Ong SE, Han YH (2009) Turnovers and housing price dynamics: evidence from Singapore condominium market. J Real Estate Finance Econ 38(3):254–274. https://doi.org/10.1007/S11146-008-9155-X
Wangzhou K et al. (2021) Effect of regret aversion and information cascade on investment decisions in the real estate sector: the mediating role of risk perception and the moderating effect of financial literacy. Front Psychol 12. https://doi.org/10.3389/FPSYG.2021.736753/FULL
Waweru N, Mwangi G, Parkinson J (2014) Behavioural factors influencing investment decisions in the Kenyan property market. Afro-Asian J Finance Account 4(1):26–49. https://doi.org/10.1504/AAJFA.2014.059500
Weixiang S et al. (2022) An empirical assessment of financial literacy and behavioral biases on investment decision: fresh evidence from small investor perception. Front Psychol 13. https://doi.org/10.3389/FPSYG.2022.977444/FULL
Wright D, Yanotti MB (2019) Home advantage: the preference for local residential real estate investment. Pac-Basin Finance J 57:101167. https://doi.org/10.1016/j.pacfin.2019.06.014
Yan J, Bao H (2018) A prospect theory-based analysis of housing satisfaction with relocations: field evidence from China. Cities 83:193–202. https://doi.org/10.1016/j.cities.2018.06.022
You T (2020) Behavioural biases and nonlinear adjustment: evidence from the housing market. Appl Econ 52(46):5046–5059. https://doi.org/10.1080/00036846.2020.1752902
Zain-ul-Abdin S et al. (2019) Real-estate investor’s psychology: heuristics and prospect factorsNANo ranking found for “Lahore Journal of Business”. Paradigms 13(1):1–6. https://doi.org/10.24312/19142130101
Zhang M et al. (2022) Moderating role of information asymmetry between cognitive biases and investment decisions: a mediating effect of risk perception. Front Psychol 13. https://doi.org/10.3389/FPSYG.2022.828956/FULL
Zhou J, Anderson RI (2013) An empirical investigation of herding behavior in the U.S. REIT market. J Real Estate Finance Econ 47(1):83–108. https://doi.org/10.1007/S11146-011-9352-X
Article CAS Google Scholar
Zhou X, Gibler K, Zahirovic-Herbert V (2015) Asymmetric buyer information influence on price in a homogeneous housing market. Urban Stud 52(5):891–905. https://doi.org/10.1177/0042098014529464
Zillante A, Read DC, Seiler MJ (2019) Using prospect theory to better understand the impact of uncertainty on real estate negotiations. J Real Estate Res 41(1):75–105. https://doi.org/10.1080/10835547.2019.12091521
Download references
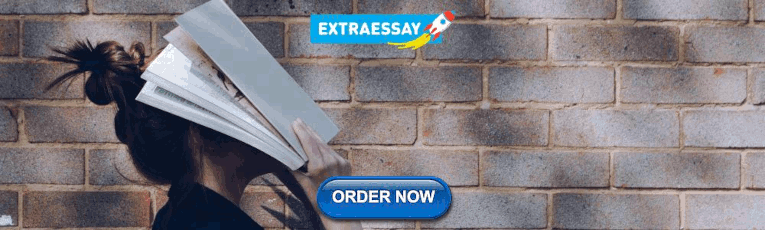
Author information
Authors and affiliations.
Department of Management Studies, Indian Institute of Information Technology, Allahabad, India
Akshita Singh, Shailendra Kumar & Utkarsh Goel
College of Administrative and Financial Sciences, Saudi Electronic University, Riyadh, Saudi Arabia
You can also search for this author in PubMed Google Scholar
Contributions
SK, UG and AJ worked towards idea generation and article search. AS was responsible for article selection and review, content analysis, and paper writing. All the authors carefully read the paper for final submission.
Corresponding author
Correspondence to Akshita Singh .
Ethics declarations
Competing interests.
The authors declare no competing interests.
Ethical approval
This article does not contain any studies with human participants performed by any of the authors.
Informed consent
Additional information.
Publisher’s note Springer Nature remains neutral with regard to jurisdictional claims in published maps and institutional affiliations.
Supplementary information
Rights and permissions.
