Assignments: .
Assignments:.
Question 5 Your friend in the U.S. gives you a simple regression fit for predicting house prices from square feet. The estimated intercept is -44850 and the estimated slope is 280.76. You believe that your housing market behaves very similarly, but houses are measured in square meters. To make predictions for inputs in square meters, what intercept must you use? Hint: there are 0.092903 square meters in 1 square foot. You do not need to round your answer. (Note: the next quiz question will ask for the slope of the new model.) i dint get answer for this could any one plz help me with it
Please comment below specific week's quiz blog post. So that I can keep on updating that blog post with updated questions and answers.
This comment has been removed by the author.
Good day Akshay, I trust that you are doing well. I am struggling to pass week 2 assignment, can you please assist me. I am desperate to pass this module and I am only getting 0%... Thank you, I would really appreat your help.
Our website uses cookies to improve your experience. Learn more
Course Status : | Completed |
Course Type : | Elective |
Duration : | 12 weeks |
--> --> --> --> --> --> Category : | |
Credit Points : | 3 |
--> --> Undergraduate/Postgraduate | Start Date : | 18 Jan 2021 |
End Date : | 09 Apr 2021 |
Enrollment Ends : | 01 Feb 2021 |
Exam Date : | 25 Apr 2021 IST |
--> --> --> --> --> --> --> --> --> --> Note: This exam date is subject to change based on seat availability. You can check final exam date on your hall ticket.
Page Visits
Course layout, books and references.
- The Elements of Statistical Learning, by Trevor Hastie, Robert Tibshirani, Jerome H. Friedman (freely available online)
- Pattern Recognition and Machine Learning, by Christopher Bishop (optional)
Instructor bio
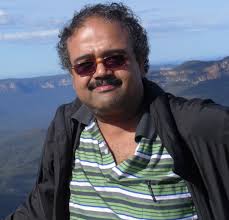
Prof. Balaraman Ravindran
Course certificate.

DOWNLOAD APP

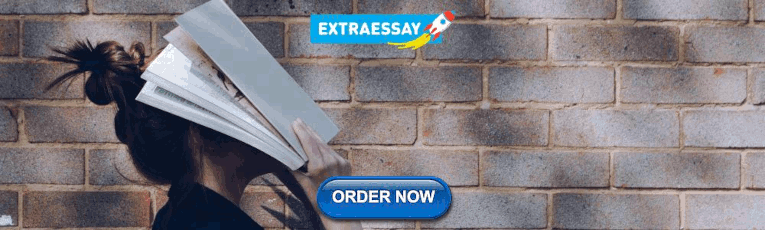
SWAYAM SUPPORT
Please choose the SWAYAM National Coordinator for support. * :
Introduction to Machine Learning Nptel Week 5 Answers
Are you looking for Introduction to Machine Learning Nptel Week 5 Answers? You’ve come to the right place! Access the latest and most accurate solutions for your Week 5 assignment in the Introduction to Machine Learning course.
Course Link: Click Here
Table of Contents
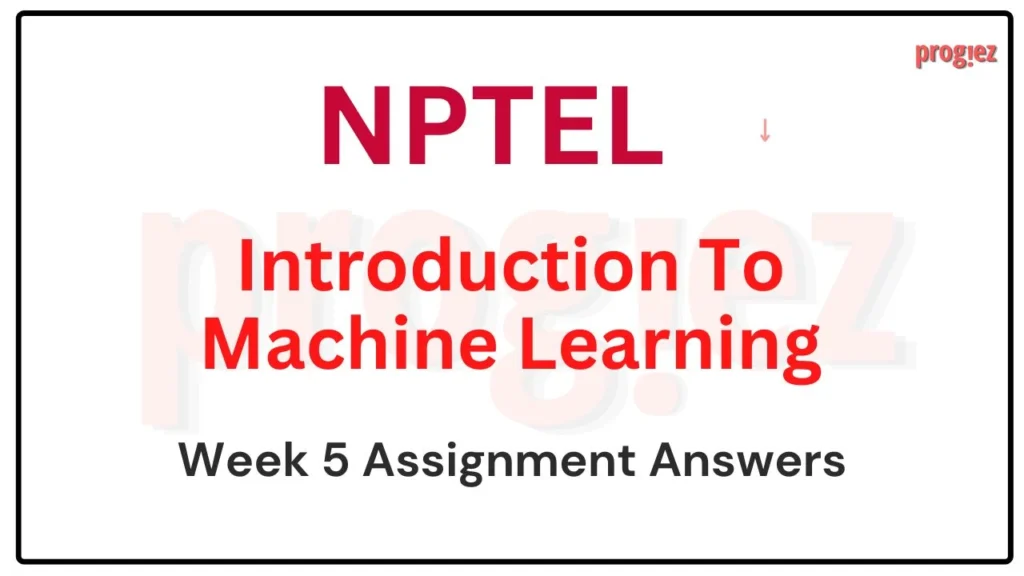
Introduction to Machine Learning Nptel Week 5 Answers (July-Dec 2024)
1. Given a 3-layer neural network which takes in 10 inputs, has 5 hidden units, and outputs 10 outputs, how many parameters are present in this network?
a) 115 b) 500 c) 25 d) 100
Answer: a) 115
2. Recall the XOR (tabulated below) example from class where we did a transformation of features to make it linearly separable. Which of the following transformations can also work?
a) Rotating x₁ and x₂ by a fixed angle. b) Adding a third dimension z = x * y c) Adding a third dimension z = x² + y² d) None of the above
Answer: c) Adding a third dimension z = x² + y²
3. We use several techniques to ensure the weights of the neural network are small (such as random initialization around 0 or regularisation). What conclusions can we draw if the weights of our ANN are high?
a) Model has overfitted. b) It was initialized incorrectly. c) At least one of (a) or (b). d) None of the above.
