Thank you for visiting nature.com. You are using a browser version with limited support for CSS. To obtain the best experience, we recommend you use a more up to date browser (or turn off compatibility mode in Internet Explorer). In the meantime, to ensure continued support, we are displaying the site without styles and JavaScript.
- View all journals
- Explore content
- About the journal
- Publish with us
- Sign up for alerts
- Review Article
- Published: 12 March 2024
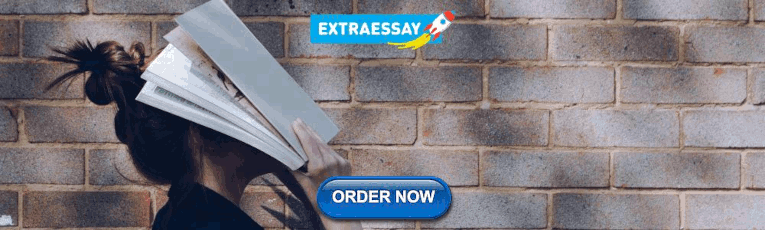
Deep learning for water quality
- Wei Zhi 1 , 2 ,
- Alison P. Appling ORCID: orcid.org/0000-0003-3638-8572 3 ,
- Heather E. Golden ORCID: orcid.org/0000-0001-5501-9444 4 ,
- Joel Podgorski ORCID: orcid.org/0000-0003-2522-1021 5 &
- Li Li ORCID: orcid.org/0000-0002-1641-3710 2
Nature Water volume 2 , pages 228–241 ( 2024 ) Cite this article
6041 Accesses
6 Citations
49 Altmetric
Metrics details
- Environmental sciences
Understanding and predicting the quality of inland waters are challenging, particularly in the context of intensifying climate extremes expected in the future. These challenges arise partly due to complex processes that regulate water quality, and arduous and expensive data collection that exacerbate the issue of data scarcity. Traditional process-based and statistical models often fall short in predicting water quality. In this Review, we posit that deep learning represents an underutilized yet promising approach that can unravel intricate structures and relationships in high-dimensional data. We demonstrate that deep learning methods can help address data scarcity by filling temporal and spatial gaps and aid in formulating and testing hypotheses via identifying influential drivers of water quality. This Review highlights the strengths and limitations of deep learning methods relative to traditional approaches, and underscores its potential as an emerging and indispensable approach in overcoming challenges and discovering new knowledge in water-quality sciences.
This is a preview of subscription content, access via your institution
Access options
Subscribe to this journal
Receive 12 digital issues and online access to articles
111,21 € per year
only 9,27 € per issue
Buy this article
- Purchase on SpringerLink
- Instant access to full article PDF
Prices may be subject to local taxes which are calculated during checkout
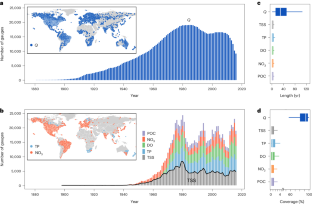
Similar content being viewed by others
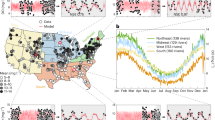
Temperature outweighs light and flow as the predominant driver of dissolved oxygen in US rivers
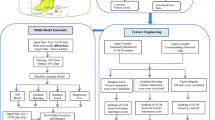
Intercomparison of deep learning models in predicting streamflow patterns: insight from CMIP6
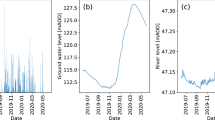
Machine learning approach towards explaining water quality dynamics in an urbanised river
Data availability.
Streamflow data (Fig. 1a ) from the Global Streamflow Indices and Metadata Archive (GSIM) were compiled from repositories at https://doi.org/10.1594/PANGAEA.887477 and https://doi.org/10.1594/PANGAEA.887470 . Water-quality data (Fig. 1b ) from the Global River Water Quality Archive (GRQA) were downloaded from https://doi.org/10.5281/zenodo.7056647 .
Turing, A. M. Computing machinery and intelligence. Mind 59 , 433–460 (1950).
Article Google Scholar
Turing, A. M. On computable numbers, with an application to the Entscheidungsproblem. Proc. Lond. Math. Soc. s2-42 , 230–265 (1937).
Muggleton, S. Alan Turing and the development of artificial intelligence. AI Commun. 27 , 3–10 (2014).
Dechter, R. Learning while searching in constraint-satisfaction problems. In Proceedings of the AAAI Conference on Artificial Intelligence Vol. 5, 178–183 (AAAI, 1986).
Hinton, G. E. & Salakhutdinov, R. R. Reducing the dimensionality of data with neural networks. Science 313 , 504–507 (2006).
Article CAS PubMed Google Scholar
Hinton, G. E., Osindero, S. & Teh, Y.-W. A fast learning algorithm for deep belief nets. Neural Comput. 18 , 1527–1554 (2006).
Article PubMed Google Scholar
Lecun, Y., Bengio, Y. & Hinton, G. Deep learning. Nature 521 , 436–444 (2015).
Reichstein, M. et al. Deep learning and process understanding for data-driven Earth system science. Nature 566 , 195–204 (2019).
Shen, C. A transdisciplinary review of deep learning research and its relevance for water resources scientists. Water Resour. Res. 54 , 8558–8593 (2018).
Shen, C. P. et al. HESS opinions: incubating deep-learning-powered hydrologic science advances as a community. Hydrol. Earth Syst. Sci. 22 , 5639–5656 (2018).
Xu, T. & Liang, F. Machine learning for hydrologic sciences: an introductory overview. Wiley Interdiscip. Rev. Water 8 , e1533 (2021).
Zhi, W. et al. From hydrometeorology to river water quality: can a deep learning model predict dissolved oxygen at the continental scale? Environ. Sci. Technol. 55 , 2357–2368 (2021).
Varadharajan, C. et al. Can machine learning accelerate process understanding and decision‐relevant predictions of river water quality? Hydrol. Process. https://doi.org/10.1002/hyp.14565 (2022).
Tripathy, K. P. & Mishra, A. K. Deep learning in hydrology and water resources disciplines: concepts, methods, applications, and research directions. J. Hydrol. https://doi.org/10.1016/j.jhydrol.2023.130458 (2023).
Perry, G. L. W., Seidl, R., Bellvé, A. M. & Rammer, W. An outlook for deep learning in ecosystem science. Ecosystems 25 , 1700–1718 (2022).
Song, T. et al. A review of artificial intelligence in marine science. Front. Earth Sci. https://doi.org/10.3389/feart.2023.1090185 (2023).
Sun, A. Y. & Scanlon, B. R. How can big data and machine learning benefit environment and water management: a survey of methods, applications, and future directions. Environ. Res. Lett. 14 , 073001 (2019).
Zhu, J.-J., Yang, M. & Ren, Z. J. Machine learning in environmental research: common pitfalls and best practices. Environ. Sci. Technol. 57 , 17671–17689 (2023).
van Klompenburg, T., Kassahun, A. & Catal, C. Crop yield prediction using machine learning: a systematic literature review. Comput. Electron. Agric. 177 , 105709 (2020).
Zhong, S. et al. Machine learning: new ideas and tools in environmental science and engineering. Environ. Sci. Technol. https://doi.org/10.1021/acs.est.1c01339 (2021).
Appling, A. P., Oliver, S. K., Read, J. S., Sadler, J. M. & Zwart, J. A. in Encyclopedia of Inland Waters 2nd edn (eds Mehner, T. & Tockner, K.) 585–606 (Elsevier, 2022).
Shen, C. et al. Differentiable modelling to unify machine learning and physical models for geosciences. Nat. Rev. Earth Environ. 4 , 552–567 (2023).
Diamond, J. S. et al. Hypoxia is common in temperate headwaters and driven by hydrological extremes. Ecol. Indic. 147 , 109987 (2023).
Article CAS Google Scholar
Creed, I. F. et al. Enhancing protection for vulnerable waters. Nat. Geosci. 10 , 809–815 (2017).
Article CAS PubMed PubMed Central Google Scholar
Nazari-Sharabian, M., Ahmad, S. & Karakouzian, M. Climate change and eutrophication: a short review. Eng. Technol. Appl. Sci. Res. 8 , 3668 (2018).
Paerl, H. W., Otten, T. G. & Kudela, R. Mitigating the expansion of harmful algal blooms across the freshwater-to-marine continuum. Environ. Sci. Technol. 52 , 5519–5529 (2018).
McCabe, M. F. et al. The future of Earth observation in hydrology. Hydrol. Earth Syst. Sci. 21 , 3879–3914 (2017).
Article PubMed PubMed Central Google Scholar
Abbott, B. W. et al. Using multi-tracer inference to move beyond single-catchment ecohydrology. Earth Sci. Rev. 160 , 19–42 (2016).
Milly, P. C. D. et al. Stationarity is dead: whither water management? Science 319 , 573–574 (2008).
Runkel, R. L., Crawford, C. G. & Cohn, T. A. Load Estimator (LOADEST): A FORTRAN Program for Estimating Constituent Loads in Streams and Rivers Report no. 4-A5 (USGS, 2004).
Hirsch, R. M., Moyer, D. L. & Archfield, S. A. Weighted regressions on time, discharge, and season (WRTDS), with an application to Chesapeake Bay River inputs. J. Am. Water Resour. Assoc. 46 , 857–880 (2010).
Zhang, Q., Blomquist, J. D., Moyer, D. L. & Chanat, J. G. Estimation bias in water-quality constituent concentrations and fluxes: a synthesis for Chesapeake Bay rivers and streams. Front. Ecol. Evol. https://doi.org/10.3389/fevo.2019.00109 (2019).
Zhi, W. et al. Distinct source water chemistry shapes contrasting concentration–discharge patterns. Water Resour. Res. 55 , 4233–4251 (2019).
Archfield, S. A. et al. Accelerating advances in continental domain hydrologic modeling. Water Resour. Res. 51 , 10078–10091 (2015).
Zhi, W. et al. BioRT-Flux-PIHM v1.0: a watershed biogeochemical reactive transport model. Geosci. Model Dev. 15 , 19 (2022).
Rahmani, F. et al. Exploring the exceptional performance of a deep learning stream temperature model and the value of streamflow data. Environ. Res. Lett. https://doi.org/10.1088/1748-9326/abd501 (2021).
Bolton, T. & Zanna, L. Applications of deep learning to ocean data inference and subgrid parameterization. J. Adv. Model. Earth Syst. 11 , 376–399 (2019).
Rasp, S., Pritchard, M. S. & Gentine, P. Deep learning to represent subgrid processes in climate models. Proc. Natl Acad. Sci. USA 115 , 9684–9689 (2018).
Irrgang, C. et al. Towards neural Earth system modelling by integrating artificial intelligence in Earth system science. Nat. Mach. Intell. 3 , 667–674 (2021).
Zhi, W., Ouyang, W., Shen, C. & Li, L. Temperature outweighs light and flow as the predominant driver of dissolved oxygen in US rivers. Nat. Water 1 , 249–260 (2023).
Willard, J. D. et al. Predicting water temperature dynamics of unmonitored lakes with meta-transfer learning. Water Resour. Res. 57 , e2021WR029579 (2021).
He, Y. et al. Water clarity mapping of global lakes using a novel hybrid deep-learning-based recurrent model with Landsat OLI images. Water Res. 215 , 118241 (2022).
Jia, X. et al. Physics-guided recurrent graph model for predicting flow and temperature in river networks. In Proc. 2021 SIAM International Conference on Data Mining (SDM) 612–620 (SIAM, 2021); https://doi.org/10.1137/1.9781611976700.69
Bao, T. et al. Partial differential equation driven dynamic graph networks for predicting stream water temperature. In 2021 IEEE International Conference on Data Mining (ICDM) 11–20 (IEEE, 2021); https://doi.org/10.1109/ICDM51629.2021.00011
Bindas, T. et al. Improving river routing using a differentiable Muskingum‐Cunge model and physics‐informed machine learning. Water Resour. Res. 60 , e2023WR035337 (2024).
Chen, S. et al. Heterogeneous stream-reservoir graph networks with data assimilation. In 2021 IEEE International Conference on Data Mining (ICDM) 1024–1029 (IEEE, 2021).
Chen, S., Zwart, J. A. & Jia, X. Physics-guided graph meta learning for predicting water temperature and streamflow in stream networks. In Proc. 28th ACM SIGKDD Conference on Knowledge Discovery and Data Mining 2752–2761 (Association for Computing Machinery, 2022).
Saha, G. K., Rahmani, F., Shen, C., Li, L. & Cibin, R. A deep learning-based novel approach to generate continuous daily stream nitrate concentration for nitrate data-sparse watersheds. Sci. Total Environ. 878 , 162930 (2023).
Kratzert, F. et al. Towards learning universal, regional, and local hydrological behaviors via machine learning applied to large-sample datasets. Hydrol. Earth Syst. Sci. 23 , 5089–5110 (2019).
Willard, J. D., Read, J. S., Topp, S., Hansen, G. J. A. & Kumar, V. Daily surface temperatures for 185,549 lakes in the conterminous United States estimated using deep learning (1980–2020). Limnol. Oceanogr. Lett. https://doi.org/10.1002/lol2.10249 (2022).
Ren, H., Cromwell, E., Kravitz, B. & Chen, X. Using long short-term memory models to fill data gaps in hydrological monitoring networks. Hydrol. Earth Syst. Sci. 26 , 1727–1743 (2022).
Latif, S. D. et al. Sediment load prediction in Johor River: deep learning versus machine learning models. Appl. Water Sci. https://doi.org/10.1007/s13201-023-01874-w (2023).
Jamei, M. et al. Designing a decomposition-based multi-phase pre-processing strategy coupled with EDBi-LSTM deep learning approach for sediment load forecasting. Ecol. Indic. 153 , 110478 (2023).
Hill, P. R., Kumar, A., Temimi, M. & Bull, D. R. HABNet: machine learning, remote sensing-based detection of harmful algal blooms. IEEE J. Sel. Top. Appl. Earth Obs. Remote Sens. 13 , 3229–3239 (2020).
D’Alimonte, D., Zibordi, G. & Berthon, J. F. Determination of CDOM and NPPM absorption coefficient spectra from coastal water remote sensing reflectance. IEEE Trans. Geosci. Remote Sens. 42 , 1770–1777 (2004).
Zhang, Y. et al. Improving remote sensing estimation of Secchi disk depth for global lakes and reservoirs using machine learning methods. GIsci. Remote Sens. 59 , 1367–1383 (2022).
Li, L. et al. River water quality shaped by land–river connectivity in a changing climate. Nat. Clim. Change https://doi.org/10.1038/s41558-023-01923-x (2024).
Rasmussen, P. P., Gray, J. R., Glysson, G. D. & Ziegler, A. C. Guidelines and Procedures for Computing Time-Series Suspended-Sediment Concentrations and Loads from In-Stream Turbidity-Sensor and Streamflow Report No. 3-C4 (USGS, 2009).
Covert, S. A., Bunch, A. R., Crawford, C. G. & Oelsner, G. P. Comparison of Surrogate Models to Estimate Pesticide Concentrations at Six U.S. Geological Survey National Water Quality Network Sites during Water Years 2013–18 Report No. 2022-5109 (USGS, 2023).
Schilling, K. E., Kim, S.-W. & Jones, C. S. Use of water quality surrogates to estimate total phosphorus concentrations in Iowa rivers. J. Hydrol. Reg. Stud. 12 , 111–121 (2017).
Wang, D. et al. Satellite retrieval of surface water nutrients in the coastal regions of the East China Sea. Remote Sens. 10 , 1896 (2018).
Guo, H. et al. Performance of deep learning in mapping water quality of Lake Simcoe with long-term Landsat archive. ISPRS J. Photogramm. Remote Sens. 183 , 451–469 (2022).
Kerins, D. & Li, L. High dissolved carbon concentration in arid rocky mountain streams. Environ. Sci. Technol. 57 , 4656–4667 (2023).
Zhang, Y. et al. A hybrid model combining mode decomposition and deep learning algorithms for detecting TP in urban sewer networks. Appl. Energy 333 , 120600 (2023).
Jiang, Y., Li, C., Song, H. & Wang, W. Deep learning model based on urban multi-source data for predicting heavy metals (Cu, Zn, Ni, Cr) in industrial sewer networks. J. Hazard. Mater. https://doi.org/10.1016/j.jhazmat.2022.128732 (2022).
Li, L. et al. Expanding the role of reactive transport models in critical zone processes. Earth Sci. Rev. 165 , 280–301 (2017).
Li, L. et al. Toward catchment hydro-biogeochemical theories. WIREs Water 8 , e1495 (2021).
Bergen, K. J., Johnson, P. A., de Hoop, M. V. & Beroza, G. C. Machine learning for data-driven discovery in solid Earth geoscience. Science 363 , eaau0323 (2019).
Kolbe, T. et al. Stratification of reactivity determines nitrate removal in groundwater. Proc. Natl Acad. Sci. USA 116 , 2494–2499 (2019).
Sun, A. Y. Discovering state‐parameter mappings in subsurface models using generative adversarial networks. Geophys. Res. Lett. https://doi.org/10.1029/2018gl080404 (2018).
Jiang, P., Shuai, P., Sun, A., Mudunuru, M. K. & Chen, X. Knowledge-informed deep learning for hydrological model calibration: an application to Coal Creek Watershed in Colorado. Hydrol. Earth Syst. Sci. Discuss. 2022 , 1–31 (2022).
Google Scholar
Jiang, Z. et al. Sub3DNet1.0: a deep-learning model for regional-scale 3D subsurface structure mapping. Geosci. Model Dev. 14 , 3421–3435 (2021).
Cromwell, E. et al. Estimating watershed subsurface permeability from stream discharge data using deep neural networks. Front. Earth Sci. https://doi.org/10.3389/feart.2021.613011 (2021).
Podgorski, J. & Berg, M. Global threat of arsenic in groundwater. Science 368 , 845–850 (2020).
Podgorski, J. & Berg, M. Global analysis and prediction of fluoride in groundwater. Nat. Commun. https://doi.org/10.1038/s41467-022-31940-x (2022).
Ransom, K. M., Nolan, B. T., Stackelberg, P. E., Belitz, K. & Fram, M. S. Machine learning predictions of nitrate in groundwater used for drinking supply in the conterminous United States. Sci. Total Environ. 807 , 151065 (2022).
Nolan, B. T., Green, C. T., Juckem, P. F., Liao, L. & Reddy, J. E. Metamodeling and mapping of nitrate flux in the unsaturated zone and groundwater, Wisconsin, USA. J. Hydrol. 559 , 428–441 (2018).
Wen, T., Liu, M., Woda, J., Zheng, G. & Brantley, S. L. Detecting anomalous methane in groundwater within hydrocarbon production areas across the United States. Water Res. 200 , 117236 (2021).