Open Access This article is licensed under a Creative Commons Attribution 4.0 International License, which permits use, sharing, adaptation, distribution and reproduction in any medium or format, as long as you give appropriate credit to the original author(s) and the source, provide a link to the Creative Commons license, and indicate if changes were made. The images or other third party material in this article are included in the article’s Creative Commons license, unless indicated otherwise in a credit line to the material. If material is not included in the article’s Creative Commons license and your intended use is not permitted by statutory regulation or exceeds the permitted use, you will need to obtain permission directly from the copyright holder. To view a copy of this license, visit http://creativecommons.org/licenses/by/4.0/ .
Reprints and permissions
About this article
Cite this article.
Singh, A., Kumar, S., Goel, U. et al. Behavioural biases in real estate investment: a literature review and future research agenda. Humanit Soc Sci Commun 10 , 846 (2023). https://doi.org/10.1057/s41599-023-02366-7
Download citation
Received : 06 March 2023
Accepted : 07 November 2023
Published : 20 November 2023
DOI : https://doi.org/10.1057/s41599-023-02366-7
Share this article
Anyone you share the following link with will be able to read this content:
Sorry, a shareable link is not currently available for this article.
Provided by the Springer Nature SharedIt content-sharing initiative
Quick links
- Explore articles by subject
- Guide to authors
- Editorial policies

Real Estate Investment - Science topic

- Recruit researchers
- Join for free
- Login Email Tip: Most researchers use their institutional email address as their ResearchGate login Password Forgot password? Keep me logged in Log in or Continue with Google Welcome back! Please log in. Email · Hint Tip: Most researchers use their institutional email address as their ResearchGate login Password Forgot password? Keep me logged in Log in or Continue with Google No account? Sign up
- DOI: 10.3905/jpm.2003.319903
- Corpus ID: 36012987
Benefits of Real Estate Investment
- Georgi Georgiev , Bhaswar Gupta , T. Kunkel
- Published 31 January 2003
- Economics, Business
75 Citations
Real estate as part of an investment portfolio in australia.
- Highly Influenced
- 10 Excerpts
Investment Portfolios and Three Dimensions of Real Estate Investment: An Australian Perspective
Why real estate, re-examination of diversification benefits from foreign real estate investment: a canadian perspective, time-varying correlation between stock market returns and real estate returns, the atracttiveness of real estate investment in polish conditions, real estate and private equity: a review of the diversification benefits and some recent developments, real estate investment, time and risk diversification in real estate investments: assessing the ex post economic value, the allocation of real estate in an investment portfolio, 42 references, commercial real estate returns, the role of real estate in the portfolio allocation process, economic risk factors and commercial real estate returns, real estate is not normal: a fresh look at real estate return distributions, gains from diversifying into real estate: three decades of portfolio returns based on the dynamic investment model, real estate returns and inflation: an added variable approach.
- Highly Influential
Diversification Categories in Investment Real Estate
International real estate diversification: empirical tests using hedged indices, global real estate markets: cycles and fundamentals, real estate diversification: by country or by continent, related papers.
Showing 1 through 3 of 0 Related Papers
Literature Review
- First Online: 27 August 2022
Cite this chapter
- Deepiga Vigneswaran 4 ,
- Michael Truebestein 5 &
- Matthias Daniel Aepli 5
Part of the book series: Contributions to Finance and Accounting ((CFA))
276 Accesses
Existing literature explores financialization of real estate markets, specifically on how this relationship coincides with the growth of institutional investors in this space. However, the focus has often been on the effects of real estate financialization on the renters’ experience. The existing literature almost only employs qualitative data analysis techniques, often by means of comparative case studies analysing housing developments conceived by either REIT-like entities or publicly listed companies. This chapter closes by identifying the gaps in the literature. There is limited exploration of return potential of affordable real estate to its equity investors. There is much needed study on the influence of these assets in the portfolios of institutional investors. Other gaps include the use of quantitative research methodologies, data collection, and analysis strategies.
This is a preview of subscription content, log in via an institution to check access.
Access this chapter
Subscribe and save.