Answer: c) At least one of (a) or (b).
4. In a basic neural network, which of the following is generally considered a good initialization strategy for the weights?
a) Initialize all weights to zero b) Initialize all weights to a constant non-zero value (e.g., 0.5) c) Initialize weights randomly with small values close to zero d) Initialize weights with large random values (e.g., between -10 and 10)
Answer: c) Initialize weights randomly with small values close to zero
5. Which of the following is the primary reason for rescaling input features before passing them to a neural network?
a) To increase the complexity of the model b) To ensure all input features contribute equally to the initial learning process c) To reduce the number of parameters in the network d) To eliminate the need for activation functions
Answer: b) To ensure all input features contribute equally to the initial learning process
6. In the Bayesian approach to machine learning, we often use the formula: P(θ|D) = P(D|θ) * P(θ) / P(D). Where θ represents the model parameters and D represents the observed data. Which of the following correctly identifies each term in this formula?
a) P(θ|D) is the likelihood, P(D|θ) is the posterior, P(θ) is the prior, P(D) is the evidence b) P(θ|D) is the posterior, P(D|θ) is the likelihood, P(θ) is the prior, P(D) is the evidence c) P(θ|D) is the evidence, P(D|θ) is the likelihood, P(θ) is the posterior, P(D) is the prior d) P(θ|D) is the prior, P(D|θ) is the evidence, P(θ) is the likelihood, P(D) is the posterior
Answer: b) P(θ|D) is the posterior, P(D|θ) is the likelihood, P(θ) is the prior, P(D) is the evidence
7. Why do we often use log-likelihood maximization instead of directly maximizing the likelihood in statistical learning?
a) Log-likelihood provides a different optimal solution than likelihood maximization b) Log-likelihood is always faster to compute than likelihood c) Log-likelihood turns products into sums, making computations easier and more numerically stable d) Log-likelihood allows us to avoid using probability altogether
Answer: c) Log-likelihood turns products into sums, making computations easier and more numerically stable
8. In machine learning, if you have an infinite amount of data, but your prior distribution is incorrect, will you still converge to the right solution?
a) Yes, with infinite data, the influence of the prior becomes negligible, and you will converge to the true underlying solution. b) No, the incorrect prior will always affect the convergence, and you may not reach the true solution even with infinite data. c) It depends on the type of model used; some models may still converge to the right solution, while others might not. d) The convergence to the right solution is not influenced by the prior, as infinite data will always lead to the correct solution regardless of the prior.
Answer: a) Yes, with infinite data, the influence of the prior becomes negligible, and you will converge to the true underlying solution.
9. Statement: Threshold function cannot be used as activation function for hidden layers. Reason: Threshold functions do not introduce non-linearity.
a) Statement is true and reason is false. b) Statement is false and reason is true. c) Both are true and the reason explains the statement. d) Both are true and the reason does not explain the statement.
Answer: a) Statement is true and reason is false.
10. Choose the correct statement (multiple may be correct):
a) MLE is a special case of MAP when prior is a uniform distribution. b) MLE acts as regularisation for MAP. c) MLE is a special case of MAP when prior is a beta distribution. d) MAP acts as regularisation for MLE.
Answer: a) MLE is a special case of MAP when prior is a uniform distribution.
d) MAP acts as regularisation for MLE.
All weeks of Introduction to Machine Learning: Click Here
For answers to additional Nptel courses, please refer to this link: NPTEL Assignment Answers
Introduction to Machine Learning Nptel Week 5 Answers (JAN-APR 2024 )
Course name: Introduction to Machine Learning
For answers or latest updates join our telegram channel: Click here to join
Q1. Consider a feedforward neural network that performs regression on a p-dimensional input to produce a scalar output. It has m hidden layers and each of these layers has k hidden units. What is the total number of trainable parameters in the network? Ignore the bias terms. pk+mk2 pk+mk2+k pk+(m−1)k2+k p2+(m−1)pk+k p2+(m−1)pk+k2
Answer: pk+(m−1)k2+k
Q2. Consider a neural network layer defined as y=ReLU(Wx). Here x∈Rp is the input, y∈Rd is the output and W∈Rd×p is the parameter matrix. The ReLU activation (defined as ReLU(z):=max(0,z) for a scalar z) is applied element-wise to Wx. Find ∂yi∂Wij where i=1,..,d and j=1,…,p. In the following options, I (condition) is an indicator function that returns 1 if the condition is true and 0 if it is false. I(yi>0)xi I(yi>0)xj I(yi≤0)xi I(yi>0)Wijxj I(yi≤0)Wijxi
Answer: I(yi>0)xj
These are Introduction to Machine Learning Nptel Week 5 Answers
Q3. Consider a two-layered neural network y=σ(W(B)σ(W(A)x)). Let h=σ(W(A)x) denote the hidden layer representation. W(A) and W(B) are arbitrary weights. Which of the following statement(s) is/are true? Note: ∇g(f) denotes the gradient of f w.r.t g. ∇h(y) depends on W(A). ∇W(A)(y) depends on W(B). ∇W(A)(h) depends on W(B). ∇W(B)(y) depends on W(A).