Willard, J., Jia, X., Xu, S., Steinbach, M. & Kumar, V. Integrating scientific knowledge with machine learning for engineering and environmental systems. ACM Comput. Surv. 55 , 1–37 (2023).
Feng, D., Beck, H., Lawson, K. & Shen, C. The suitability of differentiable, learnable hydrologic models for ungauged regions and climate change impact assessment. Hydrol. Earth Syst. Sci. Discuss. 2022 , 1–28 (2022).
Sun, A. Y., Yoon, H., Shih, C.-Y. & Zhong, Z. Applications of physics-informed scientific machine learning in subsurface science: A survey. In Knowledge Guided Machine Learning (eds Karpatne, A. et al.) 111–132 (Chapman and Hall/CRC, 2022).
Read, J. S. et al. Process‐guided deep learning predictions of lake water temperature. Water Resour. Res. 55 , 9173–9190 (2019).
Beucler, T., Rasp, S., Pritchard, M. & Gentine, P. Achieving conservation of energy in neural network emulators for climate modeling. Preprint at https://arxiv.org/abs/1906.06622 (2019).
Daw, A. et al. Physics-Guided Architecture (PGA) of Neural Networks for Quantifying Uncertainty in Lake Temperature Modeling. In Proc. 2020 SIAM International Conference on Data Mining (SDM) 532–540 (SIAM, 2020).
Shen, C. et al. Differentiable modelling to unify machine learning and physical models for geosciences. Nat. Rev. Earth Environ. https://doi.org/10.1038/s43017-023-00450-9 (2023).
Jia, X. et al. Physics Guided RNNs for Modeling Dynamical Systems: A Case Study in Simulating Lake Temperature Profiles. In Proceedings of the 2019 SIAM International Conference on Data Mining 558–566 (SIAM, 2019).
Sadler, J. M. et al. Multi-task deep learning of daily streamflow and water temperature. Water Resour. Res. 58 , e2021WR030138 (2022).
He, Q., Barajas-Solano, D., Tartakovsky, G. & Tartakovsky, A. M. Physics-informed neural networks for multiphysics data assimilation with application to subsurface transport. Adv. Water Res. 141 , 103610 (2020).
Jiang, S., Zheng, Y. & Solomatine, D. Improving AI system awareness of geoscience knowledge: symbiotic integration of physical approaches and deep learning. Geophys. Res. Lett. https://doi.org/10.1029/2020gl088229 (2020).
Höge, M., Scheidegger, A., Baity-Jesi, M., Albert, C. & Fenicia, F. Improving hydrologic models for predictions and process understanding using neural ODEs. Hydrol. Earth Syst. Sci. 26 , 5085–5102 (2022).
Molnar, C. Interpretable Machine Learning (Lulu.com, 2020).
Samek, W., Montavon, G., Lapuschkin, S., Anders, C. J. & Muller, K.-R. Explaining deep neural networks and beyond: a review of methods and applications. Proc. IEEE 109 , 247–278 (2021).
Sundararajan, M., Taly, A. & Yan, Q. Axiomatic attribution for deep networks. In International Conference on Machine Learning 3319–3328 (ICML, 2017).
Erion, G. et al. Improving performance of deep learning models with axiomatic attribution priors and expected gradients. Nat. Mach. Intell. 3 , 620–631 (2021).
Lundberg, S. M. & Lee, S.-I. A unified approach to interpreting model predictions. Adv. Neural Inf. Process. Syst. 30 , 1–10 (2017).
Ribeiro, M. T., Singh, S. & Guestrin, C. " Why should i trust you?" Explaining the predictions of any classifier. In Proc. 22nd ACM SIGKDD International Conference on Knowledge Discovery and Data Mining 1135–1144 (ACM, 2016).
Xie, W. et al. Interpretable framework of physics‐guided neural network with attention mechanism: simulating paddy field water temperature variations. Water Resour. Res. 58 , e2021WR030493 (2022).
Liu, Y., Duffy, K., Dy, J. G. & Ganguly, A. R. Explainable deep learning for insights in El Niño and river flows. Nat. Commun. https://doi.org/10.1038/s41467-023-35968-5 (2023).
Sadayappan, K., Kerins, D., Shen, C. & Li, L. Nitrate concentrations predominantly driven by human, climate, and soil properties in US rivers. Water Res. 226 , 119295 (2022).
Topp, S. N. et al. Stream temperature prediction in a shifting environment: explaining the influence of deep learning architecture. Water Resour. Res. https://doi.org/10.1029/2022wr033880 (2023).
Lee, D. et al. Integrated explainable deep learning prediction of harmful algal blooms. Technol. Forecast. Soc. Change 185 , 122046 (2022).
Zheng, H., Liu, Y., Wan, W., Zhao, J. & Xie, G. Large-scale prediction of stream water quality using an interpretable deep learning approach. J. Environ. Manage. 331 , 117309 (2023).
Hanson, P. C. et al. Predicting lake surface water phosphorus dynamics using process-guided machine learning. Ecol. Modell. 430 , 109136 (2020).
Carpenter, S. R., Booth, E. G. & Kucharik, C. J. Extreme precipitation and phosphorus loads from two agricultural watersheds. Limnol. Oceanogr. 63 , 1221–1233 (2018).
Robinne, F.-N. et al. Scientists’ warning on extreme wildfire risks to water supply. Hydrol. Process. 35 , e14086 (2021).
Whitehead, P. G., Wilby, R. L., Battarbee, R. W., Kernan, M. & Wade, A. J. A review of the potential impacts of climate change on surface water quality. Hydrol. Sci. J. 54 , 101–123 (2009).
Wang, P. et al. Exploring the application of artificial intelligence technology for identification of water pollution characteristics and tracing the source of water quality pollutants. Sci. Total Environ. 693 , 133440 (2019).
Kontos, Y. N., Kassandros, T., Katsifarakis, K. L. & Karatzas, K. Deep Learning Modeling of Groundwater Pollution Sources. In International Conference on Engineering Applications of Neural Networks 165–177 (Springer, 2021).
Zwart, J. A. et al. Near-term forecasts of stream temperature using deep learning and data assimilation in support of management decisions. J. Am. Water Res. Assoc. 59 , 317–337 (2023).
van Lieshout, C., van Oeveren, K., van Emmerik, T. & Postma, E. Automated river plastic monitoring using deep learning and cameras. Earth Space Sci. 7 , e2019EA000960 (2020).
Beria, H., Larsen, J. R., Michelon, A., Ceperley, N. C. & Schaefli, B. HydroMix v1.0: a new Bayesian mixing framework for attributing uncertain hydrological sources. Geosci. Model Dev. 13 , 2433–2450 (2020).
Tang, Y., Reed, P. & Wagener, T. How effective and efficient are multiobjective evolutionary algorithms at hydrologic model calibration? Hydrol. Earth Syst. Sci. 10 , 289–307 (2006).
Iman, M., Arabnia, H. R. & Rasheed, K. A review of deep transfer learning and recent advancements. Technologies 11 , 40 (2023).
Qian, K., Jiang, J., Ding, Y. & Yang, S.-H. DLGEA: a deep learning guided evolutionary algorithm for water contamination source identification. Neural Comput. Appl. 33 , 11889–11903 (2021).
Chen, Z. et al. A transfer learning-based LSTM strategy for imputing large-scale consecutive missing data and its application in a water quality prediction system. J. Hydrol. 602 , 126573 (2021).
Xiang, Z., Yan, J. & Demir, I. A rainfall-runoff model with LSTM-based sequence-to-sequence learning. Water Resour. Res. 56 , e2019WR025326 (2020).
Nearing, G. S. et al. What role does hydrological science play in the age of machine learning? Water Resour. Res . https://doi.org/10.1029/2020WR028091 (2021).
Guo, D. et al. A data-based predictive model for spatiotemporal variability in stream water quality. Hydrol. Earth Syst. Sci. 24 , 827–847 (2020).
Zimmer, M. A. et al. Zero or not? Causes and consequences of zero-flow stream gage readings. WIREs Water 7 , e1436 (2020).
Chaudhary, P., D’Aronco, S., Moy de Vitry, M., Leitão, J. P. & Wegner, J. D. Flood-water level estimation from social media images. ISPRS Ann. Photogramm. Remote Sens. Spatial Inf. Sci . https://doi.org/10.5194/isprs-annals-IV-2-W5-5-2019 (2019).
Kanth, A. K., Chitra, P. & Sowmya, G. G. Deep learning-based assessment of flood severity using social media streams. Stoch. Environ. Res. Risk Assess. 36 , 473–493 (2022).
Hanif, M., Khawar, A., Tahir, M. A. & Rafi, M. Deep learning based framework for classification of water quality in social media data. In Proc. MediaEval 2021 Workshop ( MediaEval 2021).
Njue, N. et al. Citizen science in hydrological monitoring and ecosystem services management: state of the art and future prospects. Sci. Total Environ. 693 , 133531 (2019).
Yevenes, M. A., Pereira, H. & Bermudez, R. Citizen science as a co-creative measure to water quality: chemical data and local participation in a rural territory. Front. Environ. Sci. 10 , 940778 (2022).
Nardi, F. et al. Citizens and Hydrology (CANDHY): conceptualizing a transdisciplinary framework for citizen science addressing hydrological challenges. Hydrol. Sci. J. 67 , 2534–2551 (2022).
Dyer, F. et al. Waterwatch data quality: an opportunity to augment professionally collected data sets. In Proc. 7th Australian Stream Management Conference 27–30 (ASM, 2014).
Rose, L. A., Karwan, D. L. & Godsey, S. E. Concentration–discharge relationships describe solute and sediment mobilization, reaction, and transport at event and longer timescales. Hydrol. Processes 32 , 2829–2844 (2018).
Hare, D. K., Helton, A. M., Johnson, Z. C., Lane, J. W. & Briggs, M. A. Continental-scale analysis of shallow and deep groundwater contributions to streams. Nat. Commun. https://doi.org/10.1038/s41467-021-21651-0 (2021).
Li, L. et al. Toward catchment hydro‐biogeochemical theories. WIREs Water https://doi.org/10.1002/wat2.1495 (2021).
Zhi, W. & Li, L. The shallow and deep hypothesis: subsurface vertical chemical contrasts shape nitrate export patterns from different land uses. Environ. Sci. Technol. 54 , 11915–11928 (2020).
Zhi, W., Klingler, C., Liu, J. & Li, L. Widespread deoxygenation in warming rivers. Nat. Clim. Change 13 , 1105–1113 (2023).
Li, L. et al. Climate controls on river chemistry. Earths Future 10 , e2021EF002603 (2022).
Harari, Y. N. Sapiens: A Brief History of Humankind (Random House, 2014).
Popper, K. The Logic of Scientific Discovery (Basic Books, 1959).
Hodges, A. Alan Turing: The Enigma: The Centenary Edition (Princeton Univ. Press, 2012).
Do, H. X., Gudmundsson, L., Leonard, M. & Westra, S. The Global Streamflow Indices and Metadata Archive (GSIM)—Part 1: the production of a daily streamflow archive and metadata. Earth Syst. Sci. Data 10 , 765–785 (2018).
Virro, H., Amatulli, G., Kmoch, A., Shen, L. & Uuemaa, E. GRQA: global river water quality archive. Earth System Sci. Data 13 , 5483–5507 (2021).
Gunn, M. A., Matherne, A. M. & Mason, J. R. R. The USGS at Embudo, New Mexico: 125 Years of Systematic Streamgaging in the United States Report No. 2014-30344 (USGS, 2014).
Burt, T. P. & McDonnell, J. J. Whither field hydrology? The need for discovery science and outrageous hydrological hypotheses. Water Resour. Res. 51 , 5919–5928 (2015).
Read, E. K. et al. Water quality data for national‐scale aquatic research: the Water Quality Portal. Water Resour. Res. 53 , 1735–1745 (2017).
Council, N. R. Confronting the Nation’s Water Problems: The Role of Research (National Academies Press, 2004).
Li, Z., Liu, H., Zhang, C. & Fu, G. Generative adversarial networks for detecting contamination events in water distribution systems using multi-parameter, multi-site water quality monitoring. Environ. Sci. Ecotechnol. 14 , 100231 (2023).
Qu, H. & Yuan, W. Water quality Anomaly detection based on optimally reconfigured convolutional autoencoder. In 2022 International Conference on Wearables, Sports and Lifestyle Management (WSLM) 137–141 (IEEE, 2022).
Shen, C., Chen, X. & Laloy, E. Broadening the use of machine learning in hydrology. Front. Water https://doi.org/10.3389/frwa.2021.681023 (2021).
Schmidhuber, J. Annotated history of modern AI and deep learning. Preprint at https://arxiv.org/abs/2212.11279 (2022).
McCulloch, W. S. & Pitts, W. A logical calculus of the ideas immanent in nervous activity. Bull. Math. Biophys. 5 , 115–133 (1943).
Rosenblatt, F. The perceptron: a probabilistic model for information storage and organization in the brain. Psychol. Rev. 65 , 386 (1958).
Amari, S.-I. Learning patterns and pattern sequences by self-organizing nets of threshold elements. IEEE Trans. Comput. 100 , 1197–1206 (1972).
Maier, H. R. & Dandy, G. C. The use of artificial neural networks for the prediction of water quality parameters. Water Resour. Res. 32 , 1013–1022 (1996).
Maier, H. R. & Dandy, G. C. Neural networks for the prediction and forecasting of water resources variables: a review of modelling issues and applications. Environ. Modell. Softw. 15 , 101–124 (2000).
Chang, F. J. & Hwang, Y. Y. A self-organization algorithm for real-time flood forecast. Hydrol. Process. 13 , 123–138 (1999).
Dawson, C. W. & Wilby, R. L. A comparison of artificial neural networks used for river flow forecasting. Hydrol. Earth Syst. Sci. 3 , 529–540 (1999).
Cigizoglu, H. K. Estimation and forecasting of daily suspended sediment data by multi-layer perceptrons. Adv. Water Res. 27 , 185–195 (2004).
Dransfeld, S., Tatnall, A. R., Robinson, I. S. & Mobley, C. D. A comparison of multi-layer perceptron and multilinear regression algorithms for the inversion of synthetic ocean colour spectra. Int. J. Remote Sens. 25 , 4829–4834 (2004).
Pankiewicz, G. S. Neural network classification of convective airmasses for a flood forecasting system. Int. J. Remote Sens. 18 , 887–898 (1997).
Addor, N., Newman, A. J., Mizukami, N. & Clark, M. P. The CAMELS data set: catchment attributes and meteorology for large-sample studies. Hydrol. Earth Syst. Sci. 21 , 5293–5313 (2017).
Newman, A. et al. Development of a large-sample watershed-scale hydrometeorological data set for the contiguous USA: data set characteristics and assessment of regional variability in hydrologic model performance. Hydrol. Earth Syst. Sci. 19 , 209 (2015).
Kratzert, F. et al. Caravan—a global community dataset for large-sample hydrology. Sci. Data https://doi.org/10.1038/s41597-023-01975-w (2023).
GEMStat Database of the Global Environment Monitoring System for Freshwater (GEMS/Water) Programme (UN Environment Programme, 2018).
Hartmann, J., Lauerwald, R. & Moosdorf, N. GLORICH—global river chemistry database. Pangaea 902360 , 520 (2019).
Rotteveel, L., Heubach, F. & Sterling, S. M. The Surface Water Chemistry (SWatCh) database: a standardized global database of water chemistry to facilitate large-sample hydrological research. Earth Syst. Sci. Data 14 , 4667–4680 (2022).
Sterle, G. et al. CAMELS-Chem: augmenting CAMELS (Catchment Attributes and Meteorology for Large-sample Studies) with atmospheric and stream water chemistry data. Hydrol. Earth Syst. Sci. Discuss. 2022 , 1–23 (2022).
D’Alimonte, D. & Zibordi, G. Phytoplankton determination in an optically complex coastal region using a multilayer perceptron neural network. IEEE Trans. Geosci. Remote Sens. 41 , 2861–2868 (2003).
Download references
Acknowledgements
W.Z. was supported by the National Natural Science Foundation of China (52121006) and by the Barry and Shirley Isett Professorship (to L.L.) at Penn State University. L.L. was supported by the US National Science Foundation via the Critical Zone Collaborative Network (EAR-2012123 and EAR-2012669), Frontier Research in Earth Sciences (EAR-2121621), Signals in Soils (EAR-2034214), and US Department of Energy Environmental System Science (DE-SC0020146). J.P. was supported by Swiss Agency for Development and Cooperation (SDC) (WABES project, 7F-09963.02.01). This paper has been reviewed in accordance with the US Environmental Protection Agency’s peer and administrative review policies and approved for publication. Mention of trade names or commercial products does not constitute endorsement or recommendation for use. Any use of trade, firm, or product names is for descriptive purposes only and does not imply endorsement or recommendation for use by the US Government. Statements in this publication reflect the authors’ professional views and opinions and should not be construed to represent any determination or policy of the US Environmental Protection Agency.
Author information
Authors and affiliations.
The National Key Laboratory of Water Disaster Prevention, Yangtze Institute for Conservation and Development, Key Laboratory of Hydrologic-Cycle and Hydrodynamic-System of Ministry of Water Resources, Hohai University, Nanjing, China
Department of Civil and Environmental Engineering, The Pennsylvania State University, University Park, PA, USA
Wei Zhi & Li Li
US Geological Survey, Reston, VA, USA
Alison P. Appling
Office of Research and Development, US Environmental Protection Agency, Cincinnati, OH, USA
Heather E. Golden
Department of Water Resources and Drinking Water, Swiss Federal Institute of Aquatic Science and Technology (EAWAG), Dübendorf, Switzerland
Joel Podgorski
You can also search for this author in PubMed Google Scholar
Contributions
W.Z. and L.L. conceived the idea for the review paper and wrote the first draft. A.P.A., H.E.G. and J.P. provided content for multiple sections and edited multiple versions of the paper. L.L. finalized the paper.
Corresponding author
Correspondence to Li Li .
Ethics declarations
Competing interests.
The authors declare no competing interests.
Peer review
Peer review information.
Nature Water thanks Danlu Guo and the other, anonymous, reviewer(s) for their contribution to the peer review of this work.
Additional information
Publisher’s note Springer Nature remains neutral with regard to jurisdictional claims in published maps and institutional affiliations.
Supplementary information
Supplementary information.
Supplementary text, Figs. 1 and 2, and Table 1.
Rights and permissions
Springer Nature or its licensor (e.g. a society or other partner) holds exclusive rights to this article under a publishing agreement with the author(s) or other rightsholder(s); author self-archiving of the accepted manuscript version of this article is solely governed by the terms of such publishing agreement and applicable law.
Reprints and permissions
About this article
Cite this article.
