- Email Alert
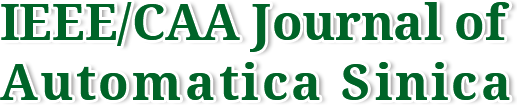
论文 全文 图 表 新闻
- Abstracting/Indexing
- Journal Metrics
- Current Editorial Board
- Early Career Advisory Board
- Previous Editor-in-Chief
- Past Issues
- Current Issue
- Special Issues
- Early Access
- Online Submission
- Information for Authors
- Share facebook twitter google linkedin
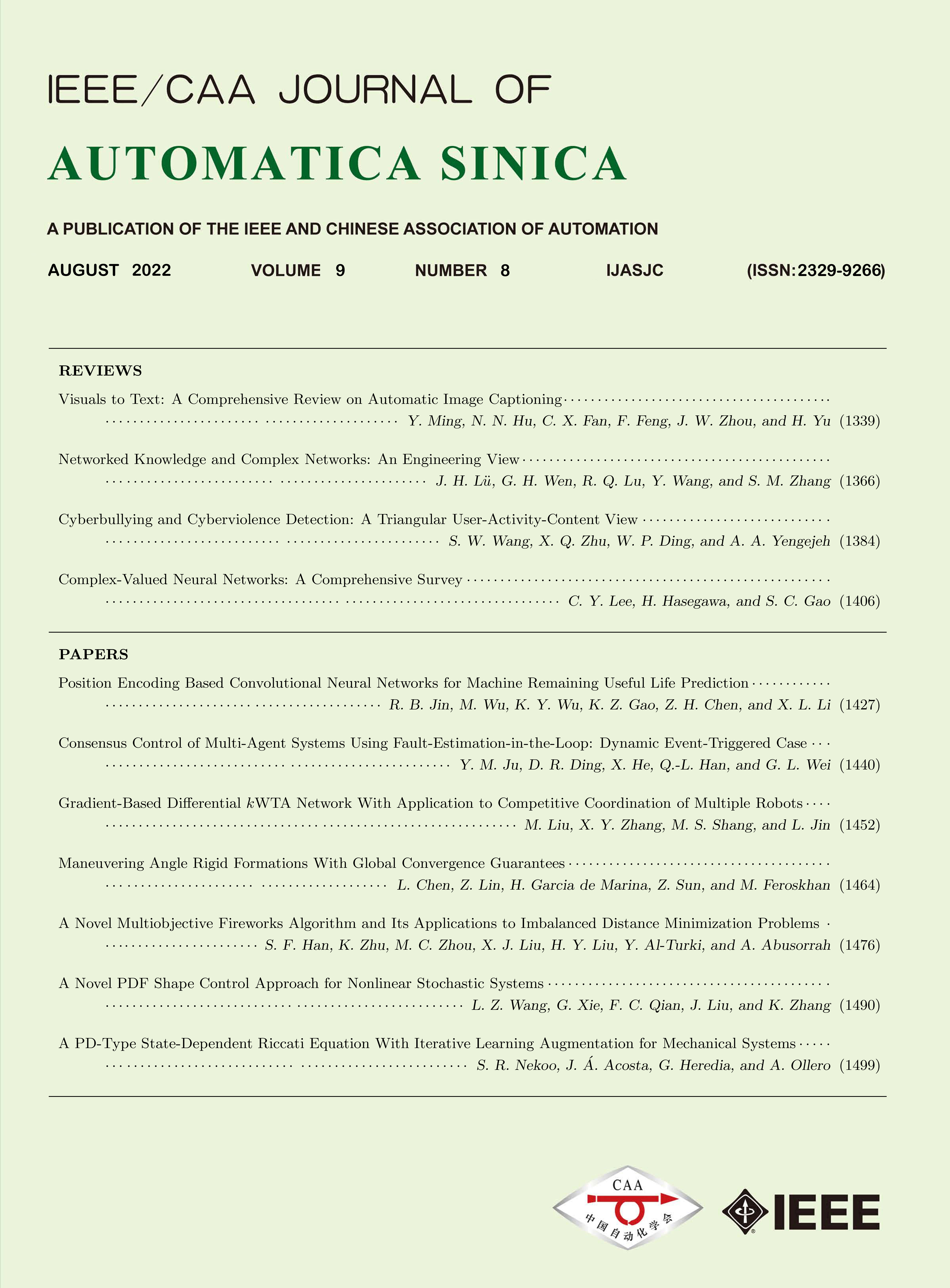
IEEE/CAA Journal of Automatica Sinica
- JCR Impact Factor: 15.3 , Top 1 (SCI Q1) CiteScore: 23.5 , Top 2% (Q1) Google Scholar h5-index: 77, TOP 5
Y. Ming, N. N. Hu, C. X. Fan, F. Feng, J. W. Zhou, and H. Yu, “Visuals to text : A comprehensive review on automatic image captioning,” , vol. 9, no. 8, pp. 1339–1365, Aug. 2022. doi: |
Y. Ming, N. N. Hu, C. X. Fan, F. Feng, J. W. Zhou, and H. Yu, “Visuals to text : A comprehensive review on automatic image captioning,” , vol. 9, no. 8, pp. 1339–1365, Aug. 2022. doi: |
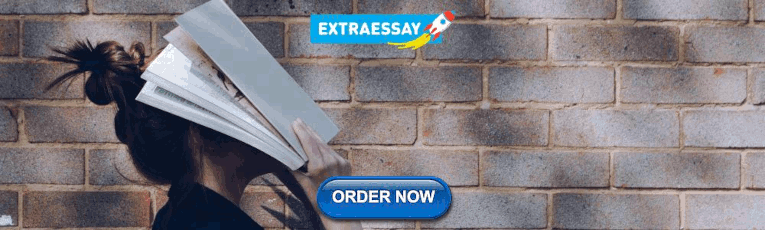
Visuals to Text: A Comprehensive Review on Automatic Image Captioning
Doi: 10.1109/jas.2022.105734.
- Yue Ming 1 , ,
- Nannan Hu 1 , ,
- Chunxiao Fan 1 , ,
- Fan Feng 1 , ,
- Jiangwan Zhou 1 , ,
- Hui Yu 2 , ,
Beijing University of Posts and Telecommunications, Beijing 100876, China
School of Creative Technologies, University of Ports
Yue Ming (Member, IEEE) received the B.Sc. degree in communication engineering in 2006, the M.Sc. degree in human-computer interaction engineering in 2008, and Ph.D. degree in signal and information processing in 2013, all from Beijing Jiaotong University. She was a Visiting Scholar in Carnegie Mellon University, USA from 2010 to 2011. She is an Associate Professor at Beijing University of Posts and Telecommunications. Her research interests include biometrics, computer vision, computer graphics, information retrieval, and pattern recognition
Nannan Hu received the B.Sc. degree in communication engineering in 2016, and the M.Sc. degree in electronic science and technology in 2019, both from Shandong Normal University. She is currently a Ph.D. candidate in electronic science and technology at Beijing University of Posts and Telecommunications. Her research interests include object recognition, NLP and multi-modal information processing
Chunxiao Fan (Member, IEEE) received the B.Sc. degree in computer science in 1984, the M.Sc. degree in decision support systems and artificial intelligence in 1989 from Northeastern University, and the Ph.D. degree in computers and communications from Beijing University of Posts and Telecommunications in 2008. She is a Professor in the School of Electronic Engineering and the Director of the Information Electronics and Intelligent Processing Center, Beijing University of Posts and Telecommunications. Her research interests include intelligent information processing, multimedia analysis and NLP
Fan Feng received the M.Sc. degree in control science and engineering from Donghua University in 2019. He is currently a Ph.D. cadidate in electronic science and technology at Beijing University of Posts and Telecommunications. His research interests include audio-visual learning and multi-modal action recognition
Jiangwan Zhou received the B.Sc. degree in computer science from Heilongjiang University in 2019. She is currently a Ph.D. cadidate in electronic science and technology at Beijing University of Posts and Telecommunications. Her research interests include video understanding, action recognition bath in RGB-domain and compressed-domain
Hui Yu (Senior Member, IEEE) received the Ph.D. degree from Brunel University London, UK. He worked at the University of Glasgow and Queen’s University Belfast before joining the University of Portsmouth in 2012. He is a Professor of visual computing with the University of Portsmouth, UK. His research interests include methods and practical development in visual computing, machine learning and AI with the applications focusing on human-machine interaction, multimedia, virtual reality and robotics as well as 4D facial expression generation, perception and analysis. He serves as an Associate Editor for IEEE Transactions on Human-Machine Systems and Neurocomputing Journal
- Corresponding author: Hui Yu, e-mail: [email protected]
- 1 We use the Flick8k dataset as the query corpus in this demo.
- 2 https://cocodataset.org/ 3 https://competitions.codalab.org/competitions/3221 4 https://forms.illinois.edu/sec/1713398
- 5 http://shannon.cs.illinois.edu/DenotationGraph/ 6 https://www.imageclef.org/photodata 7 http://visualgenome.org/
- https://forms.illinois.edu/sec/1713398
- http://shannon.cs.illinois.edu/DenotationGraph/
- 8 https://github.com/furkanbiten/GoodNews 9 https://challenger.ai/ 10 https://vizwiz.org/ 11 https://nocaps.org/
- http://visualgenome.org/
- https://github.com/furkanbiten/GoodNews
- 11 https://nocaps.org/
- https://vizwiz.org/
- https://nocaps.org/
- 12 https://github.com/tylin/coco-caption
- Revised Date: 2022-03-29
- Accepted Date: 2022-05-26
- Artificial intelligence ,
- attention mechanism ,
- encoder-decoder framework ,
- image captioning ,
- multi-modal understanding ,
- training strategies
[1] | , Springer, 2022, pp. 511−522. |
[2] | , 2017, pp. 528−536. |
[3] | , 2017, vol. 31, no. 1, pp. 4068−4074. |
[4] | , vol. 74, pp. 474–487, 2018. doi: |
[5] | , 2021, pp. 12679−12688. |
[6] | , vol. 128, no. 2, pp. 261–318, 2020. doi: |
[7] | , Springer, 2020, pp. 417−434. |
[8] | , vol. 50, no. 2, pp. 171–184, 2002. doi: |
[9] | , Citeseer, 2004, pp. 306−313. |
[10] | , 2011, pp. 220−228. |
[11] | , vol. 7, no. 1, pp. 214–222, Jan. 2020. |
[12] | , 2020, pp. 13035−13045. |
[13] | , vol. 2, pp. 351–362, 2014. doi: |
[14] | , vol. 47, pp. 853–899, 2013. doi: |
[15] | , vol. 35, no. 12, pp. 2891–2903, 2013. doi: |
[16] | , 2012, pp. 747−756. |
[17] | , 2011, pp. 444−454. |
[18] | , 2012, pp. 392−397. |
[19] | , vol. 51, no. 2, pp. 1085–1093, 2019. doi: |
[20] | , vol. 8, no. 9, pp. 1523–1538, Sept. 2021. |
[21] | , vol. 8, no. 9, pp. 1539–1539, Sept. 2021. |
[22] | , vol. 8, no. 1, pp. 94–109, Jan. 2021. |
[23] | , vol. 8, no. 8, pp. 1428–1439, Aug. 2021. |
[24] | , vol. 438, no. 28, pp. 14–33, 2021. |
[25] | , 2015, pp. 2048−2057. |
[26] | , vol. 39, no. 4, pp. 652–663, 2016. |
[27] | , 2017, pp. 375−383. |
[28] | , 2017, pp. 7008−7024. |
[29] | , 2018, pp. 6077−6086. |
[30] | |
[31] | , 2014, pp. 595−603. |
[32] | , 2015, pp. 3128−3137. |
[33] | , vol. 22, no. 3, pp. 808–818, 2019. |
[34] | , 2021, vol. 35, no. 3, pp. 2047−2055. |
[35] | , 2018, pp. 684−699. |
[36] | , 2019, pp. 765−773. |
[37] | , vol. 44, no. 5, pp. 2313–2327, 2020. doi: |
[38] | , vol. 7, no. 5, pp. 1361–1370, Sept. 2020. |
[39] | , vol. 8, no. 7, pp. 1243–1252, 2020. |
[40] | , vol. 7, no. 6, pp. 1489–1497, Nov. 2020. |
[41] | , 2020, vol. 34, no. 3, pp. 2693−2700. |
[42] | , vol. 30, no. 12, pp. 4467–4480, 2020. doi: |
[43] | |
[44] | , 2021, pp. 5579−5588. |
[45] | , Springer, 2010, pp. 15−29. |
[46] | , vol. 24, pp. 1143–1151, 2011. |
[47] | , vol. 2, pp. 207–218, 2014. doi: |
[48] | , 2014, vol. 2, pp. 592−598. |
[49] | , 2015, pp. 2596−2604. |
[50] | , 2012, vol. 26, no. 1. |
[51] | |
[52] | , 2015, vol. 29, no. 1. |
[53] | , 2015, pp. 2085−2094. |
[54] | , 2013, vol. 27, no. 1. |
[55] | -state markovian jumping particle swarm optimization algorithm,” , , , vol. 51, no. 11, pp. 6626–6638, 2020. doi: |
[56] | , 2015, pp. 2668−2676. |
[57] | , vol. 8, no. 6, pp. 1177–1187, Jun. 2021. |
[58] | , 2016, pp. 203−212. |
[59] | , 2018, pp. 503−519. |
[60] | , 2021, pp. 16549−16559. |
[61] | , 2019, pp. 784−792. |
[62] | , 2015, pp. 2625−2634. |
[63] | , 2021, pp. 15465−15474. |
[64] | , 2016, pp. 11−20. |
[65] | , 2015, pp. 1473−1482. |
[66] | |
[67] | , 2015, pp. 1440−1448. |
[68] | , 2019, pp. 2601−2610. |
[69] | , vol. 132, pp. 132–140, 2020. doi: |
[70] | |
[71] | , vol. 35, no. 4, 2021, pp. 3136−3144. |
[72] | , vol. 8, no. 10, pp. 1697–1708, Oct. 2021. |
[73] | , vol. 2, no. 9, pp. 205–234, 2022. |
[74] | , 2019, pp. 10685−10694. |
[75] | , 2019, pp. 4250−4260. |
[76] | , 2019, pp. 10323−10332. |
[77] | , 2018, pp. 5831−5840. |
[78] | , 2019, pp. 2580−2590. |
[79] | , Springer, 2020, pp. 211−229. |
[80] | , 2020, pp. 9962−9971. |
[81] | , 2021, pp.3394–3402. |
[82] | |
[83] | , 2019, pp. 29−34. |
[84] | , 2020, pp. 504−515. |
[85] | , 2015, pp. 2533−2541. |
[86] | |
[87] | , IEEE, 2016, pp. 4448−4452. |
[88] | , 2018, pp. 6959−6966. |
[89] | , vol. 28, no. 11, pp. 5241–5252, 2019. doi: |
[90] | , 2015, pp. 2407−2415. |
[91] | |
[92] | , 2016, pp. 988−997. |
[93] | , 2019, pp. 8395−8404. |
[94] | , 2019, pp. 7414−7424. |
[95] | , vol. 8, no. 1, pp. 110–120, Jan. 2021. |
[96] | , 2019, pp. 4240−4249. |
[97] | , 2019, pp. 6241−6250. |
[98] | , 2018, vol. 32, no. 1, pp. 6837–6844. |
[99] | , 2019, pp. 6271−6280. |
[100] | , 2021, vol. 35, no. 3, pp. 2584−2592. |
[101] | , vol. 7, no. 4, pp. 965–974, Jul. 2020. |
[102] | , 2017, vol. 31, no. 1, pp. 4133−4239. |
[103] | , vol. 67, pp. 100–107, 2018. doi: |
[104] | , IEEE, 2018, pp. 1709−1717. |
[105] | , vol. 50, no. 1, pp. 103–119, 2019. doi: |
[106] | , vol. 79, no. 3, pp. 2013–2030, 2020. |
[107] | , 2017, pp. 5659−5667. |
[108] | , 2018, pp. 1−5. |
[109] | , vol. 29, pp. 7615–7628, 2020. doi: |
[110] | , 2018, pp. 7219−7228. |
[111] | , vol. 364, pp. 322–329, 2019. doi: |
[112] | , vol. 85, p. 115836, 2020. |
[113] | , vol. 32, no. 1, pp. 43–51, 2021. doi: |
[114] | , , , vol. 14, no. 2, pp. 1–21, 2018. |
[115] | , vol. 98, p. 107075, 2020. |
[116] | , Springer, 2018, pp. 161−171. |
[117] | , vol. 8, no. 7, pp. 1296–1307, Jul. 2021. |
[118] | , vol. 214, p. 106730, 2021. |
[119] | , 2017, pp. 889−892. |
[120] | , Springer, 2018, pp. 21−37. |
[121] | , 2019, vol. 33, no. 1, pp. 8957−8964. |
[122] | , vol. 167, p. 107329, 2020. |
[123] | , 2019, vol. 33, no. 1, pp. 8320−8327. |
[124] | , 2019, pp. 1−8. |
[125] | , vol. 109, p. 104146, 2021. |
[126] | |
[127] | , vol.60, p. 5608816, 2021. |
[128] | , 2017, pp. 5998−6008. |
[129] | , 2020, pp. 10971−10980. |
[130] | , vol. 7, no. 5, pp. 1371–1379, Sept. 2020. |
[131] | , vol. 8, no. 5, p. 739, 2018. |
[132] | , 2019, pp. 11137−11147. |
[133] | , 2020, pp. 10327−10336. |
[134] | , 2019, pp. 8928−8937. |
[135] | , 2020, pp. 10578−10587. |
[136] | , 2021, pp. 1−8. |
[137] | |
[138] | , vol. 425, pp. 173–180, 2021. doi: |
[139] | , 2020, pp. 4226−4234. |
[140] | , vol. 7, no. 2, pp. 617–626, Mar. 2020. |
[141] | |
[142] | , 2015, pp. 4566−4575. |
[143] | , Springer, 2016, pp. 382−398. |
[144] | , 2017, pp. 873−881. |
[145] | , 2017, pp.1–10. |
[146] | , vol. 6, no. 2, pp. 221–231, Jun. 2021. doi: |
[147] | , 2019, vol. 33, no. 1, pp. 8142−8150. |
[148] | , Springer, 2020, pp. 574−590. |
[149] | , 2020, vol. 34, no. 7, pp. 13041−13049. |
[150] | , “Oscar: Object-semantics aligned pre-training for vision-language tasks,” in , Springer, 2020, pp. 121−137. |
[151] | , 2021, vol. 35, no. 2, pp. 1575−1583. |
[152] | , Springer, 2014, pp. 740−755. |
[153] | , vol. 2, pp. 67–78, 2014. doi: |
[154] | , 2015, pp. 2641−2649. |
[155] | , “The 2005 pascal visual object classes challenge,” in , Springer, 2005, pp. 117−176. |
[156] | , vol. 59, no. 2, pp. 64–73, 2016. doi: |
[157] | , 2016, pp. 70−74. |
[158] | |
[159] | , 2006, vol. 2, pp.13–23. |
[160] | , 2019, pp. 12466−12475. |
[161] | , 2019, pp. 8948−8957. |
[162] | , Springer, 2020, pp. 1−17. |
[163] | , Springer, 2020, pp. 742−758. |
[164] | , vol. 123, no. 1, pp. 32–73, 2017. doi: |
[165] | , vol. 2, no. 3, p. 18, 2017. |
[166] | , 2002, pp. 311−318. |
[167] | , 2005, vol. 29, pp. 65−72. |
[168] | , 2004, pp. 605−612. |
[169] | , 2019, pp. 10695−10704. |
[170] | , 2019, pp. 4261−4270. |
[171] | , 2019, pp. 4195−4203. |
[172] | , vol. 44, no. 2, pp. 1035–1049, 2020. doi: |
[173] | , 2020, pp. 2141−2152. |
[174] | , 2021, pp. 14050−14059. |
[175] | , 2019, pp. 1−43. |
[176] | , 2020, pp. 34−39. |
[177] | , Springer, 2020, pp. 370−386. |
[178] | |
[179] | , vol. 2, p. T2, 2021. |
[180] | |
[181] | , 2021, pp. 220−226. |
[182] | |
[183] | , 2014, vol. 2, pp. 1889−1897. |
[184] | , 2015, pp. 2422−2431. |
[185] | , 2015, pp. 3156−3164. |
[186] | , 2016, pp. 49−56. |
[187] | , 2016, pp. 1−10. |
[188] | , 2017, pp. 2193−2202. |
[189] | , 2017, pp. 1222−1231. |
[190] | & , vol. 40, no. 6, pp. 1367–1381, 2018. |
[191] | , 2018, pp. 5561−5570. |
[192] | , 2018, pp. 1−9. |
[193] | , vol. 21, no. 11, pp. 2942–2956, 2019. doi: |
[194] | , 2019, pp. 8367−8375. |
[195] | , 2020, vol. 34, no. 7, pp. 11588−11595. |
[196] | , 2020, pp. 4190−4198. |
[197] | , 2020, pp. 4217−4225. |
[198] | , 2021, vol. 35, no. 2, pp. 1317−1324. |
[199] | , vol. 143, pp. 43–49, 2021. doi: |
[200] | , 2021, vol. 35, no. 3, pp. 2286−2293. |
[201] | , 2020, pp. 7454−7464. |
[202] | , 2019, pp. 4634−4643. |
[203] | , 2018, pp. 1416−1424. |
[204] | , Springer, 2018, pp. 499−515. |
[205] | , 2017, pp. 4894−4902. |
[206] | , 2019, pp. 2621−2629. |
[207] | , 2020, pp. 4777−4786. |
[208] | , 2020, pp. 12176−12183. |
[209] | , 2021, vol. 35, no. 2, pp. 1655−1663. |
[210] | , 2019, pp. 8307−8316. |
[211] | , Springer, 2020, pp. 712−729. |
[212] | , 2020, pp. 4808−4816. |
[213] | , 2019, pp. 8650−8657. |
[214] | , 2021, pp. 8425−8435. |
[215] | , 2019, pp. 12516−12526. |
[216] | , vol. 396, pp. 92–101, 2020. doi: |
[217] | , 2020, pp. 3182−3190. |
[218] |
Proportional views
通讯作者: 陈斌, [email protected]
沈阳化工大学材料科学与工程学院 沈阳 110142
Figures( 18 ) / Tables( 4 )
Article Metrics
- PDF Downloads( 1037 )
- Abstract views( 4227 )
- HTML views( 142 )
- Conducting a comprehensive review of image captioning, covering both traditional methods and recent deep learning-based techniques, as well as the publicly available datasets, evaluation metrics, the open issues and challenges
- Focusing on the deep learning-based image captioning researches, which is categorized into the encoder-decoder framework, attention mechanism and training strategies on the basis of model structures and training manners for a detailed introduction. The performance of current state-of-the-art methods of image captioning is also compared and analyzed
- Discussing several future research directions of image captioning, such as Flexible Captioning, Unpaired Captioning, Paragraph Captioning and Non-Autoregressive Captioning
- Copyright © 2022 IEEE/CAA Journal of Automatica Sinica
- 京ICP备14019135号-24
- E-mail: [email protected] Tel: +86-10-82544459, 10-82544746
- Address: 95 Zhongguancun East Road, Handian District, Beijing 100190, China
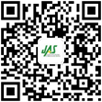
Export File

- Figure 1. The evolution of image captioning. According to the scenario, image captioning can be divided into two stages. Here, we mainly focus on generating caption for generic real life image after 2011. Before 2014, it relies on traditional methods based on retrieval and template. After 2014, various variations based on deep learning technologies have become benchmark approaches, and have got state of the art results.
