Have a language expert improve your writing
Run a free plagiarism check in 10 minutes, generate accurate citations for free.
- Knowledge Base
Methodology
- What Is a Case Study? | Definition, Examples & Methods
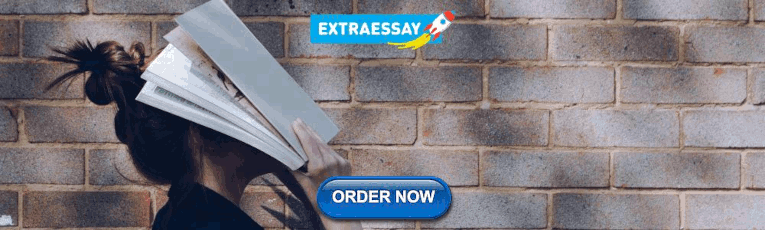
What Is a Case Study? | Definition, Examples & Methods
Published on May 8, 2019 by Shona McCombes . Revised on November 20, 2023.
A case study is a detailed study of a specific subject, such as a person, group, place, event, organization, or phenomenon. Case studies are commonly used in social, educational, clinical, and business research.
A case study research design usually involves qualitative methods , but quantitative methods are sometimes also used. Case studies are good for describing , comparing, evaluating and understanding different aspects of a research problem .
Table of contents
When to do a case study, step 1: select a case, step 2: build a theoretical framework, step 3: collect your data, step 4: describe and analyze the case, other interesting articles.
A case study is an appropriate research design when you want to gain concrete, contextual, in-depth knowledge about a specific real-world subject. It allows you to explore the key characteristics, meanings, and implications of the case.
Case studies are often a good choice in a thesis or dissertation . They keep your project focused and manageable when you don’t have the time or resources to do large-scale research.
You might use just one complex case study where you explore a single subject in depth, or conduct multiple case studies to compare and illuminate different aspects of your research problem.
Research question | Case study |
---|---|
What are the ecological effects of wolf reintroduction? | Case study of wolf reintroduction in Yellowstone National Park |
How do populist politicians use narratives about history to gain support? | Case studies of Hungarian prime minister Viktor Orbán and US president Donald Trump |
How can teachers implement active learning strategies in mixed-level classrooms? | Case study of a local school that promotes active learning |
What are the main advantages and disadvantages of wind farms for rural communities? | Case studies of three rural wind farm development projects in different parts of the country |
How are viral marketing strategies changing the relationship between companies and consumers? | Case study of the iPhone X marketing campaign |
How do experiences of work in the gig economy differ by gender, race and age? | Case studies of Deliveroo and Uber drivers in London |
Here's why students love Scribbr's proofreading services
Discover proofreading & editing
Once you have developed your problem statement and research questions , you should be ready to choose the specific case that you want to focus on. A good case study should have the potential to:
- Provide new or unexpected insights into the subject
- Challenge or complicate existing assumptions and theories
- Propose practical courses of action to resolve a problem
- Open up new directions for future research
TipIf your research is more practical in nature and aims to simultaneously investigate an issue as you solve it, consider conducting action research instead.
Unlike quantitative or experimental research , a strong case study does not require a random or representative sample. In fact, case studies often deliberately focus on unusual, neglected, or outlying cases which may shed new light on the research problem.
Example of an outlying case studyIn the 1960s the town of Roseto, Pennsylvania was discovered to have extremely low rates of heart disease compared to the US average. It became an important case study for understanding previously neglected causes of heart disease.
However, you can also choose a more common or representative case to exemplify a particular category, experience or phenomenon.
Example of a representative case studyIn the 1920s, two sociologists used Muncie, Indiana as a case study of a typical American city that supposedly exemplified the changing culture of the US at the time.
While case studies focus more on concrete details than general theories, they should usually have some connection with theory in the field. This way the case study is not just an isolated description, but is integrated into existing knowledge about the topic. It might aim to:
- Exemplify a theory by showing how it explains the case under investigation
- Expand on a theory by uncovering new concepts and ideas that need to be incorporated
- Challenge a theory by exploring an outlier case that doesn’t fit with established assumptions
To ensure that your analysis of the case has a solid academic grounding, you should conduct a literature review of sources related to the topic and develop a theoretical framework . This means identifying key concepts and theories to guide your analysis and interpretation.
There are many different research methods you can use to collect data on your subject. Case studies tend to focus on qualitative data using methods such as interviews , observations , and analysis of primary and secondary sources (e.g., newspaper articles, photographs, official records). Sometimes a case study will also collect quantitative data.
Example of a mixed methods case studyFor a case study of a wind farm development in a rural area, you could collect quantitative data on employment rates and business revenue, collect qualitative data on local people’s perceptions and experiences, and analyze local and national media coverage of the development.
The aim is to gain as thorough an understanding as possible of the case and its context.
Prevent plagiarism. Run a free check.
In writing up the case study, you need to bring together all the relevant aspects to give as complete a picture as possible of the subject.
How you report your findings depends on the type of research you are doing. Some case studies are structured like a standard scientific paper or thesis , with separate sections or chapters for the methods , results and discussion .
Others are written in a more narrative style, aiming to explore the case from various angles and analyze its meanings and implications (for example, by using textual analysis or discourse analysis ).
In all cases, though, make sure to give contextual details about the case, connect it back to the literature and theory, and discuss how it fits into wider patterns or debates.
If you want to know more about statistics , methodology , or research bias , make sure to check out some of our other articles with explanations and examples.
- Normal distribution
- Degrees of freedom
- Null hypothesis
- Discourse analysis
- Control groups
- Mixed methods research
- Non-probability sampling
- Quantitative research
- Ecological validity
Research bias
- Rosenthal effect
- Implicit bias
- Cognitive bias
- Selection bias
- Negativity bias
- Status quo bias
Cite this Scribbr article
If you want to cite this source, you can copy and paste the citation or click the “Cite this Scribbr article” button to automatically add the citation to our free Citation Generator.
McCombes, S. (2023, November 20). What Is a Case Study? | Definition, Examples & Methods. Scribbr. Retrieved September 23, 2024, from https://www.scribbr.com/methodology/case-study/
Is this article helpful?
Shona McCombes
Other students also liked, primary vs. secondary sources | difference & examples, what is a theoretical framework | guide to organizing, what is action research | definition & examples, what is your plagiarism score.
Have a language expert improve your writing
Run a free plagiarism check in 10 minutes, automatically generate references for free.
- Knowledge Base
- Methodology
- Case Study | Definition, Examples & Methods
Case Study | Definition, Examples & Methods
Published on 5 May 2022 by Shona McCombes . Revised on 30 January 2023.
A case study is a detailed study of a specific subject, such as a person, group, place, event, organisation, or phenomenon. Case studies are commonly used in social, educational, clinical, and business research.
A case study research design usually involves qualitative methods , but quantitative methods are sometimes also used. Case studies are good for describing , comparing, evaluating, and understanding different aspects of a research problem .
Table of contents
When to do a case study, step 1: select a case, step 2: build a theoretical framework, step 3: collect your data, step 4: describe and analyse the case.
A case study is an appropriate research design when you want to gain concrete, contextual, in-depth knowledge about a specific real-world subject. It allows you to explore the key characteristics, meanings, and implications of the case.
Case studies are often a good choice in a thesis or dissertation . They keep your project focused and manageable when you don’t have the time or resources to do large-scale research.
You might use just one complex case study where you explore a single subject in depth, or conduct multiple case studies to compare and illuminate different aspects of your research problem.
Research question | Case study |
---|---|
What are the ecological effects of wolf reintroduction? | Case study of wolf reintroduction in Yellowstone National Park in the US |
How do populist politicians use narratives about history to gain support? | Case studies of Hungarian prime minister Viktor Orbán and US president Donald Trump |
How can teachers implement active learning strategies in mixed-level classrooms? | Case study of a local school that promotes active learning |
What are the main advantages and disadvantages of wind farms for rural communities? | Case studies of three rural wind farm development projects in different parts of the country |
How are viral marketing strategies changing the relationship between companies and consumers? | Case study of the iPhone X marketing campaign |
How do experiences of work in the gig economy differ by gender, race, and age? | Case studies of Deliveroo and Uber drivers in London |
Prevent plagiarism, run a free check.
Once you have developed your problem statement and research questions , you should be ready to choose the specific case that you want to focus on. A good case study should have the potential to:
- Provide new or unexpected insights into the subject
- Challenge or complicate existing assumptions and theories
- Propose practical courses of action to resolve a problem
- Open up new directions for future research
Unlike quantitative or experimental research, a strong case study does not require a random or representative sample. In fact, case studies often deliberately focus on unusual, neglected, or outlying cases which may shed new light on the research problem.
If you find yourself aiming to simultaneously investigate and solve an issue, consider conducting action research . As its name suggests, action research conducts research and takes action at the same time, and is highly iterative and flexible.
However, you can also choose a more common or representative case to exemplify a particular category, experience, or phenomenon.
While case studies focus more on concrete details than general theories, they should usually have some connection with theory in the field. This way the case study is not just an isolated description, but is integrated into existing knowledge about the topic. It might aim to:
- Exemplify a theory by showing how it explains the case under investigation
- Expand on a theory by uncovering new concepts and ideas that need to be incorporated
- Challenge a theory by exploring an outlier case that doesn’t fit with established assumptions
To ensure that your analysis of the case has a solid academic grounding, you should conduct a literature review of sources related to the topic and develop a theoretical framework . This means identifying key concepts and theories to guide your analysis and interpretation.
There are many different research methods you can use to collect data on your subject. Case studies tend to focus on qualitative data using methods such as interviews, observations, and analysis of primary and secondary sources (e.g., newspaper articles, photographs, official records). Sometimes a case study will also collect quantitative data .
The aim is to gain as thorough an understanding as possible of the case and its context.
In writing up the case study, you need to bring together all the relevant aspects to give as complete a picture as possible of the subject.
How you report your findings depends on the type of research you are doing. Some case studies are structured like a standard scientific paper or thesis, with separate sections or chapters for the methods , results , and discussion .
Others are written in a more narrative style, aiming to explore the case from various angles and analyse its meanings and implications (for example, by using textual analysis or discourse analysis ).
In all cases, though, make sure to give contextual details about the case, connect it back to the literature and theory, and discuss how it fits into wider patterns or debates.
Cite this Scribbr article
If you want to cite this source, you can copy and paste the citation or click the ‘Cite this Scribbr article’ button to automatically add the citation to our free Reference Generator.
McCombes, S. (2023, January 30). Case Study | Definition, Examples & Methods. Scribbr. Retrieved 23 September 2024, from https://www.scribbr.co.uk/research-methods/case-studies/
Is this article helpful?
Shona McCombes
Other students also liked, correlational research | guide, design & examples, a quick guide to experimental design | 5 steps & examples, descriptive research design | definition, methods & examples.
A Guide To Secondary Data Analysis
What is secondary data analysis? How do you carry it out? Find out in this post.
Historically, the only way data analysts could obtain data was to collect it themselves. This type of data is often referred to as primary data and is still a vital resource for data analysts.
However, technological advances over the last few decades mean that much past data is now readily available online for data analysts and researchers to access and utilize. This type of data—known as secondary data—is driving a revolution in data analytics and data science.
Primary and secondary data share many characteristics. However, there are some fundamental differences in how you prepare and analyze secondary data. This post explores the unique aspects of secondary data analysis. We’ll briefly review what secondary data is before outlining how to source, collect and validate them. We’ll cover:
- What is secondary data analysis?
- How to carry out secondary data analysis (5 steps)
- Summary and further reading
Ready for a crash course in secondary data analysis? Let’s go!
1. What is secondary data analysis?
Secondary data analysis uses data collected by somebody else. This contrasts with primary data analysis, which involves a researcher collecting predefined data to answer a specific question. Secondary data analysis has numerous benefits, not least that it is a time and cost-effective way of obtaining data without doing the research yourself.
It’s worth noting here that secondary data may be primary data for the original researcher. It only becomes secondary data when it’s repurposed for a new task. As a result, a dataset can simultaneously be a primary data source for one researcher and a secondary data source for another. So don’t panic if you get confused! We explain exactly what secondary data is in this guide .
In reality, the statistical techniques used to carry out secondary data analysis are no different from those used to analyze other kinds of data. The main differences lie in collection and preparation. Once the data have been reviewed and prepared, the analytics process continues more or less as it usually does. For a recap on what the data analysis process involves, read this post .
In the following sections, we’ll focus specifically on the preparation of secondary data for analysis. Where appropriate, we’ll refer to primary data analysis for comparison.
2. How to carry out secondary data analysis
Step 1: define a research topic.
The first step in any data analytics project is defining your goal. This is true regardless of the data you’re working with, or the type of analysis you want to carry out. In data analytics lingo, this typically involves defining:
- A statement of purpose
- Research design
Defining a statement of purpose and a research approach are both fundamental building blocks for any project. However, for secondary data analysis, the process of defining these differs slightly. Let’s find out how.
Step 2: Establish your statement of purpose
Before beginning any data analytics project, you should always have a clearly defined intent. This is called a ‘statement of purpose.’ A healthcare analyst’s statement of purpose, for example, might be: ‘Reduce admissions for mental health issues relating to Covid-19′. The more specific the statement of purpose, the easier it is to determine which data to collect, analyze, and draw insights from.
A statement of purpose is helpful for both primary and secondary data analysis. It’s especially relevant for secondary data analysis, though. This is because there are vast amounts of secondary data available. Having a clear direction will keep you focused on the task at hand, saving you from becoming overwhelmed. Being selective with your data sources is key.
Step 3: Design your research process
After defining your statement of purpose, the next step is to design the research process. For primary data, this involves determining the types of data you want to collect (e.g. quantitative, qualitative, or both ) and a methodology for gathering them.
For secondary data analysis, however, your research process will more likely be a step-by-step guide outlining the types of data you require and a list of potential sources for gathering them. It may also include (realistic) expectations of the output of the final analysis. This should be based on a preliminary review of the data sources and their quality.
Once you have both your statement of purpose and research design, you’re in a far better position to narrow down potential sources of secondary data. You can then start with the next step of the process: data collection.
Step 4: Locate and collect your secondary data
Collecting primary data involves devising and executing a complex strategy that can be very time-consuming to manage. The data you collect, though, will be highly relevant to your research problem.
Secondary data collection, meanwhile, avoids the complexity of defining a research methodology. However, it comes with additional challenges. One of these is identifying where to find the data. This is no small task because there are a great many repositories of secondary data available. Your job, then, is to narrow down potential sources. As already mentioned, it’s necessary to be selective, or else you risk becoming overloaded.
Some popular sources of secondary data include:
- Government statistics , e.g. demographic data, censuses, or surveys, collected by government agencies/departments (like the US Bureau of Labor Statistics).
- Technical reports summarizing completed or ongoing research from educational or public institutions (colleges or government).
- Scientific journals that outline research methodologies and data analysis by experts in fields like the sciences, medicine, etc.
- Literature reviews of research articles, books, and reports, for a given area of study (once again, carried out by experts in the field).
- Trade/industry publications , e.g. articles and data shared in trade publications, covering topics relating to specific industry sectors, such as tech or manufacturing.
- Online resources: Repositories, databases, and other reference libraries with public or paid access to secondary data sources.
Once you’ve identified appropriate sources, you can go about collecting the necessary data. This may involve contacting other researchers, paying a fee to an organization in exchange for a dataset, or simply downloading a dataset for free online .
Step 5: Evaluate your secondary data
Secondary data is usually well-structured, so you might assume that once you have your hands on a dataset, you’re ready to dive in with a detailed analysis. Unfortunately, that’s not the case!
First, you must carry out a careful review of the data. Why? To ensure that they’re appropriate for your needs. This involves two main tasks:
Evaluating the secondary dataset’s relevance
- Assessing its broader credibility
Both these tasks require critical thinking skills. However, they aren’t heavily technical. This means anybody can learn to carry them out.
Let’s now take a look at each in a bit more detail.
The main point of evaluating a secondary dataset is to see if it is suitable for your needs. This involves asking some probing questions about the data, including:
What was the data’s original purpose?
Understanding why the data were originally collected will tell you a lot about their suitability for your current project. For instance, was the project carried out by a government agency or a private company for marketing purposes? The answer may provide useful information about the population sample, the data demographics, and even the wording of specific survey questions. All this can help you determine if the data are right for you, or if they are biased in any way.
When and where were the data collected?
Over time, populations and demographics change. Identifying when the data were first collected can provide invaluable insights. For instance, a dataset that initially seems suited to your needs may be out of date.
On the flip side, you might want past data so you can draw a comparison with a present dataset. In this case, you’ll need to ensure the data were collected during the appropriate time frame. It’s worth mentioning that secondary data are the sole source of past data. You cannot collect historical data using primary data collection techniques.
Similarly, you should ask where the data were collected. Do they represent the geographical region you require? Does geography even have an impact on the problem you are trying to solve?
What data were collected and how?
A final report for past data analytics is great for summarizing key characteristics or findings. However, if you’re planning to use those data for a new project, you’ll need the original documentation. At the very least, this should include access to the raw data and an outline of the methodology used to gather them. This can be helpful for many reasons. For instance, you may find raw data that wasn’t relevant to the original analysis, but which might benefit your current task.