Answer: B, D
Q4. Which of the following statement(s) about the initialization of neural network weights is/are true? Two different initializations of the same network could converge to different minima. For a given initialization, gradient descent will converge to the same minima irrespective of the learning rate. The weights should be initialized to a constant value. The initial values of the weights should be sampled from a probability distribution.
Answer: A, D
Q5. Consider the following statements about the derivatives of the sigmoid (σ(x)=11+exp(−x))) and tanh(tanh(x)=exp(x)−exp(−x)exp(x)+exp(−x)) activation functions. Which of these statement(s) is/are correct? 0<σ′(x)≤18 limx→−∞σ′(x)=0 0<tanh′(x)≤1 limx→+∞tanh′(x)=1
Answer: B, C
Q6. A geometric distribution is defined by the p.m.f. f(x;p)=(1−p)(x−1)p for x=1,2,….. Given the samples [4,5,6,5,4,3] drawn from this distribution, find the MLE of p. Using this estimate, find the probability of sampling x≥5 from the distribution. 0.289 0.325 0.417 0.366
Answer: 0.366
Q7. Consider a Bernoulli distribution with with p=0.7 (true value of the parameter). We draw samples from this distribution and compute an MAP estimate of p by assuming a prior distribution over p. Which of the following statement(s) is/are true?
If the prior is Beta(2,6), we will likely require fewer samples for converging to the true value than if the prior is Beta(6,2). If the prior is Beta(6,2), we will likely require fewer samples for converging to the true value than if the prior is Beta(2,6). With a prior of Beta(2,100), the estimate will never converge to the true value, regardless of the number of samples used. With a prior of U(0,0.5) (i.e. uniform distribution between 0 and 0.5), the estimate will never converge to the true value, regardless of the number of samples used.
Q8. Which of the following statement(s) about parameter estimation techniques is/are true? To obtain a distribution over the predicted values for a new data point, we need to compute an integral over the parameter space. The MAP estimate of the parameter gives a point prediction for a new data point. The MLE of a parameter gives a distribution of predicted values for a new data point. We need a point estimate of the parameter to compute a distribution of the predicted values for a new data point.
Answer: A, B
More Weeks of Introduction to Machine Learning: Click here
More Nptel Courses: https://progiez.com/nptel-assignment-answers
Introduction to Machine Learning Nptel Week 5 Answers (JULY-DEC 2023 )
Course Name: Introduction to Machine Learning
Q1. The perceptron learning algorithm is primarily designed for: Regression tasks Unsupervised learning Clustering tasks Linearly separable classification tasks Non-linear classification tasks
Answer: Linearly separable classification tasks
Q2. The last layer of ANN is linear for ________ and softmax for ________. Regression, Regression Classification, Classification Regression, Classification Classification, Regression
Answer: Regression, Classification
Q3. Consider the following statement and answer True/False with corresponding reason: The class outputs of a classification problem with a ANN cannot be treated independently. True. Due to cross-entropy loss function True. Due to softmax activation False. This is the case for regression with single output False. This is the case for regression with multiple outputs
Answer: True. Due to softmax activation
Q4. Given below is a simple ANN with 2 inputs X1,X2∈{0,1} and edge weights −3,+2,+2 h={1 if x≥0 0 otherwise Which of the following logical functions does it compute? XOR NOR NAND AND
Answer: AND
Q5. Using the notations used in class, evaluate the value of the neural network with a 3-3-1 architecture (2-dimensional input with 1 node for the bias term in both the layers). The parameters are as follows α=[1 1 0.4 0.6 0.3 0.5] β=[0.4 0.6 0.9] Using sigmoid function as the activation functions at both the layers, the output of the network for an input of (0.8, 0.7) will be (up to 4 decimal places) 0.7275 0.0217 0.2958 0.8213 0.7291 0.8414 0.1760 0.7552 0.9442 None of these
Answer: 0.8414
Q6. If the step size in gradient descent is too large, what can happen? Overfitting The model will not converge We can reach maxima instead of minima None of the above
Answer: The model will not converge
Q7. On different initializations of your neural network, you get significantly different values of loss. What could be the reason for this? Overfitting Some problem in the architecture Incorrect activation function Multiple local minima
Answer: Multiple local minima
Q8. The likelihood L(θ|X) is given by: P(θ|X) P(X|θ) P(X).P(θ) P(θ)/P(X)
Answer: P(X|θ)
Q9. Why is proper initialization of neural network weights important? To ensure faster convergence during training To prevent overfitting To increase the model’s capacity Initialization doesn’t significantly affect network performance To minimize the number of layers in the network
Answer: To ensure faster convergence during training
Q10. Which of these are limitations of the backpropagation algorithm? It requires error function to be differentiable It requires activation function to be differentiable The ith layer cannot be updated before the update of layer i+1 is complete All of the above (a) and (b) only None of these
Answer: All of the above
More Weeks of INTRODUCTION TO MACHINE LEARNING: Click here
More Nptel Courses: Click here
Introduction to Machine Learning Nptel Week 5 Answers (JAN-APR 2023 )
Q1. You are given the N samples of input (x) and output (y) as shown in the figure below. What will be the most appropriate model y=f(x)?