- Get 10 units per month
- Download Article/Chapter or eBook
- 1 Unit = 1 Article or 1 Chapter
- Cancel anytime
- Available as PDF
- Read on any device
- Instant download
- Own it forever
- Available as EPUB and PDF
- Compact, lightweight edition
- Dispatched in 3 to 5 business days
- Free shipping worldwide - see info
- Durable hardcover edition
Tax calculation will be finalised at checkout
Purchases are for personal use only
Institutional subscriptions
Aalbers, M. B., & Holm, A. (2008). Privatising social housing in Europe: The cases of Amsterdam and Berlin. In K. Adelhof, B. Glock, & J. Lossau (Eds.), Urban trends in Berlin and Amsterdam (Vol. 110, pp. 12–23).
Google Scholar
Aalbers, M. B., Van Loon, J., & Fernandez, R. (2017). The financialization of a social housing provider. International Journal of Urban and Regional Research, 41 (4), 572–587. https://doi.org/10.1111/1468-2427.12520
Article Google Scholar
Belotti, E. (2017). The importation of social mix policy in Italy: A case study from Lombardy. Cities, 71 , 41–48. https://doi.org/10.1016/j.cities.2017.06.013
Brill, F., & Durrant, D. (2021). The emergence of a Build to Rent model: The role of narratives and discourses. Economy and Space, 1 (18), 1–18. https://www.researchgate.net/publication/348570618_The_emergence_of_a_Build_to_Rent_model_The_role_of_narratives_and_discourses
Cooper, C. (2012). Accounting for the fictitious: Living death by mainstream economics . Interdisciplinary Perspectives on Accounting Conference, Cardiff.
Gimat, M., Marot, B., & Le Bon-Vuylsteke, M. (2020). État des connaissances sur la vente de logements sociaux en Europe: Allemagne, France, Pays-Bas et Royaume-Uni . Institut CDC pour la recherche.
Fields, D., & Uffer, S. (2016). The financialisation of rental housing: A comparative analysis of New York City and Berlin. Urban Studies, 53 (7), 1486–1502. https://doi.org/10.1177/0042098014543704
Graddy, E., & Bostic, R. (2010). The role of private agents in affordable housing policy. Journal of Public Administration Research and Theory: J-PART, 20 , 81–99.
Lawson, J., Berry, M., Hamilton, C., & Pawson, H. (2014). Enhancing affordable rental housing investment via an intermediary and guarantee . AHURI Final Report No. 220, Australian Housing and Urban Research Institute Limited. https://www.ahuri.edu.au/research/final-reports/220
Lizieri, C. (2009). Towers of capital. Office markets & international financial services . Wiley-Blackwell.
Book Google Scholar
Moreno, L. (2014). The urban process under financialised capitalism. City, 18 , 244–268.
Perry, J., & Nölke, A. (2006). The political economy of International Accounting Standards. Review of International Political Economy, 13 (4), 559–586.
Ronald, R., Lennartz, C., & Kadi, J. (2017). What ever happened to asset-based welfare? Shifting approaches to Housing Wealth and Welfare Security. Policy & Politics, 45 (2), 173–193. https://doi.org/10.1332/030557316X14786045239560
Tang, C., Oxley, M., & Mekic, D. (2017). Meeting commercial and social goals: Institutional investment in the housing association sector. Housing Studies, 32 (4), 411–427. https://doi.org/10.1080/02673037.2016.1210098
Tapp, R. (2019). Layers of finance: Historic tax credits and the fiscal geographies of urban redevelopment. Geoforum, 105 , 13–22. https://doi.org/10.1016/j.geoforum.2019.06.016
Unger, K. (2013). Der große Ausverkauf. Die Finanzialisierung der ehemals gemeinnützigen Wohnungswirtschaft in Deutschland. Zeitschrift Marxistische Erneuerung, 95 , 24–35.