What questions were participants asked?
We’ve already touched on this, but the wording of survey questions—especially for qualitative datasets—is significant. Questions may deliberately be phrased to preclude certain answers. A question’s context may also impact the findings in a way that’s not immediately obvious. Understanding these issues will shape how you perceive the data.
What is the form/shape/structure of the data?
Finally, to practical issues. Is the structure of the data suitable for your needs? Is it compatible with other sources or with your preferred analytics approach? This is purely a structural issue. For instance, if a dataset of people’s ages is saved as numerical rather than continuous variables, this could potentially impact your analysis. In general, reviewing a dataset’s structure helps better understand how they are categorized, allowing you to account for any discrepancies. You may also need to tidy the data to ensure they are consistent with any other sources you’re using.
This is just a sample of the types of questions you need to consider when reviewing a secondary data source. The answers will have a clear impact on whether the dataset—no matter how well presented or structured it seems—is suitable for your needs.
Assessing secondary data’s credibility
After identifying a potentially suitable dataset, you must double-check the credibility of the data. Namely, are the data accurate and unbiased? To figure this out, here are some key questions you might want to include:
What are the credentials of those who carried out the original research?
Do you have access to the details of the original researchers? What are their credentials? Where did they study? Are they an expert in the field or a newcomer? Data collection by an undergraduate student, for example, may not be as rigorous as that of a seasoned professor.
And did the original researcher work for a reputable organization? What other affiliations do they have? For instance, if a researcher who works for a tobacco company gathers data on the effects of vaping, this represents an obvious conflict of interest! Questions like this help determine how thorough or qualified the researchers are and if they have any potential biases.
Do you have access to the full methodology?
Does the dataset include a clear methodology, explaining in detail how the data were collected? This should be more than a simple overview; it must be a clear breakdown of the process, including justifications for the approach taken. This allows you to determine if the methodology was sound. If you find flaws (or no methodology at all) it throws the quality of the data into question.
How consistent are the data with other sources?
Do the secondary data match with any similar findings? If not, that doesn’t necessarily mean the data are wrong, but it does warrant closer inspection. Perhaps the collection methodology differed between sources, or maybe the data were analyzed using different statistical techniques. Or perhaps unaccounted-for outliers are skewing the analysis. Identifying all these potential problems is essential. A flawed or biased dataset can still be useful but only if you know where its shortcomings lie.
Have the data been published in any credible research journals?
Finally, have the data been used in well-known studies or published in any journals? If so, how reputable are the journals? In general, you can judge a dataset’s quality based on where it has been published. If in doubt, check out the publication in question on the Directory of Open Access Journals . The directory has a rigorous vetting process, only permitting journals of the highest quality. Meanwhile, if you found the data via a blurry image on social media without cited sources, then you can justifiably question its quality!
Again, these are just a few of the questions you might ask when determining the quality of a secondary dataset. Consider them as scaffolding for cultivating a critical thinking mindset; a necessary trait for any data analyst!
Presuming your secondary data holds up to scrutiny, you should be ready to carry out your detailed statistical analysis. As we explained at the beginning of this post, the analytical techniques used for secondary data analysis are no different than those for any other kind of data. Rather than go into detail here, check out the different types of data analysis in this post.
3. Secondary data analysis: Key takeaways
In this post, we’ve looked at the nuances of secondary data analysis, including how to source, collect and review secondary data. As discussed, much of the process is the same as it is for primary data analysis. The main difference lies in how secondary data are prepared.
Carrying out a meaningful secondary data analysis involves spending time and effort exploring, collecting, and reviewing the original data. This will help you determine whether the data are suitable for your needs and if they are of good quality.
Why not get to know more about what data analytics involves with this free, five-day introductory data analytics short course ? And, for more data insights, check out these posts:
- Discrete vs continuous data variables: What’s the difference?
- What are the four levels of measurement? Nominal, ordinal, interval, and ratio data explained
- What are the best tools for data mining?
Case study research: opening up research opportunities
RAUSP Management Journal
ISSN : 2531-0488
Article publication date: 30 December 2019
Issue publication date: 3 March 2020
The case study approach has been widely used in management studies and the social sciences more generally. However, there are still doubts about when and how case studies should be used. This paper aims to discuss this approach, its various uses and applications, in light of epistemological principles, as well as the criteria for rigor and validity.
Design/methodology/approach
This paper discusses the various concepts of case and case studies in the methods literature and addresses the different uses of cases in relation to epistemological principles and criteria for rigor and validity.
The use of this research approach can be based on several epistemologies, provided the researcher attends to the internal coherence between method and epistemology, or what the authors call “alignment.”
Originality/value
This study offers a number of implications for the practice of management research, as it shows how the case study approach does not commit the researcher to particular data collection or interpretation methods. Furthermore, the use of cases can be justified according to multiple epistemological orientations.
- Epistemology
Takahashi, A.R.W. and Araujo, L. (2020), "Case study research: opening up research opportunities", RAUSP Management Journal , Vol. 55 No. 1, pp. 100-111. https://doi.org/10.1108/RAUSP-05-2019-0109
Emerald Publishing Limited
Copyright © 2019, Adriana Roseli Wünsch Takahashi and Luis Araujo.
Published in RAUSP Management Journal . Published by Emerald Publishing Limited. This article is published under the Creative Commons Attribution (CC BY 4.0) licence. Anyone may reproduce, distribute, translate and create derivative works of this article (for both commercial and non-commercial purposes), subject to full attribution to the original publication and authors. The full terms of this licence may be seen at http://creativecommons.org/licences/by/4.0/legalcode
1. Introduction
The case study as a research method or strategy brings us to question the very term “case”: after all, what is a case? A case-based approach places accords the case a central role in the research process ( Ragin, 1992 ). However, doubts still remain about the status of cases according to different epistemologies and types of research designs.
Despite these doubts, the case study is ever present in the management literature and represents the main method of management research in Brazil ( Coraiola, Sander, Maccali, & Bulgacov, 2013 ). Between 2001 and 2010, 2,407 articles (83.14 per cent of qualitative research) were published in conferences and management journals as case studies (Takahashi & Semprebom, 2013 ). A search on Spell.org.br for the term “case study” under title, abstract or keywords, for the period ranging from January 2010 to July 2019, yielded 3,040 articles published in the management field. Doing research using case studies, allows the researcher to immerse him/herself in the context and gain intensive knowledge of a phenomenon, which in turn demands suitable methodological principles ( Freitas et al. , 2017 ).
Our objective in this paper is to discuss notions of what constitutes a case and its various applications, considering epistemological positions as well as criteria for rigor and validity. The alignment between these dimensions is put forward as a principle advocating coherence among all phases of the research process.
This article makes two contributions. First, we suggest that there are several epistemological justifications for using case studies. Second, we show that the quality and rigor of academic research with case studies are directly related to the alignment between epistemology and research design rather than to choices of specific forms of data collection or analysis. The article is structured as follows: the following four sections discuss concepts of what is a case, its uses, epistemological grounding as well as rigor and quality criteria. The brief conclusions summarize the debate and invite the reader to delve into the literature on the case study method as a way of furthering our understanding of contemporary management phenomena.
2. What is a case study?
The debate over what constitutes a case in social science is a long-standing one. In 1988, Howard Becker and Charles Ragin organized a workshop to discuss the status of the case as a social science method. As the discussion was inconclusive, they posed the question “What is a case?” to a select group of eight social scientists in 1989, and later to participants in a symposium on the subject. Participants were unable to come up with a consensual answer. Since then, we have witnessed that further debates and different answers have emerged. The original question led to an even broader issue: “How do we, as social scientists, produce results and seem to know what we know?” ( Ragin, 1992 , p. 16).
An important step that may help us start a reflection on what is a case is to consider the phenomena we are looking at. To do that, we must know something about what we want to understand and how we might study it. The answer may be a causal explanation, a description of what was observed or a narrative of what has been experienced. In any case, there will always be a story to be told, as the choice of the case study method demands an answer to what the case is about.
A case may be defined ex ante , prior to the start of the research process, as in Yin’s (2015) classical definition. But, there is no compelling reason as to why cases must be defined ex ante . Ragin (1992 , p. 217) proposed the notion of “casing,” to indicate that what the case is emerges from the research process:
Rather than attempt to delineate the many different meanings of the term “case” in a formal taxonomy, in this essay I offer instead a view of cases that follows from the idea implicit in many of the contributions – that concocting cases is a varied but routine social scientific activity. […] The approach of this essay is that this activity, which I call “casing”, should be viewed in practical terms as a research tactic. It is selectively invoked at many different junctures in the research process, usually to resolve difficult issues in linking ideas and evidence.
In other words, “casing” is tied to the researcher’s practice, to the way he/she delimits or declares a case as a significant outcome of a process. In 2013, Ragin revisited the 1992 concept of “casing” and explored its multiple possibilities of use, paying particular attention to “negative cases.”
According to Ragin (1992) , a case can be centered on a phenomenon or a population. In the first scenario, cases are representative of a phenomenon, and are selected based on what can be empirically observed. The process highlights different aspects of cases and obscures others according to the research design, and allows for the complexity, specificity and context of the phenomenon to be explored. In the alternative, population-focused scenario, the selection of cases precedes the research. Both positive and negative cases are considered in exploring a phenomenon, with the definition of the set of cases dependent on theory and the central objective to build generalizations. As a passing note, it is worth mentioning here that a study of multiple cases requires a definition of the unit of analysis a priori . Otherwise, it will not be possible to make cross-case comparisons.
These two approaches entail differences that go beyond the mere opposition of quantitative and qualitative data, as a case often includes both types of data. Thus, the confusion about how to conceive cases is associated with Ragin’s (1992) notion of “small vs large N,” or McKeown’s (1999) “statistical worldview” – the notion that relevant findings are only those that can be made about a population based on the analysis of representative samples. In the same vein, Byrne (2013) argues that we cannot generate nomothetic laws that apply in all circumstances, periods and locations, and that no social science method can claim to generate invariant laws. According to the same author, case studies can help us understand that there is more than one ideographic variety and help make social science useful. Generalizations still matter, but they should be understood as part of defining the research scope, and that scope points to the limitations of knowledge produced and consumed in concrete time and space.
Thus, what defines the orientation and the use of cases is not the mere choice of type of data, whether quantitative or qualitative, but the orientation of the study. A statistical worldview sees cases as data units ( Byrne, 2013 ). Put differently, there is a clear distinction between statistical and qualitative worldviews; the use of quantitative data does not by itself means that the research is (quasi) statistical, or uses a deductive logic:
Case-based methods are useful, and represent, among other things, a way of moving beyond a useless and destructive tradition in the social sciences that have set quantitative and qualitative modes of exploration, interpretation, and explanation against each other ( Byrne, 2013 , p. 9).
Other authors advocate different understandings of what a case study is. To some, it is a research method, to others it is a research strategy ( Creswell, 1998 ). Sharan Merrian and Robert Yin, among others, began to write about case study research as a methodology in the 1980s (Merrian, 2009), while authors such as Eisenhardt (1989) called it a research strategy. Stake (2003) sees the case study not as a method, but as a choice of what to be studied, the unit of study. Regardless of their differences, these authors agree that case studies should be restricted to a particular context as they aim to provide an in-depth knowledge of a given phenomenon: “A case study is an in-depth description and analysis of a bounded system” (Merrian, 2009, p. 40). According to Merrian, a qualitative case study can be defined by the process through which the research is carried out, by the unit of analysis or the final product, as the choice ultimately depends on what the researcher wants to know. As a product of research, it involves the analysis of a given entity, phenomenon or social unit.
Thus, whether it is an organization, an individual, a context or a phenomenon, single or multiple, one must delimit it, and also choose between possible types and configurations (Merrian, 2009; Yin, 2015 ). A case study may be descriptive, exploratory, explanatory, single or multiple ( Yin, 2015 ); intrinsic, instrumental or collective ( Stake, 2003 ); and confirm or build theory ( Eisenhardt, 1989 ).
both went through the same process of implementing computer labs intended for the use of information and communication technologies in 2007;
both took part in the same regional program (Paraná Digital); and
they shared similar characteristics regarding location (operation in the same neighborhood of a city), number of students, number of teachers and technicians and laboratory sizes.
However, the two institutions differed in the number of hours of program use, with one of them displaying a significant number of hours/use while the other showed a modest number, according to secondary data for the period 2007-2013. Despite the context being similar and the procedures for implementing the technology being the same, the mechanisms of social integration – an idiosyncratic factor of each institution – were different in each case. This explained differences in their use of resource, processes of organizational learning and capacity to absorb new knowledge.
On the other hand, multiple case studies seek evidence in different contexts and do not necessarily require direct comparisons ( Stake, 2003 ). Rather, there is a search for patterns of convergence and divergence that permeate all the cases, as the same issues are explored in every case. Cases can be added progressively until theoretical saturation is achieved. An example is of a study that investigated how entrepreneurial opportunity and management skills were developed through entrepreneurial learning ( Zampier & Takahashi, 2014 ). The authors conducted nine case studies, based on primary and secondary data, with each one analyzed separately, so a search for patterns could be undertaken. The convergence aspects found were: the predominant way of transforming experience into knowledge was exploitation; managerial skills were developed through by taking advantages of opportunities; and career orientation encompassed more than one style. As for divergence patterns: the experience of success and failure influenced entrepreneurs differently; the prevailing rationality logic of influence was different; and the combination of styles in career orientation was diverse.
A full discussion of choice of case study design is outside the scope of this article. For the sake of illustration, we make a brief mention to other selection criteria such as the purpose of the research, the state of the art of the research theme, the time and resources involved and the preferred epistemological position of the researcher. In the next section, we look at the possibilities of carrying out case studies in line with various epistemological traditions, as the answers to the “what is a case?” question reveal varied methodological commitments as well as diverse epistemological and ontological positions ( Ragin, 2013 ).
3. Epistemological positioning of case study research
Ontology and epistemology are like skin, not a garment to be occasionally worn ( Marsh & Furlong, 2002 ). According to these authors, ontology and epistemology guide the choice of theory and method because they cannot or should not be worn as a garment. Hence, one must practice philosophical “self-knowledge” to recognize one’s vision of what the world is and of how knowledge of that world is accessed and validated. Ontological and epistemological positions are relevant in that they involve the positioning of the researcher in social science and the phenomena he or she chooses to study. These positions do not tend to vary from one project to another although they can certainly change over time for a single researcher.
Ontology is the starting point from which the epistemological and methodological positions of the research arise ( Grix, 2002 ). Ontology expresses a view of the world, what constitutes reality, nature and the image one has of social reality; it is a theory of being ( Marsh & Furlong, 2002 ). The central question is the nature of the world out there regardless of our ability to access it. An essentialist or foundationalist ontology acknowledges that there are differences that persist over time and these differences are what underpin the construction of social life. An opposing, anti-foundationalist position presumes that the differences found are socially constructed and may vary – i.e. they are not essential but specific to a given culture at a given time ( Marsh & Furlong, 2002 ).
Epistemology is centered around a theory of knowledge, focusing on the process of acquiring and validating knowledge ( Grix, 2002 ). Positivists look at social phenomena as a world of causal relations where there is a single truth to be accessed and confirmed. In this tradition, case studies test hypotheses and rely on deductive approaches and quantitative data collection and analysis techniques. Scholars in the field of anthropology and observation-based qualitative studies proposed alternative epistemologies based on notions of the social world as a set of manifold and ever-changing processes. In management studies since the 1970s, the gradual acceptance of qualitative research has generated a diverse range of research methods and conceptions of the individual and society ( Godoy, 1995 ).
The interpretative tradition, in direct opposition to positivism, argues that there is no single objective truth to be discovered about the social world. The social world and our knowledge of it are the product of social constructions. Thus, the social world is constituted by interactions, and our knowledge is hermeneutic as the world does not exist independent of our knowledge ( Marsh & Furlong, 2002 ). The implication is that it is not possible to access social phenomena through objective, detached methods. Instead, the interaction mechanisms and relationships that make up social constructions have to be studied. Deductive approaches, hypothesis testing and quantitative methods are not relevant here. Hermeneutics, on the other hand, is highly relevant as it allows the analysis of the individual’s interpretation, of sayings, texts and actions, even though interpretation is always the “truth” of a subject. Methods such as ethnographic case studies, interviews and observations as data collection techniques should feed research designs according to interpretivism. It is worth pointing out that we are to a large extent, caricaturing polar opposites rather characterizing a range of epistemological alternatives, such as realism, conventionalism and symbolic interactionism.