- Figure 2. Overview of the deep learning-based image captioning and a simple taxonomy of the most relevant approaches. The encoder-decoder framework is the backbone of the deep learning captioning models. Better visual coding, language decoding technologies, the introduction of various innovative attention mechanisms and training strategies all bring a positive impact on captioning insofar.
- Figure 3. The framework of image captioning model based on retrieval. Preparing a corpus with a large number of image-caption pairs. Retrieval models search for visually similar images to the input image in the query corpus through similarity comparison, then select the best annotation from the corresponding candidates as the output caption.
- Figure 4. The framework of image captioning model based on template. The template-based methods are based on a predefined syntax. For a given image, it extracts relevant elements such as objects and actions, predicts their language labels in various ways, then fills them in the predefined template to generate caption.
- Figure 5. The flowchart of deep learning methods for image captioning. The trained model is passed to validation and then to test, parameter optimization and fine-tune of the model improves the results gradually, and it is a parameter sharing process.
- Figure 6. The overall technical diagram of deep learning-based image captioning. Notice that the self-attention mechanism is can also be used for visual encoding and language decoding. We summarize the attention mechanism in detail in Section III-B.
- Figure 7. Comparison of global grid features and visual region features. Global grid features correspond to a uniform grid of equally-sized image regions (left). Region features correspond to objects and other salient image regions (right).
- Figure 8. Two main ways for CNN to encode visual features. For the upper one, the global grid features of image are embedded in a feature vector; for the bottom one, fine-grained features of salient objects or regions are embedded in different region vectors.
- Figure 9. The framework of graph representation Learning. e i is a set of directed edges that represent relationships between objects, and a i , o i are nodes, which represent attributes and objects, respectively.
- Figure 10. The singe-layer LSTM strategy for language modeling. (a) Unidirection single-layer LSTM model; (b) Bidirection single-layer LSTM model, which conditioned on the visual feature.
- Figure 11. The two-layer LSTM strategy for language modeling.
- Figure 12. The triple-stream LSTM strategy for language modeling.
- Figure 13. The common structure of cross-modal attention used for image captioning. (a) The framework of attention mechanism proposed in the Show, Attend and Tell model [ 25 ], which be widely used in cross-modal attention for image captioning; (b) The attention used in single-layer LSTM; (c) The attention used as a visual sentinel in single-layer LSTM; (d) An attention layer used in two-layer LSTM; (e) Two-layer LSTM with dual attention.
- Figure 14. The common structure of intra-modal attention used for image captioning in the first stage, the Transformer model works with the traditional CNN-LSTM framework. (a) is the framework of CNN-Transformer decoder, and (b) is the framework of Transformer encoder-LSTM.
- Figure 15. The framework of intra-modal attention used with object detected features.
- Figure 16. The overall of convolution-free intra-modal attention model.
- Figure 17. Qualitative examples from some typical image captioning datasets. MS COCO and Flickr8k/30k are the most popular benchmarks in image captioning. The images used in the MS COCO, Flickr8k and Flickr30k datasets are all collected from Yahoo’s photo album site Flickr. AIC is used for Chinese captioning, which focuses on the scenes of human activities. The images used in the AIC dataset are collected from Internet search engines. Nocaps is used for novel object captioning.
- Figure 18. Qualitative examples from five popular captioning models on MS COCO val images. All models are retrained by us with ResNet-101 features. Errors are highlighted in red, and new descriptions of objects, attributes, relations are highlighted in green, blue and orange severally.
- Open access
- Published: 08 February 2023
Image caption generation using Visual Attention Prediction and Contextual Spatial Relation Extraction
- Reshmi Sasibhooshan 1 ,
- Suresh Kumaraswamy 2 &
- Santhoshkumar Sasidharan 3
Journal of Big Data volume 10 , Article number: 18 ( 2023 ) Cite this article
5471 Accesses
17 Citations
Metrics details
Automatic caption generation with attention mechanisms aims at generating more descriptive captions containing coarser to finer semantic contents in the image. In this work, we use an encoder-decoder framework employing Wavelet transform based Convolutional Neural Network (WCNN) with two level discrete wavelet decomposition for extracting the visual feature maps highlighting the spatial, spectral and semantic details from the image. The Visual Attention Prediction Network (VAPN) computes both channel and spatial attention for obtaining visually attentive features. In addition to these, local features are also taken into account by considering the contextual spatial relationship between the different objects. The probability of the appropriate word prediction is achieved by combining the aforementioned architecture with Long Short Term Memory (LSTM) decoder network. Experiments are conducted on three benchmark datasets—Flickr8K, Flickr30K and MSCOCO datasets and the evaluation results prove the improved performance of the proposed model with CIDEr score of 124.2.
Introduction
Images are extensively used for conveying enormous amount of information over internet and social media and hence there is an increasing demand for image data analytics for designing efficient information processing systems. This leads to the development of systems with capability to automatically analyze the scenario contained in the image and to express it in meaningful natural language sentences. Image caption generation is an integral part of many useful systems and applications such as visual question answering machines, surveillance video analyzers, video captioning, automatic image retrieval, assistance for visually impaired people, biomedical imaging, robotics and so on. A good captioning system will be capable of highlighting the contextual information in the image similar to human cognitive system. In the recent years, several techniques for automatic caption generation in images have been proposed that can effectively solve many computer vision challenges.
Basically image captioning is a two step process, which involves a thorough understanding of the visual contents in the image followed by the translation of these information to natural language descriptions. Visual information extraction includes the detection and recognition of objects and also the identification of their relationships. Initially, image captioning is performed using rule based or retrieval based approaches [ 1 , 2 ]. Later advanced image captioning systems are designed using deep neural architectures that uses a Convolutional Neural Network (CNN) as encoder for visual feature extraction and a Recurrent Neural Network (RNN) as decoder for text generation [ 3 ]. Algorithms using Long Short Term Memory (LSTM) [ 4 , 5 ] and Gated Recurrent Unit (GRU) [ 6 ] are introduced to obtain meaningful captions. Inclusion of attention mechanism in the model helps to extract the most relevant objects or regions in the image [ 7 , 8 ], which can be used for the generation of rich textual descriptions. These networks localize the salient regions in the images and produce improved image captions than previous works. However, they failed to extract accurate contextual information from images, defining the relationship between various objects and between each object and the background, and to project the underlying global and local semantics.
In this work, an image captioning method is proposed that uses discrete wavelet decomposition along with convolutional neural network (WCNN) for extracting the spectral information in addition to the spatial and semantic features of the image. An attempt is made to enhance the visual modelling of the input image by the incorporation of DWT pre-processing stage together with convolutional neural networks that helps to extract some of the distinctive spectral features, which are more predominant in the sub band levels of the image in addition to the spatial, semantic as well as channel details. This helps to include more finer details of each object, for example, the spatial orientation of the objects, colour details etc. In addition to these, it helps to detect the visually salient object/region in the image that draws more attraction similar to human visual system due to its peculiar features with respect to the remaining regions. A Visual Attention Prediction network (VAPN) and Contextual Spatial Relation Extractor (CSE) are employed to extract the fine-grained semantic and contextual spatial relationship existing between the objects, gathered from the feature maps obtained using WCNN. Finally, these details are fed to LSTM network for generating the most relevant captions. The word prediction capability of the LSTM decoder network can be improved through the concatenation of attention based image feature maps and contextual spatial feature maps with the previous hidden state of the language generation model. This enhances the sentence formation greatly, as each word in the generated sentence focuses only on particular spatial locations in the image and its contextual relation with other objects in the image.
The contributions made in this work are:
An image caption generation technique incorporating semantic, spatial, spectral and contextual information contained in the image is proposed.
A Visual Attention Prediction network is employed to perform atrous convolution on the feature maps generated by WCNN for extracting more semantic contents from the image. Both channel as well as spatial attention are computed from these feature maps.
A Contextual Spatial Relation Extractor that utilizes the feature maps generated by WCNN model for predicting region proposals to detect spatial relationship between different objects in the image.
The performance of the method is evaluated using three benchmark datasets—Flickr8K, Flickr30K and Microsoft COCO datasets and comparison is done with the existing state-of-the-art methods using the evaluation metrics—BLEU@N, METEOR, ROUGE-L and CIDEr.
The organization of the paper is as follows: First a brief descriptions about the previous works in image captioning, which is followed by the proposed model architecture and detailed experiments and results. Finally the conclusion of the work is also provided.
Related works
In order to generate an appropriate textual description, it is necessary to have a better understanding about the spatial and semantic contents of the image. As mentioned above, the initial attempts of image caption generation are carried out by extracting the visual features of the image using conditional random fields (CRFs) [ 9 , 10 ] and translating these features to text using sentence template or combinatorial optimization algorithms [ 1 , 11 , 12 ]. Later retrieval based approaches are used to produce captions that involves the process of retrieving one or a set of sentences from a pre-specified sentence pool based on visual similarities [ 2 , 13 ]. The evolution of deep neural network architectures helps to have visual and natural language modelling in a superior manner by generating more meaningful descriptions of the image.
The inclusion of additional attention mechanisms in the encoder—decoder framework helps to extract more appropriate semantic information from the image and thereby creating captions that look similar to human generated captions [ 14 , 15 , 16 ]. An encoder–decoder model capable of detecting dynamically attentive salient regions in the image during the creation of textual descriptions is proposed by Xu et al. [ 17 ]. Yang et al. [ 18 ] proposed a review network with enhanced global modelling capabilities for image caption generation. A Fusion-based Recurrent Multi-Modal (FRMM) model consisting of CNN-LSTM framework together with a fusion stage generates captions by combining visual and textual modality outputs [ 19 ]. A better comprehensive description can be generated using a Recurrent Fusion Network (RFNet) [ 20 ] or by modifying the CNN-LSTM architecture by incorporating semantic attribute based features [ 21 ] and context-word features [ 22 ]. Attempts are also made by simultaneously introducing a dual attention strategy in both visual as well as textual information to improve the image understanding [ 23 , 24 ]. Better descriptions can be produced using multimodal RNN framework that makes use of inferred alignments between segments of the image and sentences describing them [ 25 ]. To make mandatory correspondence between descriptive text words and image regions effective, Deng et al. proposed a Dense network and adaptive attention technique [ 26 ]. A multitask learning method through a dual learning mechanism for cross-domain image captioning is proposed in [ 27 ]. It uses reinforced learning algorithm to acquire highly rewarded captions. Attempts for better caption generation has also been done with the development of algorithms with multi-gate attention networks [ 28 ], CaptionNet model with reduced dependency on previously predicted words [ 29 ], context aware visual policy networks [ 30 ] and adaptive attention mechanisms [ 31 , 32 , 33 ]. A new positional encoding scheme is proposed for enhancing the object features in the image, which greatly improves the attention information for generating more enriched captions is presented in [ 34 ]. A novel technique to understand the image and language modality configuration for achieving better semantic extraction from images using anchor points are presented in [ 35 ]. Visual language modelling can also be accomplished using a Task-Agnostic and Modality Agnostic sequence-to-sequence learning framework [ 36 ]. Another image captioning technique employs a configuration that utilizes a set of memory vectors together with meshed connectivity between encoding and decoding sections of the transformer model [ 37 ]. A scaling rule for image captioning is proposed in [ 38 ] by introducing a LargE-scale iMage captiONer (LEMON) dataset, which is capable of identifying long-tail visual concepts even in a zero shot mechanism. [ 39 ] presents a novel methodology for vision language task that is pre-trained on much larger text-image corpora and is able to collect much better visual features or concepts.
Even though these approaches perform well in image caption generation, they still lack the inclusion of fine grained semantic details as well as contextual spatial relationship between different objects in the image, which need to be improved further with enhanced network architectures. Also, due to the presence of multiple objects in the image, it is very essential that the visual contextual relationship between objects must be in correct sequential order to generate a caption with which the contents in the image is better represented. This can be solved by introducing attention mechanisms and considering the spatial relationship between objects in the model.
Model architecture
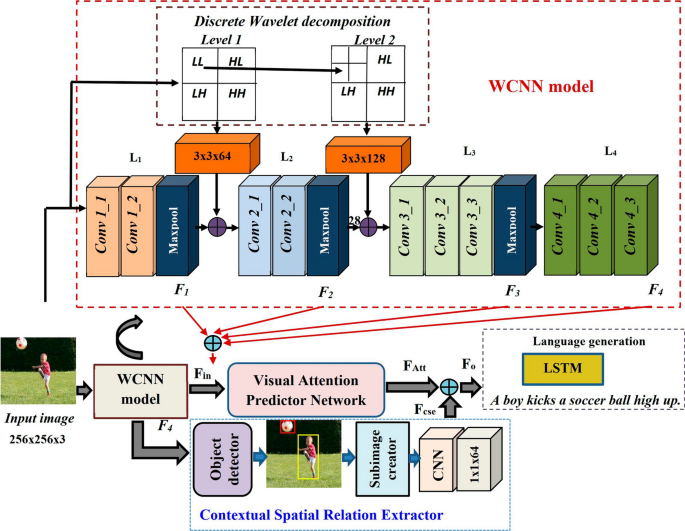
Proposed model architecture
The model consists of an encoder-decoder framework with VAPN, that converts an input image I to a sequence of encoded words, W = [ \(w_{1}\) , \(w_{2}\) ,... \(w_{L}\) ], with \(w_{i}\) \(\in\) \({\mathbb {R}}^{N}\) , describing the image, where L is the length of the generated caption and N is the vocabulary size. The detailed architecture is presented in Fig. 1 . The encoder consists of the WCNN model that incorporates two levels of discrete wavelet decomposition combined with CNN layers to obtain the visual features of the image. The features maps \(F_1\) and \(F_2\) obtained from the CNN layers are bilinearly downsampled and are concatenated together with \(F_3\) and \(F_4\) to produce a combined feature map, \(F_{in}\) of size, 32 \(\times\) 32 \(\times\) 960. This is then given to the VAPN for obtaining attention based feature maps that highlights the semantic details in I by exploiting channel as well spatial attention. In order to extract the contextual spatial relationship between the objects in I , the feature map of level \(L_{4}\) of WCNN, \(F_{4}\) , is given to the CSE network as shown in Fig. 1 . The contextual spatial feature map, \(F_{cse}\) , generated by the CSE network is concatenated with the attention based feature map, \(F_{Att}\) , to produce \(F_{o}\) and is provided to the language generation stage consisting of LSTM decoder network [ 40 ]. The detailed description of the technique is presented below.