a. y=wx˙withw>0 b. y=wx˙withw<0 c. y=xwwithw>0 d. y=xwwithw<0
Answer: c. y=xwwithw>0
Q2. For training a binary classification model with five independent variables, you choose to use neural networks. You apply one hidden layer with three neurons. What are the number of parameters to be estimated? (Consider the bias term as a parameter) a. 16 b. 21 c. 34 = 81 d. 43 = 64 e. 12 f. 22 g. 25 h. 26 i. 4 j. None of these
Answer: f. 22
Q3. Suppose the marks obtained by randomly sampled students follow a normal distribution with unknown μ. A random sample of 5 marks are 25, 55, 64, 7 and 99. Using the given samples find the maximum likelihood estimate for the mean. a. 54.2 b. 67.75 c. 50 d. Information not sufficient for estimation
Answer: c. 50
Q4. You are given the following neural networks which take two binary valued inputs x1,x2∈{0,1} and the activation function is the threshold function(h(x)=1 if x>0; 0 otherwise). Which of the following logical functions does it compute?
a. OR b. AND c. NAND d. None of the above.
Answer: a. OR
Q5. Using the notations used in class, evaluate the value of the neural network with a 3-3-1 archi- tecture (2-dimensional input with 1 node for the bias term in both the layers). The parameters are as follows α=[1−10.20.80.40.5] β=[0.80.40.5] Using sigmoid function as the activation functions at both the layers, the output of the network for an input of (0.8, 0.7) will be a. 0.6710 b. 0.9617 c. 0.6948 d. 0.7052 e. 0.2023 f. 0.7977 g. 0.2446 h. None of these
Answer: f. 0.7977
Q6. Which of the following statements are true: a. The chances of overfitting decreases with increasing the number of hidden nodes and increasing the number of hidden layers. b. A neural network with one hidden layer can represent any Boolean function given sufficient number of hidden units and appropriate activation functions. c. Two hidden layer neural networks can represent any continuous functions (within a tolerance) as long as the number of hidden units is sufficient and appropriate activation functions used.
Answer: b, c
Q7. We have a function which takes a two-dimensional input x=(x1,x2) and has two parameters w=(w1,w2) given by f(x,w)=σ(σ(x1w1)w2+x2) where σ(x)=11+e−x We use backprop- agation to estimate the right parameter values. We start by setting both the parameters to 1. Assume that we are given a training point x2=1,x1=0,y=5. Given this information answer the next two questions. What is the value of ∂f∂w2? a. 0.150 b. -0.25 c. 0.125 d. 0.098 e. 0.0746 f. 0.1604 g. None of these
Answer: e. 0.0746
Q8. If the learning rate is 0.5, what will be the value of w2 after one update using backpropagation algorithm? a. 0.4197 b. -0.4197 c. 0.6881 d. -0.6881 e. 1.3119 f. -1.3119 g. 0.5625 h. -0.5625 i. None of these
Answer: e. 1.3119
Q9. Which of the following are true when comparing ANNs and SVMs? a. ANN error surface has multiple local minima while SVM error surface has only one minima b. After training, an ANN might land on a different minimum each time, when initialized with random weights during each run. c. As shown for Perceptron, there are some classes of functions that cannot be learnt by an ANN. An SVM can learn a hyperplane for any kind of distribution. d. In training, ANN’s error surface is navigated using a gradient descent technique while SVM’s error surface is navigated using convex optimization solvers.
Answer: a, b, d
Q10. Which of the following are correct? a. A perceptron will learn the underlying linearly separable boundary with finite number of training steps. b. XOR function can be modelled by a single perceptron. c. Backpropagation algorithm used while estimating parameters of neural networks actually uses gradient descent algorithm. d. The backpropagation algorithm will always converge to global optimum, which is one of the reasons for impressive performance of neural networks.
Answer: a, c
More Weeks of Introduction to Machine Learning: Click Here
Introduction to Machine Learning Nptel Week 5 Answers (JUL-DEC 2022 )
Course Name: INTRODUCTION TO MACHINE LEARNING
Link to Enroll: Click Here
Q1. If the step size in gradient descent is too large, what can happen? a. Overfitting b. The model will not converge c. We can reach maxima instead of minima d. None of the above
Answer: b. The model will not converge
Q2. Recall the XOR(tabulated below) example from class where we did a transformation of features to make it linearly separable. Which of the following transformations can also work?

a. X‘1=X21,X‘2=X22X′1=X12,X′2=X22 b. X‘1=1+X1,X‘2=1−X2X′1=1+X1,X′2=1−X2 c. X‘1=X1X2,X‘2=−X1X2X′1=X1X2,X′2=−X1X2 d. X‘1=(X1−X2)2,X‘2=(X1+X2)2X′1=(X1−X2)2,X′2=(X1+X2)2
Answer: c. X‘1=X1X2,X‘2=−X1X2X′1=X1X2,X′2=−X1X2
Q3. What is the effect of using activation function f(x)=xf(x)=x for hidden layers in an ANN? a. No effect. It’s as good as any other activation function (sigmoid, tanh etc). b. The ANN is equivalent to doing multi-output linear regression. c. Backpropagation will not work. d. We can model highly complex non-linear functions.