If diverse ontologies and epistemologies serve as a guide to research approaches, including data collection and analysis methods, and if they should be regarded as skin rather than clothing, how does one make choices regarding case studies? What are case studies, what type of knowledge they provide and so on? The views of case study authors are not always explicit on this point, so we must delve into their texts to glean what their positions might be.
Two of the cited authors in case study research are Robert Yin and Kathleen Eisenhardt. Eisenhardt (1989) argues that a case study can serve to provide a description, test or generate a theory, the latter being the most relevant in contributing to the advancement of knowledge in a given area. She uses terms such as populations and samples, control variables, hypotheses and generalization of findings and even suggests an ideal number of case studies to allow for theory construction through replication. Although Eisenhardt includes observation and interview among her recommended data collection techniques, the approach is firmly anchored in a positivist epistemology:
Third, particularly in comparison with Strauss (1987) and Van Maanen (1988), the process described here adopts a positivist view of research. That is, the process is directed toward the development of testable hypotheses and theory which are generalizable across settings. In contrast, authors like Strauss and Van Maanen are more concerned that a rich, complex description of the specific cases under study evolve and they appear less concerned with development of generalizable theory ( Eisenhardt, 1989 , p. 546).
This position attracted a fair amount of criticism. Dyer & Wilkins (1991) in a critique of Eisenhardt’s (1989) article focused on the following aspects: there is no relevant justification for the number of cases recommended; it is the depth and not the number of cases that provides an actual contribution to theory; and the researcher’s purpose should be to get closer to the setting and interpret it. According to the same authors, discrepancies from prior expectations are also important as they lead researchers to reflect on existing theories. Eisenhardt & Graebner (2007 , p. 25) revisit the argument for the construction of a theory from multiple cases:
A major reason for the popularity and relevance of theory building from case studies is that it is one of the best (if not the best) of the bridges from rich qualitative evidence to mainstream deductive research.
Although they recognize the importance of single-case research to explore phenomena under unique or rare circumstances, they reaffirm the strength of multiple case designs as it is through them that better accuracy and generalization can be reached.
Likewise, Robert Yin emphasizes the importance of variables, triangulation in the search for “truth” and generalizable theoretical propositions. Yin (2015 , p. 18) suggests that the case study method may be appropriate for different epistemological orientations, although much of his work seems to invoke a realist epistemology. Authors such as Merrian (2009) and Stake (2003) suggest an interpretative version of case studies. Stake (2003) looks at cases as a qualitative option, where the most relevant criterion of case selection should be the opportunity to learn and understand a phenomenon. A case is not just a research method or strategy; it is a researcher’s choice about what will be studied:
Even if my definition of case study was agreed upon, and it is not, the term case and study defy full specification (Kemmis, 1980). A case study is both a process of inquiry about the case and the product of that inquiry ( Stake, 2003 , p. 136).
Later, Stake (2003 , p. 156) argues that:
[…] the purpose of a case report is not to represent the world, but to represent the case. […] The utility of case research to practitioners and policy makers is in its extension of experience.
Still according to Stake (2003 , pp. 140-141), to do justice to complex views of social phenomena, it is necessary to analyze the context and relate it to the case, to look for what is peculiar rather than common in cases to delimit their boundaries, to plan the data collection looking for what is common and unusual about facts, what could be valuable whether it is unique or common:
Reflecting upon the pertinent literature, I find case study methodology written largely by people who presume that the research should contribute to scientific generalization. The bulk of case study work, however, is done by individuals who have intrinsic interest in the case and little interest in the advance of science. Their designs aim the inquiry toward understanding of what is important about that case within its own world, which is seldom the same as the worlds of researchers and theorists. Those designs develop what is perceived to be the case’s own issues, contexts, and interpretations, its thick descriptions . In contrast, the methods of instrumental case study draw the researcher toward illustrating how the concerns of researchers and theorists are manifest in the case. Because the critical issues are more likely to be know in advance and following disciplinary expectations, such a design can take greater advantage of already developed instruments and preconceived coding schemes.
The aforementioned authors were listed to illustrate differences and sometimes opposing positions on case research. These differences are not restricted to a choice between positivism and interpretivism. It is worth noting that Ragin’s (2013 , p. 523) approach to “casing” is compatible with the realistic research perspective:
In essence, to posit cases is to engage in ontological speculation regarding what is obdurately real but only partially and indirectly accessible through social science. Bringing a realist perspective to the case question deepens and enriches the dialogue, clarifying some key issues while sweeping others aside.
cases are actual entities, reflecting their operations of real causal mechanism and process patterns;
case studies are interactive processes and are open to revisions and refinements; and
social phenomena are complex, contingent and context-specific.
Ragin (2013 , p. 532) concludes:
Lurking behind my discussion of negative case, populations, and possibility analysis is the implication that treating cases as members of given (and fixed) populations and seeking to infer the properties of populations may be a largely illusory exercise. While demographers have made good use of the concept of population, and continue to do so, it is not clear how much the utility of the concept extends beyond their domain. In case-oriented work, the notion of fixed populations of cases (observations) has much less analytic utility than simply “the set of relevant cases,” a grouping that must be specified or constructed by the researcher. The demarcation of this set, as the work of case-oriented researchers illustrates, is always tentative, fluid, and open to debate. It is only by casing social phenomena that social scientists perceive the homogeneity that allows analysis to proceed.
In summary, case studies are relevant and potentially compatible with a range of different epistemologies. Researchers’ ontological and epistemological positions will guide their choice of theory, methodologies and research techniques, as well as their research practices. The same applies to the choice of authors describing the research method and this choice should be coherent. We call this research alignment , an attribute that must be judged on the internal coherence of the author of a study, and not necessarily its evaluator. The following figure illustrates the interrelationship between the elements of a study necessary for an alignment ( Figure 1 ).
In addition to this broader aspect of the research as a whole, other factors should be part of the researcher’s concern, such as the rigor and quality of case studies. We will look into these in the next section taking into account their relevance to the different epistemologies.
4. Rigor and quality in case studies
Traditionally, at least in positivist studies, validity and reliability are the relevant quality criteria to judge research. Validity can be understood as external, internal and construct. External validity means identifying whether the findings of a study are generalizable to other studies using the logic of replication in multiple case studies. Internal validity may be established through the theoretical underpinning of existing relationships and it involves the use of protocols for the development and execution of case studies. Construct validity implies defining the operational measurement criteria to establish a chain of evidence, such as the use of multiple sources of evidence ( Eisenhardt, 1989 ; Yin, 2015 ). Reliability implies conducting other case studies, instead of just replicating results, to minimize the errors and bias of a study through case study protocols and the development of a case database ( Yin, 2015 ).
Several criticisms have been directed toward case studies, such as lack of rigor, lack of generalization potential, external validity and researcher bias. Case studies are often deemed to be unreliable because of a lack of rigor ( Seuring, 2008 ). Flyvbjerg (2006 , p. 219) addresses five misunderstandings about case-study research, and concludes that:
[…] a scientific discipline without a large number of thoroughly executed case studies is a discipline without systematic production of exemplars, and a discipline without exemplars is an ineffective one.
theoretical knowledge is more valuable than concrete, practical knowledge;
the case study cannot contribute to scientific development because it is not possible to generalize on the basis of an individual case;
the case study is more useful for generating rather than testing hypotheses;
the case study contains a tendency to confirm the researcher’s preconceived notions; and
it is difficult to summarize and develop general propositions and theories based on case studies.
These criticisms question the validity of the case study as a scientific method and should be corrected.
The critique of case studies is often framed from the standpoint of what Ragin (2000) labeled large-N research. The logic of small-N research, to which case studies belong, is different. Cases benefit from depth rather than breadth as they: provide theoretical and empirical knowledge; contribute to theory through propositions; serve not only to confirm knowledge, but also to challenge and overturn preconceived notions; and the difficulty in summarizing their conclusions is because of the complexity of the phenomena studies and not an intrinsic limitation of the method.
Thus, case studies do not seek large-scale generalizations as that is not their purpose. And yet, this is a limitation from a positivist perspective as there is an external reality to be “apprehended” and valid conclusions to be extracted for an entire population. If positivism is the epistemology of choice, the rigor of a case study can be demonstrated by detailing the criteria used for internal and external validity, construct validity and reliability ( Gibbert & Ruigrok, 2010 ; Gibbert, Ruigrok, & Wicki, 2008 ). An example can be seen in case studies in the area of information systems, where there is a predominant orientation of positivist approaches to this method ( Pozzebon & Freitas, 1998 ). In this area, rigor also involves the definition of a unit of analysis, type of research, number of cases, selection of sites, definition of data collection and analysis procedures, definition of the research protocol and writing a final report. Creswell (1998) presents a checklist for researchers to assess whether the study was well written, if it has reliability and validity and if it followed methodological protocols.
In case studies with a non-positivist orientation, rigor can be achieved through careful alignment (coherence among ontology, epistemology, theory and method). Moreover, the concepts of validity can be understood as concern and care in formulating research, research development and research results ( Ollaik & Ziller, 2012 ), and to achieve internal coherence ( Gibbert et al. , 2008 ). The consistency between data collection and interpretation, and the observed reality also help these studies meet coherence and rigor criteria. Siggelkow (2007) argues that a case study should be persuasive and that even a single case study may be a powerful example to contest a widely held view. To him, the value of a single case study or studies with few cases can be attained by their potential to provide conceptual insights and coherence to the internal logic of conceptual arguments: “[…] a paper should allow a reader to see the world, and not just the literature, in a new way” ( Siggelkow, 2007 , p. 23).
Interpretative studies should not be justified by criteria derived from positivism as they are based on a different ontology and epistemology ( Sandberg, 2005 ). The rejection of an interpretive epistemology leads to the rejection of an objective reality: “As Bengtsson points out, the life-world is the subjects’ experience of reality, at the same time as it is objective in the sense that it is an intersubjective world” ( Sandberg, 2005 , p. 47). In this event, how can one demonstrate what positivists call validity and reliability? What would be the criteria to justify knowledge as truth, produced by research in this epistemology? Sandberg (2005 , p. 62) suggests an answer based on phenomenology:
This was demonstrated first by explicating life-world and intentionality as the basic assumptions underlying the interpretative research tradition. Second, based on those assumptions, truth as intentional fulfillment, consisting of perceived fulfillment, fulfillment in practice, and indeterminate fulfillment, was proposed. Third, based on the proposed truth constellation, communicative, pragmatic, and transgressive validity and reliability as interpretative awareness were presented as the most appropriate criteria for justifying knowledge produced within interpretative approach. Finally, the phenomenological epoché was suggested as a strategy for achieving these criteria.
From this standpoint, the research site must be chosen according to its uniqueness so that one can obtain relevant insights that no other site could provide ( Siggelkow, 2007 ). Furthermore, the view of what is being studied is at the center of the researcher’s attention to understand its “truth,” inserted in a given context.
The case researcher is someone who can reduce the probability of misinterpretations by analyzing multiple perceptions, searches for data triangulation to check for the reliability of interpretations ( Stake, 2003 ). It is worth pointing out that this is not an option for studies that specifically seek the individual’s experience in relation to organizational phenomena.
In short, there are different ways of seeking rigor and quality in case studies, depending on the researcher’s worldview. These different forms pervade everything from the research design, the choice of research questions, the theory or theories to look at a phenomenon, research methods, the data collection and analysis techniques, to the type and style of research report produced. Validity can also take on different forms. While positivism is concerned with validity of the research question and results, interpretivism emphasizes research processes without neglecting the importance of the articulation of pertinent research questions and the sound interpretation of results ( Ollaik & Ziller, 2012 ). The means to achieve this can be diverse, such as triangulation (of multiple theories, multiple methods, multiple data sources or multiple investigators), pre-tests of data collection instrument, pilot case, study protocol, detailed description of procedures such as field diary in observations, researcher positioning (reflexivity), theoretical-empirical consistency, thick description and transferability.
5. Conclusions
The central objective of this article was to discuss concepts of case study research, their potential and various uses, taking into account different epistemologies as well as criteria of rigor and validity. Although the literature on methodology in general and on case studies in particular, is voluminous, it is not easy to relate this approach to epistemology. In addition, method manuals often focus on the details of various case study approaches which confuse things further.
Faced with this scenario, we have tried to address some central points in this debate and present various ways of using case studies according to the preferred epistemology of the researcher. We emphasize that this understanding depends on how a case is defined and the particular epistemological orientation that underpins that conceptualization. We have argued that whatever the epistemological orientation is, it is possible to meet appropriate criteria of research rigor and quality provided there is an alignment among the different elements of the research process. Furthermore, multiple data collection techniques can be used in in single or multiple case study designs. Data collection techniques or the type of data collected do not define the method or whether cases should be used for theory-building or theory-testing.
Finally, we encourage researchers to consider case study research as one way to foster immersion in phenomena and their contexts, stressing that the approach does not imply a commitment to a particular epistemology or type of research, such as qualitative or quantitative. Case study research allows for numerous possibilities, and should be celebrated for that diversity rather than pigeon-holed as a monolithic research method.
The interrelationship between the building blocks of research
Byrne , D. ( 2013 ). Case-based methods: Why We need them; what they are; how to do them . Byrne D. In D Byrne. and C.C Ragin (Eds.), The SAGE handbooks of Case-Based methods , pp. 1 – 10 . London : SAGE Publications Inc .
Creswell , J. W. ( 1998 ). Qualitative inquiry and research design: choosing among five traditions , London : Sage Publications .
Coraiola , D. M. , Sander , J. A. , Maccali , N. & Bulgacov , S. ( 2013 ). Estudo de caso . In A. R. W. Takahashi , (Ed.), Pesquisa qualitativa em administração: Fundamentos, métodos e usos no Brasil , pp. 307 – 341 . São Paulo : Atlas .
Dyer , W. G. , & Wilkins , A. L. ( 1991 ). Better stories, not better constructs, to generate better theory: a rejoinder to Eisenhardt . The Academy of Management Review , 16 , 613 – 627 .
Eisenhardt , K. ( 1989 ). Building theory from case study research . Academy of Management Review , 14 , 532 – 550 .
Eisenhardt , K. M. , & Graebner , M. E. ( 2007 ). Theory building from cases: Opportunities and challenges . Academy of Management Journal , 50 , 25 – 32 .
Flyvbjerg , B. ( 2006 ). Five misunderstandings about case-study research . Qualitative Inquiry , 12 , 219 – 245 .
Freitas , J. S. , Ferreira , J. C. A. , Campos , A. A. R. , Melo , J. C. F. , Cheng , L. C. , & Gonçalves , C. A. ( 2017 ). Methodological roadmapping: a study of centering resonance analysis . RAUSP Management Journal , 53 , 459 – 475 .
Gibbert , M. , Ruigrok , W. , & Wicki , B. ( 2008 ). What passes as a rigorous case study? . Strategic Management Journal , 29 , 1465 – 1474 .
Gibbert , M. , & Ruigrok , W. ( 2010 ). The “what” and “how” of case study rigor: Three strategies based on published work . Organizational Research Methods , 13 , 710 – 737 .
Godoy , A. S. ( 1995 ). Introdução à pesquisa qualitativa e suas possibilidades . Revista de Administração de Empresas , 35 , 57 – 63 .
Grix , J. ( 2002 ). Introducing students to the generic terminology of social research . Politics , 22 , 175 – 186 .
Marsh , D. , & Furlong , P. ( 2002 ). A skin, not a sweater: ontology and epistemology in political science . In D Marsh. , & G Stoker , (Eds.), Theory and Methods in Political Science , New York, NY : Palgrave McMillan , pp. 17 – 41 .
McKeown , T. J. ( 1999 ). Case studies and the statistical worldview: Review of King, Keohane, and Verba’s designing social inquiry: Scientific inference in qualitative research . International Organization , 53 , 161 – 190 .
Merriam , S. B. ( 2009 ). Qualitative research: a guide to design and implementation .
Ollaik , L. G. , & Ziller , H. ( 2012 ). Distintas concepções de validade em pesquisas qualitativas . Educação e Pesquisa , 38 , 229 – 241 .
Picoli , F. R. , & Takahashi , A. R. W. ( 2016 ). Capacidade de absorção, aprendizagem organizacional e mecanismos de integração social . Revista de Administração Contemporânea , 20 , 1 – 20 .
Pozzebon , M. , & Freitas , H. M. R. ( 1998 ). Pela aplicabilidade: com um maior rigor científico – dos estudos de caso em sistemas de informação . Revista de Administração Contemporânea , 2 , 143 – 170 .
Sandberg , J. ( 2005 ). How do we justify knowledge produced within interpretive approaches? . Organizational Research Methods , 8 , 41 – 68 .
Seuring , S. A. ( 2008 ). Assessing the rigor of case study research in supply chain management. Supply chain management . Supply Chain Management: An International Journal , 13 , 128 – 137 .
Siggelkow , N. ( 2007 ). Persuasion with case studies . Academy of Management Journal , 50 , 20 – 24 .
Stake , R. E. ( 2003 ). Case studies . In N. K. , Denzin , & Y. S. , Lincoln (Eds.). Strategies of Qualitative Inquiry , London : Sage Publications . pp. 134 – 164 .
Takahashi , A. R. W. , & Semprebom , E. ( 2013 ). Resultados gerais e desafios . In A. R. W. , Takahashi (Ed.), Pesquisa qualitativa em administração: Fundamentos, métodos e usos no brasil , pp. 343 – 354 . São Paulo : Atlas .
Ragin , C. C. ( 1992 ). Introduction: Cases of “what is a case? . In H. S. , Becker , & C. C. Ragin and (Eds). What is a case? Exploring the foundations of social inquiry , pp. 1 – 18 .
Ragin , C. C. ( 2013 ). Reflections on casing and Case-Oriented research . In D , Byrne. , & C. C. Ragin (Eds.), The SAGE handbooks of Case-Based methods , London : SAGE Publications , pp. 522 – 534 .
Yin , R. K. ( 2015 ). Estudo de caso: planejamento e métodos , Porto Alegre : Bookman .
Zampier , M. A. , & Takahashi , A. R. W. ( 2014 ). Aprendizagem e competências empreendedoras: Estudo de casos de micro e pequenas empresas do setor educacional . RGO Revista Gestão Organizacional , 6 , 1 – 18 .
Acknowledgements
Author contributions: Both authors contributed equally.
Corresponding author
Related articles, all feedback is valuable.
Please share your general feedback
Report an issue or find answers to frequently asked questions
Contact Customer Support
- Case Reports
Qualitative Case Study Methodology: Study Design and Implementation for Novice Researchers
- January 2010
- The Qualitative Report 13(4)