Wavelet transform based CNN model
The image is resized to 256 \(\times\) 256 and is split up into R, G and B components. Then each of these planes is decomposed into details and approximations using low pass and high pass discrete wavelet filters. In this work, two levels of discrete wavelet decomposition are used that results in the formation of LL , LH , HL and HH sub-bands, where L and H represents the low frequency and high frequency components of the input. In the second level decomposition, only the approximations ( LL ) of R, G and B will be further decomposed for each of the three components. These components are then stacked together at each level and are concatenated with the outputs of the first two levels of CNN having four levels ( \(L_1\) to \(L_4\) ) consisting of multiple convolutional layers and pooling layers of kernel size 2 \(\times\) 2 with stride 2 as shown in Fig. 1 . The details of various convolution layers are given in Tables 1 and 2 .
The image feature maps \(F_1\) through \(F_4\) so obtained are then given to the VAPN network for extracting the inter-spatial as well as inter-channel relationship between them.
Visual attention predictor network
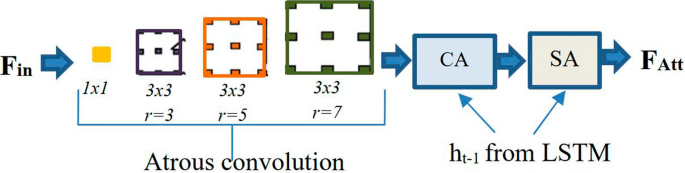
Structure of VAPN
To have good insight of the visual features of the image for acquiring the most relevant caption, it is necessary to extract out the semantic features from the input image feature maps, \(F_1\) through \(F_4\) . The size, shape, texture features etc. of the objects vary in an image, which causes difficulties in its identification or recognition. To tackle these situations, atrous convolutional network with multi-receptive field filters are employed, which extracts out more semantic details from the image as these filters are capable of delivering a wider field of view at the same computational cost. In this work, four multi-receptive filters—one having 64 filters of kernel size 1 \(\times\) 1, and the rest three of kernel size 3 \(\times\) 3 each with 64 filters of dilation rates 3, 5 and 7, respectively, are used. The combined feature map, \(F_{in}\) , is subjected to atrous convolution and the resultant enhanced feature map of size 32 \(\times\) 32 \(\times\) 256, is given to a sequential combination of channel attention (CA) network and spatial attention (SA) network as illustrated in Fig. 2 . The CA network examines the relationship between channels and more weight will be assigned to those channel having attentive regions to generate the refined channel attention map, whereas the SA network generates a spatial attention map by considering the spatial details between various feature maps.
For more accurate descriptions, the weights of channel attention and spatial attention, \(W_C\) and \(W_S\) are computed by considering \(h_{t-1}\) \(\in\) \({\mathbb {R}}^{D}\) , the hidden state of LSTM memory at \((t-1)\) time step [ 14 ]. Here D represents the hidden state dimension. This mechanism helps to include more contextual information in the image during caption generation.
In the channel-wise attention network, the feature map of \(F_{in}\) \(\in\) \({\mathbb {R}}^{H\times W \times C}\) is first average pooled channel-wise to obtain a channel feature vector, \(V_{C}\) \(\in\) \({\mathbb {R}}^{C}\) , where H , W and C represent the height, width and total number of channels of the feature map, respectively.
Then channel attention weights, \(W_C\) can be computed as,
where \(W^{'}_{C}\) \(\in\) \({\mathbb {R}}^{K}\) , \(W_{hc}\) \(\in\) \({\mathbb {R}}^{K\times D}\) and \(W_{o}\) \(\in\) \({\mathbb {R}}^{K}\) are the transformation matrices with K denoting the common mapping space dimension of image feature map and hidden state of the LSTM network, \(\odot\) and \(\oplus\) represent the element-wise multiplication and addition of vectors, \(b_{C}\) \(\in\) \({\mathbb {R}}^{K}\) and \(b_{o}\) \(\in\) \({\mathbb {R}}^{1}\) denote the bias terms. Then F \(_{in}\) is element-wise multiplied with \(W_{C}\) and the resultant channel refined feature map, F \(_{ch}\) , is given to the spatial attention network.
In the spatial attention network, the weights, \(W_S\) , can be calculated with flattened F \(_{ch}\) , as follows,
where \(W^{'}_{S}\) \(\in\) \({\mathbb {R}}^{K\times C}\) , \(W_{hs}\) \(\in\) \({\mathbb {R}}^{K\times D}\) and \(W^{'}_{o}\) \(\in\) \({\mathbb {R}}^{K}\) are the transformation matrices, \(b_{S}\) \(\in\) \({\mathbb {R}}^{K}\) and \(b^{'}_{o}\) \(\in\) \({\mathbb {R}}^{1}\) denote the bias terms. After this, F \(_{in}\) is element-wise multiplied with \(W_{S}\) to obtain the attention feature map, F \(_{Att}\) , highlighting the semantic details in the image.
Contextual spatial relation extractor
Rich image captioning can be achieved only through the exploitation of contextual spatial relation between the different objects in the image in addition to the semantic details. For this, first the regions occupied by the various objects are identified using a network configuration similar to Faster RCNN [ 41 ] incorporating WCNN model and RPN along with classifier and regression layers for creating bounding boxes. The feature map \(F_{4}\) from level \(L_{4}\) of WCNN model is given as input to the Region Proposal Network (RPN) for finding out the object regions in the image. Then these objects are paired and different sub images containing each of the identified object pairs are created and resized to 32 \(\times\) 32 for uniformity. Each of these sub images are given to the CNN layers with three sets of 64 filters, each with receptive field of 3 \(\times\) 3, to generate the features describing spatial relations between the object pairs. The spatial relation feature maps of each object pair is then stacked together and is given to 1 \(\times\) 1 \(\times\) 64 convolution layer to form the contextual spatial relation feature map, \(F_{cse}\) , of size 32 \(\times\) 32 \(\times\) 64, which is further concatenated with \(F_{Att}\) to obtain \(F_{o}\) and is given to the LSTM for the generation of the next caption word.
The prediction of the output word at time step t , is nothing but the probability of selecting suitable word from the pre-defined dictionary containing all the caption words.
The model architecture is trained to optimize the categorical cross entropy loss, \(L_{CE}\) , given by
where \(y_{1:t-1}\) represents the ground truth sequence and \(\theta\) represents the parameters. The self-critical training strategy [ 42 ] is used in this method to solve the exposure bias problem during the optimization with cross-entropy loss alone. Initially, the model is trained using cross-entropy loss and is further optimized in a reinforced learning technique with self-critical loss for achieving the best CIDEr score as reward on validation set.
Experiments and results
The detailed description of the datasets used and the performance evaluation of the proposed method are presented in this section. Both quantitative as well as qualitative analysis of the method is carried out and is compared with the existing state-of-the-art methods.
Datasets and performance evaluation metrics used
The experiments are conducted on three benchmark datasets: (1) Flickr8K (8,000 images) [ 43 ], (2) Flickr30K (31,000 images) [ 44 ] and (3) Microsoft COCO dataset (82,783 images in training set, 40,504 images in validation set and 40,775 images in test set) [ 45 ]. All the images are associated with five sentences. Among these for the Flickr8K dataset, we have selected 6,000 images for training, 1,000 images for validation and 1,000 images for testing as per the official split of the dataset. For fair comparison with previous works, the publicly available Karpathy split for Flick30K and Microsoft COCO datasets have been adopted [ 46 ]. As per this, the Flickr30K dataset split is set to 29,000 images for training, 1,000 images for validation and 1,000 images for testing. In the case of MSCOCO dataset, the split is set as 5,000 images for validation, 5,000 images for testing and all others for training. The textual descriptions are pre-processed with the publicly available code in https://github.com/karpathy/neuraltalk , so that all the captions are converted to lowercase and the non-alphanumeric characters are discarded. Each caption length is limited to 40 and those words whose occurrence is less than five times in the ground truth sentences are removed from the vocabulary.
The performance evaluation of the proposed captioning model is done using the evaluation metrics—Bilingual Evaluation Understudy (BLEU@N (for N=1,2,3,4)) [ 47 ], Metric for Evaluation of Translation with Explicit ORdering (METEOR) [ 48 ], Recall-Oriented Understudy for Gisting Evaluation (ROUGE) [ 49 ] and Consensus-based Image Description Evaluation (CIDEr) [ 50 ] denoted as B@N, MT, R and CD, respectively. These metrics measures the consistency between n-gram occurrences in the caption generated and the ground-truth descriptions. It should be noted that the fair comparison of the results are reported by optimizing the methods with cross entropy loss.
Implementation details
To encode the input image to visual features, the Wavelet transform based CNN model with Birothogonal 1.5 wavelet (bior 1.5) [ 51 ] pretrained using ImageNet dataset [ 52 ] is used. For the modified Faster R-CNN configuration used in the Contextual spatial relation extractor, we have used an IoU threshold of 0.8 for region proposal suppression, and 0.2 for object class suppression, respectively. The LSTM employed in the decoding section is having a hidden state dimension set as 512, respectively. The caption generation process terminates with the occurrence of a special END token or if predefined max sentence length is reached. Batch normalization is applied to all convolutional layers with decay set as 0.9 and epsilon as 1e-5. Optimization of the model is done using ADAM optimizer [ 53 ] with an initial learning rate of 4e-3. The exponential decay rates for the first and second moment estimates are chosen as (0.8, 0.999). The mini batch size for the Flickr8K, Flickr30K and MSCOCO dataset are taken as 16, 32 and 64. The self critical training strategy is employed in the implementation, where the model is trained initially for 50 epochs with the cross-entropy loss and it is further fine tuned with 15 epochs using the self-critical loss for achieving the best CD score on validation set. To avoid overfitting, dropout rate is set as 0.2 and L2 regularization with weight decay value set as 0.001. For the word vector representation of each word, 300 dimensional GloVe word embeddings [ 54 ] pre-trained on a large-scale corpus is employed in this work. For the selection of best caption, BeamSearch strategy [ 3 ] is adopted with a beam size of 5, which selects best caption from few selected candidates. The proposed image captioning framework is implemented using TensorFlow 2.3. To train the proposed method, we used Nvidia Tesla V100 with 16GB with 5120 CUDA cores.
Analysis for the selection of appropriate mother wavelet
The choice of the mother wavelet has been done by analyzing the performance of the proposed method on four different wavelet families—Daubechies wavelets (dbN), biorthogonal Wavelets (biorNr.Nd), Coiflets (CoifN) and Symlets (SymN), where N represents number of vanishing moments, Nr and Nd denotes the number of vanishing moments in the reconstruction and decomposition filters, respectively. Table 2 gives the detailed performance results of the proposed image captioning method for Flickr8K, Flickr30K and MSCOCO datasets. Here a model consisting of WCNN with two-level DWT decomposition, VAPN and LSTM alone is considered. The contextual spatial relation extractor is not taken into account for this experimentation. In this, the baseline method (BM) is a model similar to the one described above without DWT decomposition. From Table 2 , it is evident that bior1.5 is giving good results compared to other wavelets used in the experimentation. This is because it employs separate scaling and wavelet functions for decomposition and reconstruction purposes. Biorthogonal wavelets exhibit the property of linear phase and allow additional degrees of freedom when compared to orthogonal wavelets.
Here for the Flickr30K dataset bior2.4 secures highest B@4 score of about 25.32 but bior1.5 scores better value for CD compared to the other wavelets. For Flickr30K and MSCOCO datasets, bior1.5 achieves better B@4 and CD scores of about 26.34 and 60.58 and about 37.14 and 120.41 for the MSCOCO dataset. Hence bior1.5 wavelet is chosen as the mother wavelet for experimentation using these datasets.
Analysis for the choice of DWT decomposition levels
For analysing the performance of the model with different DWT decomposition levels, experiments are carried out with the same baseline model as used for finding out the best mother wavelet. Here bior1.5 wavelet are employed. The detailed ablation study regarding the results obtained for various DWT decomposition levels for the MSCOCO dataset are presented in Table 3 .
The model with two level DWT decomposition exhibits better performance compared to 1-level DWT decomposition scoring an improvement in the B@4, MT and CD values of about 1.26%, 1.79% and 1.18%, respectively, for the MSCOCO dataset. The method also shows little performance improvements with the use of three level decomposition stages. Hence considering the computational complexity, method with two level decomposition is preferred in the proposed work.
Quantitative analysis
The quantitative evaluation of the proposed method for the MSCOCO dataset is carried out using the above mentioned metrics and the performance comparison is done with fifteen state-of-the-art methods in image captioning. These models include Deep VS [ 25 ], emb-gLSTM [ 5 ], Soft and Hard attention [ 17 ], ATT [ 55 ], SCA-CNN [ 14 ], LSTM-A [ 56 ], Up-down [ 7 ], SCST [ 42 ], RFNet [ 20 ], GCN-LSTM [ 57 ], avtmNet [ 58 ], ERNN [ 59 ], Tri-LSTM [ 60 ] and TDA+GLD [ 61 ].
Table 4 shows the evaluation results for the comparative study on MSCOCO ‘Karpathy’ test split. From Table 4 , it is evident that the proposed method outperforms the state-of-the-art methods with good CD score of 124.2. It acquires a relative improvement of about 0.9 \(\%\) , 0.5 \(\%\) , 0.2 \(\%\) and 0.7 \(\%\) in B@4, MT, R and CD score, respectively, compared to Tri-LSTM method [ 60 ]. This is because the image feature maps of the proposed model includes spectral information also along with spatial as well as semantic details compared to the other methods by the inclusion of discrete wavelet decomposition in the CNN model, which helps to extract fine grained information during object detection. Also it takes into account of contextual spatial relationship between objects in the image and exploits both spatial and channel-wise attention of the enhanced feature maps resulted from atrous convolution employing multi-receptive field filters that are capable of locating visually salient objects of varying shapes, scales and sizes.
The comparison results of the proposed method for the Flickr8K and Flickr30K datasets are tabulated in Tables 5 , 6 . From Table 5 , it is evident that the method outperforms the state-of-the-art methods with an improvement of 2.3 \(\%\) , 2.8 \(\%\) and 2.1 \(\%\) on B@1, B@4 and MT compared to SCA-CNN [ 14 ] for Flickr8K dataset. Also the proposed method scores an improvement of 2.4 \(\%\) and 0.9 \(\%\) on B@4 and MT in comparison with the avtmNet [ 58 ] method for Flickr30K dataset. It also secures a good CD value of about 67.3.
Qualitative results
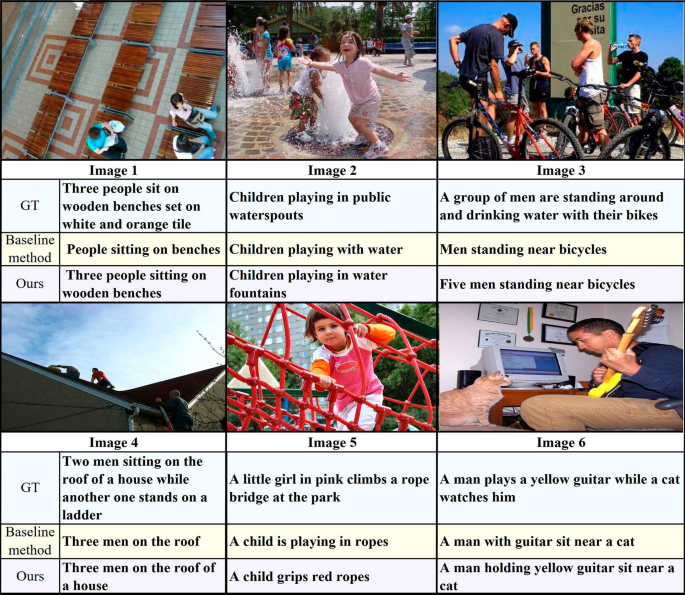
Comparison of the captions generated by the baseline approach and the proposed method for few samples images. Here GT represents the ground truth sentence
Figure 3 shows the captions generated by the proposed image captioning algorithm and the baseline approach for few sample images. Here the baseline method utilizes a VGG-16 network instead of WCNN network, together with the attention mechanism and LSTM configuration. The CSE network is not incorporated in the baseline method. For the qualitative study, images of simple scenes, complex scenes with multiple objects and also those having objects that is to be specified clearly with colour as well as their count are chosen. For the images 1 and 4, our method successfully identifies the number of persons in the image as ‘three’ . The proposed method generates more enriched captions for the images 1, 2, 5 and 6, by detecting ‘wooden benches’ , ‘water fountains’ , ‘red ropes’ and ‘yellow guitar’ . Image 3 consists of more complex scene than others with more number of objects, for which the method is able to distinguish ‘five men’ and ‘bicycles’ clearly along with the extract of relationship between them as ‘standing near’ in a better manner. Thus from Fig. 3 , it is evident that the use of attention mechnaism in WCNN structure and CSE network helps to include more finer details in the image, highlighting both spatial, semantic as well as contextual relationship between the various objects thereby generating better textual descriptions of the image.