Zhi, W., Appling, A.P., Golden, H.E. et al. Deep learning for water quality. Nat Water 2 , 228–241 (2024). https://doi.org/10.1038/s44221-024-00202-z
Download citation
Received : 13 April 2023
Accepted : 10 January 2024
Published : 12 March 2024
Issue Date : March 2024
DOI : https://doi.org/10.1038/s44221-024-00202-z
Share this article
Anyone you share the following link with will be able to read this content:
Sorry, a shareable link is not currently available for this article.
Provided by the Springer Nature SharedIt content-sharing initiative
Quick links
- Explore articles by subject
- Guide to authors
- Editorial policies
Sign up for the Nature Briefing newsletter — what matters in science, free to your inbox daily.

Advertisement
Machine learning algorithms for efficient water quality prediction
- Original Article
- Published: 26 August 2021
- Volume 8 , pages 2793–2801, ( 2022 )
Cite this article
- Mourade Azrour ORCID: orcid.org/0000-0003-1575-8140 1 ,
- Jamal Mabrouki 2 ,
- Ghizlane Fattah 3 ,
- Azedine Guezzaz 4 &
- Faissal Aziz 5
2948 Accesses
77 Citations
4 Altmetric
Explore all metrics
Water is an essential resource for human existence. In fact, more than 60% of the human body is made up of water. Our bodies consume water in every cell, in the different organisms and in the tissues. Hence, water allows stabilization of the body temperature and guarantees the normal functioning of the other bodily activities. Nevertheless, in recent years, water pollution has become a serious problem affecting water quality. Therefore, to design a model that predicts water quality is nowadays very important to control water pollution, as well as to alert users in case of poor quality detection. Motivated by these reasons, in this study, we take the advantages of machine learning algorithms to develop a model that is capable of predicting the water quality index and then the water quality class. The method we propose is based on four water parameters: temperature, pH, turbidity and coliforms. The use of the multiple regression algorithms has proven to be important and effective in predicting the water quality index. In addition, the adoption of the artificial neural network provides the most highly efficient way to classify the water quality.
This is a preview of subscription content, log in via an institution to check access.
Access this article
Subscribe and save.
- Get 10 units per month
- Download Article/Chapter or eBook
- 1 Unit = 1 Article or 1 Chapter
- Cancel anytime
Price includes VAT (Russian Federation)
Instant access to the full article PDF.
Rent this article via DeepDyve
Institutional subscriptions
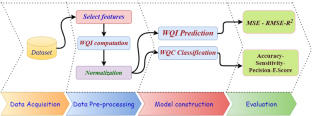
Similar content being viewed by others
Hybrid Machine Learning Algorithms for Effective Prediction of Water Quality
Optimizing Water Quality Parameters Using Machine Learning Algorithms
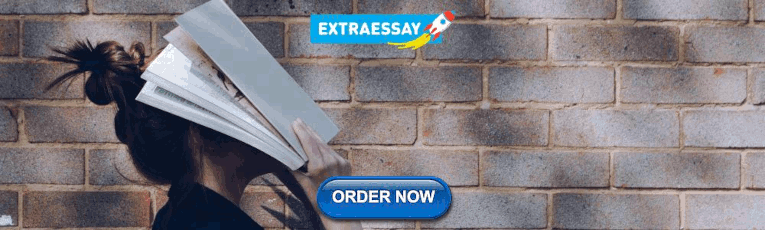
Classification and Analysis of Water Quality Using Machine Learning Algorithms
Explore related subjects.
- Artificial Intelligence
Ahmed U, Mumtaz R, Anwar H et al (2019) Efficient water quality prediction using supervised machine learning. Water 11(11):2210. https://doi.org/10.3390/w11112210
Article Google Scholar
Aldhyani THH, Al-Yaari M, Alkahtani H, Maashi M (2020) Water quality prediction using artificial intelligence algorithms. Appl Bionics Biomech. https://doi.org/10.1155/2020/6659314
Asadollah SBHS, Sharafati A, Motta D, Yaseen ZM (2021) River water quality index prediction and uncertainty analysis: a comparative study of machine learning models. J Environ Chem Eng 9(1):104599. https://doi.org/10.1016/j.jece.2020.104599
Azrour M, Farhaoui Y, Ouanan M, Guezzaz A (2019) SPIT detection in telephony over IP using K-means algorithm. Proc Comput Sci 148:542–551. https://doi.org/10.1016/j.procs.2019.01.027
Bekesiene S, Meidute-Kavaliauskiene I, Vasiliauskiene V (2021) Accurate prediction of concentration changes in ozone as an air pollutant by multiple linear regression and artificial neural networks. Mathematics 9(4):356. https://doi.org/10.3390/math9040356
Ciulla G, D’Amico A (2019) Building energy performance forecasting: a multiple linear regression approach. Appl Energy 253:113500. https://doi.org/10.1016/j.apenergy.2019.113500
Deng T, Chau K-W, Duan H-F (2021) Machine learning based marine water quality prediction for coastal hydro-environment management. J Environ Manag 284:112051. https://doi.org/10.1016/j.jenvman.2021.112051
Dezfooli D, Hosseini-Moghari S-M, Ebrahimi K, Araghinejad S (2018) Classification of water quality status based on minimum quality parameters: application of machine learning techniques. Model Earth Syst Environ. https://doi.org/10.1007/s40808-017-0406-9
El Bilali A, Taleb A (2020) Prediction of irrigation water quality parameters using machine learning models in a semi-arid environment. J Saudi Soc Agric Sci 19(7):439–451. https://doi.org/10.1016/j.jssas.2020.08.001
Ewaid SH (2017) Water quality evaluation of Al-Gharraf river by two water quality indices. Appl Water Sci 7(7):3759–3765
Griffiths O, Henderson H, Simpson M (2010) Environmental Health Practitioner Manual: (Common wealth of Australia). https://ww2.health.wa.gov.au/~/media/Files/Corporate/general-documents/Environmental-health/Aboriginal-environmental-health/Environmental-Health-Practitioner-Manual.pdf . Accessed 10 Aug 2021
Guezzaz A, Asimi Y, Azrour M, Asimi A (2021b) Mathematical validation of proposed machine learning classifier for heterogeneous traffic and anomaly detection. Big Data Min Anal 4(1):18–24. https://doi.org/10.26599/BDMA.2020.9020019
Guezzaz A, Asimi A, Asimi Y, Azrour M, Benkirane S (2021) A distributed intrusion detection approach based on machine leaning techniques for a cloud security. In: Gherabi N, Kacprzyk J (eds) Intelligent systems in big data, semantic web and machine learning. Advances in intelligent systems and computing, vol 1344. Springer, Cham. https://doi.org/10.1007/978-3-030-72588-4_6
Chapter Google Scholar
Guo Q, Zhuang T, Li Z, He S (2021) Prediction of reservoir saturation field in high water cut stage by bore-ground electromagnetic method based on machine learning. J Petrol Sci Eng 204:108678. https://doi.org/10.1016/j.petrol.2021.108678
Haghiabi AH, Nasrolahi AH, Parsaie A (2018) Water quality prediction using machine learning methods. Water Qual Res J 53(1):3–13. https://doi.org/10.2166/wqrj.2018.025
Harkins RD (1974) An objective water quality index. J (water Pollution Control Federation) 46(3):588–591
Google Scholar
Hasan MK, Alam MA, Das D, Hossain E, Hasan M (2020) Diabetes prediction using ensembling of different machine learning classifiers. IEEE Access 8:76516–76531. https://doi.org/10.1109/ACCESS.2020.2989857
Ighalo JO, Adeniyi AG, Marques G (2021) Artificial intelligence for surface water quality monitoring and assessment: a systematic literature analysis. Model Earth Syst Environ 7(2):669–681. https://doi.org/10.1007/s40808-020-01041-z
Imani M, Hasan MM, Bittencourt LF, McClymont K, Kapelan Z (2021) A novel machine learning application: water quality resilience prediction Model. Sci Total Environ 768:144459. https://doi.org/10.1016/j.scitotenv.2020.144459
Kapadia D, Jariwala N (2021) Prediction of tropospheric ozone using artificial neural network (ANN) and feature selection techniques. Model Earth Syst Environ. https://doi.org/10.1007/s40808-021-01220-6
Kicsiny R (2014) Multiple linear regression based model for solar collectors. Sol Energy 110:496–506. https://doi.org/10.1016/j.solener.2014.10.003
Kumar MJV, Samalla K (2019) Design and development of water quality monitoring system in IOT. Int J Recent Technol Eng 7(5):7
Li D, Liu S (2019) System and platform for water quality monitoring. Water Qual Monit Manag. https://doi.org/10.1016/B978-0-12-811330-1.00003-X
Lu H, Ma X (2020) Hybrid decision tree-based machine learning models for short-term water quality prediction. Chemosphere 249:126169. https://doi.org/10.1016/j.chemosphere.2020.126169
Lumb A, Sharma TC, Bibeault J-F, Klawunn P (2011) A comparative study of USA and Canadian water quality index models. Water Qual Expo Health 3(3–4):203–216
Mabrouki J, Azrour M, Boubekraoui A, El Hajjaji S (2021a) Intelligent system for the protection of people. In: Intelligent systems in big data, semantic web and machine learning. Springer, pp 157–165
Mabrouki J, Azrour M, Dhiba D, Farhaoui Y, Hajjaji SE (2021b) IoT-based data logger for weather monitoring using arduino-based wireless sensor networks with remote graphical application and alerts. Big Data Min Anal 4(1):25–32. https://doi.org/10.26599/BDMA.2020.9020018
Mabrouki J, Azrour M, Fattah G, Dhiba D, Hajjaji SE (2021c) Intelligent monitoring system for biogas detection based on the Internet of Things: Mohammedia, Morocco city landfill case. Big Data Min Anal 4(1):10–17
Mabrouki J, Fattah G, Al-Jadabi N, Abrouki Y, Dhiba D, Azrour M, Hajjaji SE (2021d) Study, simulation and modulation of solar thermal domestic hot water production systems. Model Earth Syst Environ. https://doi.org/10.1007/s40808-021-01200-w
Mabrouki J, Azrour M, El Hajjaji S (2021e) Use of internet of things for monitoring and evaluation water’s quality: comparative study. Int J Cloud Comput (in press)
Mabrouki J, Azrour M, Farhaoui Y, El Hajjaji S (2021f) Intelligent system for monitoring and detecting water quality. In: Farhaoui Y (ed) Big data and networks technologies, vol 81. Springer International Publishing, pp 172–182. https://doi.org/10.1007/978-3-030-23672-4_13
Miry AH, Aramice GA (2020) Water monitoring and analytic based thingspeak. Int J Electr Comput Eng (IJECE) 10(4):3588–3595. https://doi.org/10.11591/ijece.v10i4.pp3588-3595
Momenzadeh L, Zomorodian A, Mowla D (2011) Experimental and theoretical investigation of shelled corn drying in a microwave-assisted fluidized bed dryer using artificial neural network. Food Bioprod Process 89(1):15–21. https://doi.org/10.1016/j.fbp.2010.03.007
Nabavi-Pelesaraei A, Rafiee S, Hosseini-Fashami F, Chau K (2021) Artificial neural networks and adaptive neuro-fuzzy inference system in energy modeling of agricultural products. In: Predictive modelling for energy management and power systems engineering. Elsevier, pp 299–334. https://doi.org/10.1016/B978-0-12-817772-3.00011-2
Naga C, Talnan Jean Honoré C, Delfin OA, Bernard YO, Guillaume ZS, Henoc Sosthène A, Mpakama Z, Issiaka S (2018) Spatio-temporal analysis and water quality indices (WQI): case of the Ébrié Lagoon, Abidjan. Côte D’ivoire Hydrology 5(3):32. https://doi.org/10.3390/hydrology5030032
Pasika S, Gandla ST (2020) Smart water quality monitoring system with cost-effective using IoT. Heliyon 6(7):e04096. https://doi.org/10.1016/j.heliyon.2020.e04096
Rath S, Tripathy A, Tripathy AR (2020) Prediction of new active cases of coronavirus disease (COVID-19) pandemic using multiple linear regression model. Diabetes Metab Syndr 14(5):1467–1474. https://doi.org/10.1016/j.dsx.2020.07.045
Singha S, Pasupuleti S, Singha SS, Singh R, Kumar S (2021) Prediction of groundwater quality using efficient machine learning technique. Chemosphere 276:130265. https://doi.org/10.1016/j.chemosphere.2021.130265
Sossi Alaoui S, Aksasse B, Farhaoui Y (2020) Data mining and machine learning approaches and technologies for diagnosing diabetes in women. In: Farhaoui Y (ed) Big data and networks technologies. Springer International Publishing, pp 59–72. https://doi.org/10.1007/978-3-030-23672-4_6
The California Water System (2021) https://water.ca.gov/Water-Basics/The-California-Water-System . Accessed 3 June 2021
Tunc Dede O, Telci IT, Aral MM (2013) The use of water quality index models for the evaluation of surface water quality: a case study for Kirmir Basin, Ankara, Turkey. Water Qual Expo Health 5(1):41–56. https://doi.org/10.1007/s12403-013-0085-3
Westall F, Brack A (2018) The importance of water for life. Space Sci Rev 214(2):50. https://doi.org/10.1007/s11214-018-0476-7
Zotou I, Tsihrintzis VA, Gikas GD (2020) Water quality evaluation of a lacustrine water body in the Mediterranean based on different water quality index (WQI) methodologies. J Environ Sci Health Part A 55(5):537–548
Download references
Author information
Authors and affiliations.
Computer Sciences Department, IDMS Team, Faculty of Sciences and Techniques, Moulay Ismail University, Errachidia, Morocco
Mourade Azrour
Laboratory of Spectroscopy, Molecular Modeling, Materials, Nanomaterial, Water and Environment, CERNE2D, Faculty of Science, Mohammed V University in Rabat, Rabat, Morocco
Jamal Mabrouki
Civil Hydraulic and Environmental Engineering Laboratory, Water Treatment and Reuse Structure, Mohammadia School of Engineers, Mohammed V University in Rabat, Avenue Ibn Sina B.P 765, 10090, Agdal Rabat, Morocco
Ghizlane Fattah
Department of Computer Science and Mathematics, High School of Technology, Cadi Ayyad University, 44000, Essaouira, Morocco
Azedine Guezzaz
Laboratory of Water, Biodiversity and Climate Change, Semlalia Faculty of Sciences, University Cadi Ayyad, Marrakech, Morocco
Faissal Aziz
You can also search for this author in PubMed Google Scholar
Corresponding author
Correspondence to Mourade Azrour .
Additional information
Publisher's note.
Springer Nature remains neutral with regard to jurisdictional claims in published maps and institutional affiliations.
Rights and permissions
Reprints and permissions
About this article
Azrour, M., Mabrouki, J., Fattah, G. et al. Machine learning algorithms for efficient water quality prediction. Model. Earth Syst. Environ. 8 , 2793–2801 (2022). https://doi.org/10.1007/s40808-021-01266-6
Download citation
Received : 29 June 2021
Accepted : 13 August 2021
Published : 26 August 2021
Issue Date : June 2022
DOI : https://doi.org/10.1007/s40808-021-01266-6
Share this article
Anyone you share the following link with will be able to read this content:
Sorry, a shareable link is not currently available for this article.
Provided by the Springer Nature SharedIt content-sharing initiative
- Machine learning
- Data analysis
- Artificial intelligence
- Water quality
- Find a journal
- Publish with us
- Track your research
Information
- Author Services
Initiatives
You are accessing a machine-readable page. In order to be human-readable, please install an RSS reader.
All articles published by MDPI are made immediately available worldwide under an open access license. No special permission is required to reuse all or part of the article published by MDPI, including figures and tables. For articles published under an open access Creative Common CC BY license, any part of the article may be reused without permission provided that the original article is clearly cited. For more information, please refer to https://www.mdpi.com/openaccess .
Feature papers represent the most advanced research with significant potential for high impact in the field. A Feature Paper should be a substantial original Article that involves several techniques or approaches, provides an outlook for future research directions and describes possible research applications.
Feature papers are submitted upon individual invitation or recommendation by the scientific editors and must receive positive feedback from the reviewers.
Editor’s Choice articles are based on recommendations by the scientific editors of MDPI journals from around the world. Editors select a small number of articles recently published in the journal that they believe will be particularly interesting to readers, or important in the respective research area. The aim is to provide a snapshot of some of the most exciting work published in the various research areas of the journal.
Original Submission Date Received: .
- Active Journals
- Find a Journal
- Proceedings Series
- For Authors
- For Reviewers
- For Editors
- For Librarians
- For Publishers
- For Societies
- For Conference Organizers
- Open Access Policy
- Institutional Open Access Program
- Special Issues Guidelines
- Editorial Process
- Research and Publication Ethics
- Article Processing Charges
- Testimonials
- Preprints.org
- SciProfiles
- Encyclopedia
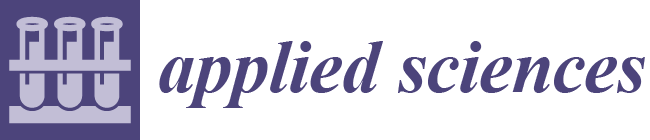
Article Menu
- Subscribe SciFeed
- Recommended Articles
- Google Scholar
- on Google Scholar
- Table of Contents
Find support for a specific problem in the support section of our website.
Please let us know what you think of our products and services.
Visit our dedicated information section to learn more about MDPI.
JSmol Viewer
A review of the artificial neural network models for water quality prediction.

1. Introduction
- First, we identified ANN-related papers in influential water-related and environmental-related journals to ensure that high-quality papers are included in the review. These papers are mainly from journals whose subjects are environmental science and ecology, water resources, engineering and application.
- Thereafter, a keyword search of the ISI Web of Science was then conducted for the period 2008–2019 using the keywords; water quality, river, lake, reservoir, WWTP, groundwater, pond, prediction, and forecasting, accompanied by the names of ANN methods (one or more), such as neural network, MLP, RBFNN, GRNN, RNN, to name but a few.
- Then, through the search process from 1 to 2, 151 articles in English relevant to our focus were selected. The basic information of the papers, including authors (year), locations, water quality variables, meteorological factors, other factors, output strategy, data size, time step, data dividing, methods, and prediction lengths are provided in Appendix A .
3. Three Basic Model Structures in Water Quality Prediction
3.1. feedforward architectures, 3.2. recurrent architectures, 3.3. hybrid architectures, 3.4. emerging methods, 4. artificial neural networks models for water quality prediction, 5.1. data collection, 5.2. output strategy, 5.3. input selection, 5.4. data dividing, 5.5. data preprocessing, 5.6. model structure determination, 5.7. model training, 6. discussion, 6.1. data are the foundation, 6.2. data processing is key, 6.3. model is the core, author contributions, acknowledgments, conflicts of interest.