- McMaster University

Abstract and Figures

Discover the world's research
- 25+ million members
- 160+ million publication pages
- 2.3+ billion citations

- Yihalem Tamiru
- Afework Mulugeta

- Zan Haggerty
- Ramachandran Saraswathy Gopakumar
- Sonu S. Babu
- Soumya Gopakumar
- Ravi Prasad Varma
- Alexander Poth
- RES SOC ADMIN PHARM

- Bob Algozzine

- B J Breitmayer
- Y.S. Lincoln
- ADV NURS SCI
- Margarete Sandelowski

- John W. Scheib
- Robert E. Stake
- Thomas J. Richards

- Recruit researchers
- Join for free
- Login Email Tip: Most researchers use their institutional email address as their ResearchGate login Password Forgot password? Keep me logged in Log in or Continue with Google Welcome back! Please log in. Email · Hint Tip: Most researchers use their institutional email address as their ResearchGate login Password Forgot password? Keep me logged in Log in or Continue with Google No account? Sign up
Root out friction in every digital experience, super-charge conversion rates, and optimize digital self-service
Uncover insights from any interaction, deliver AI-powered agent coaching, and reduce cost to serve
Increase revenue and loyalty with real-time insights and recommendations delivered to teams on the ground
Know how your people feel and empower managers to improve employee engagement, productivity, and retention
Take action in the moments that matter most along the employee journey and drive bottom line growth
Whatever they’re saying, wherever they’re saying it, know exactly what’s going on with your people
Get faster, richer insights with qual and quant tools that make powerful market research available to everyone
Run concept tests, pricing studies, prototyping + more with fast, powerful studies designed by UX research experts
Track your brand performance 24/7 and act quickly to respond to opportunities and challenges in your market
Explore the platform powering Experience Management
- Free Account
- Product Demos
- For Digital
- For Customer Care
- For Human Resources
- For Researchers
- Financial Services
- All Industries
Popular Use Cases
- Customer Experience
- Employee Experience
- Net Promoter Score
- Voice of Customer
- Customer Success Hub
- Product Documentation
- Training & Certification
- XM Institute
- Popular Resources
- Customer Stories
- Artificial Intelligence
Market Research
- Partnerships
- Marketplace
The annual gathering of the experience leaders at the world’s iconic brands building breakthrough business results, live in Salt Lake City.
- English/AU & NZ
- Español/Europa
- Español/América Latina
- Português Brasileiro
- REQUEST DEMO
- Experience Management
- Secondary Research
Try Qualtrics for free
Secondary research: definition, methods, & examples.
19 min read This ultimate guide to secondary research helps you understand changes in market trends, customers buying patterns and your competition using existing data sources.
In situations where you’re not involved in the data gathering process ( primary research ), you have to rely on existing information and data to arrive at specific research conclusions or outcomes. This approach is known as secondary research.
In this article, we’re going to explain what secondary research is, how it works, and share some examples of it in practice.
Free eBook: The ultimate guide to conducting market research
What is secondary research?
Secondary research, also known as desk research, is a research method that involves compiling existing data sourced from a variety of channels . This includes internal sources (e.g.in-house research) or, more commonly, external sources (such as government statistics, organizational bodies, and the internet).
Secondary research comes in several formats, such as published datasets, reports, and survey responses , and can also be sourced from websites, libraries, and museums.
The information is usually free — or available at a limited access cost — and gathered using surveys , telephone interviews, observation, face-to-face interviews, and more.
When using secondary research, researchers collect, verify, analyze and incorporate it to help them confirm research goals for the research period.
As well as the above, it can be used to review previous research into an area of interest. Researchers can look for patterns across data spanning several years and identify trends — or use it to verify early hypothesis statements and establish whether it’s worth continuing research into a prospective area.
How to conduct secondary research
There are five key steps to conducting secondary research effectively and efficiently:
1. Identify and define the research topic
First, understand what you will be researching and define the topic by thinking about the research questions you want to be answered.
Ask yourself: What is the point of conducting this research? Then, ask: What do we want to achieve?
This may indicate an exploratory reason (why something happened) or confirm a hypothesis. The answers may indicate ideas that need primary or secondary research (or a combination) to investigate them.
2. Find research and existing data sources
If secondary research is needed, think about where you might find the information. This helps you narrow down your secondary sources to those that help you answer your questions. What keywords do you need to use?
Which organizations are closely working on this topic already? Are there any competitors that you need to be aware of?
Create a list of the data sources, information, and people that could help you with your work.
3. Begin searching and collecting the existing data
Now that you have the list of data sources, start accessing the data and collect the information into an organized system. This may mean you start setting up research journal accounts or making telephone calls to book meetings with third-party research teams to verify the details around data results.
As you search and access information, remember to check the data’s date, the credibility of the source, the relevance of the material to your research topic, and the methodology used by the third-party researchers. Start small and as you gain results, investigate further in the areas that help your research’s aims.
4. Combine the data and compare the results
When you have your data in one place, you need to understand, filter, order, and combine it intelligently. Data may come in different formats where some data could be unusable, while other information may need to be deleted.
After this, you can start to look at different data sets to see what they tell you. You may find that you need to compare the same datasets over different periods for changes over time or compare different datasets to notice overlaps or trends. Ask yourself: What does this data mean to my research? Does it help or hinder my research?
5. Analyze your data and explore further
In this last stage of the process, look at the information you have and ask yourself if this answers your original questions for your research. Are there any gaps? Do you understand the information you’ve found? If you feel there is more to cover, repeat the steps and delve deeper into the topic so that you can get all the information you need.
If secondary research can’t provide these answers, consider supplementing your results with data gained from primary research. As you explore further, add to your knowledge and update your findings. This will help you present clear, credible information.
Primary vs secondary research
Unlike secondary research, primary research involves creating data first-hand by directly working with interviewees, target users, or a target market. Primary research focuses on the method for carrying out research, asking questions, and collecting data using approaches such as:
- Interviews (panel, face-to-face or over the phone)
- Questionnaires or surveys
- Focus groups
Using these methods, researchers can get in-depth, targeted responses to questions, making results more accurate and specific to their research goals. However, it does take time to do and administer.
Unlike primary research, secondary research uses existing data, which also includes published results from primary research. Researchers summarize the existing research and use the results to support their research goals.
Both primary and secondary research have their places. Primary research can support the findings found through secondary research (and fill knowledge gaps), while secondary research can be a starting point for further primary research. Because of this, these research methods are often combined for optimal research results that are accurate at both the micro and macro level.
First-hand research to collect data. May require a lot of time | The research collects existing, published data. May require a little time |
Creates raw data that the researcher owns | The researcher has no control over data method or ownership |
Relevant to the goals of the research | May not be relevant to the goals of the research |
The researcher conducts research. May be subject to researcher bias | The researcher collects results. No information on what researcher bias existsSources of secondary research |
Can be expensive to carry out | More affordable due to access to free data |
Sources of Secondary Research
There are two types of secondary research sources: internal and external. Internal data refers to in-house data that can be gathered from the researcher’s organization. External data refers to data published outside of and not owned by the researcher’s organization.
Internal data
Internal data is a good first port of call for insights and knowledge, as you may already have relevant information stored in your systems. Because you own this information — and it won’t be available to other researchers — it can give you a competitive edge . Examples of internal data include:
- Database information on sales history and business goal conversions
- Information from website applications and mobile site data
- Customer-generated data on product and service efficiency and use
- Previous research results or supplemental research areas
- Previous campaign results
External data
External data is useful when you: 1) need information on a new topic, 2) want to fill in gaps in your knowledge, or 3) want data that breaks down a population or market for trend and pattern analysis. Examples of external data include:
- Government, non-government agencies, and trade body statistics
- Company reports and research
- Competitor research
- Public library collections
- Textbooks and research journals
- Media stories in newspapers
- Online journals and research sites
Three examples of secondary research methods in action
How and why might you conduct secondary research? Let’s look at a few examples:
1. Collecting factual information from the internet on a specific topic or market
There are plenty of sites that hold data for people to view and use in their research. For example, Google Scholar, ResearchGate, or Wiley Online Library all provide previous research on a particular topic. Researchers can create free accounts and use the search facilities to look into a topic by keyword, before following the instructions to download or export results for further analysis.
This can be useful for exploring a new market that your organization wants to consider entering. For instance, by viewing the U.S Census Bureau demographic data for that area, you can see what the demographics of your target audience are , and create compelling marketing campaigns accordingly.
2. Finding out the views of your target audience on a particular topic
If you’re interested in seeing the historical views on a particular topic, for example, attitudes to women’s rights in the US, you can turn to secondary sources.
Textbooks, news articles, reviews, and journal entries can all provide qualitative reports and interviews covering how people discussed women’s rights. There may be multimedia elements like video or documented posters of propaganda showing biased language usage.
By gathering this information, synthesizing it, and evaluating the language, who created it and when it was shared, you can create a timeline of how a topic was discussed over time.
3. When you want to know the latest thinking on a topic
Educational institutions, such as schools and colleges, create a lot of research-based reports on younger audiences or their academic specialisms. Dissertations from students also can be submitted to research journals, making these places useful places to see the latest insights from a new generation of academics.
Information can be requested — and sometimes academic institutions may want to collaborate and conduct research on your behalf. This can provide key primary data in areas that you want to research, as well as secondary data sources for your research.
Advantages of secondary research
There are several benefits of using secondary research, which we’ve outlined below:
- Easily and readily available data – There is an abundance of readily accessible data sources that have been pre-collected for use, in person at local libraries and online using the internet. This data is usually sorted by filters or can be exported into spreadsheet format, meaning that little technical expertise is needed to access and use the data.
- Faster research speeds – Since the data is already published and in the public arena, you don’t need to collect this information through primary research. This can make the research easier to do and faster, as you can get started with the data quickly.
- Low financial and time costs – Most secondary data sources can be accessed for free or at a small cost to the researcher, so the overall research costs are kept low. In addition, by saving on preliminary research, the time costs for the researcher are kept down as well.
- Secondary data can drive additional research actions – The insights gained can support future research activities (like conducting a follow-up survey or specifying future detailed research topics) or help add value to these activities.
- Secondary data can be useful pre-research insights – Secondary source data can provide pre-research insights and information on effects that can help resolve whether research should be conducted. It can also help highlight knowledge gaps, so subsequent research can consider this.
- Ability to scale up results – Secondary sources can include large datasets (like Census data results across several states) so research results can be scaled up quickly using large secondary data sources.
Disadvantages of secondary research
The disadvantages of secondary research are worth considering in advance of conducting research :
- Secondary research data can be out of date – Secondary sources can be updated regularly, but if you’re exploring the data between two updates, the data can be out of date. Researchers will need to consider whether the data available provides the right research coverage dates, so that insights are accurate and timely, or if the data needs to be updated. Also, fast-moving markets may find secondary data expires very quickly.
- Secondary research needs to be verified and interpreted – Where there’s a lot of data from one source, a researcher needs to review and analyze it. The data may need to be verified against other data sets or your hypotheses for accuracy and to ensure you’re using the right data for your research.
- The researcher has had no control over the secondary research – As the researcher has not been involved in the secondary research, invalid data can affect the results. It’s therefore vital that the methodology and controls are closely reviewed so that the data is collected in a systematic and error-free way.
- Secondary research data is not exclusive – As data sets are commonly available, there is no exclusivity and many researchers can use the same data. This can be problematic where researchers want to have exclusive rights over the research results and risk duplication of research in the future.
When do we conduct secondary research?
Now that you know the basics of secondary research, when do researchers normally conduct secondary research?
It’s often used at the beginning of research, when the researcher is trying to understand the current landscape . In addition, if the research area is new to the researcher, it can form crucial background context to help them understand what information exists already. This can plug knowledge gaps, supplement the researcher’s own learning or add to the research.
Secondary research can also be used in conjunction with primary research. Secondary research can become the formative research that helps pinpoint where further primary research is needed to find out specific information. It can also support or verify the findings from primary research.
You can use secondary research where high levels of control aren’t needed by the researcher, but a lot of knowledge on a topic is required from different angles.
Secondary research should not be used in place of primary research as both are very different and are used for various circumstances.
Questions to ask before conducting secondary research
Before you start your secondary research, ask yourself these questions:
- Is there similar internal data that we have created for a similar area in the past?
If your organization has past research, it’s best to review this work before starting a new project. The older work may provide you with the answers, and give you a starting dataset and context of how your organization approached the research before. However, be mindful that the work is probably out of date and view it with that note in mind. Read through and look for where this helps your research goals or where more work is needed.
- What am I trying to achieve with this research?
When you have clear goals, and understand what you need to achieve, you can look for the perfect type of secondary or primary research to support the aims. Different secondary research data will provide you with different information – for example, looking at news stories to tell you a breakdown of your market’s buying patterns won’t be as useful as internal or external data e-commerce and sales data sources.
- How credible will my research be?
If you are looking for credibility, you want to consider how accurate the research results will need to be, and if you can sacrifice credibility for speed by using secondary sources to get you started. Bear in mind which sources you choose — low-credibility data sites, like political party websites that are highly biased to favor their own party, would skew your results.
- What is the date of the secondary research?
When you’re looking to conduct research, you want the results to be as useful as possible , so using data that is 10 years old won’t be as accurate as using data that was created a year ago. Since a lot can change in a few years, note the date of your research and look for earlier data sets that can tell you a more recent picture of results. One caveat to this is using data collected over a long-term period for comparisons with earlier periods, which can tell you about the rate and direction of change.
- Can the data sources be verified? Does the information you have check out?
If you can’t verify the data by looking at the research methodology, speaking to the original team or cross-checking the facts with other research, it could be hard to be sure that the data is accurate. Think about whether you can use another source, or if it’s worth doing some supplementary primary research to replicate and verify results to help with this issue.
We created a front-to-back guide on conducting market research, The ultimate guide to conducting market research , so you can understand the research journey with confidence.
In it, you’ll learn more about:
- What effective market research looks like
- The use cases for market research
- The most important steps to conducting market research
- And how to take action on your research findings
Download the free guide for a clearer view on secondary research and other key research types for your business.
Related resources
Mixed methods research 17 min read, market intelligence 10 min read, marketing insights 11 min read, ethnographic research 11 min read, qualitative vs quantitative research 13 min read, qualitative research questions 11 min read, qualitative research design 12 min read, request demo.
Ready to learn more about Qualtrics?
How to Analyse Secondary Data for a Dissertation
Secondary data refers to data that has already been collected by another researcher. For researchers (and students!) with limited time and resources, secondary data, whether qualitative or quantitative can be a highly viable source of data. In addition, with the advances in technology and access to peer reviewed journals and studies provided by the internet, it is increasingly popular as a form of data collection. The question that frequently arises amongst students however, is: how is secondary data best analysed?
The process of data analysis in secondary research
Secondary analysis (i.e., the use of existing data) is a systematic methodological approach that has some clear steps that need to be followed for the process to be effective. In simple terms there are three steps:
- Step One: Development of Research Questions
- Step Two: Identification of dataset
- Step Three: Evaluation of the dataset.
Let’s look at each of these in more detail:
Step One: Development of research questions
Using secondary data means you need to apply theoretical knowledge and conceptual skills to be able to use the dataset to answer research questions. Clearly therefore, the first step is thus to clearly define and develop your research questions so that you know the areas of interest that you need to explore for location of the most appropriate secondary data.
Step Two: Identification of Dataset
This stage should start with identification, through investigation, of what is currently known in the subject area and where there are gaps, and thus what data is available to address these gaps. Sources can be academic from prior studies that have used quantitative or qualitative data, and which can then be gathered together and collated to produce a new secondary dataset. In addition, other more informal or “grey” literature can also be incorporated, including consumer report, commercial studies or similar. One of the values of using secondary research is that original survey works often do not use all the data collected which means this unused information can be applied to different settings or perspectives.
Key point: Effective use of secondary data means identifying how the data can be used to deliver meaningful and relevant answers to the research questions. In other words that the data used is a good fit for the study and research questions.
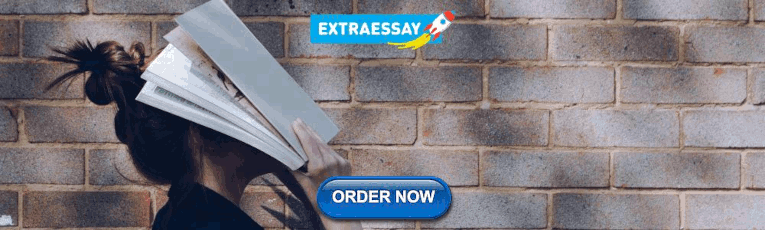
Step Three: Evaluation of the dataset for effectiveness/fit
A good tip is to use a reflective approach for data evaluation. In other words, for each piece of secondary data to be utilised, it is sensible to identify the purpose of the work, the credentials of the authors (i.e., credibility, what data is provided in the original work and how long ago it was collected). In addition, the methods used and the level of consistency that exists compared to other works. This is important because understanding the primary method of data collection will impact on the overall evaluation and analysis when it is used as secondary source. In essence, if there is no understanding of the coding used in qualitative data analysis to identify key themes then there will be a mismatch with interpretations when the data is used for secondary purposes. Furthermore, having multiple sources which draw similar conclusions ensures a higher level of validity than relying on only one or two secondary sources.
A useful framework provides a flow chart of decision making, as shown in the figure below.
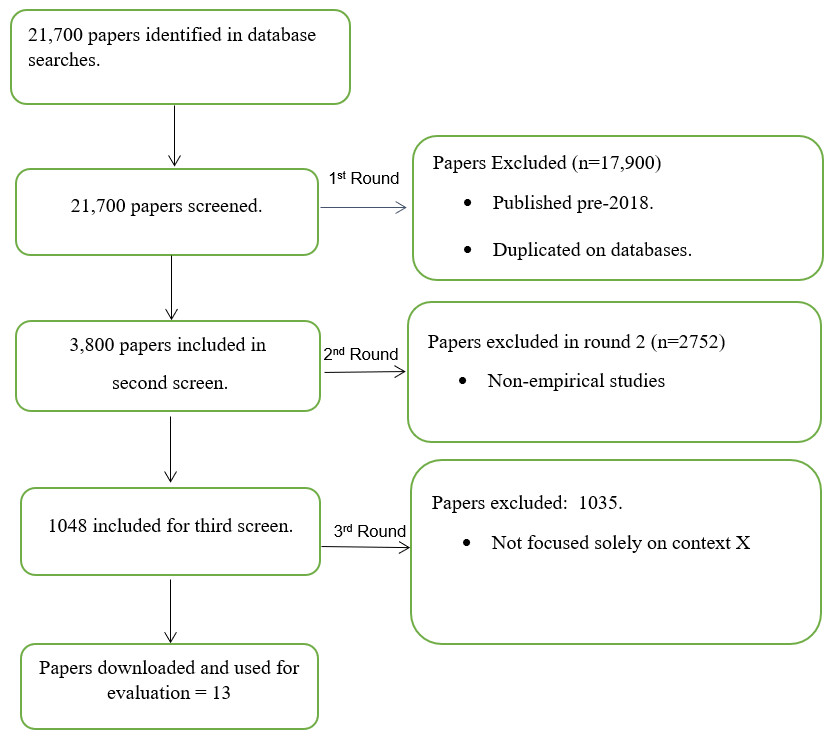
Following this process ensures that only those that are most appropriate for your research questions are included in the final dataset, but also demonstrates to your readers that you have been thorough in identifying the right works to use.
Writing up the Analysis
Once you have your dataset, writing up the analysis will depend on the process used. If the data is qualitative in nature, then you should follow the following process.
Pre-Planning
- Read and re-read all sources, identifying initial observations, correlations, and relationships between themes and how they apply to your research questions.
- Once initial themes are identified, it is sensible to explore further and identify sub-themes which lead on from the core themes and correlations in the dataset, which encourages identification of new insights and contributes to the originality of your own work.
Structure of the Analysis Presentation
Introduction.
The introduction should commence with an overview of all your sources. It is good practice to present these in a table, listed chronologically so that your work has an orderly and consistent flow. The introduction should also incorporate a brief (2-3 sentences) overview of the key outcomes and results identified.
The body text for secondary data, irrespective of whether quantitative or qualitative data is used, should be broken up into sub-sections for each argument or theme presented. In the case of qualitative data, depending on whether content, narrative or discourse analysis is used, this means presenting the key papers in the area, their conclusions and how these answer, or not, your research questions. Each source should be clearly cited and referenced at the end of the work. In the case of qualitative data, any figures or tables should be reproduced with the correct citations to their original source. In both cases, it is good practice to give a main heading of a key theme, with sub-headings for each of the sub themes identified in the analysis.
Do not use direct quotes from secondary data unless they are:
- properly referenced, and
- are key to underlining a point or conclusion that you have drawn from the data.
All results sections, regardless of whether primary or secondary data has been used should refer back to the research questions and prior works. This is because, regardless of whether the results back up or contradict previous research, including previous works shows a wider level of reading and understanding of the topic being researched and gives a greater depth to your own work.
Summary of results
The summary of the results section of a secondary data dissertation should deliver a summing up of key findings, and if appropriate a conceptual framework that clearly illustrates the findings of the work. This shows that you have understood your secondary data, how it has answered your research questions, and furthermore that your interpretation has led to some firm outcomes.
- Skip to main content
- Skip to primary sidebar
- Skip to footer
- QuestionPro

- Solutions Industries Gaming Automotive Sports and events Education Government Travel & Hospitality Financial Services Healthcare Cannabis Technology Use Case AskWhy Communities Audience Contactless surveys Mobile LivePolls Member Experience GDPR Positive People Science 360 Feedback Surveys
- Resources Blog eBooks Survey Templates Case Studies Training Help center