Figure 4 shows a few failure captions generated by the proposed captioning method. The method is unable to extract the semantics from certain images due to incorrect detection and recognition of objects and also the contextual relation between them. For images 1 and 3, the objects are not distinguished from the background and in image 6, the activity is wrongly interpreted as ‘ walking ’. In image 2, the object ‘ remote ’ is identified as ‘ phone ’ and hence the method fails to recognize the activity of that complex scene. Similar error happened in the case of image 5, with the detection of ‘ rope ’ as some fishing tool. Image 4 is formed from a group of photos combined together highlighting the various moves and techniques of tennis players. In this situations, the method fails to extract the semantics and contextual information of the image generating a caption as ‘ Men dancing with tennis racquet ’.
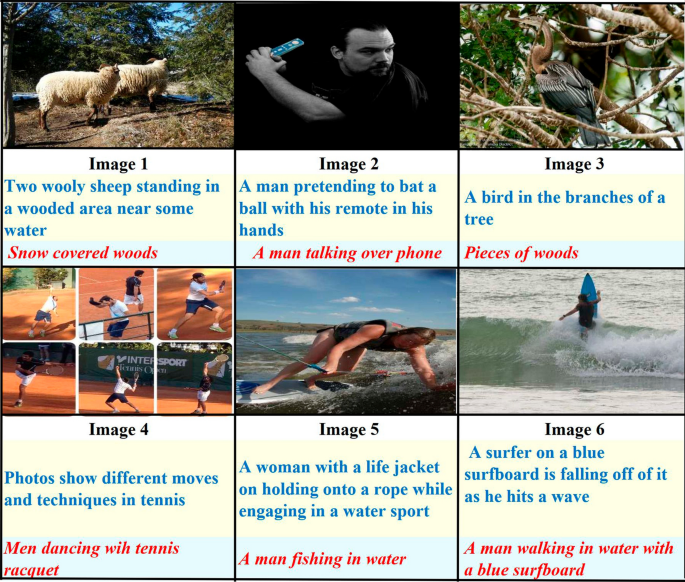
Few samples of failure captions generated by the proposed method. The descriptions given in blue and red represents the ground truth and generated captions, respectively
Ablation study
To evaluate the performance of the combination of various stages in the model, an ablation study is conducted. The evaluation results of various configurations of the proposed model with MSCOCO dataset for cross entropy loss and with self critical loss with CD score optimization are given in Table 7 . Experimental studies are conducted initially by using WCNN model as the encoder network together with atrous convolution to extract the enhanced feature maps, which are then directly fed to the LSTM network for translation. Then attention networks are included in different combinations to the enhanced feature maps, which generates enriched textual descriptions. At first, SA network alone was incorporated by considering the \(h_{t-1}\) hidden state of the LSTM that highlights the spatial locations according to the generation of words to form the descriptions. This improved the B@4 and CD score by about 0.8 \(\%\) and 1.6 \(\%\) for cross entropy loss and about 1.3 \(\%\) and 1.4 \(\%\) for self-critical loss, respectively. Further CA network is introduced and we tried both the sequential combinations with CA and SA networks: (SA+CA) and (CA+SA). The (CA+SA) combinational network proved to be better than the other by providing B@4 and CD score of about 37.1 and 120.4 for self critical loss as shown in Table 7 . Finally, the CSE network is also employed in the network, which enhances the B@4 and CD score by 38.2 and 124.2.
Figure 5 illustrates the captions generated by the proposed method for three sample images with their annotated ground-truth sentences. From the generated captions, it is evident that the proposed method is able to understand the visual concepts in the image in a superior manner and is successful in generating more suitable captions, reflecting the underlying semantics.
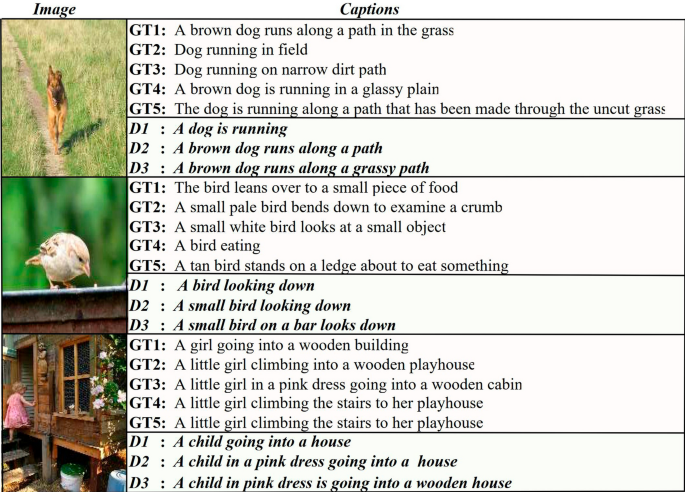
Samples of image captions generated by the DWCNN based image captioning method with the five ground truth sentences denoted as GT1, GT2, GT3, GT4 and GT5 respectively. D1 , D2 and D3 denotes the descriptions generated by the three configurations -WCNN+LSTM, WCNN+VPAN+LSTM amd WCNN+VAPN+CSE+LSTM, respectively
In this work, a deep neural architecture for image caption generation using encoder-decoder framework along with VAPN and CSE network is proposed. The inclusion of wavelet decomposition in the convolutional neural network extracts spatial, semantic and spectral information from the input image, which along with atrous convolution predicts the most salient regions in it. Rich image captions are obtained by employing spatial as well as channel-wise attention in the feature maps provided by the VAPN and also by considering the contextual spatial relationship between the objects in the image using CSE network. The experiment is conducted with three benchmark datasets, Flickr8K, Flickr16K and MSCOCO using BLEU, METEOR and CIDEr performance metrics. It achieved a good B@4, MT and CD score of 38.2, 28.9 and 124.2, respectively, for MSCOCO dataset. This proves its effectiveness. Since contextual information are utilised, finer semantics can be included in the generated captions. In future works, instead of LSTM, more advanced transformer network can be considered for achieving better CD score and finer captions. By the incorporation of temporal attention, the work can be extended for caption generation in videos.
Availability of data and materials
1. The Flickr 8k datset is available at https://www.kaggle.com/dibyansudiptiman/flickr-8k . 2. The MS COCO dataset is available at: https://cocodataset.org/ . 3. The Flickr30k dataset is available at: https://shannon.cs.illinois.edu/DenotationGraph/ .
Abbreviations
Wavelet transform based Convolutional Neural Networks
Visual Attention Prediction Network
Long Short Term Memory
Recurrent Neural Network
Gated Recurrent Unit
Contextual Spatial Relation Extractor
Conditional random field
Fusion-based Recurrent Multi-Modal
Recurrent Fusion Network
Convolutional Neural Network
Channel attention
Spatial attention
Region Proposal Network
Bilingual Evaluation Understudy
Metric for Evaluation of Translation with Explicit ORdering
Recall-Oriented Understudy for Gisting Evaluation
Consensus-based Image Description Evaluation
Li S, Kulkarni G, Berg TL, Berg AC, Choi Y. Composing simple image descriptions using web-scale n-grams. In: Proceedings of the Fifteenth Conference on Computational Natural Language Learning, 2011, pp. 220–228
Lin D. An information-theoretic definition of similarity. In: Proceedings of the Fifteenth International Conference on Machine Learning, 1998, pp. 296–304
Vinyals O, Toshev A, Bengio S, Erhan D. Show and tell: A neural image caption generator. In: IEEE Conference on Computer Vision and Pattern Recognition, 2015, pp. 3156–3164.
Jing Z, Kangkang L, Zhe W. Parallel-fusion lstm with synchronous semantic and visual information for image captioning. J Vis Commun Image Represent. 2021;75(8): 103044.
Google Scholar
Jia X, Gavves E, Fernando B, Tuytelaars T. Guiding the long-short term memory model for image caption generation. In: Proceedings of the IEEE International Conference on Computer Vision (ICCV), 2015
Gao L, Wang X, Song J, Liu Y. Fused GRU with semantic-temporal attention for video captioning. Neurocomputing. 2020;395:222–8.
Article Google Scholar
Anderson P, He X, Buehler C, Teney D, Johnson M, Gould S, Zhang L. Bottom-up and top-down attention for image captioning and visual question answering. In: CVPR, 2018: pp. 6077–6086.
Fu K, Jin J, Cui R, Sha F, Zhang C. Aligning where to see and what to tell: image captioning with region-based attention and scene-specific contexts. IEEE Trans Pattern Anal Mach Intell. 2017;39(12):2321–34.
Farhadi A, Hejrati M, Sadeghi MA, Young P, Rashtchian C, Hockenmaier J, Forsyth D. Every picture tells a story: generating sentences from images. In: Computer Vision – ECCV, 2010, pp. 15–29.
Kulkarni G, Premraj V, Dhar S, Li S, Choi Y, Berg AC, Berg TL. Baby talk: understanding and generating simple image descriptions. In: CVPR, 2011; pp. 1601–1608.
Mitchell M, Han X, Dodge J, Mensch A, Goyal A, Berg A, Yamaguchi K, Berg T, Stratos K, Daumé H. Midge: Generating image descriptions from computer vision detections. In: Proceedings of the 13th Conference of the European Chapter of the Association for Computational Linguistics, 2012; pp. 747–756.
Ushiku Y, Harada T, Kuniyoshi Y. Efficient image annotation for automatic sentence generation. In: Proceedings of the 20th ACM International Conference on Multimedia, 2012; pp. 549–558.
Mason R, Charniak E. Nonparametric method for data-driven image captioning. In: Proceedings of the 52nd Annual Meeting of the Association for Computational Linguistics (Volume 2: Short Papers), Association for Computational Linguistics, 2014; pp. 592–598.
Chen L, Zhang H, Xiao J, Nie L, Shao J, Liu W, Chua T. Sca-cnn: Spatial and channel-wise attention in convolutional networks for image captioning. In: 2017 IEEE Conference on Computer Vision and Pattern Recognition (CVPR), 2017: pp. 6298–6306.
Guo L, Liu J, Zhu X, Yao P, Lu S, Lu H. Normalized and geometry-aware self-attention network for image captioning. In: IEEE/CVF Conference on Computer Vision and Pattern Recognition (CVPR); 2020.
Pan Y, Yao T, Li Y, Mei T. X-linear attention networks for image captioning. In: IEEE/CVF Conference on Computer Vision and Pattern Recognition (CVPR); 2020.
Xu K, Ba J, Kiros R, Cho K, Courville A, Salakhudinov R, Zemel R, Bengio Y. Show, attend and tell: Neural image caption generation with visual attention. In: Proceedings of the 32nd International Conference on Machine Learning, vol. 37, 2015; pp. 2048–2057.
Yang Z, Yuan Y, Wu Y, Cohen WW, Salakhutdinov RR. Review networks for caption generation. In: Advances in Neural Information Processing Systems, vol. 29, 2016
Oruganti RM, Sah S, Pillai S, Ptucha R. Image description through fusion based recurrent multi-modal learning. In: 2016 IEEE International Conference on Image Processing (ICIP), 2016: pp. 3613–3617.
Jiang W, Ma L, Jiang Y, Liu W, Zhang T. Recurrent fusion network for image captioning. In: Proceedings of the European Conference on Computer Vision (ECCV); 2018.
Wang, W., Ding, Y., Tian, C.: A novel semantic attribute-based feature for image caption generation. In: 2018 IEEE International Conference on Acoustics, Speech and Signal Processing (ICASSP), 2018; pp. 3081–3085
Donahue J, Hendricks LA, Rohrbach M, Venugopalan S, Guadarrama S, Saenko K. Long-term recurrent convolutional networks for visual recognition and description. IEEE Trans Pattern Anal Mach Intell. 2017;39(4):677–91.
Yu L, Zhang J, Wu Q. Dual attention on pyramid feature maps for image captioning. IEEE Transactions on Multimedia; 2021
Liu M, Li L, Hu H, Guan W, Tian J. Image caption generation with dual attention mechanism. Inf Process Manag. 2020;57(2): 102178.
Karpathy A, Fei-Fei L. Deep visual-semantic alignments for generating image descriptions. IEEE Trans Pattern Anal Mach Intell. 2017;39(4):664–76.
Deng Z, Jiang Z, Lan R, Huang W, Luo X. Image captioning using densenet network and adaptive attention. Signal Process Image Commun. 2020;85: 115836.
Yang M, Zhao W, Xu W, Feng Y, Zhao Z, Chen X, Lei K. Multitask learning for cross-domain image captioning. IEEE Multimedia. 2019;21(4):1047–61.
Jiang W, Li X, Hu H, Lu Q, Liu B. Multi-gate attention network for image captioning. IEEE Access. 2021;9:69700–9. https://doi.org/10.1109/ACCESS.2021.3067607 .
Yang L, Wang H, Tang P, Li Q. Captionnet: a tailor-made recurrent neural network for generating image descriptions. IEEE Trans Multimedia. 2021;23:835–45.
Zha Z-J, Liu D, Zhang H, Zhang Y, Wu F. Context-aware visual policy network for fine-grained image captioning. IEEE Trans Pattern Anal Mach Intell. 2022;44(2):710–22.
Gao L, Li X, Song J, Shen HT. Hierarchical lstms with adaptive attention for visual captioning. IEEE Trans Pattern Anal Mach Intell. 2020;42(5):1112–31.
Yan C, Hao Y, Li L, Yin J, Liu A, Mao Z, Chen Z, Gao X. Task-adaptive attention for image captioning. IEEE Trans Circuits Syst Video Technol. 2022;32(1):43–51.
Xiao H, Shi J. Video captioning with adaptive attention and mixed loss optimization. IEEE Access. 2019;7:135757–69.
Al-Malla MA, Jafar A, Ghneim N. Image captioning model using attention and object features to mimic human image understanding. J Big Data. 2022;9:20.
Li X, Yin X, Li C, Zhang P, Hu X, Zhang L, Wang L, Hu H, Dong L, Wei F, Choi Y, Gao J. Oscar: object-semantics aligned pre-training for vision-language tasks. In: ECCV; 2020
Wang P, Yang A, Men R, Lin J, Bai S, Li Z, Ma J, Zhou C, Zhou J, Yang H. Ofa: Unifying architectures, tasks, and modalities through a simple sequence-to-sequence learning framework. CoRR; 2022. abs/2202.03052
Cornia M, Stefanini M, Baraldi L, Cucchiara R. Meshed-memory transformer for image captioning. In: Proceedings of the IEEE/CVF Conference on Computer Vision and Pattern Recognition 2020.
Hu X, Gan Z, Wang J, Yang Z, Liu Z, Lu Y, Wang L. Scaling up vision-language pre-training for image captioning. CoRR 2021. abs/2111.12233 . arXiv2111.12233
Zhang P, Li X, Hu X, Yang J, Zhang L, Wang L, Choi Y, Gao J. Vinvl: Making visual representations matter in vision-language models. CoRR 2021. abs/2101.00529
Hochreiter S, Schmidhuber J. Long short-term memory. Neural Comput. 1997;9:1735–80.
Ren S, He K, Girshick R, Sun J. Faster r-cnn: Towards real-time object detection with region proposal networks. In: Proceedings of the 28th International Conference on Neural Information Processing Systems—Volume 1. NIPS’15, 2015: 91–99.
Rennie SJ, Marcheret E, Mroueh Y, Ross J, Goel V. Self-critical sequence training for image captioning. In: 2017 IEEE Conference on Computer Vision and Pattern Recognition (CVPR), 2017; pp. 1179–1195.
Hodosh M, Young P, Hockenmaier J. Framing image description as a ranking task: data, models and evaluation metrics. J Artif Intell Res. 2013;47:853–99.
Article MathSciNet MATH Google Scholar
Young P, Lai A, Hodosh M, Hockenmaier J. From image descriptions to visual denotations: new similarity metrics for semantic inference over event descriptions. Trans Assoc Comput Linguist. 2014;2:67–78.
Lin TY, Maire M, Belongie S, Hays J, Perona P, Ramanan D, Doll P, Zitnick CL. Microsoft coco: common objects in context. In: Computer Vision – ECCV 2014; pp. 740–755.
Karpathy A, Fei-Fei L. Deep visual-semantic alignments for generating image descriptions. In: 2015 IEEE Conference on Computer Vision and Pattern Recognition (CVPR), 2015; pp. 3128–3137.
Papineni K, Roukos S, Ward T, Zhu W. Bleu: A method for automatic evaluation of machine translation. In: Proceedings of the 40th Annual Meeting on Association for Computational Linguistics, 2002; pp. 311–318.
Lavie A, Agarwal A. Meteor: An automatic metric for mt evaluation with high levels of correlation with human judgments. In: Proceedings of the Second Workshop on Statistical Machine Translation, 2007; pp. 228–231.
Lin C. ROUGE: A package for automatic evaluation of summaries. In: Text summarization branches out, 2004; pp. 74–81.
Vedantam R, Zitnick CL, Parikh D. CIDEr: Consensus-based Image Description Evaluation 2015. arXiv1411.5726
Sweldens W. The lifting scheme: a custom-design construction of biorthogonal wavelets. Appl Comput Harmon Anal. 1996;3(2):186–200.
Deng J, Dong W, Socher R, Li L, L, K, F, L. Imagenet: A large-scale hierarchical image database. In: 2009 IEEE Conference on Computer Vision and Pattern Recognition, 2009; pp. 248–255.
Kingma DP, Ba J. Adam: A Method for Stochastic Optimization (2014). arXiv1412.6980
Pennington J, Socher R, Manning CD. Glove: Global vectors for word representation. In: Empirical Methods in Natural Language Processing (EMNLP), 2014; pp. 1532–1543. http://www.aclweb.org/anthology/D14-1162
You Q, Jin H, Wang Z, Fang C, Luo J. Image captioning with semantic attention. In: 2016 IEEE Conference on Computer Vision and Pattern Recognition (CVPR), 2016; pp. 4651–4659.
Yao T, Pan Y, Li Y, Qiu Z, Mei T. Boosting image captioning with attributes. In: 2017 IEEE International Conference on Computer Vision (ICCV), 2017: 4904–4912.
Yao T, Pan Y, Li Y, Mei T. Exploring visual relationship for image captioning. In: ECCV 2018; 2017
Song H, Zhu J, Jiang Y. avtmnet: adaptive visual-text merging network for image captioning. Comput Electr Eng. 2020;84: 106630.
Wang H, Wang H, Xu K. Evolutionary recurrent neural network for image captioning. Neurocomputing. 2020;401:249–56.
Hossain MZ, Sohel F, Shiratuddin MF, Laga H, Bennamoun M. Bi-san-cap: Bi-directional self-attention for image captioning. In: 2019 Digital Image Computing: Techniques and Applications (DICTA), 2019; pp. 1–7.