Categories | Authors (Year) | Locations | Water Quality Variables | Meteorological Factors | Other Factors | Output Strategy | Dataset | Time Step | Data Dividing | Methods | Prediction Lengths |
---|---|---|---|---|---|---|---|---|---|---|---|
Feedforward | [ ] | WWTP(Turkey) | BOD; SS, TN, TP | NA | Q | Category 2 | 364 samples (1 year) | daily | Train: 67%, test:33% | ANN, MLR | NA |
Feedforward | [ ] | Mamasin dam reservoir (Turkey) | DO, EC; SS, TN, WT | RF | AODD | Category 2 | No details | No details | No details | ANN(MLP) | NA |
Feedforward | [ ] | Singapore coastal waters (Singapore) | S, DO, Chl-a;; WT | NA | NA | Category 3 | 32 samples (5 months) | No details | No details | ANN(MLP), GRNN | 1 |
Feedforward | [ ] | Feitsui Reservoir (China) | Chl-a; | NA | Bands | Category 2 | No details | No details | Train: 75%, test:25% | ANN(MLP) | NA |
Feedforward | [ ] | Pyeongchang river (Korea) | DO, TOC; WT | NA | Q | Category 3 | No details (3 months) | 5 minutes | No details | ANN, MNN, ANFIS | 12,24 |
Feedforward | [ ] | Feitsui Reservoir (China) | Chl-a; | NA | Bands | Category 2 | No details (7 years) | No details | Train:57%, validate: 29%, test: 14% | ANN(MLP) | NA |
Feedforward | [ ] | Melen River (Turkey) | BOD; COD, WT, DO, Chl-a, NH -N, NO , NO | NA | F, Ns | Category 2 | No details (over 6 years) | monthly | Train:60%, validate: 20%, test: 20% | ANN(MLP) | NA |
Feedforward | [ ] | Moshui River (China) | COD, NH -N;; | NA | mineral oil;; | Category 0 | No details (5 years) | No details | Train:80%, test: 20% | BPNN | NA |
Feedforward | [ ] | Doce River (Brazil) | WT, pH, EC, TN | NA | other ions | Category 2 | 232samples (3 years) | No details | Train:50%, validate: 25%, test: 25% | ANN | NA |
Feedforward | [ ] | NA (China) | pH, DO;; WT, S, NH -N, NO | NA | NA | Category 3 | 500 samples | No details | Train:80%, test: 20% | BPNN | NA |
Feedforward | [ ] | Gomti river (India) | DO, BOD; pH, TA, TH, TS, COD, NH -N, NO , P | RF | NA | Category 2 | 500 samples (10 years) | monthly | Train:60%, validate: 20%, test: 20% | ANN | NA |
Feedforward | [ ] | Pyeongchang River (Korea) | TOC;; | Precip | Q;; | Category 3 | No details (7 years) | No details | No details | ANN | NA |
Feedforward | [ ] | Groundwater (China) | NO , COD;; | NA | other 7 variables | Category 3 | 97 samples | No details | Train:56%, test: 44% | ANN | NA |
Feedforward | [ ] | Omerli Lake (Turkey) | DO; BOD, NH -N, NO , NO , P | NA | NA | Category 2 | No details (17 years) | No details | No details | ANN, MLR, NLR | NA |
Feedforward | [ ] | Changle River (China) | DO, TN, TP;; WT | RF | F, FTT | Category 3 | No details (18months) | monthly | No details | BPNN | NA |
Feedforward | [ ] | Sangamon River (USA) | NO ;; | AT, Precip | Q | Category 3 | No details (6 years) | weekly | Train:50%, test: 50% | ANN | 1 |
Feedforward | [ ] | Surface water (Turkey) | Chl-a; | NA | other 12 variables | Category 2 | 110 samples | No details | Train:67%, test: 33% | ANN(MLP) | NA |
Feedforward | [ ] | Gruˇza reservoir (Serbia) | DO; pH, WT, CL, TP, NO , NH -N, EC | NA | Fe, Mn | Category 2 | 180samples (3 years) | No details | Train:84%, test: 16% | ANN | NA |
Feedforward | [ ] | The tank (China) | DO;; pH, S, WT | AT | NA | Category 3 | No details (22 months) | 1 minute | Train:57%, validate: 29%, test: 14% | ANN | 30 |
Feedforward | [ ] | Groundwater (India) | S; EC | NA | WL, T, Pumping, Rainp | Category 2 | No details (7 years) | No details | Train:29%, test: 71% | ANN | NA |
Feedforward | [ ] | WWTP(China) | BOD; COD, SS, pH, NH –N | NA | Oil | Category 2 | No details | No details | Train:50%, test: 50% | RBFNN | 5 |
Feedforward | [ ] | Groundwater (Iran) | NO ; pH, EC, TDS, TH | NA | Mg, Cl, Na, K, HCO , SO , Ca, ICs | Category 2 | 818samples (nearly 17days) | 30 minutes | Train:70%, test: 30% | ANN, Linear regression (LR) | NA |
Feedforward | [ ] | Wells (Palestine) | NO ; | NA | Q, other five variables | Category 2 | 975samples (16 years) | No details | No details | MLP, RBF, GRNN | NA |
Feedforward | [ ] | Upstream and downstream (USA) | DO; pH, WT, EC | NA | Q | Category 2 | 2063, 4765 samples (18 years) | daily | Train:50%, validate:25%, test: 25% | RBFNN, ANN(MLP), MLR, | NA |
Feedforward | [ ] | WWTP (Korea) | DO;; NH -N | NA | NA | Category 3 | 1900 samples | No details | Train:45%, validate:5%, test: 50% | MNN | NA |
Feedforward | [ ] | Eastern Black Sea Basin (Turkey) | SS; Tur | NA | NA | Category 1 | 144 samples (1 year) | fortnightly | Train:75%, validate:8%, test: 17% | ANN(MLP) | NA |
Feedforward | [ ] | Kinta River (Malaysia) | DO, BOD, NH -N, pH, COD, Tur;; | NA | NA | Category 2 | 255 samples (7 months) | No details | Train:80%, validate:10%, test: 10% | ANN(MLP) | NA |
Feedforward | [ ] | Power station (New Zealand) | WT; | AT, AP, WD, WS | other 8 variables | Category 2 | 45,594 samples (2 years) | 10 minutes | Train:70%, test: 30% | ANN(MLP) | 12 |
Feedforward | [ ] | Yuan-Yang Lake (China) | WT; | SR, AP, RH, AT, WS, WD | ST | Category 2 | No details (2 months) | 10 minutes | Train:70%, validate & test: 30% | ANN(MLP) | 1 |
Feedforward | [ ] | Experimental system (UK) | BOD, NH -N, NO , P; DO, WT, pH, EC, TSS, Tur | NA | RP | Category 2 | 195samples (4 years) | No details | Train: 62%, test: 38% | ANN | NA |
Feedforward | [ ] | Lake Fuxian (China) | DO, TP, SD, Chl-a;; TN, WT, pH | NA | Month; | Category 2 and Category 3 | No details | No details | No details | ANN | NA |
Feedforward | [ ] | Doiraj River (Iran) | SS; | RF | Q | Category 1 and Category 2 | more than 3000 samples (11 years) | daily | No details | ANN, Support vector regression (SVR) | 1 |
Feedforward | [ ] | Lake Abant (Turkey) | DO, Chl-a; WT, EC | NA | MDHM | Category 2 | 6674 samples (86 days) | 15 minutes | Train:60%, validate:15%, test: 25% | ANN, Multiple nonlinear regression (MNLR) | NA |
Feedforward | [ ] | Johor River, Sayong River (Malaysia) | TDS, EC, Tur; | NA | NA | Category 1 | No details (5 years) | No details | The test set is approximately 10–40 % of the size of the training data set | ANN(MLP), RBFNN, LR | NA |
Feedforward | [ ] | Mine water (India) | BOD, COD; WT, pH, DO, TSS | NA | other | Category 2 | 73 samples | No details | Train:79%, test: 21% | ANN | NA |
Feedforward | [ ] | Heihe River (China) | DO; pH, NO , NH3-N, EC, TA, TH | NA | Cl, Ca | Category 2 | 164 samples (over 6 years) | monthly | Train:60%, validate:20%, test: 20% | ANN(MLP) | NA |
Feedforward | [ ] | Danube River (Serbia) | DO; WT, pH, NO , EC | Na, CL, SO HCO , other 11 variables | Category 2 | 1512 samples (9 years) | No details | Train:70%, validate:20%, test: 10% | GRNN | NA | |
Feedforward | [ ] | Stream Harsit (Turkey) | SS; Tur | NA | TCC, TIC | Category 1 and Category 2 | 132 samples (11months) | No details | No details | ANN(MLP) | NA |
Feedforward | [ ] | Feitsui Reservoir (China) | DO; WT, pH, EC, Tur, SS, TH, TA, NH -N | NA | NA | Category 2 | 400 samples (20 years) | No details | No details | BPNN, ANFIS, MLR | NA |
Feedforward | [ ] | Stream (USA) | WT; | AT | Form attributes, forested land cover | Category 2 | 982 (6 months) | daily | Train:90%, validate & test: 10% | ANN(MLP) | NA |
Feedforward | [ ] | The Bahr Hadus drain (Egypt) | DO, TDS;; | NA | NA | Category 0 | No details | monthly | Train:80%, test: 20% | CCNN, BPNN | NA |
Feedforward | [ ] | Karoon River (Iran) | DO, COD, BOD; EC, pH, Tur, NO , NO , P | NA | Ca, Mg, Na | Category 2 | 200 samples (17 years) | monthly | Train:80%, test: 20% | ANN(MLP), RBFNN, ANFIS | NA |
Feedforward | [ ] | Manawatu River (New Zealand) | NO ; | NA | EMS (Energy, Mean, Skewness) | Category 1 | 144 samples | weekly | Train: 70%, test: 30% | RBFNN | NA |
Feedforward | [ ] | WWTP (China) | BOD; DO, pH, SS | NA | F, TNs | Category 2 | 360 samples | daily | Train: 83%, test: 17% | HELM, Bayesian approach, ELM | NA |
Feedforward | [ ] | Nalón river (Spain) | Tur; NH -N, EC, DO, pH, WT | NA | NA | Category 2 | No details (1 year) | 15 minutes | Train: 90%, test: 10% | ANN(MLP) | NA |
Feedforward | [ ] | Groundwater (Turkey) | pH, TDS, TH | NA | SAR, SO4; CL | Category 2 | 124 samples (1 year) | monthly | Train: 84.1%, test: 15.9% | ANN | NA |
Feedforward | [ ] | Johor River (Malaysia) | DO; WT, pH, NO , NH -N | NA | NA | Category 2 | No details (10 year) | monthly | Train:60%, validate: 25%, test: 15% | ANN(MLP), ANFIS | NA |
Feedforward | [ ] | The Taipei Water Source Domain (China) | Tur; | RF | NA | Category 2 | No details (1 year) | No details | No details | BPNN | NA |
Feedforward | [ ] | Mashhad plain (Iran) | EC; | NA | CL; Lon, Lat | Category 2 and Category 3 | 122 samples | No details | Train:65%, validate: 20%, test: 15% | ANN(MLP), ANFIS, geostatistical models | NA |
Feedforward | [ ] | Tai Po River (China) | DO; pH, EC, WT, NH -N, TP, NO , NO | NA | CL | Category 2 | 252 samples (21 years) | No details | Train:85%, test: 15% | ANN, ANFIS, MLR | NA |
Feedforward | [ ] | Ireland Rivers (Ireland) | DO, BOD, Alk, TH;; WT, pH, EC | NA | DOP (dissolved oxygen percentage), CL;; | Category 2 | 3001 samples (No details) | No details | No details | ANN | NA |
Feedforward | [ ] | Twostatistical databases (European countries) | BOD; DO | NA | other 20 variables | Category 2 | 159 samples (9 years) | No details | Train:88%, test: 12% | GRNN, MLR | NA |
Feedforward | [ ] | Maroon River (Iran) | WT, Tur, pH, EC, TDS, TH; | NA | HCO , SO , CL, Na, K, Mg, Ca | Category 2 | No details (20 years) | monthly | Train:60%, validate: 15%, test: 35% | ANN(MLP), RBFNN | NA |
Feedforward | [ ] | River Zayanderud (Iran) | TSS; pH, TH | NA | Na, Mg, CO , HCO , CL, Ca | Category 2 | 1320 samples (10 years) | monthly | No details | RBFNN, TDNN | NA |
Feedforward | [ ] | Ardabil plain (Iran) | EC, TDS; | RF | RO, WL | Category 2 | No details (17 years) | 6 months | Train:71%, test: 29% | ANN, MLR | 1 |
Feedforward | [ ] | Danube River (Serbia) | BOD; WT, DO, pH, NH -N, COD, EC, NO , TH, TP | NA | other 8 variables | Category 2 | more than 32,000 samples (years) | No details | Train:72%, validate: 18%, test: 10% | GRNN | NA |
Feedforward | [ ] | Hydrometric stations (USA) | SS;; | NA | Q | Category 0 and Category 3 | No details (8 years) | daily | Train and test:80%, validate:20% | ANN(MLP), SVR, MLR | 1 |
Feedforward | [ ] | Surma River (Angladesh) | BOD, COD;; | NA | NA | Category 0 and Category 3 | No details (3 years) | No details | Train:70%, validate: 15%, test: 15% | RBFNN, MLP | NA |
Feedforward | [ ] | Groundwater (Palestine) | S; EC, TDS, NO | NA | Mg, Ca, Na | Category 2 | No details (11 years) | No details | Train: more than 50%, test: less than 50% | ANN(MLP), SVM | NA |
Feedforward | [ ] | River Danube (Hungary) | DO; pH, WT, EC | NA | RO | Category 2 | More than 151 samples (6 years) | monthly | No details | GRNN, ANN(MLP), RBFNN, MLR | NA |
Feedforward | [ ] | Langat River and Klang River (Malaysia) | DO, BOD, COD, SS, pH, NH -N; | NA | NA | Category 2 | No details (10 years) | monthly | Train:80%, validate: 20% | RBFNN | NA |
Feedforward | [ ] | Eight United States Geological Survey stations (USA) | DO; WT, EC, Tur, pH | NA | YMDH | Category 2 | 35,064 samples (4 years) | hourly | Train:70%, test: 30% | ELM, ANN(MLP) | 1, 12, 24, 48, 72, 168 |
Feedforward | [ ] | Rivers (China) | DO; WT, pH, BOD, NH -N, TN, TP | NA | other variables | Category 2 | 969 samples | No details | Train and validate: 80%, test: 20% | BPNN, SVM, MLR | NA |
Feedforward | [ ] | Syrenie Stawy Ponds (Poland) | DO, BOD, COD, TN, TP, TA | NA | CL; other ions | Category 2 | No details (19 months) | monthly | Train:60%, validate: 20%, test: 20% | ANN(MLP) | NA |
Feedforward | [ ] | Delaware River (USA) | DO; pH, EC, WT | NA | Q | Category 1 and Category 2 | 2063 samples (6 years) | daily | Train:75%, test: 25% | ANN(MLP), RBFNN, SVM | NA |
Feedforward | [ ] | Zayandeh-rood River (Iran) | NO ; EC, pH, TH | NA | Na, K, Ca, Mg, SO , CL, bicarbonate | Category 2 | No details | No details | Train:50%, validate: 30%, test: 20% | ANN(MLP) | NA |
Feedforward | [ ] | Saint John River (Canada) | TSS, COD, BOD, DO, Tur; | NA | NA | Category 2 | 39 samples (3 days) | No details | Train:60%, validate: 20%, test: 20% | BPNN, SVM | NA |
Feedforward | [ ] | Karkheh River (Iran) | BOD; TDS, EC | NA | CL, Na, SO , Mg, SAR, Ca | Category 2 | 13,800 samples (5 years) | No details | No details | ANN | NA |
Feedforward | [ ] | Xuxi River (China) | COD; WT, DO, TN, TP, NH -N, SD, SS | NA | NA | Category 2 | 110 samples (8 hours) | No details | No details | MLP | NA |
Feedforward | [ ] | Danube River (Serbia) | DO; pH, WT, EC, BOD, COD, SS, P, NO , TA, TH | NA | five metal ions | Category 2 | No details (6 years; 7 years) | monthly or fortnightly | Train:72%, validate: 18%, test: 10% | BPNN | NA |
Feedforward | [ ] | Sufi Chai river (Iran) | TDS; | NA | Q, Other 4 variables | Category 2 | 144 samples (12 years) | monthly | Train:66%, validate: 17%, test: 17% | ANN(MLP) | NA |
Feedforward | [ ] | River Tisza (Hungary) | DO; WT, EC, pH | NA | RO | Category 2 | More than 1300 samples (6 years) | No details | Train:67%, test: 33% | RBFNN, GRNN, MLR | 12 |
Feedforward | [ ] | Karoon River (Iran) | TH; EC, TDS, pH | NA | SAR; HCO , CL, SO , Ca, Mg, Na, K, TAC | Category 2 | No details (49 years) | No details | No details | ANN(MLP), RBFNN | NA |
Feedforward | [ ] | Yamuna River (India) | DO;; BOD, COD, pH, WT, NH -N | NA | Q | Category 3 | No details (4 years) | monthly | Train:75%, test: 25% | BPNN, SVM, ANFIS, ARIMA | NA |
Feedforward | [ ] | Lakes (USA) | Chl-a; TP, TN, Tur | NA | SD | Category 2 | 1087 samples (6 years) | No details | Train:75%, test: 25% | MLP, ANFIS | NA |
Feedforward | [ ] | Karoun River (Iran) | BOD, COD; EC, Tur, pH | NA | six mental ions | Category 2 | 200 samples (16 years) | No details | No details | ANN, ANFIS, Least Squares SVM(LSSVM) | NA |
Feedforward | [ ] | Lakes (USA) | TN, TP; pH, EC, Tur | NA | NA | Category 2 | 1217 samples | No details | Train:55%, validate: 22%, test: 23% | ANN, LR | NA |
Feedforward | [ ] | Three rivers (USA) | WT; | AT | Q, DOY | Category 2 | No details (8 years) | No details | No details | ELM, ANN(MLP), MLR | NA |
Feedforward | [ ] | St. Johns River (USA) | DO; NH -N, TDS, pH, WT | NA | CL | Category 2 | 232 samples (12 years) | half a month | Train:75%, test: 25% | CCNN, DWT, VMD-MLP, MLP | NA |
Recurrent | [ ] | Talkheh Rud River (Iran) | TDS; | NA | Q | Category 1 | No details (13 years) | No details | Train:69%, validate & test: 31% | Elman, ANN(MLP) | 1 |
Recurrent | [ ] | Hyriopsis Cumingii ponds (China) | DO;; pH, WT | SR, WS, AT | NA | Category 3 | 816 samples (34 days) | No details | Train and validate:80%, test: 20% | Elman | NA |
Recurrent | [ ] | Danube River (Serbia) | DO; WT, pH, EC | NA | Q | Category 2 | 61 samples | monthly or semi-monthly | Train: 85%, test: 15% | Elman, GRNN, BPNN, MLR | NA |
Recurrent | [ ] | Chou-Shui River (China) | pH, Alk | NA | As;; Ca | Category 3 | No details (8 years) | No details | No details | Systematical dynamic-neural modeling (SDM), BPNN, NARX | NA |
Recurrent | [ ] | Yenicaga Lake (Turkey) | DO; WT, EC, pH | NA | WL, DOY, hour | Category 2 | 13,744 samples (573 days) | 15 minutes | Train:60%, validate: 15%, test: 25% | TLRN, RNN, TDNN | NA |