Home Market Research
Secondary Research: Definition, Methods and Examples.
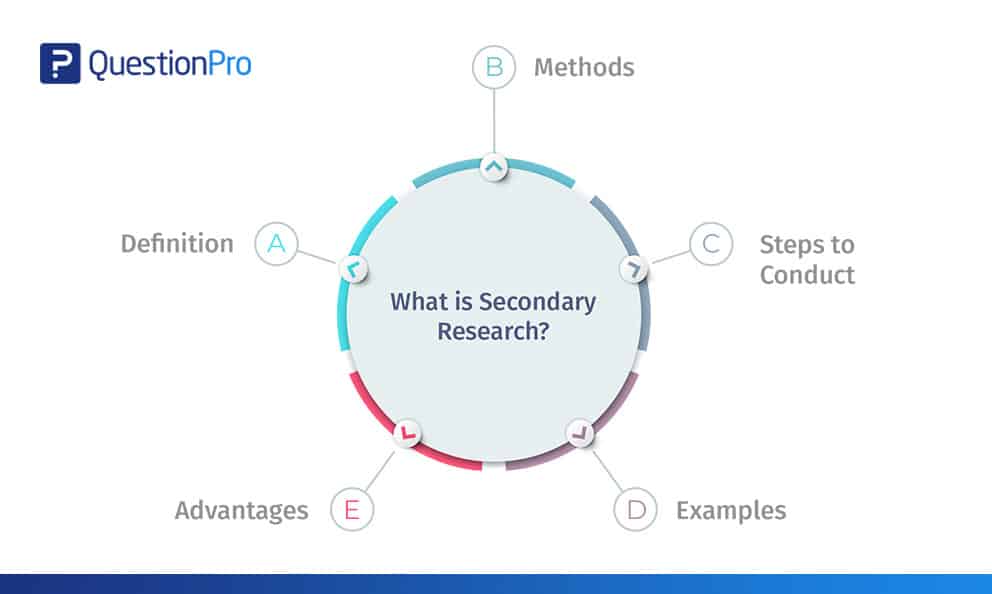
In the world of research, there are two main types of data sources: primary and secondary. While primary research involves collecting new data directly from individuals or sources, secondary research involves analyzing existing data already collected by someone else. Today we’ll discuss secondary research.
One common source of this research is published research reports and other documents. These materials can often be found in public libraries, on websites, or even as data extracted from previously conducted surveys. In addition, many government and non-government agencies maintain extensive data repositories that can be accessed for research purposes.
LEARN ABOUT: Research Process Steps
While secondary research may not offer the same level of control as primary research, it can be a highly valuable tool for gaining insights and identifying trends. Researchers can save time and resources by leveraging existing data sources while still uncovering important information.
What is Secondary Research: Definition
Secondary research is a research method that involves using already existing data. Existing data is summarized and collated to increase the overall effectiveness of the research.
One of the key advantages of secondary research is that it allows us to gain insights and draw conclusions without having to collect new data ourselves. This can save time and resources and also allow us to build upon existing knowledge and expertise.
When conducting secondary research, it’s important to be thorough and thoughtful in our approach. This means carefully selecting the sources and ensuring that the data we’re analyzing is reliable and relevant to the research question . It also means being critical and analytical in the analysis and recognizing any potential biases or limitations in the data.
LEARN ABOUT: Level of Analysis
Secondary research is much more cost-effective than primary research , as it uses already existing data, unlike primary research, where data is collected firsthand by organizations or businesses or they can employ a third party to collect data on their behalf.
LEARN ABOUT: Data Analytics Projects
Secondary Research Methods with Examples
Secondary research is cost-effective, one of the reasons it is a popular choice among many businesses and organizations. Not every organization is able to pay a huge sum of money to conduct research and gather data. So, rightly secondary research is also termed “ desk research ”, as data can be retrieved from sitting behind a desk.
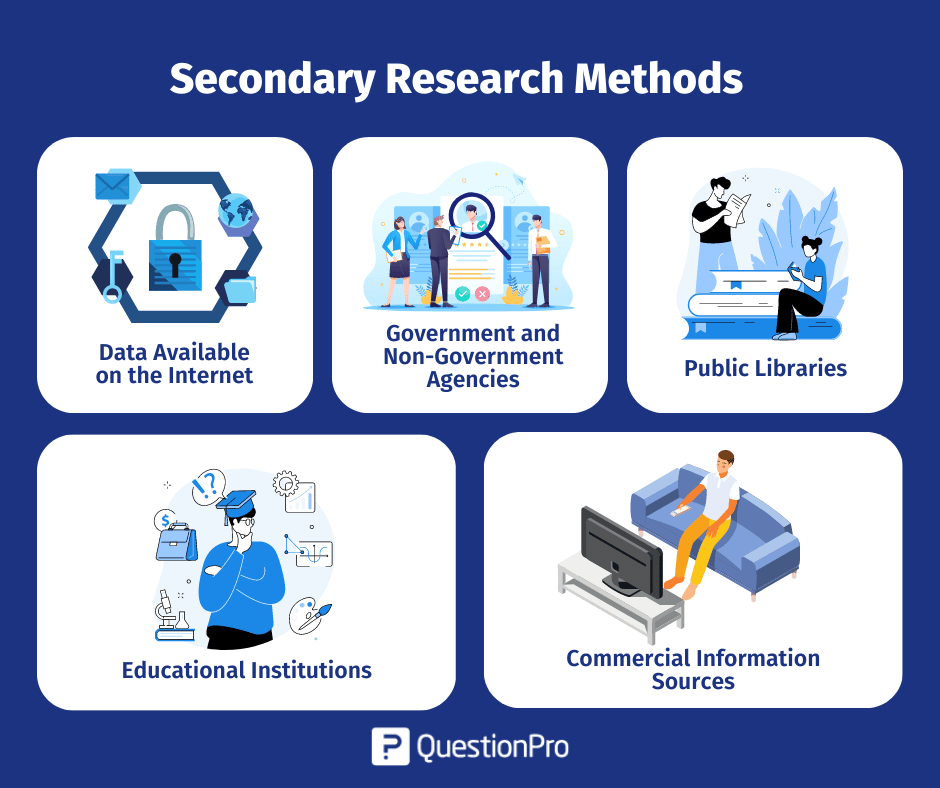
The following are popularly used secondary research methods and examples:
1. Data Available on The Internet
One of the most popular ways to collect secondary data is the internet. Data is readily available on the internet and can be downloaded at the click of a button.
This data is practically free of cost, or one may have to pay a negligible amount to download the already existing data. Websites have a lot of information that businesses or organizations can use to suit their research needs. However, organizations need to consider only authentic and trusted website to collect information.
2. Government and Non-Government Agencies
Data for secondary research can also be collected from some government and non-government agencies. For example, US Government Printing Office, US Census Bureau, and Small Business Development Centers have valuable and relevant data that businesses or organizations can use.
There is a certain cost applicable to download or use data available with these agencies. Data obtained from these agencies are authentic and trustworthy.
3. Public Libraries
Public libraries are another good source to search for data for this research. Public libraries have copies of important research that were conducted earlier. They are a storehouse of important information and documents from which information can be extracted.
The services provided in these public libraries vary from one library to another. More often, libraries have a huge collection of government publications with market statistics, large collection of business directories and newsletters.
4. Educational Institutions
Importance of collecting data from educational institutions for secondary research is often overlooked. However, more research is conducted in colleges and universities than any other business sector.
The data that is collected by universities is mainly for primary research. However, businesses or organizations can approach educational institutions and request for data from them.
5. Commercial Information Sources
Local newspapers, journals, magazines, radio and TV stations are a great source to obtain data for secondary research. These commercial information sources have first-hand information on economic developments, political agenda, market research, demographic segmentation and similar subjects.
Businesses or organizations can request to obtain data that is most relevant to their study. Businesses not only have the opportunity to identify their prospective clients but can also know about the avenues to promote their products or services through these sources as they have a wider reach.
Learn More: Data Collection Methods: Types & Examples
Key Differences between Primary Research and Secondary Research
Understanding the distinction between primary research and secondary research is essential in determining which research method is best for your project. These are the two main types of research methods, each with advantages and disadvantages. In this section, we will explore the critical differences between the two and when it is appropriate to use them.
Research is conducted first hand to obtain data. Researcher “owns” the data collected. | Research is based on data collected from previous researches. |
is based on raw data. | Secondary research is based on tried and tested data which is previously analyzed and filtered. |
The data collected fits the needs of a researcher, it is customized. Data is collected based on the absolute needs of organizations or businesses. | Data may or may not be according to the requirement of a researcher. |
Researcher is deeply involved in research to collect data in primary research. | As opposed to primary research, secondary research is fast and easy. It aims at gaining a broader understanding of subject matter. |
Primary research is an expensive process and consumes a lot of time to collect and analyze data. | Secondary research is a quick process as data is already available. Researcher should know where to explore to get most appropriate data. |
How to Conduct Secondary Research?
We have already learned about the differences between primary and secondary research. Now, let’s take a closer look at how to conduct it.
Secondary research is an important tool for gathering information already collected and analyzed by others. It can help us save time and money and allow us to gain insights into the subject we are researching. So, in this section, we will discuss some common methods and tips for conducting it effectively.
Here are the steps involved in conducting secondary research:
1. Identify the topic of research: Before beginning secondary research, identify the topic that needs research. Once that’s done, list down the research attributes and its purpose.
2. Identify research sources: Next, narrow down on the information sources that will provide most relevant data and information applicable to your research.
3. Collect existing data: Once the data collection sources are narrowed down, check for any previous data that is available which is closely related to the topic. Data related to research can be obtained from various sources like newspapers, public libraries, government and non-government agencies etc.
4. Combine and compare: Once data is collected, combine and compare the data for any duplication and assemble data into a usable format. Make sure to collect data from authentic sources. Incorrect data can hamper research severely.
4. Analyze data: Analyze collected data and identify if all questions are answered. If not, repeat the process if there is a need to dwell further into actionable insights.
Advantages of Secondary Research
Secondary research offers a number of advantages to researchers, including efficiency, the ability to build upon existing knowledge, and the ability to conduct research in situations where primary research may not be possible or ethical. By carefully selecting their sources and being thoughtful in their approach, researchers can leverage secondary research to drive impact and advance the field. Some key advantages are the following:
1. Most information in this research is readily available. There are many sources from which relevant data can be collected and used, unlike primary research, where data needs to collect from scratch.
2. This is a less expensive and less time-consuming process as data required is easily available and doesn’t cost much if extracted from authentic sources. A minimum expenditure is associated to obtain data.
3. The data that is collected through secondary research gives organizations or businesses an idea about the effectiveness of primary research. Hence, organizations or businesses can form a hypothesis and evaluate cost of conducting primary research.
4. Secondary research is quicker to conduct because of the availability of data. It can be completed within a few weeks depending on the objective of businesses or scale of data needed.
As we can see, this research is the process of analyzing data already collected by someone else, and it can offer a number of benefits to researchers.
Disadvantages of Secondary Research
On the other hand, we have some disadvantages that come with doing secondary research. Some of the most notorious are the following:
1. Although data is readily available, credibility evaluation must be performed to understand the authenticity of the information available.
2. Not all secondary data resources offer the latest reports and statistics. Even when the data is accurate, it may not be updated enough to accommodate recent timelines.
3. Secondary research derives its conclusion from collective primary research data. The success of your research will depend, to a greater extent, on the quality of research already conducted by primary research.
LEARN ABOUT: 12 Best Tools for Researchers
In conclusion, secondary research is an important tool for researchers exploring various topics. By leveraging existing data sources, researchers can save time and resources, build upon existing knowledge, and conduct research in situations where primary research may not be feasible.
There are a variety of methods and examples of secondary research, from analyzing public data sets to reviewing previously published research papers. As students and aspiring researchers, it’s important to understand the benefits and limitations of this research and to approach it thoughtfully and critically. By doing so, we can continue to advance our understanding of the world around us and contribute to meaningful research that positively impacts society.
QuestionPro can be a useful tool for conducting secondary research in a variety of ways. You can create online surveys that target a specific population, collecting data that can be analyzed to gain insights into consumer behavior, attitudes, and preferences; analyze existing data sets that you have obtained through other means or benchmark your organization against others in your industry or against industry standards. The software provides a range of benchmarking tools that can help you compare your performance on key metrics, such as customer satisfaction, with that of your peers.
Using QuestionPro thoughtfully and strategically allows you to gain valuable insights to inform decision-making and drive business success. Start today for free! No credit card is required.
LEARN MORE FREE TRIAL
MORE LIKE THIS
Top 5 change management models to transform your organization.
Sep 20, 2024
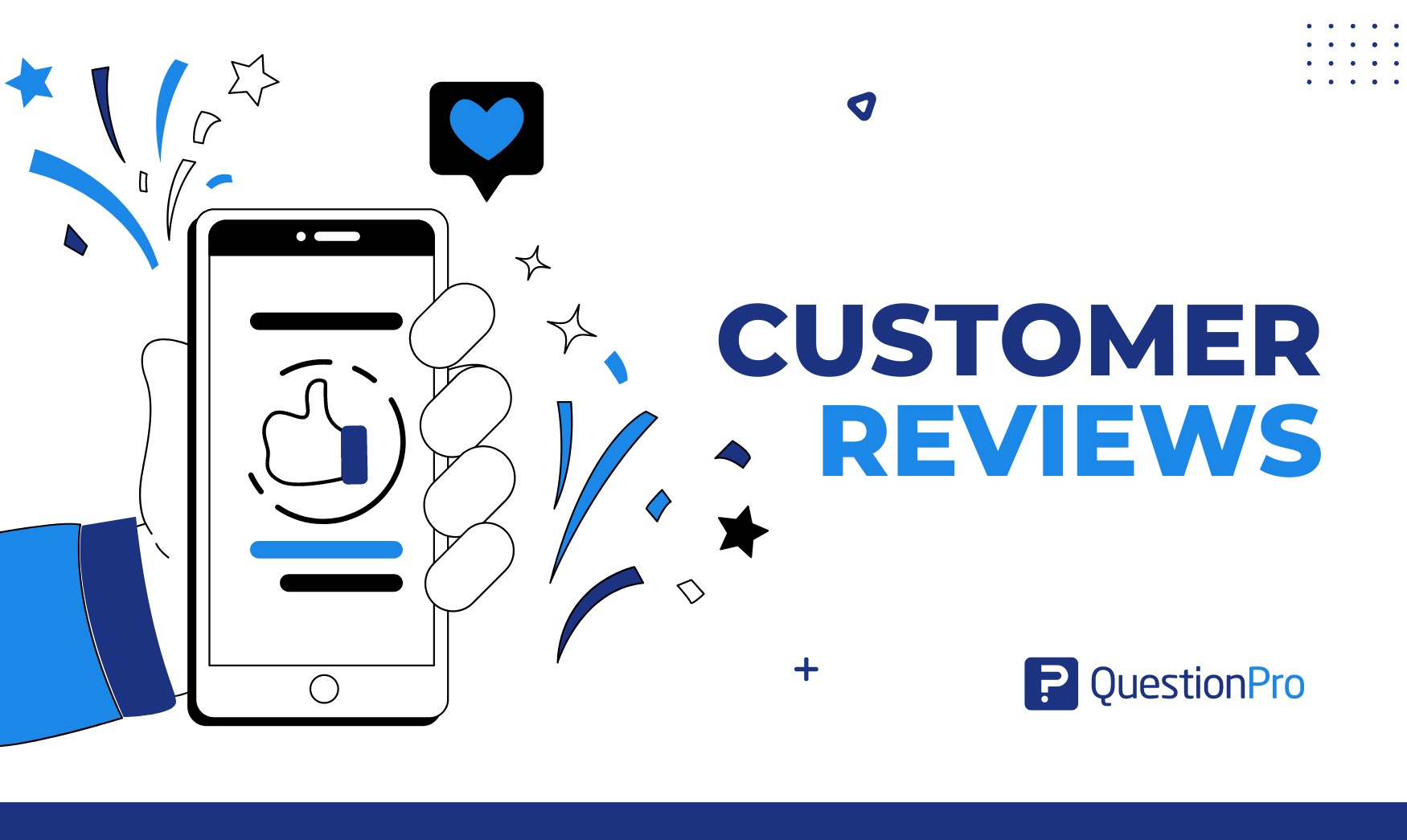
Customer Reviews: How to Ask and Easy Ways to Get Them
Sep 19, 2024
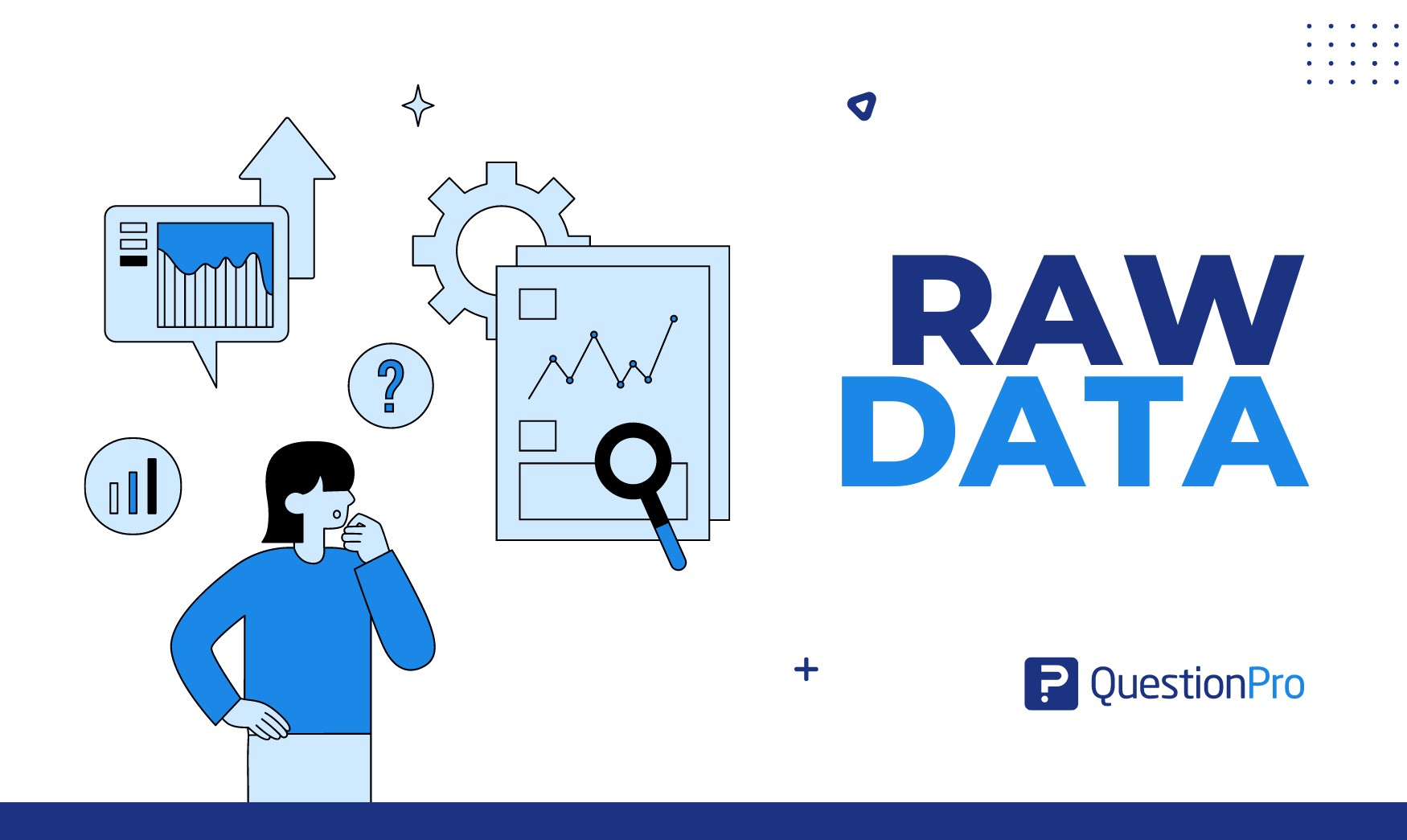
Raw Data: What it is + How to Process It
Sep 18, 2024
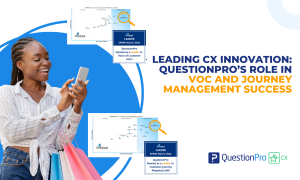
QuestionPro: Leading the Charge in Customer Journey Management and Voice of the Customer Platforms
Sep 17, 2024
Other categories
- Academic Research
- Artificial Intelligence
- Assessments
- Brand Awareness
- Case Studies
- Communities
- Consumer Insights
- Customer effort score
- Customer Engagement
- Customer Experience
- Customer Loyalty
- Customer Research
- Customer Satisfaction
- Employee Benefits
- Employee Engagement
- Employee Retention
- Friday Five
- General Data Protection Regulation
- Insights Hub
- Life@QuestionPro
- Market Research
- Mobile diaries
- Mobile Surveys
- New Features
- Online Communities
- Question Types
- Questionnaire
- QuestionPro Products
- Release Notes
- Research Tools and Apps
- Revenue at Risk
- Survey Templates
- Training Tips
- Tuesday CX Thoughts (TCXT)
- Uncategorized
- What’s Coming Up
- Workforce Intelligence
- Privacy Policy