Wu J, Chen T, Wu H, Yang Z, Luo G, Lin L. Fine-grained image captioning with global-local discriminative objective. IEEE Trans Multimedia. 2021;23:2413–27.
Wang S, Meng Y, Gu Y, Zhang L, Ye X, Tian J, Jiao L. Cascade attention fusion for fine-grained image captioning based on multi-layer lstm. In: 2021 IEEE International Conference on Acoustics, Speech and Signal Processing, 2021; pp. 2245–2249.
Download references
Acknowledgements
Not applicable.
Author information
Authors and affiliations.
Department of Electronics and Communication Engineering, College of Engineering Trivandrum, Thiruvanathapuram, 695016, Kerala, India
Reshmi Sasibhooshan
Department of Electronics and Communication Engineering, Government Engineering College, Mananthavady, Wayanad, 670644, Kerala, India
Suresh Kumaraswamy
Department of Electronics and Communication Engineering, Government Engineering College, Painav, Idukki, 685603, Kerala, India
Santhoshkumar Sasidharan
You can also search for this author in PubMed Google Scholar
Contributions
RS has performed design, implementation and experiments, and written original version of the final manuscript under the guidance, supervision and support of SK and SS. All authors contributed equally in this work and all authors read and approved the final manuscript.
Corresponding author
Correspondence to Reshmi Sasibhooshan .
Ethics declarations
Ethics approval and consent to participate.
Not applicable
Consent for publication
The authors have no objection in publishing the manuscript if accepted after review.
Competing interests
The authors declare that they have no competing interests.
Additional information
Publisher's note.
Springer Nature remains neutral with regard to jurisdictional claims in published maps and institutional affiliations.
Rights and permissions
Open Access This article is licensed under a Creative Commons Attribution 4.0 International License, which permits use, sharing, adaptation, distribution and reproduction in any medium or format, as long as you give appropriate credit to the original author(s) and the source, provide a link to the Creative Commons licence, and indicate if changes were made. The images or other third party material in this article are included in the article's Creative Commons licence, unless indicated otherwise in a credit line to the material. If material is not included in the article's Creative Commons licence and your intended use is not permitted by statutory regulation or exceeds the permitted use, you will need to obtain permission directly from the copyright holder. To view a copy of this licence, visit http://creativecommons.org/licenses/by/4.0/ .
Reprints and permissions
About this article
Cite this article.
Sasibhooshan, R., Kumaraswamy, S. & Sasidharan, S. Image caption generation using Visual Attention Prediction and Contextual Spatial Relation Extraction. J Big Data 10 , 18 (2023). https://doi.org/10.1186/s40537-023-00693-9
Download citation
Received : 24 February 2022
Accepted : 21 January 2023
Published : 08 February 2023
DOI : https://doi.org/10.1186/s40537-023-00693-9
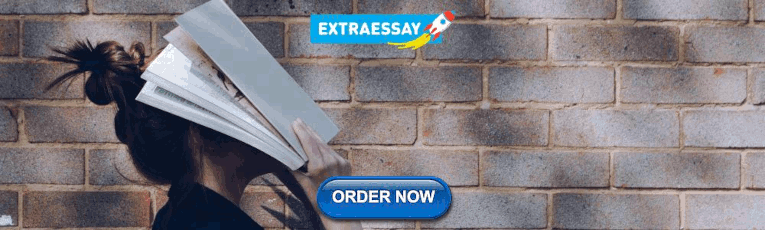
Share this article
Anyone you share the following link with will be able to read this content:
Sorry, a shareable link is not currently available for this article.
Provided by the Springer Nature SharedIt content-sharing initiative
- Image captioning
- Wavelet transform based Convolutional Neural Network
- Visual Attention Prediction
- Contextual Spatial Relation Extraction
Show and tell: A neural image caption generator
Research areas.
Machine Perception
Machine Intelligence
Meet the teams driving innovation
Our teams advance the state of the art through research, systems engineering, and collaboration across Google.
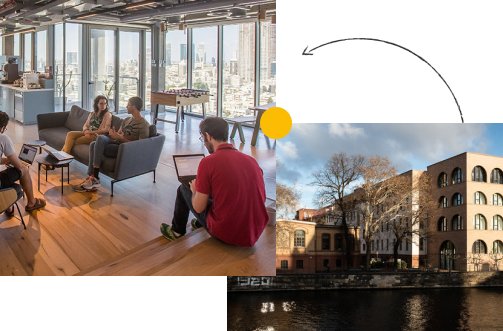
Information
- Author Services
Initiatives
You are accessing a machine-readable page. In order to be human-readable, please install an RSS reader.
All articles published by MDPI are made immediately available worldwide under an open access license. No special permission is required to reuse all or part of the article published by MDPI, including figures and tables. For articles published under an open access Creative Common CC BY license, any part of the article may be reused without permission provided that the original article is clearly cited. For more information, please refer to https://www.mdpi.com/openaccess .
Feature papers represent the most advanced research with significant potential for high impact in the field. A Feature Paper should be a substantial original Article that involves several techniques or approaches, provides an outlook for future research directions and describes possible research applications.
Feature papers are submitted upon individual invitation or recommendation by the scientific editors and must receive positive feedback from the reviewers.
Editor’s Choice articles are based on recommendations by the scientific editors of MDPI journals from around the world. Editors select a small number of articles recently published in the journal that they believe will be particularly interesting to readers, or important in the respective research area. The aim is to provide a snapshot of some of the most exciting work published in the various research areas of the journal.
Original Submission Date Received: .
- Active Journals
- Find a Journal
- Proceedings Series
- For Authors
- For Reviewers
- For Editors
- For Librarians
- For Publishers
- For Societies
- For Conference Organizers
- Open Access Policy
- Institutional Open Access Program
- Special Issues Guidelines
- Editorial Process
- Research and Publication Ethics
- Article Processing Charges
- Testimonials
- Preprints.org
- SciProfiles
- Encyclopedia
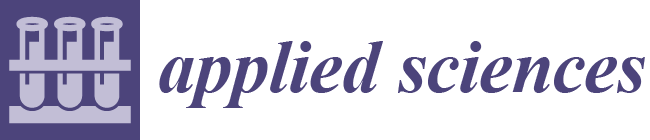
Article Menu
- Subscribe SciFeed
- Recommended Articles
- Google Scholar
- on Google Scholar
- Table of Contents
Find support for a specific problem in the support section of our website.
Please let us know what you think of our products and services.
Visit our dedicated information section to learn more about MDPI.
JSmol Viewer
A systematic literature review on image captioning.
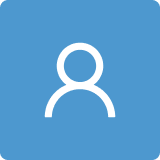
1. Introduction
2. slr methodology, 2.1. search sources.
- IEEE Xplore
- Web of Science—WOS (previously known as Web of Knowledge)
2.2. Search Questions
- What techniques have been used in image caption generation?
- What are the challenges in image captioning?
- How does the inclusion of novel image description and addition of semantics improve the performance of image captioning?
- What are the newest researches on image captioning?
2.3. Search Query
- Year ○ 2016; ○ 2017; ○ 2018; ○ 2019;
- Feature extractors: ○ AlexNet; ○ VGG-16 Net; ○ ResNet; ○ GoogleNet (including all nine Inception models); ○ DenseNet;
- Language models: ○ LSTM; ○ RNN; ○ CNN; ○ cGRU; ○ TPGN;
- Methods: ○ Encoder-decoder; ○ Attention mechanism; ○ Novel objects; ○ Semantics;
- Results on datasets: ○ MS COCO; ○ Flickr30k;
- Evaluation metrics: ○ BLEU-1; ○ BLEU-2; ○ BLEU-3; ○ BLEU-4; ○ CIDEr; ○ METEOR;
4. Discussion
4.1. model architecture and computational resources, 4.1.1. encoder—cnn, 4.1.2. decoder—lstm, 4.1.3. attention mechanism, 4.2. datasets, 4.3. human-like feeling, 4.4. comparison of results, 5. conclusions, author contributions, conflicts of interest.
Image Encoder | Image Decoder | Method | MS COCO | Flickr30k | |||||||||||||||||||||||||||||
---|---|---|---|---|---|---|---|---|---|---|---|---|---|---|---|---|---|---|---|---|---|---|---|---|---|---|---|---|---|---|---|---|---|
Year | Citation | AlexNet | VGGNet | GoogleNet | ResNet | DenseNet | LSTM | RNN | CNN | cGRU | TPGN | Encoder-Decoder | Attention | Novel Objects | Semantics | Bleu-1 | Bleu-2 | Bleu-3 | Bleu-4 | CIDEr | Meteor | Bleu-1 | Bleu-2 | Bleu-3 | Bleu-4 | CIDEr | Meteor | ||||||
2016 | [ ] | x | x | Lifelog Dataset | |||||||||||||||||||||||||||||
2016 | [ ] | x | x | x | x | 67.2 | 49.2 | 35.2 | 24.4 | 62.1 | 42.6 | 28.1 | 19.3 | ||||||||||||||||||||
2016 | [ ] | x | x | x | x | 50.0 | 31.2 | 20.3 | 13.1 | 61.8 | 16.8 | ||||||||||||||||||||||
2016 | [ ] | x | x | x | x | 71.4 | 50.5 | 35.2 | 24.5 | 63.8 | 21.9 | ||||||||||||||||||||||
2017 | [ ] | x | x | ||||||||||||||||||||||||||||||
2017 | [ ] | x | x | x | x | 63.8 | 44.6 | 30.7 | 21.1 | ||||||||||||||||||||||||
2017 | [ ] | x | x | CUB-Justify | |||||||||||||||||||||||||||||
2017 | [ ] | x | x | x | 27.2 | ||||||||||||||||||||||||||||
2017 | [ ] | x | x | x | 66.9 | 46 | 32.5 | 22.6 | |||||||||||||||||||||||||
2017 | [ ] | x | x | x | x | 70.1 | 50.2 | 35.8 | 25.5 | 24.1 | 67.9 | 44 | 29.2 | 20.9 | 19.7 | ||||||||||||||||||
2017 | [ ] | x | x | Visual Genome Dataset | |||||||||||||||||||||||||||||
2017 | [ ] | x | x | x | 19.9 | 13.7 | 13.1 | ||||||||||||||||||||||||||
2017 | [ ] | x | x | x | x | ||||||||||||||||||||||||||||
2017 | [ ] | x | x | x | x | 70.9 | 53.9 | 40.6 | 30.5 | 90.9 | 24.3 | ||||||||||||||||||||||
2017 | [ ] | x | 31.1 | 93.2 | |||||||||||||||||||||||||||||
2017 | [ ] | x | x | x | x | 71.3 | 53.9 | 40.3 | 30.4 | 93.7 | 25.1 | ||||||||||||||||||||||
2017 | [ ] | x | x | x | x | 30.7 | 93.8 | 24.5 | |||||||||||||||||||||||||
2017 | [ ] | x | x | x | x | x | x | 72.4 | 55.5 | 41.8 | 31.3 | 95.5 | 24.8 | 64.9 | 46.2 | 32.4 | 22.4 | 47.2 | 19.4 | ||||||||||||||
2017 | [ ] | x | x | x | x | 73.4 | 56.7 | 43 | 32.6 | 96 | 24 | ||||||||||||||||||||||
2017 | [ ] | x | x | x | 32.1 | 99.8 | 25.7 | 66 | |||||||||||||||||||||||||
2017 | [ ] | x | x | x | 42 | 31.9 | 101.1 | 25.7 | 32.5 | 22.9 | 44.1 | 19 | |||||||||||||||||||||
2017 | [ ] | x | x | x | 39.3 | 29.9 | 102 | 24.8 | 37.2 | 30.1 | 76.7 | 21.5 | |||||||||||||||||||||
2017 | [ ] | x | x | x | x | x | x | 91 | 83.1 | 72.8 | 61.7 | 102.9 | 35 | ||||||||||||||||||||
2017 | [ ] | x | x | x | x | 73.1 | 56.7 | 42.9 | 32.3 | 105.8 | 25.8 | ||||||||||||||||||||||
2017 | [ ] | x | x | x | x | 74.2 | 58 | 43.9 | 33.2 | 108.5 | 26.6 | 67.7 | 49.4 | 35.4 | 25.1 | 53.1 | 20.4 | ||||||||||||||||
2018 | [ ] | x | x | Recall Evaluation metric | |||||||||||||||||||||||||||||
2018 | [ ] | x | x | OI, VG, VRD | |||||||||||||||||||||||||||||
2018 | [ ] | x | x | X | X | 71.6 | 51.8 | 37.1 | 26.5 | 24.3 | |||||||||||||||||||||||
2018 | [ ] | x | x | APRC, CSMC | |||||||||||||||||||||||||||||
2018 | [ ] | x | x | x | x | F-1 score metrics | 21.6 | ||||||||||||||||||||||||||
2018 | [ ] | x | x | x | x | x | |||||||||||||||||||||||||||
2018 | [ ] | x | x | x | x | 72.5 | 51 | 36.2 | 25.9 | 24.5 | 68.4 | 45.5 | 31.3 | 21.4 | 19.9 | ||||||||||||||||||
2018 | [ ] | x | x | x | x | 74 | 56 | 42 | 31 | 26 | 73 | 55 | 40 | 28 | |||||||||||||||||||
2018 | [ ] | x | x | x | x | x | 63 | 42.1 | 24.2 | 14.6 | 25 | 42.3 | |||||||||||||||||||||
2018 | [ ] | x | x | x | x | 74.4 | 58.1 | 44.3 | 33.8 | 26.2 | |||||||||||||||||||||||
2018 | [ ] | x | x | x | x | IAPRTC-12 | |||||||||||||||||||||||||||
2018 | [ ] | x | x | x | x | ||||||||||||||||||||||||||||
2018 | [ ] | x | x | x | x | x | R | ||||||||||||||||||||||||||
2018 | [ ] | x | x | x | x | x | x | 50.5 | 30.8 | 19.1 | 12.1 | 60 | 17 | ||||||||||||||||||||
2018 | [ ] | x | x | x | x | x | 51 | 32.2 | 20.7 | 13.6 | 65.4 | 17 | |||||||||||||||||||||
2018 | [ ] | x | x | x | x | x | x | 66.7 | 23.8 | 77.2 | 22.4 | ||||||||||||||||||||||
2018 | [ ] | x | x | x | x | 68.8 | 51.3 | 37 | 26.5 | 83.9 | 23.4 | 60.7 | 42.5 | 29.2 | 19.9 | 39.5 | 19.1 | ||||||||||||||||
2018 | [ ] | x | x | x | 71 | 53.7 | 39.1 | 28.1 | 89 | 24.1 | |||||||||||||||||||||||
2018 | [ ] | x | x | x | 70.8 | 53.6 | 39.1 | 28.4 | 89.8 | 24.8 | 61.5 | 43.8 | 30.5 | 21.3 | 46.4 | 20 | |||||||||||||||||
2018 | [ ] | x | x | x | 39.5 | 28.2 | 90.7 | 24.3 | |||||||||||||||||||||||||
2018 | [ ] | x | x | 72 | 54.7 | 40 | 29 | 91.7 | 62.7 | 43.5 | 29.6 | 20.5 | 19.3 | ||||||||||||||||||||
2018 | [ ] | x | x | x | x | 57.1 | 30.5 | 98 | 25.4 | ||||||||||||||||||||||||
2018 | [ ] | x | x | X | x | 74.0 | 56.7 | 43.3 | 31.3 | 98.3 | 25.5 | 64.6 | 43.8 | 31.9 | 22.4 | 39.6 | 19.2 | ||||||||||||||||
2018 | [ ] | x | x | x | x | 91.6 | 83.6 | 73.4 | 62.5 | 99 | 34.8 | ||||||||||||||||||||||
2018 | [ ] | x | x | x | x | 30.2 | 101.8 | 25.8 | |||||||||||||||||||||||||
2018 | [ ] | x | x | x | x | 34 | 103.6 | 26.3 | |||||||||||||||||||||||||
2018 | [ ] | x | x | x | x | x | 74.2 | 57.7 | 43.8 | 33 | 105.8 | 66.3 | 48.1 | 34.4 | 24.5 | 52.9 | |||||||||||||||||
2018 | [ ] | x | x | x | x | x | 44.3 | 34.5 | 105.9 | 26.5 | 33.4 | 24 | 44.2 | 19 | |||||||||||||||||||
2018 | [ ] | x | x | x | x | 44.3 | 34 | 106.0 | 26.3 | ||||||||||||||||||||||||
2018 | [ ] | x | x | x | x | 73 | 56.9 | 43.6 | 33.3 | 108.1 | |||||||||||||||||||||||
2018 | [ ] | x | x | x | 75 | 34.6 | 108.2 | 26.9 | |||||||||||||||||||||||||
2018 | [ ] | x | x | x | x | x | 76.1 | 58.1 | 44.9 | 34.9 | 109.1 | 26.7 | 73.1 | 54 | 38.6 | 27.9 | 59.4 | 21.7 | |||||||||||||||
2018 | [ ] | x | x | x | x | x | x | 76.4 | 60.4 | 47.9 | 37 | 112.5 | 27.4 | ||||||||||||||||||||
2018 | [ ] | X | X | X | X | X | x | 79.4 | 63.1 | 48.2 | 36.1 | 119.6 | 28.1 | 72.1 | 48.7 | 36.9 | 21.2 | 53.6 | 20.5 | ||||||||||||||
2018 | [ ] | x | x | x | x | 38.3 | 123.2 | 28.6 | |||||||||||||||||||||||||
2018 | [ ] | x | x | x | x | 38.1 | 126.1 | 28.3 | |||||||||||||||||||||||||
2019 | [ ] | x | x | x | x | 71.9 | 52.9 | 38.7 | 28.4 | 24.3 | 69.4 | 45.7 | 33.2 | 22.6 | 23 | ||||||||||||||||||
2019 | [ ] | x | x | x | x | 21.9 | |||||||||||||||||||||||||||
2019 | [ ] | x | x | x | x | 12.6 | |||||||||||||||||||||||||||
2019 | [ ] | x | x | x | x | 67.1 | 48.8 | 34.3 | 23.9 | 73.3 | 21.8 | ||||||||||||||||||||||
2019 | [ ] | x | x | x | x | x | x | 66.6 | 48.9 | 35.5 | 25.8 | 82.1 | 23.1 | 61.5 | 42.1 | 28.6 | 19.3 | 39.9 | 18.2 | ||||||||||||||
2019 | [ ] | x | x | x | x | 70.6 | 53.4 | 39.5 | 29.2 | 88.1 | 23.7 | ||||||||||||||||||||||
2019 | [ ] | x | x | x | x | x | 69.5 | 52.1 | 38.6 | 28.7 | 91.9 | 24.1 | 66.6 | 48.4 | 34.6 | 24.7 | 52.4 | 20.2 | |||||||||||||||
2019 | [ ] | x | x | x | x | x | 72.1 | 55.1 | 41.5 | 31.4 | 95.6 | 24.7 | |||||||||||||||||||||