Wainwright, T., & Manville, G. (2017). Financialization and the third sector: Innovation in social housing bond markets. Environment and Planning A, 49 (4), 819–838. https://doi.org/10.1177/0308518X16684140
Wijburg, G. (2019). Reasserting state power by remaking markets? The introduction of real estate investment trusts in France and its implications for state-finance relations in the Greater Paris region. Geoforum, 100 , 1–11. https://doi.org/10.1016/j.geoforum.2019.01.012
Wijburg, G., & Waldron, R. (2020). Financialised privatisation, affordable housing and institutional investment: The case of England. Critical Housing Analysis, 7 (1), 114–129. https://doi.org/10.13060/23362839.2020.7.1.508
Wijburg, G., Aalbers, M., & Heeg, S. (2018). The financialisation of rental housing 2.0: Releasing housing into the privatised mainstream of capital accumulation. Antipode, 50 (4), 1098–1119. https://doi.org/10.1111/anti.12382
Download references
Author information
Authors and affiliations.
EBG Investment Solutions AG, Zurich, Switzerland
Deepiga Vigneswaran
University of Lucerne (HSLU), Lucerne, Switzerland
Michael Truebestein & Matthias Daniel Aepli
You can also search for this author in PubMed Google Scholar
Rights and permissions
Reprints and permissions
Copyright information
© 2022 The Author(s), under exclusive license to Springer Nature Switzerland AG
About this chapter
Vigneswaran, D., Truebestein, M., Aepli, M.D. (2022). Literature Review. In: Affordable Housing as a Profitable Impact Investment. Contributions to Finance and Accounting. Springer, Cham. https://doi.org/10.1007/978-3-031-07091-4_3
Download citation
DOI : https://doi.org/10.1007/978-3-031-07091-4_3
Published : 27 August 2022
Publisher Name : Springer, Cham
Print ISBN : 978-3-031-07090-7
Online ISBN : 978-3-031-07091-4
eBook Packages : Economics and Finance Economics and Finance (R0)
Share this chapter
Anyone you share the following link with will be able to read this content:
Sorry, a shareable link is not currently available for this article.
Provided by the Springer Nature SharedIt content-sharing initiative
- Publish with us
Policies and ethics
- Find a journal
- Track your research
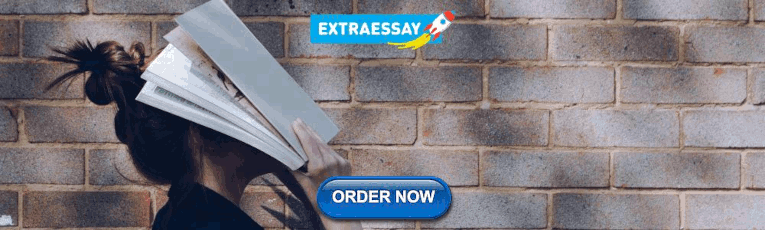
COMMENTS
The literature review of 86 studies examined behavioural biases in real estate investment. This section presents a few findings from the study that give a broad overview of the...
This article provided a comprehensive overview of residential property investor types and differentiations engaged within the existing social science literature, and a review and synthesis of key topics addressed in relation to these residential property investors.
Find methods information, sources, references or conduct a literature review on REAL ESTATE INVESTMENT
This paper is a survey of the literature on Real Estate Investment Trusts, commonly as REITs. The literature is separated into three major research topics: investment financing decisions, and return and risk issues. The central papers addressing each. optics are described and their results are summarized.
This article discusses the recent literature on the benefits of including real estate and private equity investments in an investor's portfolio, and examines certain topics that have attracted … Expand
The REIT markets have become an attractive trading avenue to add real estate exposure to a portfolio because of their historically high returns and high dividend payouts, but a review of extant literature by Stelk, Zhou, and Anderson (Citation 2017) document evidence that the link between REIT returns and physical real estate returns is mixed.
We review real estate literature with respect to contributions that (i) document factors from theoretical market models and frameworks; (ii) examine the findings of surveys of investors’ selection criteria and market perceptions; and (iii) run quantitative or qualitative market analyses.
Existing literature in the realm of private investments and affordable housing cover topics such as impact investing, financialization, the involvement of institutional investors, the growth of the alternative investment space, and the shift from state to private finance relations.
Summary. The real estate market displays many of the behavioral biases documented in the traditional financial markets. During bull markets, investor overconfidence, optimism, representativeness, and self-attribution bias among others drive prices far above their fundamental values.
The paper is organised by how investing in a real estate asset is analysed: in a mixed-asset portfolio context or a real-estate-only portfolio context. A fourth section focuses on research that has explored currency risk, one of the prevailing risks associated with international investing.