Recurrent | [ ] | Dahan River (China) | TP;; EC, SS, pH, DO, BOD, COD, WT, NH -N | NA | Coli | Category 3 | 280 samples (11 years) | monthly | Train:75%, test: 25% | NARX, BPNN, MLR | 1 |
Recurrent | [ ] | Taihu Lake (China) | DO, TP;; | NA | NA | Category 0 | 657 samples (7 years) | monthly | Train:90%, test: 10% | LSTM, BPNN, OS-ELM | NA |
Recurrent | [ ] | WWTP(China) | BOD, TP;; COD, TSS, pH, DO, WT | NA | ORP | Category 2 and Category 3 | 5000 samples | No details | Train:45%, validate: 15%, test: 40% | RESN | NA |
Recurrent | [ ] | Mariculturebase (China) | WT, pH; EC, S, Chl-a, Tur, DO | NA | NA | Category 2 | 710 samples (21 days) | 5 minutes | Train:86%, test: 14% | LSTM, RNN | >32 |
Recurrent | [ ] | Marine aquaculture base (China) | pH, WT;; | NA | NA | Category 0 | 710 samples | No details | Train:86%, test: 14% | SRU | NA |
Recurrent | [ ] | Geum River basin (Korea) | BOD, COD, SS; | AT, WS | WL, Q | Category 2 | No details (10 years) | daily | Train:70%, test: 30% | RNN, LSTM | 1 |
Recurrent | [ ] | Lakes (USA) | WT;; | NA | NA | Category 0 | 1520 samples | No details | Train:65%, test: 35% | LSTM | NA |
Recurrent | [ ] | Reservoir (China) | Chl-a;; WT, pH, EC, DO, Tur | NA | ORP | Category 0 and Category 2 | 1440 samples (5 days) | 5 minutes | No details | TL-FNN, RNN, LSTM | NA |
Recurrent | [ ] | Two gauged stations (USA) | SS;; | NA | Q | Category 1 | 10,060 samples (30 years) | daily | Train: 70–90%, test: 30–10% | WANN | NA |
Recurrent | [ ] | Agricultural catchment (France) | NO , SS; | RF | Q | Category 1 and Category 2 | 26,355 samples (1 year) | daily | Train: 66.67%, test: 33.33% | SOM-MLP, MLP | NA |
Recurrent | [ ] | Four streams (USA) | WT; | SR, AT | NA | Category 2 | No details (4 years) | 10 minutes | Train:50%, validate: 25%, test: 25% | u GA-ANN, BPNN, RBFNN | NA |
Hybrid | [ ] | Chaohu Lake (China) | TP, TN, Chl-a; | NA | Bands | Category 2 | 18,368 (TN),1050(TP) samples (more than 3 years) | No details | Train:86%, test: 14% | GA-BP, BPNN, RBFNN | NA |
Hybrid | [ ] | Two stations (USA) | SS;; | NA | Q | Category 1 and Category 3 | 730 samples (2 years) | daily | Train:50%, test: 50% | ANN-differential evolution | NA |
Hybrid | [ ] | B¨uy ¨ uk Menderes river (Turkey) | WT, DO, B;; | NA | NA | Category 0 | 108 samples (9 years) | monthly | Train:67%, test: 33% | ARIMA-ANN, ANN, ARIMA | NA |
Hybrid | [ ] | Karkheh reservoir (Iran) | water quality variables | NA | NA | Category 2 | No details (6 months) | No details | No details | PSO-ANN | NA |
Hybrid | [ ] | WWTP(China) | DO; COD, BOD, SS | NA | other two variables | Category 3 | No details | daily | No details | SOM-RBFNN, ANN(MLP) | NA |
Hybrid | [ ] | Bangkok canals (Thailand) | DO;; WT, pH, BOD, COD, SS, NH -N, TP, NO , NO , | NA | total coliform, hydrogen sulfide | Category 3 | 13,846 samples (5 years) | monthly | Train: 70%, test: 30% | FCM-MLP, MLP | 1 |
Hybrid | [ ] | Lake Baiyangdian (China) | Chl-a; WT, pH, DO, SD, TP, TN, NH –N, BOD, COD | Precip, Evap | WL, LV, Sth | Category 2 | No details (10 years) | monthly | No details | WANN, ANN, ARIMA | NA |
Hybrid | [ ] | Songhua River (China) | DO, NH -N;; | NA | NA | Category 0 | No details (7 years) | monthly | Train:71%, test: 29% | BWNN, ANN, WANN, ARIMA | 1 |
Hybrid | [ ] | Gazacoastal aquifer (Palestine) | NO ; EC, TDS, NO , | CL, SO , Ca, Mg, Na | Category 2 | No details (10 year) | No details | No details | K-means-ANN | NA | |
Hybrid | [ ] | WWTP (Turkey) | COD; SS, pH, WT | NA | Q | Category 2 | 265 samples (3 years) | daily | Train:50%, validate:25%, test: 25% | k-means-MLP, Arima-RBF, ANN(MLP), MLR, RBFNN, GRNN, ANFIS | NA |
Hybrid | [ ] | Yangtze River (China) | DO, NH -N;; | NA | NA | Category 0 | 480 samples (9 years) | weekly | Train:67%, validate & test: 33% | ARIMA-RBFNN | 1 |
Hybrid | [ ] | Taihu Lake (China) | DO, EC, pH, NH -N, TN, COD, TP, BOD, COD; | NA | VP, petroleum, other 11 variables | Category 2 | 2680 samples | No details | Train:75%, test: 25% | PCA-GA-BPNN | NA |
Hybrid | [ ] | Gauging station (Iran) | DO, WT, S;; Tur, Chl-a | NA | NA | Category 0 and Category 2 and Category 3 | 650, 540 samples | daily, hourly | Train:70%, validate: 15%, test: 15% | WANN, ANN | 1, 2, 3 |
Hybrid | [ ] | Two gauging stations (USA) | SS;; | NA | Q | Category 0 and Category 3 | 1974 samples (8 years) | daily | Train:75%, test: 25% | WANN | NA |
Hybrid | [ ] | River Yamuna (India) | COD;; | NA | NA | Category 0 | 120 samples (10 years) | monthly | Train:92.5%, test: 7.5% | ANN, ANFIS, WANFIS | 9 |
Hybrid | [ ] | Two catchments (Poland) | WT; | AT | Q, declination of the Sun | Category 2 | No details (10 years) | daily | No details | MLP, ANFIS, WNN, Product-Unit ANNs (PUNN), ensemble aggregation approach | 1, 3, 5 |
Hybrid | [ ] | South San Francisco bay (USA) | Chl-a;; | NA | NA | Category 0 | No details (20 years) | monthly | Train:60%, validate: 20%, test: 20% | WANN, MLR, GA-SVR | 1 |
Hybrid | [ ] | Asi River (Turkey) | EC;; | NA | Q | Category 0 and Category 3 | 274 samples (23 years) | No details | Train:75%, test: 25% | WANN, ANN | NA |
Hybrid | [ ] | Klamath River (USA) | DO;; pH, WT, EC, SD | NA | NA | Category 0 and Category 2 | No details | monthly | Train:80%, validate: 10%, test: 10% | WANN, ANN, MLR | NA |
Hybrid | [ ] | Prawn culture ponds (China) | WT; | NA | NA | Category 0 | 1152 samples (8 days) | 10 minutes | Train:87.5%, test: 12.5% | EMD-BPNN, BPNN | 1 |
Hybrid | [ ] | WWTP(China) | BOD; COD, SS, DO, pH | NA | NA | Category 2 | 598 samples (19 months) | daily | No details | Chaos Theory-PCA-ANN | NA |
Hybrid | [ ] | Charlotte harbor marine waters | TN; | NA | NA | Category 0 | No details (13 years) | monthly | Train:70%, validate: 15%, test: 15% | WANN, wavelet-gene expression programing (WGEP), TDNN, GEP, MLR | 1 |
Hybrid | [ ] | Groundwater (Iran) | EC, Tur, pH, NO , NO | NA | Cu | Category 2 | No details (8 years) | No details | Train:80%, test: 20% | PCA-ANN | NA |
Hybrid | [ ] | Downstream (China) | WT, DO, pH, EC, TN, TP, Tur, Chl-a; | NA | NA | Category 0 | No details (13 months) | daily | Train:80%, validate: 10%, test: 10% | Ensemble-ANN | 1 |
Hybrid | [ ] | Karaj River (Iran) | NO ; | NA | CL; Q | Category 0 and Category 1 and Category 3 | No details | monthly | Train:80%, validate: 10%, test: 10% | WANN, ANN, MLR | NA |
Hybrid | [ ] | Crab ponds (China) | DO;; WT | SR, WS, AT, AH | NA | Category 3 | 700 samples (22 days) | 20 minutes | Train:71%, test: 29% | RBFNN-IPSO-LSSVM, BPNN | 3 |
Hybrid | [ ] | Guanting reservoirs (China) | DO, COD, NH -N;; | NA | NA | Category 0 | No details (18 weeks) | weekly | No details | Kalman-BPNN | 2 |
Hybrid | [ ] | Toutle River (USA) | SS;; | NA | Q | Category 0 and Category 3 | 2000 samples (8 years) | daily | No details | A least-square ensemble models-WANN | NA |
Hybrid | [ ] | WWTP (China) | DO; pH | NA | NA | Category 2 | 50 samples | No details | Train:70%, test: 30% | FNN-WNN | NA |
Hybrid | [ ] | Clackamas River (USA) | DO;; WT | NA | Q | Category 3 | 1623 samples (6 years) | daily | Train:78%, test: 22% | WANN, WMLR, ANN(MLP), MLR | 1, 31 |
Hybrid | [ ] | Representative lakes (China) | Chl-a; WT, pH;; NH -N, TN, TP, DO, BOD | NA | other 17 variables | Category 3 | No details (3 years) | No details | Train:80%, test: 20% | GA-BP | NA |
Hybrid | [ ] | Miyun reservoir (China) | DO, COD, NH -N; | NA | NA | Category 0 | 5000 samples (2 years) | weekly | Train:98%, test: 2% | PSO-WNN, WNN, BPNN, SVM | NA |
Hybrid | [ ] | Aji-Chay River (Iran) | EC;; | NA | NA | Category 0 | 315 samples (26 years) | monthly | Train:90%, test: 10% | WA-ELM, ANFIS | 1, 2, 3 |
Hybrid | [ ] | Yangtze River (China) | DO, COD , BOD;; | NA | NA | Category 3 | 65 samples (2 months) | daily | Train:50%, validate: 16%, test: 34% | IABC-BPNN, BPNN | NA |
Hybrid | [ ] | WWTP(China) | COD; COD, SS, pH, NH -N | NA | NA | Category 2 | 250 samples | No details | No details | WANN, ANN(MLP) | NA |
Hybrid | [ ] | The Stream Veszprémi-Séd (Hungary) | pH, EC, DO, Tur;; | NA | NA | Category 2 | No details (7 years) | yearly | No details | DE-ANN | NA |
Hybrid | [ ] | Shrimp pond (China) | DO; WT, NH -N, pH | AT, AH, AP, WS | NA | Category 2 | 2880 samples (20 days) | 10 minutes | Train:75%, test: 25% | SAE-LSTM, SAE-BPNN, LSTM, BPNN | 18, 36, 72 |
Hybrid | [ ] | Four basins (Iran) | TDS; EC | NA | Na, CL | Category 2 | No details (20 years) | No details | Train:80%, test: 20% | WANN, GEP, WANFIS | NA |
Hybrid | [ ] | Blue River (USA) | pH, DO, Tur; WT | NA | Q | Category 0 and Category 3 | No details (4 years) | daily | Train:80%, test: 20% | WANN, WGEP | 1 |
Hybrid | [ ] | Chattahoochee River (USA) | pH;; | NA | Q | Category 3 | 730 samples (2 years) | daily | Train:75%, test: 20% | WANN, ANN, WMLR, MLR | 1, 2, 3 |
Hybrid | [ ] | Morava River Basin (Serbia) | WT, EC; SS, DO | NA | other ions | Category 2 | No details (10 years) | 15 days | No details | PCA-ANN | NA |
Hybrid | [ ] | Tai Lake, Victoria Bay (China) | DO;; WT, pH, NO , TP | Precip | NA | Category 3 | No details (7 years) | No details | Train:80%, test: 20% | IGRA-LSTM, BPNN, ARIMA | NA |
Hybrid | [ ] | WWTP (Saudi Arabia) | C, DO, SS, pH | NA | CL;; | Category 3 | 774 samples | No details | No details | PCA-ELM | NA |
Hybrid | [ ] | Prespa Lake (Greece) | DO, Chl-a;; | NA | NA | Category 0 | 363 samples (11 months) | daily | Train:70%, validate: 15%, test: 15% | CEEMDAN-VMD -ELM) | NA |
Hybrid | [ ] | The Warta River (Poland) | WT;; | AT | NA | Category 3 | No details (22 to 27 years) | daily | Train:4/9, validate: 2/9, test: 1/3 | WANN(MLP), MLP | 1 |
Hybrid | [ ] | Ashi River (China) | DO, NH -N, Tur;; | NA | NA | Category 0 | 846 samples (4 hours) | more than 4 months | Train:70%, test: 30% | IGA-BPNN | 1 |
Hybrid | [ ] | Qiantang River (China) | pH, TP, DO;; | NA | NA | Category 0 | 1448 samples | No details | Train:70%, test: 30% | DS-RNN, RNN, BPNN, SVR | NA |
Hybrid | [ ] | The Johor river (Malaysia) | NH -N, SS, pH; Tur, WT, | NA | COD , Mg, Na | Category 2 | No details (1 year) | No details | No details | WANFIS, MLP, RBFNN, ANFIS | NA |
Hybrid | [ ] | Hilo Bay (the Pacific Ocean) | Chl-a, S;; | NA | NA | Category 0 | No details (5 years) | daily | No details | Bates–Granger (BG)-least square based ensemble (LSE)-WANN | 1, 3, 5 |
Hybrid | [ ] | WWTP (China) | COD, TP, pH, TN; DO, NH -N, BOD, TH | NA | CL, oil-related quality indicators | Category 2 | 23,268 samples (4 years) | hourly | Train:80%, test: 20% | PSO-LSTM | 1 |
Hybrid | [ ] | Beihai Lake (China) | pH, Chl-a, DO, BOD, EC; | NA | HA;; | Category 3 | No details (5 days) | 30 minutes | Train:70%, test: 30% | PSO-GA-BPNN | 12 |
Hybrid | [ ] | River (China) | COD;; | NA | NA | Category 0 | 460 samples (14 months) | 12 hours | Train:95%, test: 5% | LSTM-RNN | 1 |
Hybrid | [ ] | Zhejiang Institute of Freshwater Fisheries (China) | DO; WT | AT, AH, WS, WD, SR, AP | SM, ST | Category 4 | 5006 samples (1 year) | 10 minutes | Train:80%, test: 20% | attention-RNN | 6, 12, 48, 144, 288 |
Hybrid | [ ] | Taihu Lake (China) | pH; DO, COD, NH -N | NA | NA | Category 2 | 28 samples (6 months) | Weekly | Train:75%, test: 25% | grey theory-GRNN, BPNN, RBFNN | 1 |
Emerging | [ ] | Wastewater factory (China) | TP; WT, TSS, pH, NH -N, NO , DO | NA | other 3 variables | Category 2 | 1000 samples (4 months) | No details | Train:80%, test: 20% | SODBN | NA |
Emerging | [ ] | Recirculating Aquaculture Systems (China) | DO;; EC, pH, WT | NA | NA | Category 3 | 4500 samples (13 months) | 10 minutes | Train:67%, validate: 11%, test: 22% | CNN, BPNN | 18 |
- Han, H.G.; Qiao, J.F.; Chen, Q.L. Model predictive control of dissolved oxygen concentration based on a self-organizing RBF neural network. Control Eng. Pract. 2012 , 20 , 465–476. [ Google Scholar ] [ CrossRef ]
- Zheng, F.; Tao, R.; Maier, H.R.; See, L.; Savic, D.; Zhang, T.; Chen, Q.; Assumpção, T.H.; Yang, P.; Heidari, B.; et al. Crowdsourcing Methods for Data Collection in Geophysics: State of the Art, Issues, and Future Directions. Rev. Geophys. 2018 , 56 , 698–740. [ Google Scholar ] [ CrossRef ]
- Liu, S.; Yan, M.; Tai, H.; Xu, L.; Li, D. Prediction of dissolved oxygen content in aquaculture of hyriopsis cumingii using elman neural network. IFIP Adv. Inf. Commun. Technol. 2012 , 370 AICT , 508–518. [ Google Scholar ] [ CrossRef ] [ Green Version ]
- Chen, S.; Fang, G.; Huang, X.; Zhang, Y. Water quality prediction model of a water diversion project based on the improved artificial bee colony-backpropagation neural network. Water 2018 , 10 , 806. [ Google Scholar ] [ CrossRef ] [ Green Version ]
- Fijani, E.; Barzegar, R.; Deo, R.; Tziritis, E.; Konstantinos, S. Design and implementation of a hybrid model based on two-layer decomposition method coupled with extreme learning machines to support real-time environmental monitoring of water quality parameters. Sci. Total Environ. 2019 , 648 , 839–853. [ Google Scholar ] [ CrossRef ] [ PubMed ]
- Wang, Y.; Zhou, J.; Chen, K.; Wang, Y.; Liu, L. Water quality prediction method based on LSTM neural network. In Proceedings of the 2017 12th International Conference on Intelligent Systems and Knowledge Engineering, ISKE 2017, Nanjing, China, 24–26 November 2017. [ Google Scholar ] [ CrossRef ]
- Rajaee, T.; Boroumand, A. Forecasting of chlorophyll-a concentrations in South San Francisco Bay using five different models. Appl. Ocean Res. 2015 , 53 , 208–217. [ Google Scholar ] [ CrossRef ]
- Araghinejad, S. Data-Driven Modeling: Using MATLAB® in Water Resources and Environmental Engineering ; Springer: Berlin, Germany, 2014; ISBN 978-94-007-7505-3. [ Google Scholar ]
- Nourani, V.; Alami, M.T.; Vousoughi, F.D. Self-organizing map clustering technique for ANN-based spatiotemporal modeling of groundwater quality parameters. J. Hydroinformatics 2016 , 18 , 288–309. [ Google Scholar ] [ CrossRef ]
- Zare, A.H.; Bayat, V.M.; Daneshkare, A.P. Forecasting nitrate concentration in groundwater using artificial neural network and linear regression models. Int. Agrophysics 2011 , 25 , 187–192. [ Google Scholar ]
- Huo, S.; He, Z.; Su, J.; Xi, B.; Zhu, C. Using Artificial Neural Network Models for Eutrophication Prediction. Procedia Environ. Sci. 2013 , 18 , 310–316. [ Google Scholar ] [ CrossRef ] [ Green Version ]
- Chang, F.J.; Chen, P.A.; Chang, L.C.; Tsai, Y.H. Estimating spatio-temporal dynamics of stream total phosphate concentration by soft computing techniques. Sci. Total Environ. 2016 , 562 , 228–236. [ Google Scholar ] [ CrossRef ]
- Zhang, G.; Patuwo, B.E.; Hu, M.Y. Forecasting with artificial neural networks: The state of the art. Int. J. Forecast. 1998 , 14 , 35–62. [ Google Scholar ] [ CrossRef ]
- Anmala, J.; Meier, O.W.; Meier, A.J.; Grubbs, S. GIS and artificial neural network-based water quality model for a stream network in the upper green river basin, Kentucky, USA. J. Environ. Eng. 2015 , 141 , 1–15. [ Google Scholar ] [ CrossRef ]
- Li, L.; Jiang, P.; Xu, H.; Lin, G.; Guo, D.; Wu, H. Water quality prediction based on recurrent neural network and improved evidence theory: A case study of Qiantang River, China. Environ. Sci. Pollut. Res. 2019 , 26 , 19879–19896. [ Google Scholar ] [ CrossRef ] [ PubMed ]
- Zhang, L.; Zou, Z.; Shan, W. Development of a method for comprehensive water quality forecasting and its application in Miyun reservoir of Beijing, China. J. Environ. Sci. 2017 , 56 , 240–246. [ Google Scholar ] [ CrossRef ]