2019 | [ ] | x | x | x | x | 73.4 | 56.6 | 42.8 | 32.2 | 99.9 | 25.4 | 65.2 | 47.1 | 33.6 | 23.9 | 19.9 | |||||||||||||||||
2019 | [ ] | x | x | x | x | x | 73.9 | 57.1 | 43.3 | 33 | 101.6 | 26 | 66.1 | 47.2 | 33.4 | 23.2 | 19.4 | ||||||||||||||||
2019 | [ ] | x | x | x | 73.5 | 56.9 | 43.3 | 32.9 | 103.3 | 25.4 | 67.1 | 48.7 | 34.9 | 23.9 | 53.3 | 20.1 | |||||||||||||||||
2019 | [ ] | x | x | x | 73.1 | 54.9 | 40.5 | 29.9 | 107.2 | 25.6 | |||||||||||||||||||||||
2019 | [ ] | x | x | x | x | x | x | x | 55 | 110.1 | 26.1 | ||||||||||||||||||||||
2019 | [ ] | x | x | x | 75.8 | 59.6 | 46 | 35.6 | 110.5 | 27.3 | |||||||||||||||||||||||
2019 | [ ] | x | x | x | x | x | x | 79.2 | 63.2 | 48.3 | 36.3 | 120.2 | 27.6 | ||||||||||||||||||||
2019 | [ ] | x | x | x | x | x | x | 79.9 | 37.5 | 125.6 | 28.5 | 73.8 | 55.1 | 40.3 | 29.4 | 66.6 | 23 | ||||||||||||||||
2019 | [ ] | x | x | x | x | x | x | 38.6 | 28.3 | 126.3 |
- Kiros, R.; Salakhutdinov, R.; Zemel, R. Multimodal neural language models. In Proceedings of the International Conference on Machine Learning, Beijing, China, 21–26 June 2014; pp. 595–603. [ Google Scholar ]
- Vinyals, O.; Toshev, A.; Bengio, S.; Erhan, D. Show and tell: A neural image caption generator. In Proceedings of the IEEE Conference on Computer Vision and Pattern Recognition, Boston, MA, USA, 7–12 June 2015; pp. 3156–3164. [ Google Scholar ]
- Yan, S.; Xie, Y.; Wu, F.; Smith, J.S.; Lu, W.; Zhang, B. Image captioning based on a hierarchical attention mechanism and policy gradient optimization. J. Latex Cl. Files 2015 , 14 , 8. [ Google Scholar ]
- Karpathy, A.; Li, F.-F. Deep visual-semantic alignments for generating image descriptions. In Proceedings of the IEEE Conference on Computer Vision and Pattern Recognition, Boston, MA, USA, 7–12 June 2015; Volume 39, pp. 3128–3137. [ Google Scholar ] [ CrossRef ]
- Xu, K.; Ba, J.; Kiros, R.; Cho, K.; Courville, A.; Salakhutdinov, R.; Zemel, R.; Bengio, Y. Show, attend and tell: Neural image caption generation with visual attention. In Proceedings of the 32nd International Conference on Machine Learning (ICML’15), Lille, France, 6–11 July 2015. [ Google Scholar ]
- Hossain, M.Z.; Sohel, F.; Shiratuddin, M.F.; Laga, H. A comprehensive survey of deep learning for image captioning. ACM Comput. Surv. (CSUR) 2019 , 51 , 118. [ Google Scholar ] [ CrossRef ]
- Bai, S.; An, S. A survey on automatic image caption generation. Neurocomputing 2018 , 311 , 291–304. [ Google Scholar ] [ CrossRef ]
- Shabir, S.; Arafat, S.Y. An image conveys a message: A brief survey on image description generation. In Proceedings of the 2018 1st International Conference on Power, Energy and Smart Grid (ICPESG), Azad Kashmir, Pakistan, 9–10 April 2018; pp. 1–6. [ Google Scholar ] [ CrossRef ]
- Jenisha, T.; Purushotham, S. A survey of neural network algorithms used for image annotation. IIOAB J. 2016 , 7 , 236–252. [ Google Scholar ]
- Fan, C.; Crandall, D.J. DeepDiary: Automatic caption generation for lifelogging image streams. In Proceedings of the European Conference on Computer Vision, Amsterdam, The Netherlands, 11–14 October 2016; pp. 459–473. [ Google Scholar ] [ CrossRef ]
- Wang, C.; Yang, H.; Bartz, C.; Meinel, C. Image captioning with deep bidirectional LSTMs. In Proceedings of the 24th ACM International Conference on Multimedia, Amsterdam, The Netherlands, 15–19 October 2016; pp. 988–997. [ Google Scholar ] [ CrossRef ]
- Mathews, A.P.; Xie, L.; He, X. Senticap: Generating image descriptions with sentiments. In Proceedings of the Thirtieth AAAI Conference on Artificial Intelligence, Phoenix, AZ, USA, 12–17 February 2016. [ Google Scholar ]
- Sugano, Y.; Bulling, A. Seeing with humans: Gaze-assisted neural image captioning. arXiv 2016 , arXiv:1608.05203. [ Google Scholar ]
- Venugopalan, S.; Anne Hendricks, L.; Rohrbach, M.; Mooney, R.; Darrell, T.; Saenko, K. Captioning images with diverse objects. In Proceedings of the IEEE Conference on Computer Vision and Pattern Recognition, Honolulu, HI, USA, 21–26 July 2017; pp. 5753–5761. [ Google Scholar ]
- He, X.; Shi, B.; Bai, X.; Xia, G.-S.; Zhang, Z.; Dong, W. Image caption generation with part of speech guidance. Pattern Recognit. Lett. 2017 , 119 , 229–237. [ Google Scholar ] [ CrossRef ]
- Vedantam, R.; Bengio, S.; Murphy, K.; Parikh, D.; Chechik, G. Context-aware captions from context-agnostic supervision. In Proceedings of the IEEE Conference on Computer Vision and Pattern Recognition, Honolulu, HI, USA, 21–26 July 2017; pp. 251–260. [ Google Scholar ] [ CrossRef ]
- Shetty, R.; Rohrbach, M.; Anne Hendricks, L.; Fritz, M.; Schiele, B. Speaking the same language: Matching machine to human captions by adversarial training. In Proceedings of the IEEE International Conference on Computer Vision, Venice, Italy, 22–29 October 2017; pp. 4135–4144. [ Google Scholar ]
- Xin, M.; Zhang, H.; Yuan, D.; Sun, M. Learning discriminative action and context representations for action recognition in still images. In Proceedings of the IEEE International Conference on Multimedia and Expo (ICME), Hong Kong, China, 10–14 July 2017; pp. 757–762. [ Google Scholar ] [ CrossRef ]
- Yuan, A.; Li, X.; Lu, X. FFGS: Feature fusion with gating structure for image caption generation. In Proceedings of the Computer Vision, Tianjin, China, 11–14 October 2017; Springer: Singapore, 2017; pp. 638–649. [ Google Scholar ] [ CrossRef ]
- Yang, L.; Tang, K.; Yang, J.; Li, L.J. Dense captioning with joint inference and visual context. In Proceedings of the IEEE Conference on Computer Vision and Pattern Recognition, Honolulu, HI, USA, 21–26 July 2017; pp. 2193–2202. [ Google Scholar ] [ CrossRef ]
- Kilickaya, M.; Akkus, B.K.; Cakici, R.; Erdem, A.; Erdem, E.; Ikizler-Cinbis, N. Data-driven image captioning via salient region discovery. IET Comput. Vis. 2017 , 11 , 398–406. [ Google Scholar ] [ CrossRef ]
- Shah, P.; Bakrola, V.; Pati, S. Image captioning using deep neural architectures. In Proceedings of the 2017 International Conference on Innovations in Information, Embedded and Communication Systems (ICIIECS), Coimbatore, India, 17–18 March 2017; pp. 1–4. [ Google Scholar ] [ CrossRef ]
- Huang, Q.; Smolensky, P.; He, X.; Deng, L.; Wu, D. Tensor product generation networks for deep NLP modeling. In Proceedings of the Conference of the North American Chapter of the Association for Computational Linguistics: Human Language Technologies (NAACL-HLT), New Orleans, LA, USA, 1–6 June 2018; pp. 1263–1273. [ Google Scholar ] [ CrossRef ]
- Liu, C.; Sun, F.; Wang, C. MAT: A multimodal translator for image captioning. In Proceedings of the Artificial Neural Networks and Machine Learning, Pt II, Alghero, Italy, 11–14 September 2017; Lintas, A., Rovetta, S., Verschure, P., Villa, A.E.P., Eds.; Springer International Publishing Ag: Cham, Switzerland, 2017; Volume 10614, p. 784. [ Google Scholar ]
- Ren, Z.; Wang, X.; Zhang, N.; Lv, X.; Li, L.-J. Deep reinforcement learning-based image captioning with embedding reward. In Proceedings of the IEEE Conference on Computer Vision and Pattern Recognition, Honolulu, HI, USA, 21–26 July 2017; pp. 290–298. [ Google Scholar ]
- Pedersoli, M.; Lucas, T.; Schmid, C.; Verbeek, J. Areas of attention for image captioning. In Proceedings of the IEEE International Conference on Computer Vision, Venice, Italy, 22–29 October 2017; pp. 1242–1250. [ Google Scholar ]
- Fu, K.; Jin, J.; Cui, R.; Sha, F.; Zhang, C. Aligning where to see and what to tell: Image captioning with region-based attention and scene-specific contexts. IEEE Trans. Pattern Anal. Mach. Intell. 2017 , 39 , 2321–2334. [ Google Scholar ] [ CrossRef ]
- Yao, T.; Pan, Y.; Li, Y.; Qiu, Z.; Mei, T. Boosting image captioning with attributes. In Proceedings of the IEEE Conference on Computer Vision, Venice, Italy, 22–29 October 2017; pp. 4894–4902. [ Google Scholar ] [ CrossRef ]
- Vinyals, O.; Toshev, A.; Bengio, S.; Erhan, D. Show and tell: Lessons learned from the 2015 MSCOCO image captioning challenge. IEEE Trans. Pattern Anal. Mach. Intell. 2017 , 39 , 652–663. [ Google Scholar ] [ CrossRef ]
- Xu, K.; Wang, H.; Tang, P. Image captioning with deep LSTM based on sequential residual. In Proceedings of the IEEE International Conference on Multimedia and Expo (ICME), Hong Kong, China, 10–14 July 2017; pp. 361–366. [ Google Scholar ] [ CrossRef ]
- Dai, B.; Fidler, S.; Urtasun, R.; Lin, D. Towards diverse and natural image descriptions via a conditional GAN. In Proceedings of the IEEE International Conference on Computer Vision, Venice, Italy, 22–29 October 2017; pp. 2970–2979. [ Google Scholar ] [ CrossRef ]
- Dai, B.; Lin, D. Contrastive learning for image captioning. In Advances in Neural Information Processing Systems ; MIT Press: London, UK, 2017; pp. 898–907. [ Google Scholar ]
- Liu, C.; Sun, F.; Wang, C.; Wang, F.; Yuille, A. MAT: A multimodal attentive translator for image captioning. In Proceedings of the Twenty-Sixth International Joint Conference on Artificial Intelligence, Melbourne, Australia, 19–25 August 2017; pp. 4033–4039. [ Google Scholar ] [ CrossRef ]
- Lu, J.; Xiong, C.; Parikh, D.; Socher, R. Knowing when to look: Adaptive attention via a visual sentinel for image captioning. In Proceedings of the IEEE Conference on Computer Vision and Pattern Recognition, Honolulu, HI, USA, 21–26 July 2017; pp. 375–383. [ Google Scholar ]
- Nguyen, D.-K.; Okatani, T. Multi-task learning of hierarchical vision-language representation. arXiv 2018 , arXiv:1812.00500. [ Google Scholar ]
- Zhang, J.; Shih, K.; Tao, A.; Catanzaro, B.; Elgammal, A. An interpretable model for scene graph generation. arXiv 2018 , arXiv:1811.09543. [ Google Scholar ]
- Yan, S.; Wu, F.; Smith, J.S.; Lu, W.; Zhang, B. Image captioning using adversarial networks and reinforcement learning. In Proceedings of the 24th International Conference on Pattern Recognition (ICPR), Beijing, China, 20–24 August 2018; pp. 248–253. [ Google Scholar ] [ CrossRef ]
- Liu, D.; Fu, J.; Qu, Q.; Lv, J. BFGAN: Backward and forward generative adversarial networks for lexically constrained sentence generation. arXiv 2018 , arXiv:1806.08097. [ Google Scholar ]
- Wu, Y.; Zhu, L.; Jiang, L.; Yang, Y. Decoupled novel object captioner. In Proceedings of the 26th ACM International Conference on Multimedia, Seoul, Korea, 22–26 October 2018; ACM: New York, NY, USA, 2018; pp. 1029–1037. [ Google Scholar ] [ CrossRef ]
- Wang, W.; Ding, Y.; Tian, C. A novel semantic attribute-based feature for image caption generation. In Proceedings of the 2018 IEEE International Conference on Acoustics, Speech and Signal Processing (ICASSP), Calgary, AB, Canada, 15–20 April 2018; pp. 3081–3085. [ Google Scholar ] [ CrossRef ]
- Chang, Y.-S. Fine-grained attention for image caption generation. Multimed. Tools Appl. 2018 , 77 , 2959–2971. [ Google Scholar ] [ CrossRef ]
- Wu, Q.; Shen, C.; Wang, P.; Dick, A.; van den Hengel, A. Image captioning and visual question answering based on attributes and external knowledge. IEEE Trans. Pattern Anal. Mach. Intell. 2018 , 40 , 1367–1381. [ Google Scholar ] [ CrossRef ]
- Baig, M.M.A.; Shah, M.I.; Wajahat, M.A.; Zafar, N.; Arif, O. Image caption generator with novel object injection. In Proceedings of the 2018 Digital Image Computing: Techniques and Applications (DICTA), Canberra, Australia, 10–13 December 2018; pp. 1–8. [ Google Scholar ] [ CrossRef ]
- Chen, F.; Ji, R.; Sun, X.; Wu, Y.; Su, J. GroupCap: Group-based image captioning with structured relevance and diversity constraints. In Proceedings of the IEEE Conference on Computer Vision and Pattern Recognition, Salt Lake City, UT, USA, 18–22 June 2018; pp. 1345–1353. [ Google Scholar ]
- Kinghorn, P.; Zhang, L.; Shao, L. A region-based image caption generator with refined descriptions. Neurocomputing 2018 , 272 , 416–424. [ Google Scholar ] [ CrossRef ] [ Green Version ]
- Cornia, M.; Baraldi, L.; Cucchiara, R. Show, control and tell: A framework for generating controllable and grounded captions. arXiv 2018 , arXiv:1811.10652. [ Google Scholar ]
- Huang, F.; Zhang, X.; Li, Z.; Zhao, Z. Bi-directional spatial-semantic attention networks for image-text matching. IEEE Trans. Image Process. 2018 , 28 , 2008–2020. [ Google Scholar ] [ CrossRef ]
- Chen, T.; Zhang, Z.; You, Q.; Fang, C.; Wang, Z.; Jin, H.; Luo, J. “Factual” or “emotional”: Stylized image captioning with adaptive learning and attention. In Proceedings of the European Conference on Computer Vision (ECCV), Munich, Germany, 8–14 September 2018; pp. 527–543. [ Google Scholar ] [ CrossRef ]
- You, Q.; Jin, H.; Luo, J. Image captioning at will: A versatile scheme for effectively injecting sentiments into image descriptions. arXiv 2018 , arXiv:1801.10121. [ Google Scholar ]
- Mathews, A.; Xie, L.; He, X. SemStyle: Learning to generate stylised image captions using unaligned text. In Proceedings of the IEEE Conference on Computer Vision and Pattern Recognition, Salt Lake City, UT, USA, 18–22 June 2018; pp. 8591–8600. [ Google Scholar ] [ CrossRef ]
- Wang, Q.; Chan, A.B. CNN+CNN: Convolutional decoders for image captioning. arXiv 2018 , arXiv:1805.09019. [ Google Scholar ]
- Aneja, J.; Deshpande, A.; Schwing, A. Convolutional image captioning. In Proceedings of the IEEE Conference on Computer Vision and Pattern Recognition, Salt Lake City, UT, USA, 18–22 June 2018; pp. 5561–5570. [ Google Scholar ]
- Cornia, M.; Baraldi, L.; Serra, G.; Cucchiara, R. Paying more attention to saliency: Image captioning with saliency and context attention. ACM Trans. Multimed. Comput. Commun. Appl. (TOMM) 2018 , 14 , 48. [ Google Scholar ] [ CrossRef ]
- Shi, H.; Li, P. Image captioning based on deep reinforcement learning. In Proceedings of the 10th International Conference on Internet Multimedia Computing and Service, Nanjing, China, 17–19 August 2018; p. 45. [ Google Scholar ]
- Fan, Y.; Xu, J.; Sun, Y.; He, B. Long-term recurrent merge network model for image captioning. In Proceedings of the 2018 IEEE 30th International Conference on Tools with Artificial Intelligence (ICTAI), Volos, Greece, 5–7 November 2018; pp. 254–259. [ Google Scholar ] [ CrossRef ]