Home » Secondary Data – Types, Methods and Examples
Secondary Data – Types, Methods and Examples
Table of Contents

Secondary Data
Definition:
Secondary data refers to information that has been collected, processed, and published by someone else, rather than the researcher gathering the data firsthand. This can include data from sources such as government publications, academic journals, market research reports, and other existing datasets.
Secondary Data Types
Types of secondary data are as follows:
- Published data: Published data refers to data that has been published in books, magazines, newspapers, and other print media. Examples include statistical reports, market research reports, and scholarly articles.
- Government data: Government data refers to data collected by government agencies and departments. This can include data on demographics, economic trends, crime rates, and health statistics.
- Commercial data: Commercial data is data collected by businesses for their own purposes. This can include sales data, customer feedback, and market research data.
- Academic data: Academic data refers to data collected by researchers for academic purposes. This can include data from experiments, surveys, and observational studies.
- Online data: Online data refers to data that is available on the internet. This can include social media posts, website analytics, and online customer reviews.
- Organizational data: Organizational data is data collected by businesses or organizations for their own purposes. This can include data on employee performance, financial records, and customer satisfaction.
- Historical data : Historical data refers to data that was collected in the past and is still available for research purposes. This can include census data, historical documents, and archival records.
- International data: International data refers to data collected from other countries for research purposes. This can include data on international trade, health statistics, and demographic trends.
- Public data : Public data refers to data that is available to the general public. This can include data from government agencies, non-profit organizations, and other sources.
- Private data: Private data refers to data that is not available to the general public. This can include confidential business data, personal medical records, and financial data.
- Big data: Big data refers to large, complex datasets that are difficult to manage and analyze using traditional data processing methods. This can include social media data, sensor data, and other types of data generated by digital devices.
Secondary Data Collection Methods
Secondary Data Collection Methods are as follows:
- Published sources: Researchers can gather secondary data from published sources such as books, journals, reports, and newspapers. These sources often provide comprehensive information on a variety of topics.
- Online sources: With the growth of the internet, researchers can now access a vast amount of secondary data online. This includes websites, databases, and online archives.
- Government sources : Government agencies often collect and publish a wide range of secondary data on topics such as demographics, crime rates, and health statistics. Researchers can obtain this data through government websites, publications, or data portals.
- Commercial sources: Businesses often collect and analyze data for marketing research or customer profiling. Researchers can obtain this data through commercial data providers or by purchasing market research reports.
- Academic sources: Researchers can also obtain secondary data from academic sources such as published research studies, academic journals, and dissertations.
- Personal contacts: Researchers can also obtain secondary data from personal contacts, such as experts in a particular field or individuals with specialized knowledge.
Secondary Data Formats
Secondary data can come in various formats depending on the source from which it is obtained. Here are some common formats of secondary data:
- Numeric Data: Numeric data is often in the form of statistics and numerical figures that have been compiled and reported by organizations such as government agencies, research institutions, and commercial enterprises. This can include data such as population figures, GDP, sales figures, and market share.
- Textual Data: Textual data is often in the form of written documents, such as reports, articles, and books. This can include qualitative data such as descriptions, opinions, and narratives.
- Audiovisual Data : Audiovisual data is often in the form of recordings, videos, and photographs. This can include data such as interviews, focus group discussions, and other types of qualitative data.
- Geospatial Data: Geospatial data is often in the form of maps, satellite images, and geographic information systems (GIS) data. This can include data such as demographic information, land use patterns, and transportation networks.
- Transactional Data : Transactional data is often in the form of digital records of financial and business transactions. This can include data such as purchase histories, customer behavior, and financial transactions.
- Social Media Data: Social media data is often in the form of user-generated content from social media platforms such as Facebook, Twitter, and Instagram. This can include data such as user demographics, content trends, and sentiment analysis.
Secondary Data Analysis Methods
Secondary data analysis involves the use of pre-existing data for research purposes. Here are some common methods of secondary data analysis:
- Descriptive Analysis: This method involves describing the characteristics of a dataset, such as the mean, standard deviation, and range of the data. Descriptive analysis can be used to summarize data and provide an overview of trends.
- Inferential Analysis: This method involves making inferences and drawing conclusions about a population based on a sample of data. Inferential analysis can be used to test hypotheses and determine the statistical significance of relationships between variables.
- Content Analysis: This method involves analyzing textual or visual data to identify patterns and themes. Content analysis can be used to study the content of documents, media coverage, and social media posts.
- Time-Series Analysis : This method involves analyzing data over time to identify trends and patterns. Time-series analysis can be used to study economic trends, climate change, and other phenomena that change over time.
- Spatial Analysis : This method involves analyzing data in relation to geographic location. Spatial analysis can be used to study patterns of disease spread, land use patterns, and the effects of environmental factors on health outcomes.
- Meta-Analysis: This method involves combining data from multiple studies to draw conclusions about a particular phenomenon. Meta-analysis can be used to synthesize the results of previous research and provide a more comprehensive understanding of a particular topic.
Secondary Data Gathering Guide
Here are some steps to follow when gathering secondary data:
- Define your research question: Start by defining your research question and identifying the specific information you need to answer it. This will help you identify the type of secondary data you need and where to find it.
- Identify relevant sources: Identify potential sources of secondary data, including published sources, online databases, government sources, and commercial data providers. Consider the reliability and validity of each source.
- Evaluate the quality of the data: Evaluate the quality and reliability of the data you plan to use. Consider the data collection methods, sample size, and potential biases. Make sure the data is relevant to your research question and is suitable for the type of analysis you plan to conduct.
- Collect the data: Collect the relevant data from the identified sources. Use a consistent method to record and organize the data to make analysis easier.
- Validate the data: Validate the data to ensure that it is accurate and reliable. Check for inconsistencies, missing data, and errors. Address any issues before analyzing the data.
- Analyze the data: Analyze the data using appropriate statistical and analytical methods. Use descriptive and inferential statistics to summarize and draw conclusions from the data.
- Interpret the results: Interpret the results of your analysis and draw conclusions based on the data. Make sure your conclusions are supported by the data and are relevant to your research question.
- Communicate the findings : Communicate your findings clearly and concisely. Use appropriate visual aids such as graphs and charts to help explain your results.
Examples of Secondary Data
Here are some examples of secondary data from different fields:
- Healthcare : Hospital records, medical journals, clinical trial data, and disease registries are examples of secondary data sources in healthcare. These sources can provide researchers with information on patient demographics, disease prevalence, and treatment outcomes.
- Marketing : Market research reports, customer surveys, and sales data are examples of secondary data sources in marketing. These sources can provide marketers with information on consumer preferences, market trends, and competitor activity.
- Education : Student test scores, graduation rates, and enrollment statistics are examples of secondary data sources in education. These sources can provide researchers with information on student achievement, teacher effectiveness, and educational disparities.
- Finance : Stock market data, financial statements, and credit reports are examples of secondary data sources in finance. These sources can provide investors with information on market trends, company performance, and creditworthiness.
- Social Science : Government statistics, census data, and survey data are examples of secondary data sources in social science. These sources can provide researchers with information on population demographics, social trends, and political attitudes.
- Environmental Science : Climate data, remote sensing data, and ecological monitoring data are examples of secondary data sources in environmental science. These sources can provide researchers with information on weather patterns, land use, and biodiversity.
Purpose of Secondary Data
The purpose of secondary data is to provide researchers with information that has already been collected by others for other purposes. Secondary data can be used to support research questions, test hypotheses, and answer research objectives. Some of the key purposes of secondary data are:
- To gain a better understanding of the research topic : Secondary data can be used to provide context and background information on a research topic. This can help researchers understand the historical and social context of their research and gain insights into relevant variables and relationships.
- To save time and resources: Collecting new primary data can be time-consuming and expensive. Using existing secondary data sources can save researchers time and resources by providing access to pre-existing data that has already been collected and organized.
- To provide comparative data : Secondary data can be used to compare and contrast findings across different studies or datasets. This can help researchers identify trends, patterns, and relationships that may not have been apparent from individual studies.
- To support triangulation: Triangulation is the process of using multiple sources of data to confirm or refute research findings. Secondary data can be used to support triangulation by providing additional sources of data to support or refute primary research findings.
- To supplement primary data : Secondary data can be used to supplement primary data by providing additional information or insights that were not captured by the primary research. This can help researchers gain a more complete understanding of the research topic and draw more robust conclusions.
When to use Secondary Data
Secondary data can be useful in a variety of research contexts, and there are several situations in which it may be appropriate to use secondary data. Some common situations in which secondary data may be used include:
- When primary data collection is not feasible : Collecting primary data can be time-consuming and expensive, and in some cases, it may not be feasible to collect primary data. In these situations, secondary data can provide valuable insights and information.
- When exploring a new research area : Secondary data can be a useful starting point for researchers who are exploring a new research area. Secondary data can provide context and background information on a research topic, and can help researchers identify key variables and relationships to explore further.
- When comparing and contrasting research findings: Secondary data can be used to compare and contrast findings across different studies or datasets. This can help researchers identify trends, patterns, and relationships that may not have been apparent from individual studies.
- When triangulating research findings: Triangulation is the process of using multiple sources of data to confirm or refute research findings. Secondary data can be used to support triangulation by providing additional sources of data to support or refute primary research findings.
- When validating research findings : Secondary data can be used to validate primary research findings by providing additional sources of data that support or refute the primary findings.
Characteristics of Secondary Data
Secondary data have several characteristics that distinguish them from primary data. Here are some of the key characteristics of secondary data:
- Non-reactive: Secondary data are non-reactive, meaning that they are not collected for the specific purpose of the research study. This means that the researcher has no control over the data collection process, and cannot influence how the data were collected.
- Time-saving: Secondary data are pre-existing, meaning that they have already been collected and organized by someone else. This can save the researcher time and resources, as they do not need to collect the data themselves.
- Wide-ranging : Secondary data sources can provide a wide range of information on a variety of topics. This can be useful for researchers who are exploring a new research area or seeking to compare and contrast research findings.
- Less expensive: Secondary data are generally less expensive than primary data, as they do not require the researcher to incur the costs associated with data collection.
- Potential for bias : Secondary data may be subject to biases that were present in the original data collection process. For example, data may have been collected using a biased sampling method or the data may be incomplete or inaccurate.
- Lack of control: The researcher has no control over the data collection process and cannot ensure that the data were collected using appropriate methods or measures.
- Requires careful evaluation : Secondary data sources must be evaluated carefully to ensure that they are appropriate for the research question and analysis. This includes assessing the quality, reliability, and validity of the data sources.
Advantages of Secondary Data
There are several advantages to using secondary data in research, including:
- Time-saving : Collecting primary data can be time-consuming and expensive. Secondary data can be accessed quickly and easily, which can save researchers time and resources.
- Cost-effective: Secondary data are generally less expensive than primary data, as they do not require the researcher to incur the costs associated with data collection.
- Large sample size : Secondary data sources often have larger sample sizes than primary data sources, which can increase the statistical power of the research.
- Access to historical data : Secondary data sources can provide access to historical data, which can be useful for researchers who are studying trends over time.
- No ethical concerns: Secondary data are already in existence, so there are no ethical concerns related to collecting data from human subjects.
- May be more objective : Secondary data may be more objective than primary data, as the data were not collected for the specific purpose of the research study.
Limitations of Secondary Data
While there are many advantages to using secondary data in research, there are also some limitations that should be considered. Some of the main limitations of secondary data include:
- Lack of control over data quality : Researchers do not have control over the data collection process, which means they cannot ensure the accuracy or completeness of the data.
- Limited availability: Secondary data may not be available for the specific research question or study design.
- Lack of information on sampling and data collection methods: Researchers may not have access to information on the sampling and data collection methods used to gather the secondary data. This can make it difficult to evaluate the quality of the data.
- Data may not be up-to-date: Secondary data may not be up-to-date or relevant to the current research question.
- Data may be incomplete or inaccurate : Secondary data may be incomplete or inaccurate due to missing or incorrect data points, data entry errors, or other factors.
- Biases in data collection: The data may have been collected using biased sampling or data collection methods, which can limit the validity of the data.
- Lack of control over variables: Researchers have limited control over the variables that were measured in the original data collection process, which can limit the ability to draw conclusions about causality.
About the author
Muhammad Hassan
Researcher, Academic Writer, Web developer
You may also like

Primary Data – Types, Methods and Examples

Quantitative Data – Types, Methods and Examples

Information in Research – Types and Examples

Qualitative Data – Types, Methods and Examples

Research Data – Types Methods and Examples

An official website of the United States government
The .gov means it’s official. Federal government websites often end in .gov or .mil. Before sharing sensitive information, make sure you’re on a federal government site.
The site is secure. The https:// ensures that you are connecting to the official website and that any information you provide is encrypted and transmitted securely.
- Publications
- Account settings
The PMC website is updating on October 15, 2024. Learn More or Try it out now .
- Advanced Search
- Journal List
- HHS Author Manuscripts