Answer: b. The ANN is equivalent to doing multi-output linear regression.
Q4. Which of the following functions can be used on the last layer of an ANN for classification? a. Softmax b. Sigmoid c. Tanh d. Linear
Q5. Statement: Threshold function cannot be used as activation function for hidden layers. Reason: Threshold functions do not introduce non-linearity. a. Statement is true and reason is false. b. Statement is false and reason is true. c. Both are true and the reason explains the statement. d. Both are true and the reason does not explain the statement.
Answer: a. Statement is true and reason is false.
Q6. We use several techniques to ensure the weights of the neural network are small (such as random initialization around 0 or regularisation). What conclusions can we draw if weights of our ANN are high? a. Model has overfitted. b. It was initialized incorrectly. c. At least one of (a) or (b). d. None of the above.
Answer: c. At least one of (a) or (b).
Q7. On different initializations of your neural network, you get significantly different values of loss. What could be the reason for this? a. Overfitting b. Some problem in the architecture c. Incorrect activation function d. Multiple local minima
Answer: a. Overfitting
Q8. The likelihood L(θ|X)L(θ|X) is given by: a. P(θ|X)P(θ|X) b. P(X|θ)P(X|θ) c. P(X).P(θ)P(X).P(θ) d. P(θ)P(X)P(θ)P(X)
Answer: b. P(X|θ)P(X|θ)
Q9. You are trying to estimate the probability of it raining today using maximum likelihood estimation. Given that in nn days, it rained nrnr times, what is the probability of it raining today? a. nrnnrn b. nrnr+nnrnr+n c. nnr+nnnr+n d. None of the above.
Answer: a. nrnnrn
Q10. Choose the correct statement (multiple may be correct): a. MLE is a special case of MAP when prior is a uniform distribution. b. MLE acts as regularisation for MAP. c. MLE is a special case of MAP when prior is a beta distribution . d. MAP acts as regularisation for MLE.
Answer: a, d
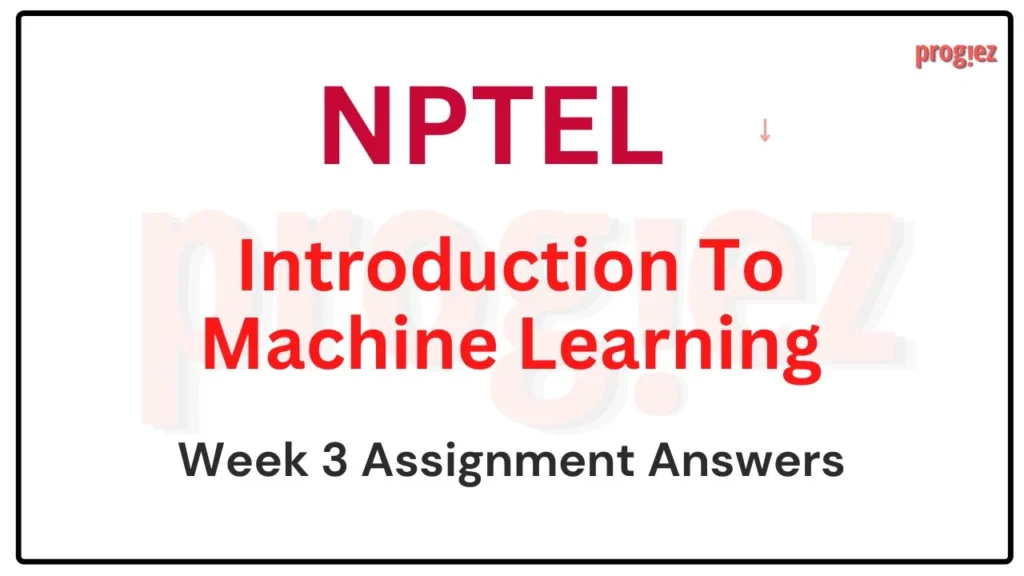
DBC Itanagar
All India News
NPTEL Introduction to Machine Learning Week 5 Assignment Answers 2024

1. Given a 3 layer neural network which takes in 10 inputs, has 5 hidden units and outputs 10 outputs, how many parameters are present in this network?
2. Recall the XOR(tabulated below) example from class where we did a transformation of features to make it linearly separable. Which of the following transformations can also work?
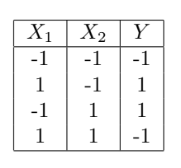
- Rotating x 1 and x 2 by a fixed angle.
- Adding a third dimension z=x∗y
- Adding a third dimension z=x 2 +y 2
- None of the above
3. We use several techniques to ensure the weights of the neural network are small (such as random initialization around 0 or regularisation). What conclusions can we draw if weights of our ANN are high?
(a) Model has overfitted. (b) It was initialized incorrectly. At least one of (a) or (b). None of the above.
4. In a basic neural network, which of the following is generally considered a good initialization strategy for the weights?