- Seo, I.W.; Yun, S.H.; Choi, S.Y. Forecasting Water Quality Parameters by ANN Model Using Pre-processing Technique at the Downstream of Cheongpyeong Dam. Procedia Eng. 2016 , 154 , 1110–1115. [ Google Scholar ] [ CrossRef ] [ Green Version ]
- Heddam, S. Modelling hourly dissolved oxygen concentration (DO) using dynamic evolving neural-fuzzy inference system (DENFIS)-based approach: Case study of Klamath River at Miller Island Boat Ramp, OR, USA. Environ. Sci. Pollut. Res. 2014 , 21 , 9212–9227. [ Google Scholar ] [ CrossRef ]
- Wang, T.S.; Tan, C.H.; Chen, L.; Tsai, Y.C. Applying artificial neural networks and remote sensing to estimate chlorophyll-a concentration in water body. In Proceedings of the 2008 2nd International Sympoisum Intelligent Information Technology Application IITA, Shanghai, China, 20–22 December 2008; pp. 540–544. [ Google Scholar ] [ CrossRef ]
- Maier, H.R.; Dandy, G.C. Neural networks for the prediction and forecasting of water resources variables: A review of modelling issues and applications. Environ. Model. Softw. 2000 , 15 , 101–124. [ Google Scholar ] [ CrossRef ]
- Loke, E.; Warnaars, E.A.; Jacobsen, P.; Nelen, F.; Do Céu Almeida, M. Artificial neural networks as a tool in urban storm drainage. Water Sci. Technol. 1997 , 36 , 101–109. [ Google Scholar ] [ CrossRef ]
- Tota-Maharaj, K.; Scholz, M. Artificial neural network simulation of combined permeable pavement and earth energy systems treating storm water. J. Environ. Eng. 2012 , 138 , 499–509. [ Google Scholar ] [ CrossRef ]
- Nour, M.H.; Smith, D.W.; El-Din, M.G.; Prepas, E.E. The application of artificial neural networks to flow and phosphorus dynamics in small streams on the Boreal Plain, with emphasis on the role of wetlands. Ecol. Modell. 2006 , 191 , 19–32. [ Google Scholar ] [ CrossRef ]
- Csábrági, A.; Molnár, S.; Tanos, P.; Kovács, J. Application of artificial neural networks to the forecasting of dissolved oxygen content in the Hungarian section of the river Danube. Ecol. Eng. 2017 , 100 , 63–72. [ Google Scholar ] [ CrossRef ]
- Šiljić Tomić, A.N.; Antanasijević, D.Z.; Ristić, M.; Perić-Grujić, A.A.; Pocajt, V.V. Modeling the BOD of Danube River in Serbia using spatial, temporal, and input variables optimized artificial neural network models. Environ. Monit. Assess. 2016 , 188 . [ Google Scholar ] [ CrossRef ] [ PubMed ]
- Ye, Q.; Yang, X.; Chen, C.; Wang, J. River Water Quality Parameters Prediction Method Based on LSTM-RNN Model. In Proceedings of the 2019 Chinese Control and Decision Conference CCDC, Nanchang, China, 3–5 June 2019; pp. 3024–3028. [ Google Scholar ] [ CrossRef ]
- Maier, H.R.; Jain, A.; Dandy, G.C.; Sudheer, K.P. Methods used for the development of neural networks for the prediction of water resource variables in river systems: Current status and future directions. Environ. Model. Softw. 2010 , 25 , 891–909. [ Google Scholar ] [ CrossRef ]
- Pu, F.; Ding, C.; Chao, Z.; Yu, Y.; Xu, X. Water-quality classification of inland lakes using Landsat8 images by convolutional neural networks. Remote Sens. 2019 , 11 , 1674. [ Google Scholar ] [ CrossRef ] [ Green Version ]
- Nourani, V.; Hosseini Baghanam, A.; Adamowski, J.; Kisi, O. Applications of hybrid wavelet-Artificial Intelligence models in hydrology: A review. J. Hydrol. 2014 , 514 , 358–377. [ Google Scholar ] [ CrossRef ]
- Wu, W.; Dandy, G.C.; Maier, H.R. Protocol for developing ANN models and its application to the assessment of the quality of the ANN model development process in drinking water quality modelling. Environ. Model. Softw. 2014 , 54 , 108–127. [ Google Scholar ] [ CrossRef ]
- Cabaneros, S.M.; Calautit, J.K.; Hughes, B.R. A review of artificial neural network models for ambient air pollution prediction. Environ. Model. Softw. 2019 , 119 , 285–304. [ Google Scholar ] [ CrossRef ]
- Elkiran, G.; Nourani, V.; Abba, S.I. Multi-step ahead modelling of river water quality parameters using ensemble artificial intelligence-based approach. J. Hydrol. 2019 , 577 , 123962. [ Google Scholar ] [ CrossRef ]
- Cong, Q.; Yu, W. Integrated soft sensor with wavelet neural network and adaptive weighted fusion for water quality estimation in wastewater treatment process. Measurement 2018 , 124 , 436–446. [ Google Scholar ] [ CrossRef ]
- Humphrey, G.B.; Maier, H.R.; Wu, W.; Mount, N.J.; Dandy, G.C.; Abrahart, R.J.; Dawson, C.W. Improved validation framework and R-package for artificial neural network models. Environ. Model. Softw. 2017 , 92 , 82–106. [ Google Scholar ] [ CrossRef ] [ Green Version ]
- Iglesias, C.; Martínez Torres, J.; García Nieto, P.J.; Alonso Fernández, J.R.; Díaz Muñiz, C.; Piñeiro, J.I.; Taboada, J. Turbidity Prediction in a River Basin by Using Artificial Neural Networks: A Case Study in Northern Spain. Water Resour. Manag. 2014 , 28 , 319–331. [ Google Scholar ] [ CrossRef ]
- Gholamreza, A.; Afshin, M.-D.; Shiva, H.A.; Nasrin, R. Application of artificial neural networks to predict total dissolved solids in the river Zayanderud, Iran. Environ. Eng. Res. 2016 , 21 , 333–340. [ Google Scholar ] [ CrossRef ] [ Green Version ]
- Najah, A.; El-Shafie, A.; Karim, O.A.; El-Shafie, A.H. Application of artificial neural networks for water quality prediction. Neural Comput. Appl. 2012 , 22 , 187–201. [ Google Scholar ] [ CrossRef ]
- Zhao, J.; Zhao, C.; Zhang, F.; Wu, G.; Wang, H. Water Quality Prediction in the Waste Water Treatment Process Based on Ridge Regression Echo State Network. IOP Conf. Ser. Mater. Sci. Eng. 2018 , 435 . [ Google Scholar ] [ CrossRef ] [ Green Version ]
- Zhai, W.; Zhou, X.; Man, J.; Xu, Q.; Jiang, Q.; Yang, Z.; Jiang, L.; Gao, Z.; Yuan, Y.; Gao, W. Prediction of water quality based on artificial neural network with grey theory. IOP Conf. Ser. Earth Environ. Sci. 2019 , 295 . [ Google Scholar ] [ CrossRef ]
- Palani, S.; Liong, S.Y.; Tkalich, P. An ANN application for water quality forecasting. Mar. Pollut. Bull. 2008 , 56 , 1586–1597. [ Google Scholar ] [ CrossRef ] [ PubMed ]
- Antanasijević, D.; Pocajt, V.; Povrenović, D.; Perić-Grujić, A.; Ristić, M. Modelling of dissolved oxygen content using artificial neural networks: Danube River, North Serbia, case study. Environ. Sci. Pollut. Res. 2013 , 20 , 9006–9013. [ Google Scholar ] [ CrossRef ]
- Aleksandra, Š.; Antanasijevi, D. Perić-Grujić, A.; Ristić, M.; Pocajt, V. Artificial neural network modelling of biological oxygen demand in rivers at the national level with input selection based on Monte Carlo simulations. Environ. Sci. Pollut. Res. 2015 , 22 , 4230–4241. [ Google Scholar ] [ CrossRef ]
- Ay, M.; Kisi, O. Modelling of chemical oxygen demand by usinAg ANNs, ANFIS and k-means clustering techniques. J. Hydrol. 2014 , 511 , 279–289. [ Google Scholar ] [ CrossRef ]
- Qiao, J.; Hu, Z.; Li, W. Soft measurement modeling based on chaos theory for biochemical oxygen demand (BOD). Water 2016 , 8 , 581. [ Google Scholar ] [ CrossRef ] [ Green Version ]
- Liu, Y.; Zhang, Q.; Song, L.; Chen, Y. Attention-based recurrent neural networks for accurate short-term and long-term dissolved oxygen prediction. Comput. Electron. Agric. 2019 , 165 , 104964. [ Google Scholar ] [ CrossRef ]
- Djerioui, M.; Bouamar, M.; Ladjal, M.; Zerguine, A. Chlorine Soft Sensor Based on Extreme Learning Machine for Water Quality Monitoring. Arab. J. Sci. Eng. 2019 , 44 , 2033–2044. [ Google Scholar ] [ CrossRef ]
- Heddam, S.; Kisi, O. Extreme learning machines: A new approach for modeling dissolved oxygen (DO) concentration with and without water quality variables as predictors. Environ. Sci. Pollut. Res. 2017 , 24 , 16702–16724. [ Google Scholar ] [ CrossRef ] [ PubMed ]
- Zhu, S.; Heddam, S.; Wu, S.; Dai, J.; Jia, B. Extreme learning machine-based prediction of daily water temperature for rivers. Environ. Earth Sci. 2019 , 78 , 1–17. [ Google Scholar ] [ CrossRef ]
- Elbisy, M.S.; Ali, H.M.; Abd-Elall, M.A.; Alaboud, T.M. The use of feed-forward back propagation and cascade correlation for the neural network prediction of surface water quality parameters. Water Resour. 2014 , 41 , 709–718. [ Google Scholar ] [ CrossRef ]
- Baek, G.; Cheon, S.P.; Kim, S.; Kim, Y.; Kim, H.; Kim, C.; Kim, S. Modular neural networks prediction model based A 2/O process control system. Int. J. Precis. Eng. Manuf. 2012 , 13 , 905–913. [ Google Scholar ] [ CrossRef ]
- Ding, D.; Zhang, M.; Pan, X.; Yang, M.; He, X. Modeling extreme events in time series prediction. In Proceedings of the ACM SIGKDD International Conference Knowledge Discovery & Data Mining, Anchorage, AK, USA, 4–8 August 2019; Volume 1, pp. 1114–1122. [ Google Scholar ] [ CrossRef ]
- Khani, S.; Rajaee, T. Modeling of Dissolved Oxygen Concentration and Its Hysteresis Behavior in Rivers Using Wavelet Transform-Based Hybrid Models. Clean-Soil Air Water 2017 , 45 . [ Google Scholar ] [ CrossRef ]
- Lim, H.; An, H.; Kim, H.; Lee, J. Prediction of pollution loads in the Geum River upstream using the recurrent neural network algorithm. Korean J. Agrcultural Sci. 2019 , 46 , 67–78. [ Google Scholar ] [ CrossRef ]
- Li, Z.; Peng, F.; Niu, B.; Li, G.; Wu, J.; Miao, Z. Water Quality Prediction Model Combining Sparse Auto-encoder and LSTM Network. IFAC-PapersOnLine 2018 , 51 , 831–836. [ Google Scholar ] [ CrossRef ]
- Evrendilek, F.; Karakaya, N. Monitoring diel dissolved oxygen dynamics through integrating wavelet denoising and temporal neural networks. Environ. Monit. Assess. 2014 , 186 , 1583–1591. [ Google Scholar ] [ CrossRef ]
- Wang, F.; Wang, X.; Chen, B.; Zhao, Y.; Yang, Z. Chlorophyll a simulation in a lake ecosystem using a model with wavelet analysis and artificial neural network. Environ. Manag. 2013 , 51 , 1044–1054. [ Google Scholar ] [ CrossRef ] [ PubMed ]
- Ta, X.; Wei, Y. Research on a dissolved oxygen prediction method for recirculating aquaculture systems based on a convolution neural network. Comput. Electron. Agric. 2018 , 145 , 302–310. [ Google Scholar ] [ CrossRef ]
- Qiao, J.; Wang, G.; Li, X.; Li, W. A self-organizing deep belief network for nonlinear system modeling. Appl. Soft Comput. J. 2018 , 65 , 170–183. [ Google Scholar ] [ CrossRef ]
- Sharaf El Din, E.; Zhang, Y.; Suliman, A. Mapping concentrations of surface water quality parameters using a novel remote sensing and artificial intelligence framework. Int. J. Remote Sens. 2017 , 38 , 1023–1042. [ Google Scholar ] [ CrossRef ]
- Hameed, M.; Sharqi, S.S.; Yaseen, Z.M.; Afan, H.A.; Hussain, A.; Elshafie, A. Application of artificial intelligence (AI) techniques in water quality index prediction: A case study in tropical region, Malaysia. Neural Comput. Appl. 2017 , 28 , 893–905. [ Google Scholar ] [ CrossRef ]
- Zhang, Y.F.; Fitch, P.; Thorburn, P.J. Predicting the trend of dissolved oxygen based on the kPCA-RNN model. Water 2020 , 12 , 585. [ Google Scholar ] [ CrossRef ] [ Green Version ]
- Alizadeh, M.J.; Kavianpour, M.R. Development of wavelet-ANN models to predict water quality parameters in Hilo Bay, Pacific Ocean. Mar. Pollut. Bull. 2015 , 98 , 171–178. [ Google Scholar ] [ CrossRef ]
- Zounemat-Kermani, M.; Seo, Y.; Kim, S.; Ghorbani, M.A.; Samadianfard, S.; Naghshara, S.; Kim, N.W.; Singh, V.P. Can decomposition approaches always enhance soft computing models? Predicting the dissolved oxygen concentration in the St. Johns River, Florida. Appl. Sci. 2019 , 9 , 2534. [ Google Scholar ] [ CrossRef ] [ Green Version ]
- Wang, Y.; Zheng, T.; Zhao, Y.; Jiang, J.; Wang, Y.; Guo, L.; Wang, P. Monthly water quality forecasting and uncertainty assessment via bootstrapped wavelet neural networks under missing data for Harbin, China. Environ. Sci. Pollut. Res. 2013 , 20 , 8909–8923. [ Google Scholar ] [ CrossRef ]
- Lee, K.J.; Yun, S.T.; Yu, S.; Kim, K.H.; Lee, J.H.; Lee, S.H. The combined use of self-organizing map technique and fuzzy c-means clustering to evaluate urban groundwater quality in Seoul metropolitan city, South Korea. J. Hydrol. 2019 , 569 , 685–697. [ Google Scholar ] [ CrossRef ]
- Hu, Z.; Zhang, Y.; Zhao, Y.; Xie, M.; Zhong, J.; Tu, Z.; Liu, J. A water quality prediction method based on the deep LSTM network considering correlation in smart mariculture. Sensors 2019 , 19 , 1420. [ Google Scholar ] [ CrossRef ] [ PubMed ] [ Green Version ]
- Liu, J.; Yu, C.; Hu, Z.; Zhao, Y.; Xia, X.; Tu, Z.; Li, R. Automatic and accurate prediction of key water quality parameters based on SRU deep learning in mariculture. In Proceedings of the 2018 IEEE International Conference on Advanced Manufacturing ICAM 2018, Yunlin, Taiwan, 16–18 November 2018; pp. 437–440. [ Google Scholar ] [ CrossRef ]
- Yan, J.; Xu, Z.; Yu, Y.; Xu, H.; Gao, K. Application of a hybrid optimized bp network model to estimatewater quality parameters of Beihai Lake in Beijing. Appl. Sci. 2019 , 9 , 1863. [ Google Scholar ] [ CrossRef ] [ Green Version ]
- Huang, M.; Zhang, T.; Ruan, J.; Chen, X. A New Efficient Hybrid Intelligent Model for Biodegradation Process of DMP with Fuzzy Wavelet Neural Networks. Sci. Rep. 2017 , 7 , 1–9. [ Google Scholar ] [ CrossRef ] [ PubMed ] [ Green Version ]
- Deng, W.; Wang, G.; Zhang, X.; Guo, Y.; Li, G. Water quality prediction based on a novel hybrid model of ARIMA and RBF neural network. In Proceedings of the 2014 IEEE 3rd International Conference Cloud Computing Intelligence System, Shenzhen, China, 27–29 November 2014; pp. 33–40. [ Google Scholar ] [ CrossRef ]
- Ömer Faruk, D. A hybrid neural network and ARIMA model for water quality time series prediction. Eng. Appl. Artif. Intell. 2010 , 23 , 586–594. [ Google Scholar ] [ CrossRef ]
- Ravansalar, M.; Rajaee, T. Evaluation of wavelet performance via an ANN-based electrical conductivity prediction model. Environ. Monit. Assess. 2015 , 187 . [ Google Scholar ] [ CrossRef ]
- Sakizadeh, M.; Malian, A.; Ahmadpour, E. Groundwater Quality Modeling with a Small Data Set. Groundwater 2016 , 54 , 115–120. [ Google Scholar ] [ CrossRef ]
- Lin, Q.; Yang, W.; Zheng, C.; Lu, K.; Zheng, Z.; Wang, J.; Zhu, J. Deep-learning based approach for forecast of water quality in intensive shrimp ponds. Indian J. Fish. 2018 , 65 , 75–80. [ Google Scholar ] [ CrossRef ] [ Green Version ]
- Dogan, E.; Ates, A.; Yilmaz, C.; Eren, B. Application of Artificial Neural Networks to Estimate Wastewater Treatment Plant Inlet Biochemical Oxygen Demand. Environ. Prog. 2008 , 27 , 439–446. [ Google Scholar ] [ CrossRef ]
- Elhatip, H.; Kömür, M.A. Evaluation of water quality parameters for the Mamasin dam in Aksaray City in the central Anatolian part of Turkey by means of artificial neural networks. Environ. Geol. 2008 , 53 , 1157–1164. [ Google Scholar ] [ CrossRef ]
- Al-Mahallawi, K.; Mania, J.; Hani, A.; Shahrour, I. Using of neural networks for the prediction of nitrate groundwater contamination in rural and agricultural areas. Environ. Earth Sci. 2012 , 65 , 917–928. [ Google Scholar ] [ CrossRef ]
- Hong, Y.S.T. Dynamic nonlinear state-space model with a neural network via improved sequential learning algorithm for an online real-time hydrological modeling. J. Hydrol. 2012 , 468–469 , 11–21. [ Google Scholar ] [ CrossRef ]
- Bayram, A.; Kankal, M.; Önsoy, H. Estimation of suspended sediment concentration from turbidity measurements using artificial neural networks. Environ. Monit. Assess. 2012 , 184 , 4355–4365. [ Google Scholar ] [ CrossRef ] [ PubMed ]