Qualitative Secondary Analysis: A Case Exemplar
Judith ann tate.
The Ohio State University, College of Nursing
Mary Beth Happ
Qualitative secondary analysis (QSA) is the use of qualitative data collected by someone else or to answer a different research question. Secondary analysis of qualitative data provides an opportunity to maximize data utility particularly with difficult to reach patient populations. However, QSA methods require careful consideration and explicit description to best understand, contextualize, and evaluate the research results. In this paper, we describe methodologic considerations using a case exemplar to illustrate challenges specific to QSA and strategies to overcome them.
Health care research requires significant time and resources. Secondary analysis of existing data provides an efficient alternative to collecting data from new groups or the same subjects. Secondary analysis, defined as the reuse of existing data to investigate a different research question ( Heaton, 2004 ), has a similar purpose whether the data are quantitative or qualitative. Common goals include to (1) perform additional analyses on the original dataset, (2) analyze a subset of the original data, (3) apply a new perspective or focus to the original data, or (4) validate or expand findings from the original analysis ( Hinds, Vogel, & Clarke-Steffen, 1997 ). Synthesis of knowledge from meta-analysis or aggregation may be viewed as an additional purpose of secondary analysis ( Heaton, 2004 ).
Qualitative studies utilize several different data sources, such as interviews, observations, field notes, archival meeting minutes or clinical record notes, to produce rich descriptions of human experiences within a social context. The work typically requires significant resources (e.g., personnel effort/time) for data collection and analysis. When feasible, qualitative secondary analysis (QSA) can be a useful and cost-effective alternative to designing and conducting redundant primary studies. With advances in computerized data storage and analysis programs, sharing qualitative datasets has become easier. However, little guidance is available for conducting, structuring procedures, or evaluating QSA ( Szabo & Strang, 1997 ).
QSA has been described as “an almost invisible enterprise in social research” ( Fielding, 2004 ). Primary data is often re-used; however, descriptions of this practice are embedded within the methods section of qualitative research reports rather than explicitly identified as QSA. Moreover, searching or classifying reports as QSA is difficult because many researchers refrain from identifying their work as secondary analyses ( Hinds et al., 1997 ; Thorne, 1998a ). In this paper, we provide an overview of QSA, the purposes, and modes of data sharing and approaches. A unique, expanded QSA approach is presented as a methodological exemplar to illustrate considerations.
QSA Typology
Heaton (2004) classified QSA studies based on the relationship between the secondary and primary questions and the scope of data analyzed. Types of QSA included studies that (1) investigated questions different from the primary study, (2) applied a unique theoretical perspective, or (3) extended the primary work. Heaton’s literature review (2004) showed that studies varied in the choice of data used, from selected portions to entire or combined datasets.
Modes of Data Sharing
Heaton (2004) identified three modes of data sharing: formal, informal and auto-data. Formal data sharing involves accessing and analyzing deposited or archived qualitative data by an independent group of researchers. Historical research often uses formal data sharing. Informal data sharing refers to requests for direct access to an investigator’s data for use alone or to pool with other data, usually as a result of informal networking. In some instances, the primary researchers may be invited to collaborate. The most common mode of data sharing is auto-data, defined as further exploration of a qualitative data set by the primary research team. Due to the iterative nature of qualitative research, when using auto-data, it may be difficult to determine where the original study questions end and discrete, distinct analysis begins ( Heaton, 1998 ).
An Exemplar QSA
Below we describe a QSA exemplar conducted by the primary author of this paper (JT), a member of the original research team, who used a supplementary approach to examine concepts revealed but not fully investigated in the primary study. First, we describe an overview of the original study on which the QSA was based. Then, the exemplar QSA is presented to illustrate: (1) the use of auto-data when the new research questions are closely related to or extend the original study aims ( Table 1 ), (2) the collection of additional clinical record data to supplement the original dataset and (3) the performance of separate member checking in the form of expert review and opinion. Considerations and recommendations for use of QSA are reviewed with illustrations taken from the exemplar study ( Table 2 ). Finally, discussion of conclusions and implications is included to assist with planning and implementation of QSA studies.
Research question comparison
Primary study | QSA | |
---|---|---|
What is the process of care and communication in weaning LTMV patients from mechanical ventilation | What are the defining characteristics and cues of psychological symptoms such as anxiety and agitation exhibited by patients who are experiencing prolonged critical illness? | |
What interpersonal interactions (communication contacts, extent and content of communications) contribute to weaning success or are associated with inconsistent/plateau weaning patterns | How do clinicians discriminate between various psychological symptoms and behavioral signs? | |
What therapeutic strategies (e.g., medications/nutrients, use of instruction or comfort measures, rehabilitative treatments) contribute to weaning success or are associated with inconsistent/plateau weaning patterns | What therapeutic strategies (e.g., medications, non-pharmacologic methods) do clinicians undertake in response to patients’ anxiety and agitation? | |
What social (patient, family, clinician characteristics) and environmental factors (noise, lighting, room size/arrangement, work pattern, workload) contribute to weaning success or are associated with inconsistent/plateau weaning patterns | How do physiologic, social and behavioral characteristics of the patient influence the clinician’s interpretation and management of anxiety and agitation? What contextual factors influence interpretation and management of psychological symptoms and behavioral signs? |
Application of the Exemplar Qualitative Secondary Analysis (QSA)
QSA Example | |
---|---|
; ; ; ; ). | |
). |
Aitken, L. M., Marshall, A. P., Elliott, R., & McKinley, S. (2009). Critical care nurses' decision making: sedation assessment and management in intensive care. Journal of Clinical Nursing, 18 (1), 36–45.
Morse, J., & Field, P. (1995). Qualitative research methods for health professionals. (2nd ed.). Thousand Oaks, CA: Sage Publishing.
Patel, R. P., Gambrell, M., Speroff, T.,…Strength, C. (2009). Delirium and sedation in the intensive care unit: Survey of behaviors and attitudes of 1384 healthcare professionals. Critical Care Medicine, 37 (3), 825–832.
Shehabi, Y., Botha, J. A., Boyle, M. S., Ernest, D., Freebairn, R. C., Jenkins, I. R., … Seppelt, I. M. (2008). Sedation and delirium in the intensive care unit: an Australian and New Zealand perspective. Anaesthesia & Intensive Care, 36 (4), 570–578.
Tanios, M. A., de Wit, M., Epstein, S. K., & Devlin, J. W. (2009). Perceived barriers to the use of sedation protocols and daily sedation interruption: a multidisciplinary survey. Journal of Critical Care, 24 (1), 66–73.
Weinert, C. R., & Calvin, A. D. (2007). Epidemiology of sedation and sedation adequacy for mechanically ventilated patients in a medical and surgical intensive care unit. Critical Care Medicine , 35(2), 393–401.
The Primary Study
Briefly, the original study was a micro-level ethnography designed to describe the processes of care and communication with patients weaning from prolonged mechanical ventilation (PMV) in a 28-bed Medical Intensive Care Unit ( Broyles, Colbert, Tate, & Happ, 2008 ; Happ, Swigart, Tate, Arnold, Sereika, & Hoffman, 2007 ; Happ et al, 2007 , 2010 ). Both the primary study and the QSA were approved by the Institutional Review Board at the University of Pittsburgh. Data were collected by two experienced investigators and a PhD student-research project coordinator. Data sources consisted of sustained field observations, interviews with patients, family members and clinicians, and clinical record review, including all narrative clinical documentation recorded by direct caregivers.
During iterative data collection and analysis in the original study, it became apparent that anxiety and agitation had an effect on the duration of ventilator weaning episodes, an observation that helped to formulate the questions for the QSA ( Tate, Dabbs, Hoffman, Milbrandt & Happ, 2012 ). Thus, the secondary topic was closely aligned as an important facet of the primary phenomenon. The close, natural relationship between the primary and QSA research questions is demonstrated in the side-by-side comparison in Table 1 . This QSA focused on new questions which extended the original study to recognition and management of anxiety or agitation, behaviors that often accompany mechanical ventilation and weaning but occur throughout the trajectory of critical illness and recovery.
Considerations when Undertaking QSA ( Table 2 )
Practical advantages.
A key practical advantage of QSA is maximizing use of existing data. Data collection efforts represent a significant percentage of the research budget in terms of cost and labor ( Coyer & Gallo, 2005 ). This is particularly important in view of the competition for research funding. Planning and implementing a qualitative study involves considerable time and expertise not only for data collecting (e.g., interviews, participant observation or focus group), but in establishing access, credibility and relationships ( Thorne, 1994 ) and in conducting the analysis. The cost of QSA is often seen as negligible since the outlay of resources for data collection is assumed by the original study. However, QSA incurs costs related to storage, researcher’s effort for review of existing data, analysis, and any further data collection that may be necessary.
Another advantage of QSA is access to data from an assembled cohort. In conducting original primary research, practical concerns arise when participants are difficult to locate or reluctant to divulge sensitive details to a researcher. In the case of vulnerable critically ill patients, participation in research may seem an unnecessary burden to family members who may be unwilling to provide proxy consent ( Fielding, 2004 ). QSA permits new questions to be asked of data collected previously from these vulnerable groups ( Rew, Koniak-Griffin, Lewis, Miles, & O'Sullivan, 2000 ), or from groups or events that occur with scarcity ( Thorne, 1994 ). Participants’ time and effort in the primary study therefore becomes more worthwhile. In fact, it is recommended that data already collected from existing studies of vulnerable populations or about sensitive topics be analyzed prior to engaging new participants. In this way, QSA becomes a cumulative rather than a repetitive process ( Fielding, 2004 ).
Data Adequacy and Congruency
Secondary researchers must determine that the primary data set meets the needs of the QSA. Data may be insufficient to answer a new question or the focus of the QSA may be so different as to render the pursuit of a QSA impossible ( Heaton, 1998 ). The underlying assumptions, sampling plan, research questions, and conceptual framework selected to answer the original study question may not fit the question posed during QSA ( Coyer & Gallo, 2005 ). The researchers of the primary study may have selectively sampled participants and analyzed the resulting data in a manner that produced a narrow or uneven scope of data ( Hinds et al., 1997 ). Thus, the data needed to fully answer questions posed by the QSA may be inadequately addressed in the primary study. A critical review of the existing dataset is an important first step in determining whether the primary data fits the secondary questions ( Hinds et al., 1997 ).
Passage of Time
The timing of the QSA is another important consideration. If the primary study and secondary study are performed sequentially, findings of the original study may influence the secondary study. On the other hand, studies performed concurrently offer the benefit of access to both the primary research team and participants member checking ( Hinds et al., 1997 ).
The passage of time since the primary study was conducted can also have a distinct effect on the usefulness of the primary dataset. Data may be outdated or contain a historical bias ( Coyer & Gallo, 2005 ). Since context changes over time, characteristics of the phenomena of interest may have changed. Analysis of older datasets may not illuminate the phenomena as they exist today.( Hinds et al., 1997 ) Even if participants could be re-contacted, their perspectives, memories and experiences change. The passage of time also has an affect on the relationship of the primary researchers to the data – so auto-data may be interpreted differently by the same researcher with the passage of time. Data are bound by time and history, therefore, may be a threat to internal validity unless a new investigator is able to account for these effects when interpreting data ( Rew et al., 2000 ).
Researcher stance/Context involvement
Issues related to context are a major source of criticism of QSA ( Gladstone, Volpe, & Boydell, 2007 ). One of the hallmarks of qualitative research is the relationship of the researcher to the participants. It can be argued that removing active contact with participants violates this premise. Tacit understandings developed in the field may be difficult or impossible to reconstruct ( Thorne, 1994 ). Qualitative fieldworkers often react and redirect the data collection based on a growing knowledge of the setting. The setting may change as a result of external or internal factors. Interpretation of researchers as participants in a unique time and social context may be impossible to re-construct even if the secondary researchers were members of the primary team ( Mauthner, Parry, & Milburn, 1998 ). Because the context in which the data were originally produced cannot be recovered, the ability of the researcher to react to the lived experience may be curtailed in QSA ( Gladstone et al., 2007 ). Researchers utilize a number of tactics to filter and prioritize what to include as data that may not be apparent in either the written or spoken records of those events ( Thorne, 1994 ). Reflexivity between the researcher, participants and setting is impossible to recreate when examining pre-existing data.
Relationship of QSA Researcher to Primary Study
The relationship of the QSA researcher to the primary study is an important consideration. When the QSA researcher is not part of the original study team, contractual arrangements detailing access to data, its format, access to the original team, and authorship are required ( Hinds et al., 1997 ). The QSA researcher should assess the condition of the data, documents including transcripts, memos and notes, and clarity and flow of interactions ( Hinds et al., 1997 ). An outline of the original study and data collection procedures should be critically reviewed ( Heaton, 1998 ). If the secondary researcher was not a member of the original study team, access to the original investigative team for the purpose of ongoing clarification is essential ( Hinds et al., 1997 ).
Membership on the original study team may, however, offer the secondary researcher little advantage depending on their role in the primary study. Some research team members may have had responsibility for only one type of data collection or data source. There may be differences in involvement with analysis of the primary data.
Informed Consent of Participants
Thorne (1998) questioned whether data collected for one study purpose can ethically be re-examined to answer another question without participants’ consent. Many institutional review boards permit consent forms to include language about the possibility of future use of existing data. While this mechanism is becoming routine and welcomed by researchers, concerns have been raised that a generic consent cannot possibly address all future secondary questions and may violate the principle of full informed consent ( Gladstone et al., 2007 ). Local variations in study approval practices by institutional review boards may influence the ability of researchers to conduct a QSA.
Rigor of QSA
The primary standards for evaluating rigor of qualitative studies are trustworthiness (logical relationship between the data and the analytic claims), fit (the context within which the findings are applicable), transferability (the overall generalizability of the claims) and auditabilty (the transparency of the procedural steps and the analytic moves processes) ( Lincoln & Guba, 1991 ). Thorne suggests that standard procedures for assuring rigor can be modified for QSA ( Thorne, 1994 ). For instance, the original researchers may be viewed as sources of confirmation while new informants, other related datasets and validation by clinical experts are sources of triangulation that may overcome the lack of access to primary subjects ( Heaton, 2004 ; Thorne, 1994 ).
Our observations, derived from the experience of posing a new question of existing qualitative data serves as a template for researchers considering QSA. Considerations regarding quality, availability and appropriateness of existing data are of primary importance. A realistic plan for collecting additional data to answer questions posed in QSA should consider burden and resources for data collection, analysis, storage and maintenance. Researchers should consider context as a potential limitation to new analyses. Finally, the cost of QSA should be fully evaluated prior to making a decision to pursue QSA.
Acknowledgments
This work was funded by the National Institute of Nursing Research (RO1-NR07973, M Happ PI) and a Clinical Practice Grant from the American Association of Critical Care Nurses (JA Tate, PI).
Publisher's Disclaimer: This is a PDF file of an unedited manuscript that has been accepted for publication. As a service to our customers we are providing this early version of the manuscript. The manuscript will undergo copyediting, typesetting, and review of the resulting proof before it is published in its final citable form. Please note that during the production process errors may be discovered which could affect the content, and all legal disclaimers that apply to the journal pertain.
Disclosure statement: Drs. Tate and Happ have no potential conflicts of interest to disclose that relate to the content of this manuscript and do not anticipate conflicts in the foreseeable future.
Contributor Information
Judith Ann Tate, The Ohio State University, College of Nursing.
Mary Beth Happ, The Ohio State University, College of Nursing.
- Broyles L, Colbert A, Tate J, Happ MB. Clinicians’ evaluation and management of mental health, substance abuse, and chronic pain conditions in the intensive care unit. Critical Care Medicine. 2008; 36 (1):87–93. [ PubMed ] [ Google Scholar ]
- Coyer SM, Gallo AM. Secondary analysis of data. Journal of Pediatric Health Care. 2005; 19 (1):60–63. [ PubMed ] [ Google Scholar ]
- Fielding N. Getting the most from archived qualitative data: Epistemological, practical and professional obstacles. International Journal of Social Research Methodology. 2004; 7 (1):97–104. [ Google Scholar ]
- Gladstone BM, Volpe T, Boydell KM. Issues encountered in a qualitative secondary analysis of help-seeking in the prodrome to psychosis. Journal of Behavioral Health Services & Research. 2007; 34 (4):431–442. [ PubMed ] [ Google Scholar ]
- Happ MB, Swigart VA, Tate JA, Arnold RM, Sereika SM, Hoffman LA. Family presence and surveillance during weaning from prolonged mechanical ventilation. Heart & Lung: The Journal of Acute and Critical Care. 2007; 36 (1):47–57. [ PMC free article ] [ PubMed ] [ Google Scholar ]
- Happ MB, Swigart VA, Tate JA, Hoffman LA, Arnold RM. Patient involvement in health-related decisions during prolonged critical illness. Research in Nursing & Health. 2007; 30 (4):361–72. [ PubMed ] [ Google Scholar ]
- Happ MB, Tate JA, Swigart V, DiVirgilio-Thomas D, Hoffman LA. Wash and wean: Bathing patients undergoing weaning trials during prolonged mechanical ventilation. Heart & Lung: The Journal of Acute and Critical Care. 2010; 39 (6 Suppl):S47–56. [ PMC free article ] [ PubMed ] [ Google Scholar ]
- Heaton J. Secondary analysis of qualitative data. Social Research Update. 1998;(22) [ Google Scholar ]
- Heaton J. Reworking Qualitative Data. London: SAGE Publications; 2004. [ Google Scholar ]
- Hinds PS, Vogel RJ, Clarke-Steffen L. The possibilities and pitfalls of doing a secondary analysis of a qualitative data set. Qualitative Health Research. 1997; 7 (3):408–424. [ Google Scholar ]
- Lincoln YS, Guba EG. Naturalistic inquiry. Beverly Hills, CA: Sage Publishing; 1991. [ Google Scholar ]
- Mauthner N, Parry O, Milburn K. The data are out there, or are they? Implications for archiving and revisiting qualitative data. Sociology. 1998; 32 :733–745. [ Google Scholar ]
- Rew L, Koniak-Griffin D, Lewis MA, Miles M, O'Sullivan A. Secondary data analysis: new perspective for adolescent research. Nursing Outlook. 2000; 48 (5):223–229. [ PubMed ] [ Google Scholar ]