- Shen, K.; Kar, A.; Fidler, S. Lifelong learning for image captioning by asking natural language questions. arXiv 2018 , arXiv:1812.00235. [ Google Scholar ]
- Yu, N.; Song, B.; Yang, J.; Zhang, J. Topic-oriented image captioning based on order-embedding. IEEE Trans. Image Process. 2019 , 28 , 2743–3754. [ Google Scholar ] [ CrossRef ]
- Kim, B.; Lee, Y.H.; Jung, H.; Cho, C. Distinctive-attribute extraction for image captioning. Eur. Conf. Comput. Vis. 2018 , 133–144. [ Google Scholar ] [ CrossRef ]
- Delbrouck, J.-B.; Dupont, S. Bringing back simplicity and lightliness into neural image captioning. arXiv 2018 , arXiv:1810.06245. [ Google Scholar ]
- Sow, D.; Qin, Z.; Niasse, M.; Wan, T. A sequential guiding network with attention for image captioning. arXiv 2018 , arXiv:1811.00228. [ Google Scholar ]
- Zhu, X.; Li, L.; Liu, J.; Li, Z.; Peng, H.; Niu, X. Image captioning with triple-attention and stack parallel LSTM. Neurocomputing 2018 , 319 , 55–65. [ Google Scholar ] [ CrossRef ]
- Fang, F.; Wang, H.; Chen, Y.; Tang, P. Looking deeper and transferring attention for image captioning. Multimed. Tools Appl. 2018 , 77 , 31159–31175. [ Google Scholar ] [ CrossRef ]
- Fang, F.; Wang, H.; Tang, P. Image captioning with word level attention. In Proceedings of the 25th IEEE International Conference on Image Processing (ICIP), Athens, Greece, 7–10 October 2018. [ Google Scholar ] [ CrossRef ]
- Zhu, X.; Li, L.; Liu, J.; Peng, H.; Niu, X. Captioning transformer with stacked attention modules. Appl. Sci. 2018 , 8 , 739. [ Google Scholar ] [ CrossRef ]
- Wang, F.; Gong, X.; Huang, L. Time-dependent pre-attention model for image captioning. In Proceedings of the 24th International Conference on Pattern Recognition (ICPR), Beijing, China, 20–24 August 2018; pp. 3297–3302. [ Google Scholar ] [ CrossRef ]
- Ren, L.; Hua, K. Improved image description via embedded object structure graph and semantic feature matching. In Proceedings of the IEEE International Symposium on Multimedia (ISM), Taichung, Taiwan, 10–12 December 2018; pp. 73–80. [ Google Scholar ] [ CrossRef ]
- Jiang, W.; Ma, L.; Jiang, Y.G.; Liu, W. Recurrent fusion network for image captioning. In Proceedings of the European Conference on Computer Vision (ECCV), Munich, Germany, 8–14 September 2018; pp. 499–515. [ Google Scholar ]
- Yang, M.; Zhao, W.; Xu, W.; Feng, Y.; Zhao, Z.; Chen, X.; Lei, K. Multitask learning for cross-domain image captioning. IEEE Trans. Multimed. 2018 , 21 , 1047–1061. [ Google Scholar ] [ CrossRef ]
- Chen, C.; Mu, S.; Xiao, W.; Ye, Z.; Wu, L.; Ju, Q. Improving image captioning with conditional generative adversarial nets. arXiv 2018 , arXiv:1805.07112. [ Google Scholar ]
- Du, J.; Qin, Y.; Lu, H.; Zhang, Y. Attend more times for image captioning. arXiv 2018 , arXiv:1812.03283. [ Google Scholar ]
- Yuan, A.; Li, X.; Lu, X. 3G structure for image caption generation. Neurocomputing 2019 , 330 , 17–28. [ Google Scholar ] [ CrossRef ]
- Kim, D.-J.; Choi, J.; Oh, T.-H.; Kweon, I.S. Dense relational captioning: Triple-stream networks for relationship-based captioning. In Proceedings of the IEEE Conference on Computer Vision and Pattern Recognition (CVPR), Long Beach, CA, USA, 15–21 June 2019. [ Google Scholar ]
- Mishra, A.; Liwicki, M. Using deep object features for image descriptions. arXiv 2019 , arXiv:1902.09969. [ Google Scholar ]
- Xu, N.; Liu, A.-A.; Liu, J.; Nie, W.; Su, Y. Scene graph captioner: Image captioning based on structural visual representation. J. Vis. Commun. Image Represent. 2019 , 58 , 477–485. [ Google Scholar ] [ CrossRef ]
- Tan, Y.H.; Chan, C.S. Phrase-based image caption generator with hierarchical LSTM network. Neurocomputing 2019 , 333 , 86–100. [ Google Scholar ] [ CrossRef ]
- Tan, J.H.; Chan, C.S.; Chuah, J.H. COMIC: Towards a compact image captioning model with attention. IEEE Trans. Multimed. 2019 . [ Google Scholar ] [ CrossRef ]
- He, C.; Hu, H. Image captioning with text-based visual attention. Neural Process. Lett. 2019 , 49 , 177–185. [ Google Scholar ] [ CrossRef ]
- Li, J.; Ebrahimpour, M.K.; Moghtaderi, A.; Yu, Y.-Y. Image captioning with weakly-supervised attention penalty. arXiv 2019 , arXiv:1903.02507. [ Google Scholar ]
- He, X.; Yang, Y.; Shi, B.; Bai, X. VD-SAN: Visual-densely semantic attention network for image caption generation. Neurocomputing 2019 , 328 , 48–55. [ Google Scholar ] [ CrossRef ]
- Zhao, D.; Chang, Z.; Guo, S. A multimodal fusion approach for image captioning. Neurocomputing 2019 , 329 , 476–485. [ Google Scholar ] [ CrossRef ]
- Wang, W.; Hu, H. Image captioning using region-based attention joint with time-varying attention. Neural Process. Lett. 2019 , 1–13. [ Google Scholar ] [ CrossRef ]
- Zhou, Y.; Sun, Y.; Honavar, V. Improving image captioning by leveraging knowledge graphs. In Proceedings of the 2019 IEEE Winter Conference on Applications of Computer Vision (WACV), Waikoloa Village, HI, USA, 7–11 January 2019; pp. 283–293. [ Google Scholar ] [ CrossRef ]
- Ren, L.; Qi, G.; Hua, K. Improving diversity of image captioning through variational autoencoders and adversarial learning. In Proceedings of the 2019 IEEE Winter Conference on Applications of Computer Vision (WACV), Waikoloa Village, HI, USA, 7–11 January 2019; pp. 263–272. [ Google Scholar ] [ CrossRef ]
- Zhang, M.; Yang, Y.; Zhang, H.; Ji, Y.; Shen, H.T.; Chua, T.-S. More is better: Precise and detailed image captioning using online positive recall and missing concepts mining. IEEE Trans. Image Process. 2019 , 28 , 32–44. [ Google Scholar ] [ CrossRef ]
- Li, X.; Jiang, S. Know more say less: Image captioning based on scene graphs. IEEE Trans. Multimed. 2019 . [ Google Scholar ] [ CrossRef ]
- Gao, L.; Li, X.; Song, J.; Shen, H.T. Hierarchical LSTMs with adaptive attention for visual captioning. IEEE Trans. Pattern Anal. Mach. Intell. 2019 . [ Google Scholar ] [ CrossRef ]
- Zha, Z.J.; Liu, D.; Zhang, H.; Zhang, Y.; Wu, F. Context-aware visual policy network for fine-grained image captioning. IEEE Trans. Pattern Anal. Mach. Intell. 2019 . [ Google Scholar ] [ CrossRef ]
- KOUSTUBH. ResNet, AlexNet, VGGNet, Inception: Understanding Various Architectures of Convolutional Networks. Available online: https://cv-tricks.com/cnn/understand-resnet-alexnet-vgg-inception/ (accessed on 24 May 2019).
- He, S.; Tavakoli, H.R.; Borji, A.; Pugeault, N. A synchronized multi-modal attention-caption dataset and analysis. arXiv 2019 , arXiv:1903.02499. [ Google Scholar ]
- Plummer, B.A.; Wang, L.; Cervantes, C.M.; Caicedo, J.C.; Hockenmaier, J.; Lazebnik, S. Flickr30k entities: Collecting region-to-phrase correspondences for richer image-to-sentence models. In Proceedings of the 2015 IEEE International Conference on Computer Vision (ICCV), Santiago, Chile, 7–13 December 2015; pp. 2641–2649. [ Google Scholar ] [ CrossRef ]
- Lin, T.-Y.; Maire, M.; Belongie, S.; Hays, J.; Perona, P.; Ramanan, D.; Dollár, P.; Zitnick, C.L. Microsoft COCO: Common objects in context. In Proceedings of the Computer Vision—ECCV 2014, Zurich, Switzerland, 6–12 September 2014; Springer International Publishing: Cham, Switzerland, 2014; pp. 740–755. [ Google Scholar ] [ CrossRef ]
- Ordonez, V.; Han, X.; Kuznetsova, P.; Kulkarni, G.; Mitchell, M.; Yamaguchi, K.; Stratos, K.; Goyal, A.; Dodge, J.; Mensch, A.; et al. Large scale retrieval and generation of image descriptions. Int. J. Comput. Vis. 2016 , 119 , 46–59. [ Google Scholar ] [ CrossRef ]
- Agrawal, H.; Desai, K.; Chen, X.; Jain, R.; Batra, D.; Parikh, D.; Lee, S.; Anderson, P. Nocaps: Novel object captioning at scale. arXiv 2018 , arXiv:1812.08658. [ Google Scholar ]
- Tariq, A.; Foroosh, H. A context-driven extractive framework for generating realistic image descriptions. IEEE Trans. Image Process. 2017 , 26 , 619–632. [ Google Scholar ] [ CrossRef ]
- Zhang, H.; Shang, X.; Luan, H.; Wang, M.; Chua, T.-S. Learning from collective intelligence: Feature learning using social images and tags. ACM Trans. Multimed. Comput. Commun. Appl. (TOMM) 2017 , 13 , 1. [ Google Scholar ] [ CrossRef ]
- Sharma, S.; Suhubdy, D.; Michalski, V.; Kahou, S.E.; Bengio, Y. ChatPainter: Improving text to image generation using dialogue. arXiv 2018 , arXiv:1802.08216. [ Google Scholar ] [ CrossRef ]
Click here to enlarge figure
1Q | ArXiv | IEEE | WOS |
---|---|---|---|
Found | 29 | 9 | 18 |
Relevant | 11 | 6 (1) | 10(6) |
2Q | ArXiv | IEEE | WOS |
---|---|---|---|
Found | 48 | 19 | 78 |
Relevant | 16 (3) | 3 (6) | 12 (11) |
3Q | ArXiv | IEEE | WOS |
---|---|---|---|
Found | 26 | 28 | 42 |
Relevant | 6 (4) | 8 (6) | 2 (10) |
4Q | ArXiv | IEEE | WOS |
---|---|---|---|
Found | 38 | 20 | 25 |
Relevant | 8 (3) | 5 (3) | 7 (3) |
Image Encoder | Image Decoder | Method | MS COCO | Flickr30k | |||||||||||||||||||||||
---|---|---|---|---|---|---|---|---|---|---|---|---|---|---|---|---|---|---|---|---|---|---|---|---|---|---|---|
Year | Citation | AlexNet | VGGNet | GoogleNet | ResNet | DenseNet | LSTM | RNN | CNN | cGRU | TPGN | Encoder-Decoder | Attention | Novel objects | Semantics | Bleu-1 | Bleu-2 | Bleu-3 | Bleu-4 | CIDEr | Meteor | Bleu-1 | Bleu-2 | Bleu-3 | Bleu-4 | CIDEr | Meteor |
2016 | [ ] | x | x | x | x | 71.4 | 50.5 | 35.2 | 24.5 | 63.8 | 21.9 | ||||||||||||||||
2016 | [ ] | x | x | x | x | 50.0 | 31.2 | 20.3 | 13.1 | 61.8 | 16.8 | ||||||||||||||||
2016 | [ ] | x | x | x | x | 67.2 | 49.2 | 35.2 | 24.4 | 62.1 | 42.6 | 28.1 | 19.3 |
Image Encoder | Image Decoder | Method | MS COCO | Flickr30k | |||||||||||||||||||||||
---|---|---|---|---|---|---|---|---|---|---|---|---|---|---|---|---|---|---|---|---|---|---|---|---|---|---|---|
Year | Citation | AlexNet | VGGNet | GoogleNet | ResNet | DenseNet | LSTM | RNN | CNN | cGRU | TPGN | Encoder-Decoder | Attention | Novel Objects | Semantics | Bleu-1 | Bleu-2 | Bleu-3 | Bleu-4 | CIDEr | Meteor | Bleu-1 | Bleu-2 | Bleu-3 | Bleu-4 | CIDEr | Meteor |
2017 | [ ] | x | x | x | x | 74.2 | 58 | 43.9 | 33.2 | 108.5 | 26.6 | 67.7 | 49.4 | 35.4 | 25.1 | 53.1 | 20.4 | ||||||||||
2017 | [ ] | x | x | x | x | 73.1 | 56.7 | 42.9 | 32.3 | 105.8 | 25.8 | ||||||||||||||||
2017 | [ ] | x | x | x | x | x | x | 91 | 83.1 | 72.8 | 61.7 | 102.9 | 35 | ||||||||||||||
2017 | [ ] | x | x | x | 39.3 | 29.9 | 102 | 24.8 | 37.2 | 30.1 | 76.7 | 21.5 | |||||||||||||||
2017 | [ ] | x | x | x | 42 | 31.9 | 101.1 | 25.7 | 32.5 | 22.9 | 44.1 | 19 |
Image Encoder | Image Decoder | Method | MS COCO | Flickr30k | |||||||||||||||||||||||
---|---|---|---|---|---|---|---|---|---|---|---|---|---|---|---|---|---|---|---|---|---|---|---|---|---|---|---|
Year | Citation | AlexNet | VGGNet | GoogleNet | ResNet | DenseNet | LSTM | RNN | CNN | cGRU | TPGN | Encoder-Decoder | Attention | Novel Objects | Semantics | Bleu-1 | Bleu-2 | Bleu-3 | Bleu-4 | CIDEr | Meteor | Bleu-1 | Bleu-2 | Bleu-3 | Bleu-4 | CIDEr | Meteor |
2018 | [ ] | x | x | x | x | 38.1 | 126.1 | 28.3 | |||||||||||||||||||
2018 | [ ] | x | x | x | x | 38.3 | 123.2 | 28.6 | |||||||||||||||||||
2018 | [ ] | X | X | X | X | X | x | 79.4 | 63.1 | 48.2 | 36.1 | 119.6 | 28.1 | 72.1 | 48.7 | 36.9 | 21.2 | 53.6 | 20.5 | ||||||||
2018 | [ ] | x | x | x | x | x | x | 76.4 | 60.4 | 47.9 | 37 | 112.5 | 27.4 | ||||||||||||||
2018 | [ ] | x | x | x | x | x | 76.1 | 58.1 | 44.9 | 34.9 | 109.1 | 26.7 | 73.1 | 54 | 38.6 | 27.9 | 59.4 | 21.7 |
Image Encoder | Image Decoder | Method | MS COCO | Flickr30k | |||||||||||||||||||||||
---|---|---|---|---|---|---|---|---|---|---|---|---|---|---|---|---|---|---|---|---|---|---|---|---|---|---|---|
Year | Citation | AlexNet | VGGNet | GoogleNet | ResNet | DenseNet | LSTM | RNN | CNN | cGRU | TPGN | Encoder-Decoder | Attention | Novel Objects | Semantics | Bleu-1 | Bleu-2 | Bleu-3 | Bleu-4 | CIDEr | Meteor | Bleu-1 | Bleu-2 | Bleu-3 | Bleu-4 | CIDEr | Meteor |
2019 | [ ] | x | x | x | x | x | x | 38.6 | 28.3 | 126.3 | |||||||||||||||||
2019 | [ ] | x | x | x | x | x | x | 79.9 | 37.5 | 125.6 | 28.5 | 73.8 | 55.1 | 40.3 | 29.4 | 66.6 | 23 | ||||||||||
2019 | [ ] | x | x | x | x | x | x | 79.2 | 63.2 | 48.3 | 36.3 | 120.2 | 27.6 | ||||||||||||||
2019 | [ ] | x | x | x | 75.8 | 59.6 | 46 | 35.6 | 110.5 | 27.3 | |||||||||||||||||
2019 | [ ] | x | x | x | x | x | x | x | 55 | 110.1 | 26.1 |
Encoder/Decoder | Year | LSTM | RNN | CNN | cGRU | TPGN |
---|---|---|---|---|---|---|
2016 | [ ] | |||||
2017 | [ ] | [ ] | [ ] | |||
2018 | ||||||
2019 | ||||||
2016 | [ , , ] | |||||
2017 | [ , , , , , , , , ] | [ , ] | [ ] | |||
2018 | [ , , , , , , , , ] | [ , ] | ||||
2019 | [ , , , , , , ] | |||||
2016 | [ ] | |||||
2017 | [ , , , ] | |||||
2018 | [ , , , , ] | [ ] | ||||
2019 | [ , , ] | |||||
2016 | ||||||
2017 | [ , , , , , ] | [ ] | [ ] | |||
2018 | [ , , , , , , , , , , ] | [ , ] | ||||
2019 | [ , , , , , ] | [ ] | ||||
2016 | ||||||
2017 | ||||||
2018 | ||||||
2019 | [ ] |
Year/Method | Encoder-Decoder | Attention Mechanism | Novel Objects | Semantics |
---|---|---|---|---|
[ , , ] | [ ] | [ ] | ||
[ , , , , , , , , , , , , , ] | [ , , , , , , , ] | [ , , , ] | [ , ] | |
[ , , , , , , , , , , , , , , , , , , , , , , , , , , ] | [ , , , , , , , , , , , , , , , , , , , , , , , , ] | [ , , , , , , , , , , , ] | [ , , , , , , , , ] | |
[ , , , , , , , , , , ] | [ , , , , , , , , , , , , ] | [ , , , , , , , , , , , ] | [ , , , , , , , ] |
CONVOLUTIONAL NEURAL NETWORKS ARCHITECTURES | |||||
---|---|---|---|---|---|
Architecture | #Params | #Multiply-Adds | Top-1 Accuracy | Top-5 Accuracy | Year |
61M | 724M | 57.1 | 80.2 | 2012 | |
138M | 15.5B | 70.5 | 91.2 | 2013 | |
7M | 1.43B | 69.8 | 89.3 | 2013 | |
25.5M | 3.9B | 75.2 | 93 | 2015 |
Share and Cite
Staniūtė, R.; Šešok, D. A Systematic Literature Review on Image Captioning. Appl. Sci. 2019 , 9 , 2024. https://doi.org/10.3390/app9102024
Staniūtė R, Šešok D. A Systematic Literature Review on Image Captioning. Applied Sciences . 2019; 9(10):2024. https://doi.org/10.3390/app9102024
Staniūtė, Raimonda, and Dmitrij Šešok. 2019. "A Systematic Literature Review on Image Captioning" Applied Sciences 9, no. 10: 2024. https://doi.org/10.3390/app9102024
Article Metrics
Article access statistics, further information, mdpi initiatives, follow mdpi.