- Initialize all weights to zero
- Initialize all weights to a constant non-zero value (e.g., 0.5)
- Initialize weights randomly with small values close to zero
- Initialize weights with large random values (e.g., between -10 and 10)
5. Which of the following is the primary reason for rescaling input features before passing them to a neural network?
- To increase the complexity of the model
- To ensure all input features contribute equally to the initial learning process
- To reduce the number of parameters in the network
- To eliminate the need for activation functions
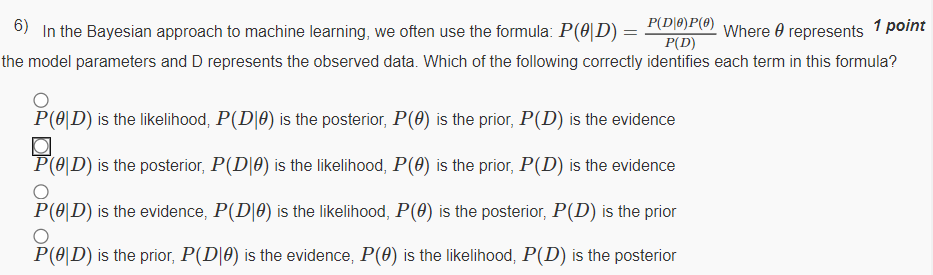
7. Why do we often use log-likelihood maximization instead of directly maximizing the likelihood in statistical learning?
- Log-likelihood provides a different optimal solution than likelihood maximization
- Log-likelihood is always faster to compute than likelihood
- Log-likelihood turns products into sums, making computations easier and more numerically stable
- Log-likelihood allows us to avoid using probability altogether
8. In machine learning, if you have an infinite amount of data, but your prior distribution is incorrect, will you still converge to the right solution?
- Yes, with infinite data, the influence of the prior becomes negligible, and you will converge to the true underlying solution.
- No, the incorrect prior will always affect the convergence, and you may not reach the true solution even with infinite data.
- It depends on the type of model used; some models may still converge to the right solution, while others might not.
- The convergence to the right solution is not influenced by the prior, as infinite data will always lead to the correct solution regardless of the prior.
9. Statement: Threshold function cannot be used as activation function for hidden layers. Reason: Threshold functions do not introduce non-linearity.
- Statement is true and reason is false.
- Statement is false and reason is true.
- Both are true and the reason explains the statement.
- Both are true and the reason does not explain the statement.
10. Choose the correct statement (multiple may be correct):
- MLE is a special case of MAP when prior is a uniform distribution.
- MLE acts as regularisation for MAP.
- MLE is a special case of MAP when prior is a beta disrubution .
- MAP acts as regularisation for MLE.

Leave a Reply Cancel reply
Your email address will not be published. Required fields are marked *
Save my name, email, and website in this browser for the next time I comment.
Latest News
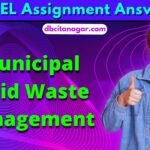
NPTEL Municipal Solid Waste Management Week 9 Assignment Answers 2024
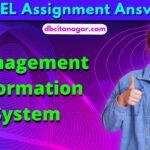
NPTEL Management Information System Week 9 Assignment Answers 2024

NPTEL Introduction to Machine Learning Week 9 Assignment Answers 2024

NPTEL Introduction to Internet of Things Week 9 Assignment Answers 2024

NPTEL Introduction to Industry 4.0 and Industrial Internet of Things Week 9 Assignment Answers 2024
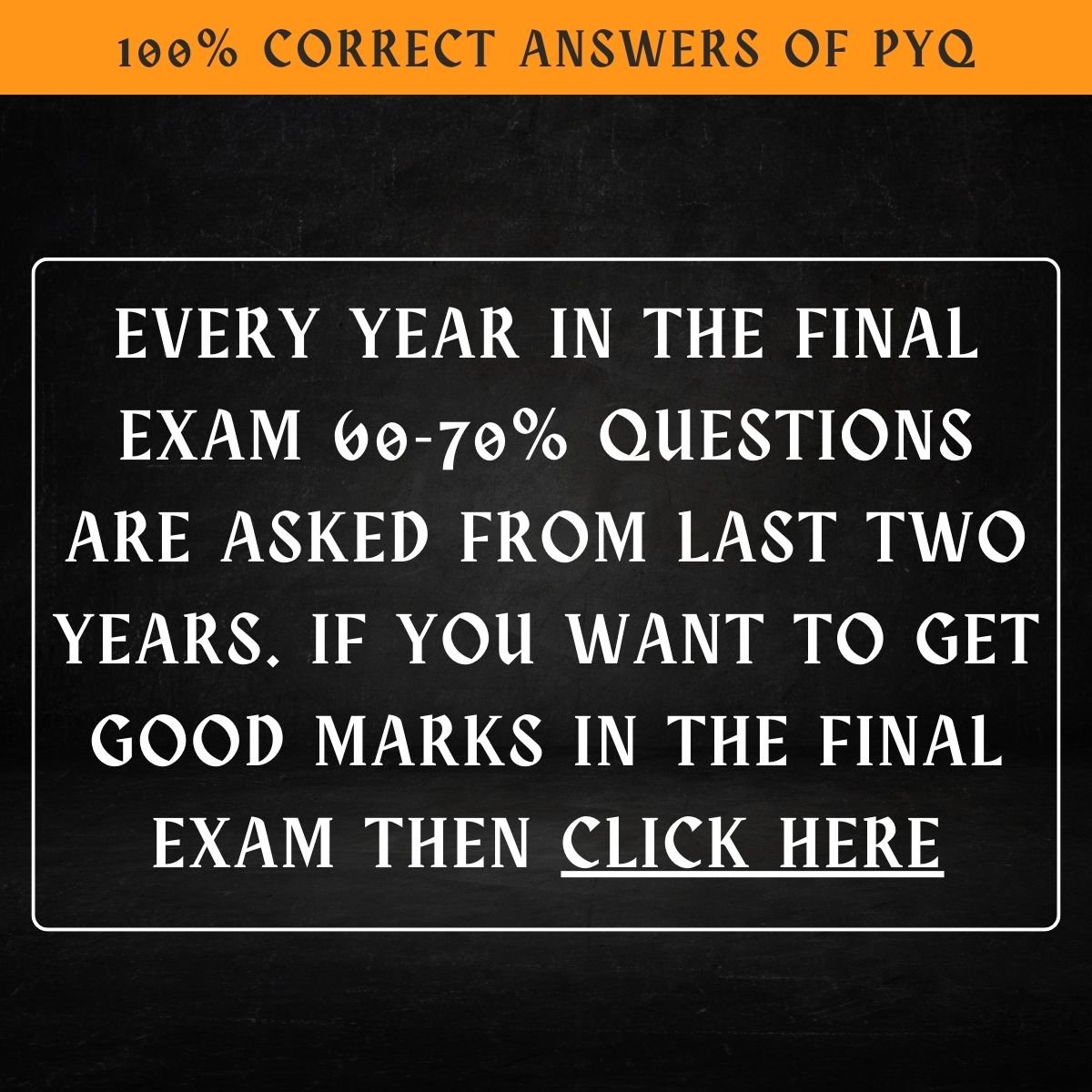