- Bayram, A.; Kankal, M.; Tayfur, G.; Önsoy, H. Prediction of suspended sediment concentration from water quality variables. Neural Comput. Appl. 2014 , 24 , 1079–1087. [ Google Scholar ] [ CrossRef ] [ Green Version ]
- Tabari, H.; Talaee, P.H. Reconstruction of river water quality missing data using artificial neural networks. Water Qual. Res. J. Canada 2015 , 50 , 326–335. [ Google Scholar ] [ CrossRef ]
- Zounemat-Kermani, M.; Kişi, Ö.; Adamowski, J.; Ramezani-Charmahineh, A. Evaluation of data driven models for river suspended sediment concentration modeling. J. Hydrol. 2016 , 535 , 457–472. [ Google Scholar ] [ CrossRef ]
- Olyaie, E.; Zare Abyaneh, H.; Danandeh Mehr, A. A comparative analysis among computational intelligence techniques for dissolved oxygen prediction in Delaware River. Geosci. Front. 2017 , 8 , 517–527. [ Google Scholar ] [ CrossRef ] [ Green Version ]
- Ostad-Ali-Askari, K.; Shayannejad, M.; Ghorbanizadeh-Kharazi, H. Artificial neural network for modeling nitrate pollution of groundwater in marginal area of Zayandeh-rood River, Isfahan, Iran. KSCE J. Civ. Eng. 2017 , 21 , 134–140. [ Google Scholar ] [ CrossRef ]
- Alagha, J.S.; Seyam, M.; Md Said, M.A.; Mogheir, Y. Integrating an artificial intelligence approach with k-means clustering to model groundwater salinity: The case of Gaza coastal aquifer (Palestine). Hydrogeol. J. 2017 , 25 , 2347–2361. [ Google Scholar ] [ CrossRef ]
- Miller, T.; Poleszczuk, G. Prediction of the Seasonal Changes of the Chloride Concentrations in Urban Water Reservoir. Ecol. Chem. Eng. S 2017 , 24 , 595–611. [ Google Scholar ] [ CrossRef ] [ Green Version ]
- Graf, R.; Zhu, S.; Sivakumar, B. Forecasting river water temperature time series using a wavelet–neural network hybrid modelling approach. J. Hydrol. 2019 , 578 . [ Google Scholar ] [ CrossRef ]
- Luo, W.; Zhu, S.; Wu, S.; Dai, J. Comparing artificial intelligence techniques for chlorophyll-a prediction in US lakes. Environ. Sci. Pollut. Res. 2019 , 26 , 30524–30532. [ Google Scholar ] [ CrossRef ] [ PubMed ]
- Najah Ahmed, A.; Binti Othman, F.; Abdulmohsin Afan, H.; Khaleel Ibrahim, R.; Ming Fai, C.; Shabbir Hossain, M.; Ehteram, M.; Elshafie, A. Machine learning methods for better water quality prediction. J. Hydrol. 2019 , 578 . [ Google Scholar ] [ CrossRef ]
- Yeon, I.S.; Kim, J.H.; Jun, K.W. Application of artificial intelligence models in water quality forecasting. Environ. Technol. 2008 , 29 , 625–631. [ Google Scholar ] [ CrossRef ] [ PubMed ]
- Dogan, E.; Sengorur, B.; Koklu, R. Modeling biological oxygen demand of the Melen River in Turkey using an artificial neural network technique. J. Environ. Manag. 2009 , 90 , 1229–1235. [ Google Scholar ] [ CrossRef ] [ PubMed ]
- Miao, Q.; Yuan, H.; Shao, C.; Liu, Z. Water quality prediction of moshui river in china based on BP neural network. In Proceedings of the 2009 International Conference Computing Intelligent Natural Computing CINC, Wuhan, China, 6–7 June 2009; pp. 7–10. [ Google Scholar ] [ CrossRef ]
- Oliveira Souza da Costa, A.; Ferreira Silva, P.; Godoy Sabará, M.; Ferreira da Costa, E. Use of neural networks for monitoring surface water quality changes in a neotropical urban stream. Environ. Monit. Assess. 2009 , 155 , 527–538. [ Google Scholar ] [ CrossRef ] [ PubMed ]
- Shen, X.; Chen, M.; Yu, J. Water environment monitoring system based on neural networks for shrimp cultivation. In Proceedings of the 2009 International Conference Artifitial Intelligence and Computional Intelligence AICI, Shanghai, China, 7–8 November 2009; pp. 427–431. [ Google Scholar ] [ CrossRef ]
- Singh, K.P.; Basant, A.; Malik, A.; Jain, G. Artificial neural network modeling of the river water quality—A case study. Ecol. Modell. 2009 , 220 , 888–895. [ Google Scholar ] [ CrossRef ]
- Yeon, I.S.; Jun, K.W.; Lee, H.J. The improvement of total organic carbon forecasting using neural networks discharge model. Environ. Technol. 2009 , 30 , 45–51. [ Google Scholar ] [ CrossRef ] [ Green Version ]
- Zuo, J.; Yu, J.T. Application of neural network in groundwater denitrification process. In Proceedings of the 2009 Asia-Pacific Conference Information Processing APCIP, Shenzhen, China, 18–19 July 2009; pp. 79–82. [ Google Scholar ] [ CrossRef ]
- Akkoyunlu, A.; Akiner, M.E. Feasibility Assessment of Data-Driven Models in Predicting Pollution Trends of Omerli Lake, Turkey. Water Resour. Manag. 2010 , 24 , 3419–3436. [ Google Scholar ] [ CrossRef ]
- Chen, D.; Lu, J.; Shen, Y. Artificial neural network modelling of concentrations of nitrogen, phosphorus and dissolved oxygen in a non-point source polluted river in Zhejiang Province, southeast China. Hydrol. Process. 2010 , 24 , 290–299. [ Google Scholar ] [ CrossRef ]
- Piotrowski, A.P.; Napiorkowski, M.J.; Napiorkowski, J.J.; Osuch, M. Comparing various artificial neural network types for water temperature prediction in rivers. J. Hydrol. 2015 , 529 , 302–315. [ Google Scholar ] [ CrossRef ]
- Alizadeh, M.J.; Jafari Nodoushan, E.; Kalarestaghi, N.; Chau, K.W. Toward multi-day-ahead forecasting of suspended sediment concentration using ensemble models. Environ. Sci. Pollut. Res. 2017 , 24 , 28017–28025. [ Google Scholar ] [ CrossRef ] [ PubMed ]
- Šiljić Tomić, A.; Antanasijević, D.; Ristić, M.; Perić-Grujić, A.; Pocajt, V. Application of experimental design for the optimization of artificial neural network-based water quality model: A case study of dissolved oxygen prediction. Environ. Sci. Pollut. Res. 2018 , 25 , 9360–9370. [ Google Scholar ] [ CrossRef ] [ PubMed ]
- Shamshirband, S.; Jafari Nodoushan, E.; Adolf, J.E.; Abdul Manaf, A.; Mosavi, A.; Chau, K. Ensemble models with uncertainty analysis for multi-day ahead forecasting of chlorophyll a concentration in coastal waters. Eng. Appl. Comput. Fluid Mech. 2019 , 13 , 91–101. [ Google Scholar ] [ CrossRef ] [ Green Version ]
- Rajaee, T.; Benmaran, R.R. Prediction of water quality parameters (NO 3 , CL) in Karaj river by using a combination of Wavelet Neural Network, ANN and MLR models. J. Water Soil 2016 , 30 , 15–29. [ Google Scholar ] [ CrossRef ]
- Markus, M.; Hejazi, M.I.; Bajcsy, P.; Giustolisi, O.; Savic, D.A. Prediction of weekly nitrate-N fluctuations in a small agricultural watershed in Illinois. J. Hydroinformatics 2010 , 12 , 251–261. [ Google Scholar ] [ CrossRef ]
- Merdun, H.; Çinar, Ö. Utilization of two artificial neural network methods in surface water quality modeling. Environ. Eng. Manag. J. 2010 , 9 , 413–421. [ Google Scholar ] [ CrossRef ]
- Ranković, V.; Radulović, J.; Radojević, I.; Ostojić, A.; Čomić, L. Neural network modeling of dissolved oxygen in the Gruža reservoir, Serbia. Ecol. Modell. 2010 , 221 , 1239–1244. [ Google Scholar ] [ CrossRef ]
- Zhu, X.; Li, D.; He, D.; Wang, J.; Ma, D.; Li, F. A remote wireless system for water quality online monitoring in intensive fish culture. Comput. Electron. Agric. 2010 , 71 , S3. [ Google Scholar ] [ CrossRef ]
- Banerjee, P.; Singh, V.S.; Chatttopadhyay, K.; Chandra, P.C.; Singh, B. Artificial neural network model as a potential alternative for groundwater salinity forecasting. J. Hydrol. 2011 , 398 , 212–220. [ Google Scholar ] [ CrossRef ]
- Han, H.G.; Chen, Q.L.; Qiao, J.F. An efficient self-organizing RBF neural network for water quality prediction. Neural Netw. 2011 , 24 , 717–725. [ Google Scholar ] [ CrossRef ]
- Asadollahfardi, G.; Taklify, A.; Ghanbari, A. Application of Artificial Neural Network to Predict TDS in Talkheh Rud River. J. Irrig. Drain. Eng. 2012 , 138 , 363–370. [ Google Scholar ] [ CrossRef ]
- Ay, M.; Kisi, O. Modeling of dissolved oxygen concentration using different neural network techniques in Foundation Creek, El Paso County, Colorado. J. Environ. Eng. 2012 , 138 , 654–662. [ Google Scholar ] [ CrossRef ]
- Gazzaz, N.M.; Yusoff, M.K.; Aris, A.Z.; Juahir, H.; Ramli, M.F. Artificial neural network modeling of the water quality index for Kinta River (Malaysia) using water quality variables as predictors. Mar. Pollut. Bull. 2012 , 64 , 2409–2420. [ Google Scholar ] [ CrossRef ] [ PubMed ]
- Liu, W.C.; Chen, W.B. Prediction of water temperature in a subtropical subalpine lake using an artificial neural network and three-dimensional circulation models. Comput. Geosci. 2012 , 45 , 13–25. [ Google Scholar ] [ CrossRef ]
- Kakaei Lafdani, E.; Moghaddam Nia, A.; Ahmadi, A. Daily suspended sediment load prediction using artificial neural networks and support vector machines. J. Hydrol. 2013 , 478 , 50–62. [ Google Scholar ] [ CrossRef ]
- Karakaya, N.; Evrendilek, F.; Gungor, K.; Onal, D. Predicting diel, diurnal and nocturnal dynamics of dissolved oxygen and chlorophyll-a using regression models and neural networks. Clean-Soil Air Water 2013 , 41 , 872–877. [ Google Scholar ] [ CrossRef ]
- Antanasijević, D.; Pocajt, V.; Perić-Grujić, A.; Ristić, M. Modelling of dissolved oxygen in the danube river using artificial neural networks and Monte carlo simulation uncertainty analysis. J. Hydrol. 2014 , 519 , 1895–1907. [ Google Scholar ] [ CrossRef ]
- Chen, W.B.; Liu, W.C. Artificial neural network modeling of dissolved oxygen in reservoir. Environ. Monit. Assess. 2014 , 186 , 1203–1217. [ Google Scholar ] [ CrossRef ]
- Han, H.G.; Wang, L.D.; Qiao, J.F. Hierarchical extreme learning machine for feedforward neural network. Neurocomputing 2014 , 128 , 128–135. [ Google Scholar ] [ CrossRef ]
- Ding, Y.R.; Cai, Y.J.; Sun, P.D.; Chen, B. The use of combined neural networks and genetic algorithms for prediction of river water quality. J. Appl. Res. Technol. 2014 , 12 , 493–499. [ Google Scholar ] [ CrossRef ]
- Faramarzi, M.; Yunus, M.A.M.; Nor, A.S.M.; Ibrahim, S. The application of the Radial Basis Function Neural Network in estimation of nitrate contamination in Manawatu river. In Proceedings of the 2014 International Conference Computional Science Technology ICCST, Kota Kinabalu, Malaysia, 27–28 August 2014; pp. 1–5. [ Google Scholar ] [ CrossRef ]
- Nemati, S.; Fazelifard, M.H.; Terzi, Ö.; Ghorbani, M.A. Estimation of dissolved oxygen using data-driven techniques in the Tai Po River, Hong Kong. Environ. Earth Sci. 2015 , 74 , 4065–4073. [ Google Scholar ] [ CrossRef ]
- Li, X.; Sha, J.; Wang, Z.L. Chlorophyll-A Prediction of lakes with different water quality patterns in China based on hybrid neural networks. Water 2017 , 9 , 524. [ Google Scholar ] [ CrossRef ] [ Green Version ]
- Montaseri, M.; Zaman Zad Ghavidel, S.; Sanikhani, H. Water quality variations in different climates of Iran: Toward modeling total dissolved solid using soft computing techniques. Stoch. Environ. Res. Risk Assess. 2018 , 32 , 2253–2273. [ Google Scholar ] [ CrossRef ]
- Rajaee, T.; Jafari, H. Utilization of WGEP and WDT models by wavelet denoising to predict water quality parameters in rivers. J. Hydrol. Eng. 2018 , 23 . [ Google Scholar ] [ CrossRef ]
- Barzegar, R.; Asghari Moghaddam, A.; Adamowski, J.; Ozga-Zielinski, B. Multi-step water quality forecasting using a boosting ensemble multi-wavelet extreme learning machine model. Stoch. Environ. Res. Risk Assess. 2018 , 32 , 799–813. [ Google Scholar ] [ CrossRef ]
- Csábrági, A.; Molnár, S.; Tanos, P.; Kovács, J.; Molnár, M.; Szabó, I.; Hatvani, I.G. Estimation of dissolved oxygen in riverine ecosystems: Comparison of differently optimized neural networks. Ecol. Eng. 2019 , 138 , 298–309. [ Google Scholar ] [ CrossRef ]
- Klçaslan, Y.; Tuna, G.; Gezer, G.; Gulez, K.; Arkoc, O.; Potirakis, S.M. ANN-based estimation of groundwater quality using a wireless water quality network. Int. J. Distrib. Sens. Netw. 2014 , 2014 , 1–8. [ Google Scholar ] [ CrossRef ] [ Green Version ]
- Yang, T.M.; Fan, S.K.; Fan, C.; Hsu, N.S. Establishment of turbidity forecasting model and early-warning system for source water turbidity management using back-propagation artificial neural network algorithm and probability analysis. Environ. Monit. Assess. 2014 , 186 , 4925–4934. [ Google Scholar ] [ CrossRef ]
- Khashei-Siuki, A.; Sarbazi, M. Evaluation of ANFIS, ANN, and geostatistical models to spatial distribution of groundwater quality (case study: Mashhad plain in Iran). Arab. J. Geosci. 2015 , 8 , 903–912. [ Google Scholar ] [ CrossRef ]
- Yousefi, P.; Naser, G.; Mohammadi, H. Surface water quality model: Impacts of influential variables. J. Water Resour. Plan. Manag. 2018 , 144 , 1–10. [ Google Scholar ] [ CrossRef ]
- Najah, A.; El-Shafie, A.; Karim, O.A.; El-Shafie, A.H. Performance of ANFIS versus MLP-NN dissolved oxygen prediction models in water quality monitoring. Environ. Sci. Pollut. Res. 2014 , 21 , 1658–1670. [ Google Scholar ] [ CrossRef ] [ PubMed ]
- Sinshaw, T.A.; Surbeck, C.Q.; Yasarer, H.; Najjar, Y. Artificial Neural Network for Prediction of Total Nitrogen and Phosphorus in US Lakes. J. Environ. Eng. 2019 , 145 , 1–11. [ Google Scholar ] [ CrossRef ]
- Partal, T.; Cigizoglu, H.K. Estimation and forecasting of daily suspended sediment data using wavelet-neural networks. J. Hydrol. 2008 , 358 , 317–331. [ Google Scholar ] [ CrossRef ]
- Anctil, F.; Filion, M.; Tournebize, J. A neural network experiment on the simulation of daily nitrate-nitrogen and suspended sediment fluxes from a small agricultural catchment. Ecol. Modell. 2009 , 220 , 879–887. [ Google Scholar ] [ CrossRef ] [ Green Version ]
- Alagha, J.S.; Said, M.A.M.; Mogheir, Y. Modeling of nitrate concentration in groundwater using artificial intelligence approach-a case study of Gaza coastal aquifer. Environ. Monit. Assess. 2014 , 186 , 35–45. [ Google Scholar ] [ CrossRef ]
- Salami, E.S.; Ehteshami, M. Simulation, evaluation and prediction modeling of river water quality properties (case study: Ireland Rivers). Int. J. Environ. Sci. Technol. 2015 , 12 , 3235–3242. [ Google Scholar ] [ CrossRef ] [ Green Version ]
- Ahmed, A.A.M. Prediction of dissolved oxygen in Surma River by biochemical oxygen demand and chemical oxygen demand using the artificial neural networks (ANNs). J. King Saud Univ. Eng. Sci. 2017 , 29 , 151–158. [ Google Scholar ] [ CrossRef ] [ Green Version ]
- Najafzadeh, M.; Ghaemi, A. Prediction of the five-day biochemical oxygen demand and chemical oxygen demand in natural streams using machine learning methods. Environ. Monit. Assess. 2019 , 191 . [ Google Scholar ] [ CrossRef ]
- Sahoo, G.B.; Schladow, S.G.; Reuter, J.E. Forecasting stream water temperature using regression analysis, artificial neural network, and chaotic non-linear dynamic models. J. Hydrol. 2009 , 378 , 325–342. [ Google Scholar ] [ CrossRef ]
- Wu, M.; Zhang, W.; Wang, X.; Luo, D. Application of MODIS satellite data in monitoring water quality parameters of Chaohu Lake in China. Environ. Monit. Assess. 2009 , 148 , 255–264. [ Google Scholar ] [ CrossRef ]
- Kişi, Ö. River suspended sediment concentration modeling using a neural differential evolution approach. J. Hydrol. 2010 , 389 , 227–235. [ Google Scholar ] [ CrossRef ]
- Afshar, A.; Kazemi, H. Multi objective calibration of large scaled water quality model using a hybrid particle swarm optimization and neural network algorithm. KSCE J. Civ. Eng. 2012 , 16 , 913–918. [ Google Scholar ] [ CrossRef ]
- Areerachakul, S.; Sophatsathit, P.; Lursinsap, C. Integration of unsupervised and supervised neural networks to predict dissolved oxygen concentration in canals. Ecol. Modell. 2013 , 261–262 , 1–7. [ Google Scholar ] [ CrossRef ]