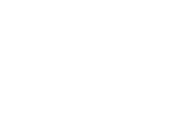
Subscribe to receive issue release notifications and newsletters from MDPI journals
arXiv's Accessibility Forum starts next month!
Help | Advanced Search
Computer Science > Computer Vision and Pattern Recognition
Title: comparative evaluation of cnn architectures for image caption generation.
Abstract: Aided by recent advances in Deep Learning, Image Caption Generation has seen tremendous progress over the last few years. Most methods use transfer learning to extract visual information, in the form of image features, with the help of pre-trained Convolutional Neural Network models followed by transformation of the visual information using a Caption Generator module to generate the output sentences. Different methods have used different Convolutional Neural Network Architectures and, to the best of our knowledge, there is no systematic study which compares the relative efficacy of different Convolutional Neural Network architectures for extracting the visual information. In this work, we have evaluated 17 different Convolutional Neural Networks on two popular Image Caption Generation frameworks: the first based on Neural Image Caption (NIC) generation model and the second based on Soft-Attention framework. We observe that model complexity of Convolutional Neural Network, as measured by number of parameters, and the accuracy of the model on Object Recognition task does not necessarily co-relate with its efficacy on feature extraction for Image Caption Generation task.
Comments: | Article Published in International Journal of Advanced Computer Science and Applications(IJACSA), Volume 11 Issue 12, 2020 |
Subjects: | Computer Vision and Pattern Recognition (cs.CV); Artificial Intelligence (cs.AI); Machine Learning (cs.LG); Multimedia (cs.MM); Neural and Evolutionary Computing (cs.NE) |
Cite as: | [cs.CV] |
(or [cs.CV] for this version) | |
Focus to learn more arXiv-issued DOI via DataCite | |
Journal reference: | in International Journal of Advanced Computer Science and Applications, 11(12), 2020 |
: | Focus to learn more DOI(s) linking to related resources |
Submission history
Access paper:.
- Other Formats

References & Citations
- Google Scholar
- Semantic Scholar
DBLP - CS Bibliography
Bibtex formatted citation.

Bibliographic and Citation Tools
Code, data and media associated with this article, recommenders and search tools.
- Institution
arXivLabs: experimental projects with community collaborators
arXivLabs is a framework that allows collaborators to develop and share new arXiv features directly on our website.
Both individuals and organizations that work with arXivLabs have embraced and accepted our values of openness, community, excellence, and user data privacy. arXiv is committed to these values and only works with partners that adhere to them.
Have an idea for a project that will add value for arXiv's community? Learn more about arXivLabs .
The CAA Captioner–Enhancing Image Captioning with Contrastive Learning and Attention on Attention Mechanism
- Conference paper
- First Online: 01 September 2024
- Cite this conference paper
- Zhao Cui 7
Part of the book series: Smart Innovation, Systems and Technologies ((SIST,volume 388))
Included in the following conference series:
- The World Conference on Intelligent and 3D Technologies
Image Captioning is a complex interdisciplinary task, situated at the intersection of CV (computer vision) and NLP (natural language processing). In recent years, with the development of encoder decoder frameworks and attention mechanisms, although significant progress has been made in this task, there is still a need to optimize the accuracy and completeness of text generation to improve the generalization ability of the model. We propose an Image Captioning method that combines a contrastive learning-enhanced ResNet101 encoder with an Attention on Attention (AoA) improved Transformer decoder to achieve better performance in generating accurate and natural descriptions. We utilize contrastive learning to enhance the model's understanding of image content. Additionally, we apply data augmentation techniques to enhance the model's generalization ability, and optimize the attention mechanism of the Transformer decoder using the AoA module. Our experimental results demonstrate the effectiveness of contrastive learning in handling complex scenes, multiple objects, and detailed descriptions, as our approach achieves superior performance on widely adopted Image Captioning datasets.
This is a preview of subscription content, log in via an institution to check access.
Access this chapter
Subscribe and save.
- Get 10 units per month
- Download Article/Chapter or eBook
- 1 Unit = 1 Article or 1 Chapter
- Cancel anytime
- Available as PDF
- Read on any device
- Instant download
- Own it forever
- Available as EPUB and PDF
- Durable hardcover edition
- Free shipping worldwide - see info
Tax calculation will be finalised at checkout
Purchases are for personal use only
Institutional subscriptions
Torralba, A., Russell, B.C., Yuen, J.: LabelMe: online image annotation and applications. P Ieee 98 (8), 1467–1484 (2010). https://doi.org/10.1109/Jproc.2010.2050290
Aydin, Z., Murray, J.I., Waterston, R.H., Noble, W.S.: Using machine learning to speed up manual image annotation: application to a 3D imaging protocol for measuring single cell gene expression in the developing C. elegans embryo. Bmc Bioinformatics, vol. 11 (2010). Artn 8410.1186/1471–2105–11–84
Google Scholar
Vinyals, O., Toshev, A., Bengio, S., Erhan, D.: Show and tell: a neural image caption generator. 2015 IEEE Conference on Computer Vision and Pattern Recognition (Cvpr), pp. 3156–3164 (2015). https://doi.org/10.1109/cvpr.2015.7298935
Xu, K. et al.: Show, attend and tell: neural image caption generation with visual attention. Pr. Mach. Learn. Res. 37 , 2048–2057 (2015)
Vaswani, A. et al.: Attention is all you need. Adv. Neur. In. 30 (2017). https://doi.org/10.48550/arXiv.1706.03762
Chen, T., Kornblith, S., Norouzi, M., Hinton, G.: A Simple Framework for Contrastive Learning of Visual Representations. arXiv (2002). https://doi.org/10.48550/arXiv.2002.05709 .
Chen, X., Xie, S., He, K.: An Empirical Study of Training Self-Supervised Vision Transformers. arXiv (2104). https://doi.org/10.48550/arXiv.2104.02057
Chen, X., Fan, H., Girshick, R., He, K.: Improved baselines with momentum contrastive learning. arXiv (2003). https://doi.org/10.48550/arXiv.2003.04297
He, K., Fan, H., Wu, Y., Xie, S., Girshick, R.: Momentum Contrast for Unsupervised Visual Representation Learning. arXiv (2020). https://doi.org/10.48550/arXiv.1911.05722
Cornia, M., Stefanini, M., Baraldi, L., Cucchiara, R.: Meshed-Memory Transformer for Image Captioning. arXiv (2020). https://doi.org/10.48550/arXiv.1912.08226
He, K.M., Zhang, X.Y., Ren, S.Q., Sun, J.: Deep residual learning for image recognition. In: Proceedings of the IEEE Conference on Computer Vision and Pattern Recognition, pp. 770–778 (2016). https://doi.org/10.1109/Cvpr.2016.90
Huang, L., Wang, W.M., Chen, J., Wei, X.Y.: Attention on attention for image captioning. In: Proceedings of the IEEE/CVF International Conference on Computer Vision, pp. 4633–4642 (2019). https://doi.org/10.1109/Iccv.2019.00473
Loshchilov, I., Hutter, F.: Decoupled Weight Decay Regularization. p. arXiv:1711.05101 . https://doi.org/10.48550/arXiv.1711.05101
Lin, T.Y., et al.: Microsoft COCO: common objects in context. Lect. Notes Comput. Sc. 8693, 740–755 (2014). https://doi.org/10.1007/978-3-319-10602-1_48
Hodosh, M., Young, P., Hockenmaier, J.: Framing image description as a ranking task: data, models and evaluation metrics. J. Artif. Intell. Res. 853–899 (2013)
Young, P., Lai, A., Hodosh, M., Hockenmaier, J.: From image descriptions to visual denotations: new similarity metrics for semantic inference over event descriptions. TACL 2 , 67–78 (2014)
Article Google Scholar
Chen, X., et al.: Microsoft COCO Captions: Data Collection and Evaluation Server. arXiv (2015). https://doi.org/10.48550/arXiv.1504.00325
Yao, T., Pan, Y., Li, Y., Qiu, Z., Mei, T.: Boosting Image Captioning with Attributes. arXiv (2016). https://doi.org/10.48550/arXiv.1611.01646
Jiang, W., Ma, L., Jiang, Y.G., Liu, W., Zhang, T.: Recurrent Fusion Network for Image Captioning. arXiv (2018). https://doi.org/10.48550/arXiv.1807.09986
Anderson, P., et al.: Bottom-Up and Top-Down Attention for Image Captioning and Visual Question Answering. arXiv (2017). https://doi.org/10.48550/arXiv.1707.07998
Yao, T., Pan, Y., Li, Y., Mei, T.: Exploring Visual Relationship for Image Captioning. arXiv (2018). https://doi.org/10.48550/arXiv.1809.07041
Liu, Z., et al.: Swin transformer: hierarchical vision transformer using shifted windows. In: 2021 Ieee/Cvf International Conference on Computer Vision (Iccv 2021), pp. 9992–10002 (2021). https://doi.org/10.1109/Iccv48922.2021.00986
Smith, S.J., et al.: Continual Diffusion: Continual Customization of Text-to-Image Diffusion with C-LoRA. arXiv (2023). https://doi.org/10.48550/arXiv.2304.06027
Download references
Author information
Authors and affiliations.
Ocean University of China, Qingdao, China
You can also search for this author in PubMed Google Scholar
Corresponding author
Correspondence to Zhao Cui .
Editor information
Editors and affiliations.
Technical University of Sofia, Sofia, Bulgaria
Roumen Kountchev (Deceased)
Interscience Institute of Management and Technology, Bhubaneswar, Odisha, India
Srikanta Patnaik
School of Electrical, Shanghai Institute of Technology, Shanghai, China
Wenfeng Wang
TK Engineering, Sofia, Bulgaria
Roumiana Kountcheva
Rights and permissions
Reprints and permissions
Copyright information
© 2024 The Author(s), under exclusive license to Springer Nature Singapore Pte Ltd.
About this paper
Cite this paper.
Cui, Z. (2024). The CAA Captioner–Enhancing Image Captioning with Contrastive Learning and Attention on Attention Mechanism. In: Kountchev (Deceased), R., Patnaik, S., Wang, W., Kountcheva, R. (eds) AI Methods and Applications in 3D Technologies. WCI3DT 2023. Smart Innovation, Systems and Technologies, vol 388. Springer, Singapore. https://doi.org/10.1007/978-981-97-2144-3_22
Download citation
DOI : https://doi.org/10.1007/978-981-97-2144-3_22
Published : 01 September 2024
Publisher Name : Springer, Singapore
Print ISBN : 978-981-97-2143-6
Online ISBN : 978-981-97-2144-3
eBook Packages : Intelligent Technologies and Robotics Intelligent Technologies and Robotics (R0)
Share this paper
Anyone you share the following link with will be able to read this content:
Sorry, a shareable link is not currently available for this article.
Provided by the Springer Nature SharedIt content-sharing initiative
- Publish with us
Policies and ethics
- Find a journal
- Track your research
IEEE Account
- Change Username/Password
- Update Address
Purchase Details
- Payment Options
- Order History
- View Purchased Documents
Profile Information
- Communications Preferences
- Profession and Education
- Technical Interests
- US & Canada: +1 800 678 4333
- Worldwide: +1 732 981 0060
- Contact & Support
- About IEEE Xplore
- Accessibility
- Terms of Use
- Nondiscrimination Policy
- Privacy & Opting Out of Cookies
A not-for-profit organization, IEEE is the world's largest technical professional organization dedicated to advancing technology for the benefit of humanity. © Copyright 2024 IEEE - All rights reserved. Use of this web site signifies your agreement to the terms and conditions.
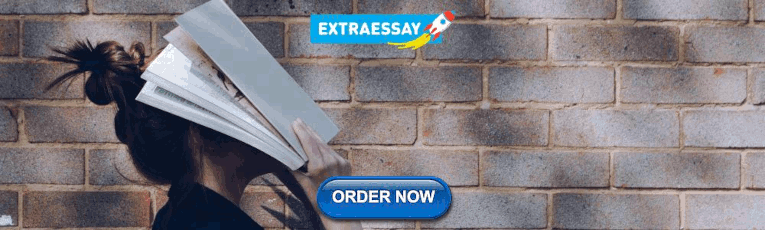
COMMENTS
The model is trained in such a way that if input image is given to model it generates captions which nearly describes the image. The accuracy of model and smoothness or command of language model learns from image descriptions is tested on different datasets. ... Date Added to IEEE Xplore: 25 April 2019 ISBN Information: Electronic ISBN: 978-1 ...
Show and tell: A neural image caption generator ... In this paper, we present a generative model based on a deep recurrent architecture that combines recent advances in computer vision and machine translation and that can be used to generate natural sentences describing an image. ... Date Added to IEEE Xplore: 15 October 2015 ISBN Information ...
Published in: 2017 International Conference on Computational Intelligence in Data Science (ICCIDS) Date of Conference: 02-03 June 2017. Date Added to IEEE Xplore: 01 February 2018. ISBN Information: DOI: 10.1109/ICCIDS.2017.8272660. Publisher: IEEE. Conference Location: Chennai, India. Authors. Figures.
An Image Caption Generator is a sophisticated AI system that combines computer vision and natural language processing to automatically create descriptive textual captions for images.
Oriol Vinyals, Alexander Toshev, Samy Bengio, Dumitru Erhan. View a PDF of the paper titled Show and Tell: A Neural Image Caption Generator, by Oriol Vinyals and 3 other authors. Automatically describing the content of an image is a fundamental problem in artificial intelligence that connects computer vision and natural language processing.
Image captioning refers to automatic generation of descriptive texts according to the visual content of images. It is a technique integrating multiple disciplines including the computer vision (CV), natural language processing (NLP) and artificial intelligence. In recent years, substantial research efforts have been devoted to generate image caption with impressive progress. To summarize the ...
Image captioning is a research area of immense importance, aiming to generate natural language descriptions for visual content in the form of still images. ... and Dumitru Erhan. 2015. Show and tell: A neural image caption generator. In Proceedings of the IEEE Conference on Computer Vision ... and Yongdong Zhang. 2020. Multi-level policy and ...
The organization of the paper is as follows: First a brief descriptions about the previous works in image captioning, which is followed by the proposed model architecture and detailed experiments and results. ... Bengio S, Erhan D. Show and tell: A neural image caption generator. In: IEEE Conference on Computer Vision and Pattern Recognition ...
Abstract. Automatically describing the content of an image is a. fundamental problem in artificial intelligence that connects. computer vision and natural language processing. In this. paper, we present a generative model based on a deep recurrent. architecture that combines recent advances in computer. vision and machine translation and that ...
Image captioning is a research area of immense importance, aiming to generate natural language descriptions for visual content in the form of still images. The advent of deep learning and more recently vision-language pre-training techniques has revolutionized the field, leading to more sophisticated methods and improved performance. In this survey paper, we provide a structured review of deep ...
This paper introduces a system that uses an attention mechanism alongside an encoder and a decoder to generate the captions. It uses a pre-trained Convolutional Neural Network (CNN) viz. Inception V3 to extract features of the image and then a Recurrent Neural Network (RNN) viz. GRU to generate a relevant caption.
"encoder", by first pre-training it for an image classification task and using the last hidden layer as an input to the RNN decoder that generates sentences (see Fig.1). We call this model the Neural Image Caption, or NIC. Our contributions are as follows. First, we present an end-to-end system for the problem. It is a neural net which
Image Caption Generator. December 2023. DOI: 10.2991/978-94-6463-314-6_35. License. CC BY-NC 4.0. In book: Proceedings of the Fourth International Conference on Advances in Computer Engineering ...
A novel semantic attribute-based feature for image caption generation. In Proceedings of the 2018 IEEE International Conference on Acoustics, Speech and Signal Processing (ICASSP), Calgary, AB, Canada, 15-20 April 2018; pp. 3081-3085. [Google Scholar] Chang, Y.-S. Fine-grained attention for image caption generation. Multimed.
Aided by recent advances in Deep Learning, Image Caption Generation has seen tremendous progress over the last few years. Most methods use transfer learning to extract visual information, in the form of image features, with the help of pre-trained Convolutional Neural Network models followed by transformation of the visual information using a Caption Generator module to generate the output ...
Image captioning is an interesting and challenging task with applications in diverse domains such as image retrieval, organizing and locating images of users' interest, etc. It has huge potential for replacing manual caption generation for images and is especially suitable for large-scale image data. Recently, deep neural network based methods have achieved great success in the field of ...
This model enables an individual to input an image and output a description for the same. The research paper makes use of the functionalities of Deep Learning and NLP (Natural Language Processing). Image Caption Generation is an important task as it allows us automate the task of generating captions for any image. This functionality enables us to easily organize files without paying heed to ...
Google's Neural Image Caption (NIC) model, proposed in 2015, is the most representative and practical achievement. This paper first introduced the encoder-decoder framework for solving the Image Caption task, using CNN as the image feature extractor and encoder, and RNN as the extractor and decoder from image information to text language.
Image Caption Generator Megha J Panicker, Vikas Upadhayay, Gunjan Sethi, Vrinda Mathur Abstract — In the modern era, image captioning has become one of the most widely required tools. Moreover, there are inbuilt applications that generate and provide a caption for a certain image, all these things are done with the help of deep neural
International Research Journal of Engineering and Technology (IRJET) e-ISSN: 2395-0056 Volume: 08 Issue: 07 | July 2021 www.irjet.net p-ISSN: 2395-0072 ... Image Caption Generator using CNN-LSTM Preksha Khant1, Vishal Deshmukh2, ... No. Authors Research Paper Publication Year Dataset and methodology Conclusions
Our proposed model shows better understanding of the image and provides us with desirable results. Published in: Article #: Date of Conference: 13-14 October 2022. Date Added to IEEE Xplore: 08 December 2022. ISBN Information: Electronic ISBN: 978-1-6654-7433-7. USB ISBN: 978-1-6654-7432-. Print on Demand (PoD) ISBN: 978-1-6654-7434-4.
This paper presents a far reaching investigation of the cutting edge models and progression in exploration that has occurred in image captioning. Key Words: Computer Vision, Natural Language Processing, Artificial Intelligence, Image Captioning, Technology. 1. INTRODUCTION Image caption generator is a mainstream research
The process of generating a textual description for images is known as image captioning. Now a days it is one of the recent and growing research problem. Day by day various solutions are being introduced for solving the problem. Even though, many solutions are already available, a lot of attention is still required for getting better and precise results. So, we came up with the idea of ...