- Szabo V, Strang VR. Secondary analysis of qualitative data. Advances in Nursing Science. 1997; 20 (2):66–74. [ PubMed ] [ Google Scholar ]
- Tate JA, Dabbs AD, Hoffman LA, Milbrandt E, Happ MB. Anxiety and agitation in mechanically ventilated patients. Qualitative health research. 2012; 22 (2):157–173. [ PMC free article ] [ PubMed ] [ Google Scholar ]
- Thorne S. Secondary analysis in qualitative research: Issues and implications. In: Morse JM, editor. Critical Issues in Qualitative Research. Second. Thousand Oaks, CA: SAGE; 1994. [ Google Scholar ]
- Thorne S. Ethical and representational issues in qualitative secondary analysis. Qualitative Health Research. 1998; 8 (4):547–555. [ PubMed ] [ Google Scholar ]
A Case Study Exploring Data Synthesis Strategies on Tabular vs. Aggregated Data Sources for Official Statistics
- Conference paper
- First Online: 13 September 2024
- Cite this conference paper
- Mohamed Aghaddar 9 ,
- Liu Nuo Su 9 ,
- Manel Slokom 9 , 10 ,
- Lucas Barnhoorn 9 &
- Peter-Paul de Wolf 9
Part of the book series: Lecture Notes in Computer Science ((LNCS,volume 14915))
Included in the following conference series:
- International Conference on Privacy in Statistical Databases
29 Accesses
In this paper, we investigate different approaches for generating synthetic microdata from open-source aggregated data. Specifically, we focus on macro-to-micro data synthesis. We explore the potential of the Gaussian copulas framework to estimate joint distributions from aggregated data. Our generated synthetic data is intended for educational and software testing use cases. We propose three scenarios to achieve realistic and high-quality synthetic microdata: (1) zero knowledge, (2) internal knowledge, and (3) external knowledge. The three scenarios involve different knowledge of the underlying properties of the real microdata, i.e., standard deviation, and covariate. Our evaluation includes matching tests to evaluate the privacy of the synthetic datasets. Our results indicate that macro-to-micro synthesis achieves better privacy preservation compared to other methods, demonstrating both the potential and challenges of synthetic data generation in maintaining data privacy while providing useful data for analysis.
The views expressed in this paper are those of the authors and do not necessarily reflect the policy of Statistics Netherlands.
This is a preview of subscription content, log in via an institution to check access.
Access this chapter
Subscribe and save.
- Get 10 units per month
- Download Article/Chapter or eBook
- 1 Unit = 1 Article or 1 Chapter
- Cancel anytime
- Available as PDF
- Read on any device
- Instant download
- Own it forever
- Available as EPUB and PDF
- Compact, lightweight edition
- Dispatched in 3 to 5 business days
- Free shipping worldwide - see info
Tax calculation will be finalised at checkout
Purchases are for personal use only
Institutional subscriptions
https://opendata.cbs.nl/statline/#/CBS/nl/ .
https://synthpop.org.uk/ .
https://sdv.dev/ .
The Nomenclature of Economic Activities (for short NACE).
https://docs.sdv.dev/sdmetrics/metrics/metrics-glossary/newrowsynthesis .
Acharya, A., Sikdar, S., Das, S., Rangwala, H.: GenSyn: a multi-stage framework for generating synthetic microdata using macro data sources. In: IEEE International Conference on Big Data (Big Data), pp. 685–692 (2022)
Google Scholar
Choi, E., Biswal, S., Malin, B., Duke, J., Stewart, W.F., Sun, J.: Generating multi-label discrete patient records using Generative Adversarial Networks. In: Doshi-Velez, F., Fackler, J., Kale, D., Ranganath, R., Wallace, B., Wiens, J. (eds.) Proceedings of the 2nd Machine Learning for Healthcare Conference, vol. 68, pp. 286–305 (2017)
Choupani, A.A., Mamdoohi, A.R.: Population synthesis using iterative proportional fitting (IPF): a review and future research. Transp. Res. Procedia 17 , 223–233 (2016)
Article Google Scholar
Dandekar, R.A., Cohen, M., Kirkendall, N.: Sensitive micro data protection using Latin hypercube sampling technique. In: Domingo-Ferrer, J. (ed.) Inference Control in Statistical Databases. LNCS, vol. 2316, pp. 117–125. Springer, Heidelberg (2002). https://doi.org/10.1007/3-540-47804-3_9
Chapter Google Scholar
Domingo-Ferrer, J.: A survey of inference control methods for privacy-preserving data mining. In: Aggarwal, C.C., Yu, P.S. (eds.) Privacy-Preserving Data Mining: Models and Algorithms, vol. 34, pp. 53–80. Springer, US (2008). https://doi.org/10.1007/978-0-387-70992-5_3
Domingo-Ferrer, J., Torra, V.: Disclosure risk assessment in statistical data protection. J. Comput. Appl. Math. 164–165 , 285–293 (2004). Proceedings of the 10th International Congress on Computational and Applied Mathematics
Article MathSciNet Google Scholar
Drechsler, J., Reiter, J.P.: An empirical evaluation of easily implemented, nonparametric methods for generating synthetic datasets. Comput. Stat. Data Anal. 55 (12), 3232–3243 (2011)
Garofalo, G., Slokom, M., Preuveneers, D., Joosen, W., Larson, M.: Machine learning meets data modification. In: Batina, L., Bäck, T., Buhan, I., Picek, S. (eds.) Security and Artificial Intelligence. LNCS, vol. 13049, pp. 130–155. Springer, Cham (2022). https://doi.org/10.1007/978-3-030-98795-4_7
Hundepool, A., et al.: Statistical Disclosure Control. Wiley, NewYork (2012)
Book Google Scholar
Kim, J., Lee, S.: A simulated annealing algorithm for the creation of synthetic population in activity-based travel demand model. KSCE J. Civ. Eng. 20 , 2513–2523 (2015)
Li, Z., Zhao, Y., Fu, J.: Sync: a copula based framework for generating synthetic data from aggregated sources (2020)
Liew, C.K., Choi, U.J., Liew, C.J.: A data distortion by probability distribution. ACM Trans. Database Syst. 10 (3), 395–411 (1985)
Muralidhar, K.: A re-examination of the Census Bureau reconstruction and reidentification attack. In: Domingo-Ferrer, J., Laurent, M. (eds.) PSD 2022. LNCS, vol. 13463, pp. 312–323. Springer, Cham (2022). https://doi.org/10.1007/978-3-031-13945-1_22
Muralidhar, K., Domingo-Ferrer, J.: Database reconstruction is not so easy and is different from reidentification. J. Off. Stat. 39 (3), 381–398 (2023)
Murata, T., Harada, T.: Nation-wide synthetic reconstruction method. In: 2017 IEEE Symposium Series on Computational Intelligence (SSCI), pp. 1–6 (2017)
Park, N., Mohammadi, M., Gorde, K., Jajodia, S., Park, H., Kim, Y.: Data synthesis based on Generative Adversarial Networks. In: Proceedings of the 44th International Conference on Very Large Data Bases (VLDB Endowment), vol. 11, no. 10, pp. 1071–1083 (2018)
Rubin, D.B.: Discussion statistical disclosure limitation. J. Off. Stat. 9 (2), 461–468 (1993)
Thogarchety, P., Das, K.: Synthetic data generation using genetic algorithm. In: 2023 2nd International Conference for Innovation in Technology (INOCON), pp. 1–6 (2023)
Torra, V.: Privacy in data mining. In: Maimon, O., Rokach, L. (eds.) Data Mining and Knowledge Discovery Handbook, pp. 687–716. Springer, Boston (2009). https://doi.org/10.1007/978-0-387-09823-4_35
Voas, D., Williamson, P.: An evaluation of the combinatorial optimisation approach to the creation of synthetic microdata. Int. J. Popul. Geogr. 6 , 349–366 (2000)
Xu, L., Skoularidou, M., Cuesta-Infante, A., Veeramachaneni, K.: Modeling tabular data using conditional GAN. In: Wallach, H., Larochelle, H., Beygelzimer, A., d’Alche Buc, F., Fox, E., Garnett, R. (eds.) Advances in Neural Information Processing Systems 32, pp. 7335–7345 (2019)
Download references
Acknowledgments
We are grateful to Guus van de Burgt for providing the data and invaluable insights, and to Arjen de Boer for his effective project management and extensive knowledge, both of which were crucial to the success of this project.
This work was partly supported by the AI, Media, and Democracy Lab, NWA.1332.20.009.
Author information
Authors and affiliations.
Statistics Netherlands, The Hague, The Netherlands
Mohamed Aghaddar, Liu Nuo Su, Manel Slokom, Lucas Barnhoorn & Peter-Paul de Wolf
Centrum Wiskunde & Informatica, Amsterdam, The Netherlands
Manel Slokom
You can also search for this author in PubMed Google Scholar
Corresponding authors
Correspondence to Mohamed Aghaddar , Liu Nuo Su , Manel Slokom , Lucas Barnhoorn or Peter-Paul de Wolf .
Editor information
Editors and affiliations.
Rovira i Virgili University, Tarragona, Tarragona, Spain
Josep Domingo-Ferrer
EURECOM, Biot, France
1.1 A.1 Dataset Rules
We explain the types of relationships present in the dataset and the rules the synthesizer must account for. We divide the rules into five categories: non-negative rules, summation rules, equality rules, inequality rules, and if-then rules. The non-negative rule imposes that the variable must be greater or equal to zero (e.g. number of workers and various expenses). For the summation rule, the sum of certain variables must equal another variable per definition. Think of the total expense variable being the sum of individual expenses, or the net revenue being a summation of sales and costs. The equality rule suggests that specific variables must be equal to another variable ( \(=\) ), while the inequality rule imposes that some variables must be lesser or equal ( \(\le \) ) or greater or equal ( \(\ge \) ) than other variables. This is the case of repeated variables in the survey (equality rules), and the variable of the number of workers being greater or equal to the full-time equivalent workers (inequality rule). The if-then rules impose the same rules as the previous ones, with the addition of requiring a certain condition to be satisfied (as some rules can only exist if a variable meets a certain value).
1.2 A.2 Extra Analytical Validity Results
For micro-to-micro data synthesis, we measure the KS-Complement metric, which assesses the similarity between a real variable and its synthetic counterpart based on their marginal distributions. Our results are provided in Tables 3 and 4 (Fig. 5 ).
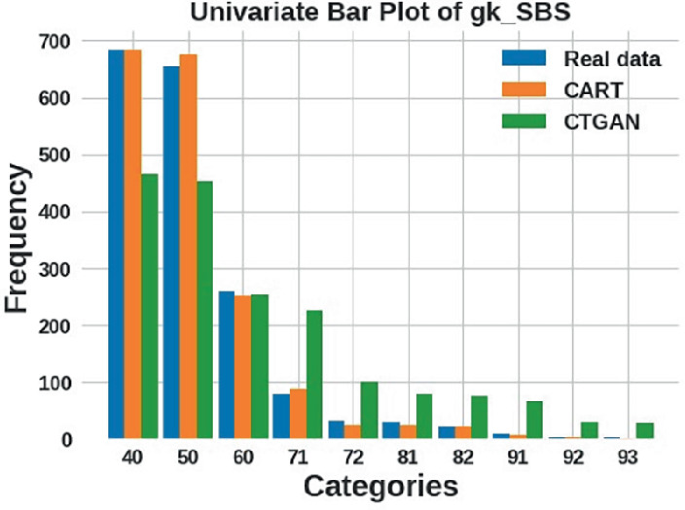
Univariate distribution of the gk_SBS variable
1.3 A.3 Residuals from the Regression Models
We analyze the performance of the synthetic data by examining the residuals of the linear regression model. Figure 6 shows the residual plots for the models trained on the real data, CART synthetic data, and CTGAN synthetic data. The residuals represent the differences between the observed and predicted values.
In the residual plot for the model trained on the real data (Fig. 6 a), the residuals are evenly distributed around zero, indicating a good fit of the model. In contrast, the residual plot for the CART synthetic data (Fig. 6 b) shows a similar distribution, though with a slightly larger variance, suggesting that the CART synthetic data maintains the relationships in the real data fairly well. The residual plot for the CTGAN synthetic data (Fig. 6 c) reveals a different pattern. The residuals are more spread out and exhibit a clear upward trend, indicating that the CTGAN model has a downward bias and struggles to capture the variability in the real data. This pattern suggests that the CTGAN synthetic data may not be as effective in preserving the underlying relationships present in the real data. Overall, these residual plots highlight that the CART synthetic data provides a better approximation of the real data compared to the CTGAN synthetic data, aligning with the findings from the prediction accuracy analysis.
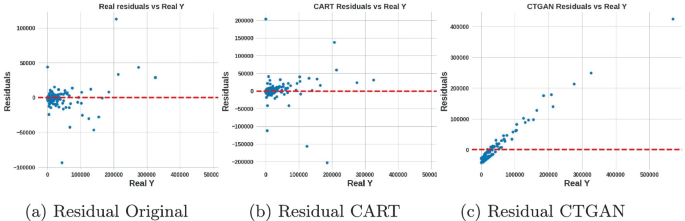
Residual plot, with the prediction model trained on the real and micro-to-micro synthetic datasets
The residual plots for the synthetic data generated using the macro-to-micro approaches are shown in Fig. 7 . In Scenario 1 (Fig. 7 a), where no knowledge of the real data was used, the residuals are widely scattered and show a large deviation from zero. This indicates poor accuracy in the synthetic data, as the model fails to capture the true relationships present in the real data. Scenario 2 (Fig. 7 b), which incorporates internal knowledge, shows an improvement in the residuals compared to Scenario 1. The residuals are closer to zero, suggesting that the synthetic data better approximates the real data, although there are still noticeable deviations. Scenario 3 (Fig. 7 c), which leverages external knowledge, exhibits the most favorable residuals among the three scenarios. The residuals are tightly clustered around zero, indicating a higher accuracy in the synthetic data. This demonstrates that utilizing external insights significantly enhances the quality of the synthetic data generation process.
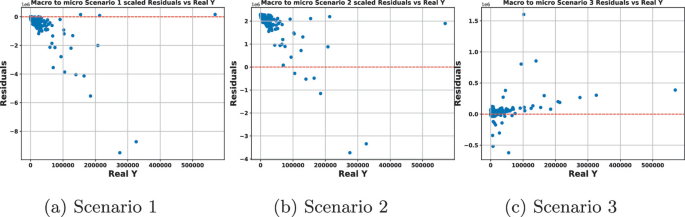
Residual plot on the synthetic data generated using macro-to-micro scenarios with scaled data.
Rights and permissions
Reprints and permissions
Copyright information
© 2024 The Author(s), under exclusive license to Springer Nature Switzerland AG
About this paper
Cite this paper.
Aghaddar, M., Su, L.N., Slokom, M., Barnhoorn, L., de Wolf, PP. (2024). A Case Study Exploring Data Synthesis Strategies on Tabular vs. Aggregated Data Sources for Official Statistics. In: Domingo-Ferrer, J., Önen, M. (eds) Privacy in Statistical Databases. PSD 2024. Lecture Notes in Computer Science, vol 14915. Springer, Cham. https://doi.org/10.1007/978-3-031-69651-0_28
Download citation
DOI : https://doi.org/10.1007/978-3-031-69651-0_28
Published : 13 September 2024
Publisher Name : Springer, Cham
Print ISBN : 978-3-031-69650-3
Online ISBN : 978-3-031-69651-0
eBook Packages : Computer Science Computer Science (R0)
Share this paper
Anyone you share the following link with will be able to read this content:
Sorry, a shareable link is not currently available for this article.
Provided by the Springer Nature SharedIt content-sharing initiative
- Publish with us
Policies and ethics
- Find a journal
- Track your research
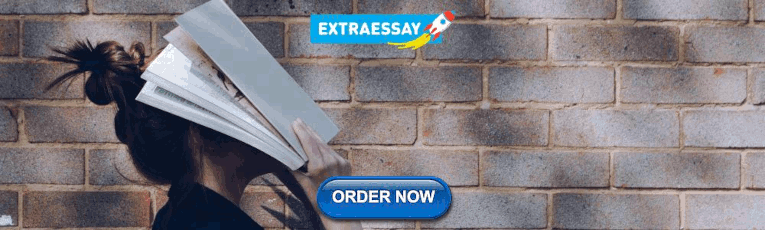
IMAGES
VIDEO
COMMENTS
Revised on January 12, 2024. Secondary research is a research method that uses data that was collected by someone else. In other words, whenever you conduct research using data that already exists, you are conducting secondary research. On the other hand, any type of research that you undertake yourself is called primary research.
Keywords: Case study, secondary data, learning, teaching note, management education, management studies Paper type: Conceptual paper . Published Article. International Management Review, 8(2), 63-70, 2012. ... to design case studies form the available secondary based non-copyrighted and copyrighted information as well. These types of case ...
Secondary data analysis is a valuable research approach that can be used to advance knowledge across many disciplines through the use of quantitative, qualitative, or mixed methods data to answer new research questions (Polit & Beck, 2021).This research method dates to the 1960s and involves the utilization of existing or primary data, originally collected for a variety, diverse, or assorted ...
Revised on November 20, 2023. A case study is a detailed study of a specific subject, such as a person, group, place, event, organization, or phenomenon. Case studies are commonly used in social, educational, clinical, and business research. A case study research design usually involves qualitative methods, but quantitative methods are ...
Introduction. Secondary data analysis is a valuable research approach that can be used to advance knowledge across many disciplines through the use of quantitative, qualitative, or mixed methods data to answer new research questions (Polit & Beck, 2021).This research method dates to the 1960s and involves the utilization of existing or primary data, originally collected for a variety, diverse ...
A multiple case studies approach was adopted that spanned over 2 years, as it is difficult to investigate all the aspects of a phenomenon in a single case study (Cruzes, Dybå, Runeson, & Höst, 2015). The purpose here is to suggest, help, and guide future research students based on what authors have learned while conducting an in-depth case ...
The purpose of case study research is twofold: (1) to provide descriptive information and (2) to suggest theoretical relevance. Rich description enables an in-depth or sharpened understanding of the case. It is unique given one characteristic: case studies draw from more than one data source. Case studies are inherently multimodal or mixed ...
The case studies conducted in this paper were based on secondary data (case study type II) [57], assuming that secondary data sources can provide insights as rich and valuable when compared to ...
Abstract. This study focuses on employing the case study research method approach using only secondary data. This method was used to study an institutional change in the petroleum exploration and production sector in India where the institution, once modified, gradually reverted near to its pre-change state.
Case studies tend to focus on qualitative data using methods such as interviews, observations, and analysis of primary and secondary sources (e.g., newspaper articles, photographs, official records). Sometimes a case study will also collect quantitative data. Example: Mixed methods case study. For a case study of a wind farm development in a ...
A case study is one of the most commonly used methodologies of social research. This article attempts to look into the various dimensions of a case study research strategy, the different epistemological strands which determine the particular case study type and approach adopted in the field, discusses the factors which can enhance the effectiveness of a case study research, and the debate ...
Step 3: Design your research process. After defining your statement of purpose, the next step is to design the research process. For primary data, this involves determining the types of data you want to collect (e.g. quantitative, qualitative, or both) and a methodology for gathering them. For secondary data analysis, however, your research ...
The authors conducted nine case studies, based on primary and secondary data, with each one analyzed separately, so a search for patterns could be undertaken. The convergence aspects found were: the predominant way of transforming experience into knowledge was exploitation; managerial skills were developed through by taking advantages of ...
The full case study of the application of the presented methodology can be found in the forthcoming paper by the same authors under the title of "Evaluation of assumptions of agent-based models of refugee movement based on ethical principles and secondary qualitative analysis."
McMaster University, West Hamilton, Ontario, Canada. Qualitative case study methodology prov ides tools for researchers to study. complex phenomena within their contexts. When the approach is ...
This case study reflects upon the advantages and challenges to using large-scale freely available social science data in the testing of theoretically derived hypotheses. The original study was designed to test the role of three types of strain, derived from General Strain Theory, on substance abuse and dependence by two cohorts of females: a ...
Secondary research, also known as desk research, is a research method that involves compiling existing data sourced from a variety of channels. This includes internal sources (e.g.in-house research) or, more commonly, external sources (such as government statistics, organizational bodies, and the internet).
The process of data analysis in secondary research. Secondary analysis (i.e., the use of existing data) is a systematic methodological approach that has some clear steps that need to be followed for the process to be effective. In simple terms there are three steps: Step One: Development of Research Questions. Step Two: Identification of dataset.
So, rightly secondary research is also termed " desk research ", as data can be retrieved from sitting behind a desk. The following are popularly used secondary research methods and examples: 1. Data Available on The Internet. One of the most popular ways to collect secondary data is the internet.
Types of secondary data are as follows: Published data: Published data refers to data that has been published in books, magazines, newspapers, and other print media. Examples include statistical reports, market research reports, and scholarly articles. Government data: Government data refers to data collected by government agencies and departments.
Heaton (2004) classified QSA studies based on the relationship between the secondary and primary questions and the scope of data analyzed. Types of QSA included studies that (1) investigated questions different from the primary study, (2) applied a unique theoretical perspective, or (3) extended the primary work.
While secondary data analysis of quantitative data has become commonplace and encouraged across disciplines, the practice of secondary data analysis with qualitative data has met more criticism and concerns regarding potential methodological and ethical problems.
Heaton (2004) classified QSA studies based on the relationship between the secondary and primary questions and the scope of data analyzed. Types of QSA included studies that (a) investigated questions different from the primary study, (b) applied a unique theoretical perspective, or (c) extended the primary work.
A Case Study Exploring Data Synthesis Strategies on Tabular vs. Aggregated Data Sources for Official Statistics. ... Fu, J.: Sync: a copula based framework for generating synthetic data from aggregated sources (2020) Google Scholar Liew, C.K., Choi, U.J., Liew, C.J.: A data distortion by probability distribution. ACM Trans . Database ...
The study focused on parameter recording and logging to provide an easy access of the information for analysis and future planning. Table 1 presents the summary of the related studies explored the use of the IoT based sensors in different applications. Most of the existing studies did not focus on detection and classification of faults and were ...