Sign in to your account
Username or Email Address
Remember Me
NPTEL Introduction To Machine Learning Week 5 Assignment Answer 2023
NPTEL Introduction To Machine Learning Week 5 Assignment Solutions
1. The perceptron learning algorithm is primarily designed for:
- Regression tasks
- Unsupervised learning
- Clustering ta s ks
- Linearly separable classification tasks
- Non-linear classification tasks
2. The last layer of ANN is linear for and softmax for .
- Regression, Regression
- Classificat i on, Clas s ification
- Regression, Classification
- Classification, Regression
3. Consider the following statement and answer True/False with corresponding reason: The class outputs of a classification problem wit h a ANN cannot be treated independently.
- True. Due to cross-entropy loss function
- True. Due to softmax activation
- False. This is the c a se for regression with single output
- False. This is the case for regression with multiple outputs
4. Given below is a simple ANN with 2 inputs X1,X2∈{0,1} and edge weights −3,+2,+2
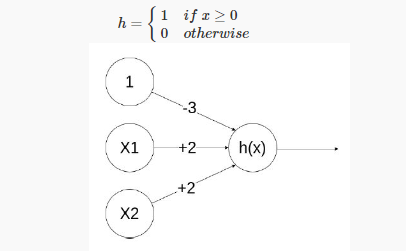
Which of the following logical functions does it compute?
5. Using the notations used in class, evaluate the value of the neural network with a 3-3-1 architecture (2-dimensional input with 1 node for the bias term in both the layers). The parameters are as follows

Using sigmoid function as the activation functions at both the layers, the output of the network for an input of (0.8, 0.7) will be (up to 4 decimal places)
6. If the step size in gradient descent is too large, what can happen?
- Overfitting
- The model will not converge
- We can reach maxi m a instead of minima
- None of the above
7. On different initializations of your neural network, you get significantly different values of loss. What could be the reason for this?
- Some problem in the architecture
- Incorrect activatio n function
- Multiple local minima
8. The likelihood L(θ|X) is given by:
9. Why is proper initialization of neural network weights important?
- To ensure faster convergence during training
- To prevent overfitting
- To increase the mod e l’s capacity
- Initialization doesn’t significantly affect network performance
- To minimize the number of layers in the network
10. Which of these are limitations of the backpropagation algorithm?
- It requires error function to be differentiable
- It requires activation function to be differentiable
- The ith layer cannot be update d before the update of layer i+1 is complete
- All of the above
- (a) and (b) only
Please Enable JavaScript in your Browser to Visit this Site.
You must be logged in to post a comment.
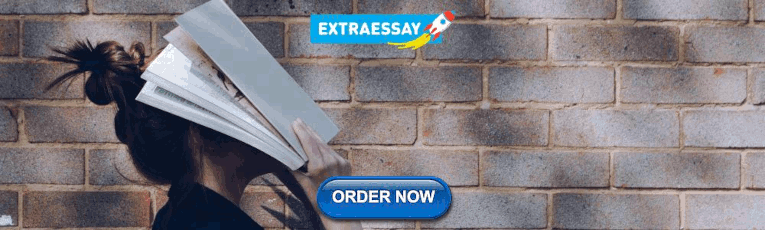
IMAGES
VIDEO
COMMENTS
Click here to check out week-4 assignment solutions, Scroll down for the solutions for week-5 assignment. In this exercise, you will implement the back-propagation algorithm for neural networks and apply it to the task of hand-written digit recognition. Before starting on the programming exercise, we strongly recommend watching the video ...
The entire code for week 5 Neural network has been uploaded here. If you're a beginner this is the way to go for you. I have tried to solve every question in multiple ways possible and have left a link on how each respective logic was built. Feel free to download and have fun. - suhasraju/Coursera-Machine-learning-Week-5-Programming-assignment
#machinelearning #nptel #swayam #python #ml All week Assignment SolutionIntroduction To Machine Learning - https://www.youtube.com/playlist?list=PL__28a0xF...
python machine-learning deep-learning neural-network solutions mooc tensorflow linear-regression coursera recommendation-system logistic-regression decision-trees unsupervised-learning andrew-ng supervised-machine-learning unsupervised-machine-learning coursera-assignment coursera-specialization andrew-ng-machine-learning
Machine Learning (Stanford University) Week 5 assignments - Abhiroyq1/Machine-Learning-Week-5-solutions
Welcome to our NPTEL course on "Machine Learning, ML." In this video, we delve into the key takeaways and answers from Week 5 of the course.Join us on this j...
updated Week 5 Intro To ML-IIT Madras:4.e6.d7.gNote:- These answers might be not 100% right, please don't copy paste blindly 🙏NPTEL Introduction to machine ...
by Akshay Daga (APDaga) - April 25, 2021. 4. The complete week-wise solutions for all the assignments and quizzes for the course "Coursera: Machine Learning by Andrew NG" is given below: Recommended Machine Learning Courses: Coursera: Machine Learning. Coursera: Deep Learning Specialization.
scikit-learn #. One of the most prominent Python libraries for machine learning: Contains many state-of-the-art machine learning algorithms. Builds on numpy (fast), implements advanced techniques. Wide range of evaluation measures and techniques. Offers comprehensive documentation about each algorithm.
These are Introduction To Machine Learning IIT-KGP Week 5 Answers. Q3.In logistic regression, we learn the conditional distribution p (y|x), where y is the class label and x is a data point. If h (x) is the output of the logistic regression classifier for an input x, then p (y|x) equals: Answer A. Answer: B. [0.1]
In this course we intend to introduce some of the basic concepts of machine learning from a mathematically well motivated perspective. ... We will assume that the students know programming for some of the assignments.If the students have done introductory courses on probability theory and linear ... Week 5: Neural Networks - Introduction ...
By the end of this Specialization, you will have mastered key concepts and gained the practical know-how to quickly and powerfully apply machine learning to challenging real-world problems. If you're looking to break into AI or build a career in machine learning, the new Machine Learning Specialization is the best place to start.
These are Introduction to Machine Learning Nptel Week 5 Answers. Q10. Choose the correct statement (multiple may be correct): a. MLE is a special case of MAP when prior is a uniform distribution. b. MLE acts as regularisation for MAP. c. MLE is a special case of MAP when prior is a beta distribution . d.
Introduction to Machine Learning Week 5 | NPTEL | Solution July 2023 | By IIT MadrasIntroduction To Machine LearningABOUT THE COURSE :With the increased avai...
NPTEL Introduction to Machine Learning Week 5 Assignment Answers 2024. 1. Given a 3 layer neural network which takes in 10 inputs, has 5 hidden units and outputs 10 outputs, how many parameters are present in this network? 2. Recall the XOR (tabulated below) example from class where we did a transformation of features to make it linearly separable.
Quiz Answers, Assessments, Programming Assignments for the Linear Algebra course. - Coursera-Imperial-College-London-Mathematics-For-Machine-Learning-Linear-Algebra/All Assessments and Programming Assignments/Week 5 (Eigenvalues and Eigenvectors)/Page Rank.py at master · prestonsn/Coursera-Imperial-College-London-Mathematics-For-Machine-Learning-Linear-Algebra
Welcome to our NPTEL course on " Introduction to Machine Learning." In this video, we delve into the key takeaways and answers from Week 5 of the course.Join...
Assignment 5 (Sol.) Introduction to Machine Learning Prof. B. Ravindran. You are given the following neural networks which take two binary valued inputsx 1 , x 2 ∈ { 0 , 1 } and the activation function is the threshold function(h(x) = 1 ifx >0; 0 otherwise).
Answer :- For Answer Click Here. 5. Using the notations used in class, evaluate the value of the neural network with a 3-3-1 architecture (2-dimensional input with 1 node for the bias term in both the layers). The parameters are as follows. Using sigmoid function as the activation functions at both the layers, the output of the network for an ...
Here's a full videos Solution of the NPTEL Swayam Introduction To Machine Learning- IITKGP Week 5 Assignment 5 answers.#nptelassignmentsolution #nptel2022 #s...
Inside the course folder, you will find files named week-01.md, week-02.md, and so on up to week-12.md. These files contain the assignment answers for each respective week. Select the Week File: Click on the file corresponding to the week you are interested in. For example, if you need answers for Week 3, open the week-03.md file. Review the ...
Contains Optional Labs and Solutions of Programming Assignment for the Machine Learning Specialization By Stanford University and Deeplearning.ai - Coursera (2023) by Prof. Andrew NG - Coursera-Machine-Learning-Specialization/Course 2 - Advanced Learning Algorithms/Course 2 - Week 3/Practice Labs/C2_W3_Assignment.ipynb at main · VuBacktracking ...
Introduction To Machine Learning - IITKGP Week 5 : Assignment 5 Answers || July-2023 NPTEL1.https://youtu.be/48wdjrD81H02. Join telegram Channel -- https://t...