- Gazzaz, N.M.; Yusoff, M.K.; Ramli, M.F.; Juahir, H.; Aris, A.Z. Artificial Neural Network Modeling of the Water Quality Index Using Land Use Areas as Predictors. Water Environ. Res. 2015 , 87 , 99–112. [ Google Scholar ] [ CrossRef ] [ PubMed ]
- Heddam, S. Simultaneous modelling and forecasting of hourly dissolved oxygen concentration (DO) using radial basis function neural network (RBFNN) based approach: A case study from the Klamath River, Oregon, USA. Model. Earth Syst. Environ. 2016 , 2 , 1–18. [ Google Scholar ] [ CrossRef ] [ Green Version ]
- Liu, S.; Xu, L.; Li, D. Multi-scale prediction of water temperature using empirical mode decomposition with back-propagation neural networks. Comput. Electr. Eng. 2016 , 49 , 1–8. [ Google Scholar ] [ CrossRef ]
- Yu, H.; Chen, Y.; Hassan, S.; Li, D. Dissolved oxygen content prediction in crab culture using a hybrid intelligent method. Sci. Rep. 2016 , 6 , 1–10. [ Google Scholar ] [ CrossRef ]
- Zhao, Y.; Zou, Z.; Wang, S. A Back Propagation Neural Network Model based on kalman filter for water quality prediction. In Proceedings of the International Conference Natrual Computation, Zhangjiajie, China, 15–17 August 2015; pp. 149–153. [ Google Scholar ] [ CrossRef ]
- Karaboga, D.; Basturk, B. A powerful and efficient algorithm for numerical function optimization: Artificial bee colony (ABC) algorithm. J. Glob. Optim. 2007 , 39 , 459–471. [ Google Scholar ] [ CrossRef ]
- Zhou, J.; Wang, Y.; Xiao, F.; Wang, Y.; Sun, L. Water quality prediction method based on IGRA and LSTM. Water 2018 , 10 , 1148. [ Google Scholar ] [ CrossRef ] [ Green Version ]
- Jin, T.; Cai, S.; Jiang, D.; Liu, J. A data-driven model for real-time water quality prediction and early warning by an integration method. Environ. Sci. Pollut. Res. 2019 , 26 , 30374–30385. [ Google Scholar ] [ CrossRef ]
- Tian, W.; Liao, Z.; Wang, X. Transfer learning for neural network model in chlorophyll-a dynamics prediction. Environ. Sci. Pollut. Res. 2019 , 26 , 29857–29871. [ Google Scholar ] [ CrossRef ] [ PubMed ]
- Yan, J.; Chen, X.; Yu, Y.; Zhang, X. Application of a parallel particle swarm optimization-long short term memory model to improve water quality data. Water 2019 , 11 , 1317. [ Google Scholar ] [ CrossRef ] [ Green Version ]
- Chu, H.B.; Lu, W.X.; Zhang, L. Application of artificial neural network in environmental water quality assessment. J. Agric. Sci. Technol. 2013 , 15 , 343–356. [ Google Scholar ]
- Rajaee, T.; Ebrahimi, H.; Nourani, V. A review of the arti fi cial intelligence methods in groundwater level modeling. J. Hydrol. 2019 , 572 , 336–351. [ Google Scholar ] [ CrossRef ]
- Rajaee, T.; Ravansalar, M.; Adamowski, J.F.; Deo, R.C. A New Approach to Predict Daily pH in Rivers Based on the “à trous” Redundant Wavelet Transform Algorithm. Water. Air. Soil Pollut. 2018 , 229 . [ Google Scholar ] [ CrossRef ]
- Verma, A.K.; Singh, T.N. Prediction of water quality from simple field parameters. Environ. Earth Sci. 2013 , 69 , 821–829. [ Google Scholar ] [ CrossRef ]
- Ruben, G.B.; Zhang, K.; Bao, H.; Ma, X. Application and Sensitivity Analysis of Artificial Neural Network for Prediction of Chemical Oxygen Demand. Water Resour. Manag. 2018 , 32 , 273–283. [ Google Scholar ] [ CrossRef ]
- Wen, X.; Fang, J.; Diao, M.; Zhang, C. Artificial neural network modeling of dissolved oxygen in the Heihe River, Northwestern China. Environ. Monit. Assess. 2013 , 185 , 4361–4371. [ Google Scholar ] [ CrossRef ]
- Emamgholizadeh, S.; Kashi, H.; Marofpoor, I.; Zalaghi, E. Prediction of water quality parameters of Karoon River (Iran) by artificial intelligence-based models. Int. J. Environ. Sci. Technol. 2014 , 11 , 645–656. [ Google Scholar ] [ CrossRef ] [ Green Version ]
- Li, X.; Sha, J.; Wang, Z. liang A comparative study of multiple linear regression, artificial neural network and support vector machine for the prediction of dissolved oxygen. Hydrol. Res. 2017 , 48 , 1214–1225. [ Google Scholar ] [ CrossRef ]
- DeWeber, J.T.; Wagner, T. A regional neural network ensemble for predicting mean daily river water temperature. J. Hydrol. 2014 , 517 , 187–200. [ Google Scholar ] [ CrossRef ]
- Ahmadi, A.; Fatemi, Z.; Nazari, S. Assessment of input data selection methods for BOD simulation using data-driven models: A case study. Environ. Monit. Assess. 2018 , 190 . [ Google Scholar ] [ CrossRef ] [ PubMed ]
- Read, J.S.; Jia, X.; Willard, J.; Appling, A.P.; Zwart, J.A.; Oliver, S.K.; Karpatne, A.; Hansen, G.J.A.; Hanson, P.C.; Watkins, W.; et al. Process-Guided Deep Learning Predictions of Lake Water Temperature. Water Resour. Res. 2019 , 55 , 9173–9190. [ Google Scholar ] [ CrossRef ] [ Green Version ]
- Bowden, G.J.; Dandy, G.C.; Maier, H.R. Input determination for neural network models in water resources applications. Part 1—Background and methodology. J. Hydrol. 2005 , 301 , 75–92. [ Google Scholar ] [ CrossRef ]
- Chang, F.J.; Chen, P.A.; Liu, C.W.; Liao, V.H.C.; Liao, C.M. Regional estimation of groundwater arsenic concentrations through systematical dynamic-neural modeling. J. Hydrol. 2013 , 499 , 265–274. [ Google Scholar ] [ CrossRef ]
- Bontempi, G.; Ben Taieb, S.; Le Borgne, Y.A. Machine learning strategies for time series forecasting. In Business Intelligence ; Spriger: Berlin/Heidelberg, Germany, 2013; ISBN 9783642363177. [ Google Scholar ]
- Kohavi, R. A Study of Cross-Validation and Bootstrap for Accuracy Estimation and Model Selection. In Proceedings of the 14th International Joint Conference on Artificial Intelligence, IJCAI’95, Morgan Kaufmann, United States, Montreal, QC, Canada, 20–25 August 1995; pp. 1137–1143. [ Google Scholar ]
- Chen, L.; Hsu, H.H.; Kou, C.H.; Yeh, H.C.; Wang, T.S. Applying Multi-temporal Satellite Imageries to Estimate Chlorophyll-a Concentration in Feitsui Reservoir using ANNs. IJCAI Int. Jt. Conf. Artif. Intell. 2009 , 345–348. [ Google Scholar ] [ CrossRef ]
- Ebadati, N.; Hooshmandzadeh, M. Water quality assessment of river using RBF and MLP methods of artificial network analysis (case study: Karoon River Southwest of Iran). Environ. Earth Sci. 2019 , 78 , 1–12. [ Google Scholar ] [ CrossRef ]
- Olyaie, E.; Banejad, H.; Chau, K.W.; Melesse, A.M. A comparison of various artificial intelligence approaches performance for estimating suspended sediment load of river systems: A case study in United States. Environ. Monit. Assess. 2015 , 187 . [ Google Scholar ] [ CrossRef ]
- Parmar, K.S.; Bhardwaj, R. River Water Prediction Modeling Using Neural Networks, Fuzzy and Wavelet Coupled Model. Water Resour. Manag. 2015 , 29 , 17–33. [ Google Scholar ] [ CrossRef ]
- Rajaee, T.; Shahabi, A. Evaluation of wavelet-GEP and wavelet-ANN hybrid models for prediction of total nitrogen concentration in coastal marine waters. Arab. J. Geosci. 2016 , 9 . [ Google Scholar ] [ CrossRef ]
- Dragoi, E.N.; Kovács, Z.; Juzsakova, T.; Curteanu, S.; Cretescu, I. Environmental assesment of surface waters based on monitoring data and neuro-evolutive modelling. Process Saf. Environ. Prot. 2018 , 120 , 136–145. [ Google Scholar ] [ CrossRef ]
- Voza, D.; Vuković, M. The assessment and prediction of temporal variations in surface water quality—A case study. Environ. Monit. Assess. 2018 , 190 . [ Google Scholar ] [ CrossRef ] [ PubMed ]
Click here to enlarge figure
Abbreviations | Full Name | Abbreviations | Full Name | Abbreviations | Full Name | Abbreviations | Full Name |
---|---|---|---|---|---|---|---|
AH | air humidity | EC | Electrical conductivity | ORP | Oxidation reduction potential | TCC | total chromium concentration |
AODD | August, October, December, data | Evap | evaporation | Q | discharge | TIC | total iron concentration |
AP | air pressure | FTT | flow travel time | pH | Pondus Hydrogenii | TAC | total anions and cations |
AT | air temperature | Fe | iron | Precip | precipitation | TNs | total nutrients |
As | Arsenic | F | flow | P | phosphate | TA | total alkalinity |
B | boron | HCO | bicarbonate | RH | relative humidity | TP | total phosphorus |
BOD | Biochemical Oxygen Demand; | HA | Hydrogenated Amine | RP | Redox potential | Tur | turbidity |
C | carbon | ICs | ionic concentrations | RO | runoff | TDS | total dissolved solids |
Cl | chloride | K | potassium | RF | rainfall | TN | total nitrogen |
Cu | Copper | Lon | longitude | RainP | Rainy period | TH | total hardness |
Ca | calcium | Lat | latitude | SR | solar radiation | TOC | total organic carbon |
CO | Carbonate | LV | lake volume | Sth | sunshine time hours | TSS | total suspended solids |
Coli | Coliform | MDHM | month, day, hour, minute | SD | transparence | VP | volatile phenol |
COD | Chemical Oxygen Demand | Mn | manganese; | SAR | sodium absorption ratio | WL | Water Level |
COD | permanganate index | Mg | magnesium | SM | Soil Moisture | WT | water temperature |
Chl-a | Chlorophyll a | Na | sodium | ST | soil temperature | WS | wind speed |
DO | dissolved oxygen | Ns | nutrients | SO | sulphate | WD | wind direction |
DOY | day of year | NO | nitrite | S | salinity | YMDH | the year numbers |
Categories | Structure(s) | Advantage(s) | Reference(s) |
---|---|---|---|
MLPs | They are based on an understanding of the biological nervous system | Solving the nonlinear problems | [ , , , , , , ] |
TDNNs | They are based on the structure of MLPs | Using time delay cells to deal with the dynamic nature of sample data | [ ] |
RBFNNs | The structure of RBFNNs is similar to the MLPs The radial basis activation function is in the hidden layer | To overcome the local minimum problems | [ , , , ] |
GRNNs | A modified form of the RBFNNs model There is a pattern and a summation layer between the input and output layers | Solving the small sample problems | [ , , , , , ] |
WNNs | Wavelet function replace the linear sigmoid activation functions of MLPs | Solving the non-stationary problems | [ , ] |
ELMs | The structure of ELMs is similar to the MLPs Only need to learn the output weight | Reducing the computation problems because the weights of the input and hidden layer need not be adjusted | [ , , , , ] |
CCNN | Start with input and output layer without a hidden layer | A constructive neural network that aims to solve the problems of the determination of potential neurons which are not relevant to the output layer | [ ] |
MNNs | A special feedforward network Choosing the neural network which have the maximum similarity between the inputs and centroids of the cluster | Solving the problem of low prediction accuracy | [ , ] |
RNNs | The RNNs are developed with the development of deep learning | Solving the problems of long-term dependence which are not captured by the feedforward network | [ , , , , ] |
LSTMs | Its structure is similar to RNNs Memory cell state is added to hidden layer | Addressing the well-known vanishing gradient problem of RNNs | [ , , , , ] |
TLRN | Its structure is similar to MLPs It has the local recurrent connections in the hidden layer | Reducing the influence of the noise and owning the advantage of adaptive memory depth | [ ] |
NARX | Sub-classes of RNNs Their recurrent connections are from the output | Solving the problems of long-term dependence | [ ] |
Elman | A context layer that can store the internal states is added besides the traditional three layers | It is useful in dynamic system modeling because of the context layer | [ ] |
ESN | Different from the above recurrent neural networks The three layers are input, reservoir, and readout layer | To overcome the problems of the local minima and gradient vanishing | [ ] |
RESN | They are based on the structure of ESN which has a large and sparsely connected reservoir | To overcome the ill-posed problem existing in the ESN | [ ] |
Hybrid methods | The combination of conventional or preprocess methods with ANNs The internal integration of ANN methods or | Exploring the advantages of each methods | [ ] |
CNN | Input, convolution, fully connection, and output layers | An emerging method to solve the dissolved oxygen prediction problem | [ ] |
SODBN | They are based on the structure of DBN whose visible and hidden layers are stacked sequentially | Investigating the problem of dynamically determining the structure of DBN | [ ] |
Water Quality Variables | Categories | Unit | Major Sensors | Research Scenarios |
---|---|---|---|---|
DO | chemical | mg/L | ✓ | river, lake, reservoir, WWTP, ponds, coastal waters, creek, drain |
BOD | chemical | mg/L | - | river, lake, WWTP, mine water experimental system |
COD | chemical | mg/L | - | river, lake, reservoir, WWTP, groundwater, mine water |
WT | physical | °C | ✓ | river, lake, ponds, catchment, stream, coastal waters |
Chl-a | biological | μg/L | ✓ | lake, reservoir, surface water, coastal waters |
pH | physical | none | ✓ | river, lake, WWTP, stream, coastal waters |
SS | physical | mg/L | - | river, stream, coastal waters, creek, catchment |
EC | physical | us cm | ✓ | river, lake, reservoir, groundwater, stream |
TP | physical | μg/L | - | river, lake, WWTP |
NH -N | chemical | mg/L | ✓ | river, lake, reservoir, groundwater experimental system |
Tur | physical | FNU | ✓ | river, stream |
NO | chemical | mg/L | - | river, groundwater, catchment, wells, aquifer experimental system |
TDS | physical | mg/L | - | river, groundwater, drain |
S | physical | psu | ✓ | groundwater, coastal waters |
TN | chemical | mg/L | - | lake, WWTP, coastal waters |
B | physical | mg/L | - | river |
TH | physical | mg/L | - | river |
TOC | chemical | mg/L | - | river |
TSS | physical | mg/L | - | river |
COD | chemical | mg/L | - | river |
NO | chemical | mg/L | - | groundwater |
P | physical | mg/L | - | experimental system |
SD | physical | cm | - | lake |
Categories | Authors (Year) | Methods | Scenario (s) | Time Step | Dataset (Samples) |
---|---|---|---|---|---|
Feedforward | [ ] | GRNN, BPNN, RBFNN | lake | weekly | 28 (6 months) |
[ ] | ANN(MLP), GRNN | coastal waters | No details | 32 (5 months) | |
[ ] | BPNN | river | No details | 39 (3 days) | |
[ ] | ANN | mine water | No details | 73 | |
[ ] | ANN | groundwater | No details | 97 | |
[ ] | ANN(MLP) | surface water | No details | 110 | |
[ ] | MLP | river | No details | 110 (8 hours) | |
[ ] | ANN(MLP) | plain | No details | 122 | |
[ ] | ANN | groundwater | monthly | 124 (1 year) | |
[ ] | ANN(MLP) | stream | No details | 132 (11 months) | |
[ ] | ANN(MLP) | basin | fortnightly | 144 (1 year) | |
[ ] | ANN(MLP) | river | monthly | 144 (12 years) | |
[ ] | RBFNN | river | weekly | 144 | |
[ ] | GRNN, ANN(MLP), RBFNN, MLR | river | monthly | More than 151 samples (6 years) | |
[ ] | GRNN, MLR | Open-source data | No details | 159 (9 years) | |
[ ] | ANN(MLP) | river | monthly | 164 (over 6 years) | |
[ ] | ANN | reservoir | No details | 180 (3 years) | |
[ ] | ANN | system | No details | 195 (4 years) | |
[ ] | ANN(MLP), RBFNN | river | monthly | 200 (17 years) | |
[ ] | ANN | river | No details | 200 (16 years) | |
[ ] | ANN | river | No details | 232 (3 years) | |
[ ] | CCNN, MLP | river | half a month | 232 (12 years) | |
[ ] | ANN | river | No details | 252 (21 years) | |
[ ] | ANN(MLP) | river | No details | 255 (7 months) | |
[ ] | ANN(MLP), RBFNN, GRNN | WWTP | daily | 265 (3 years) | |
[ ] | ELM | WWTP | daily | 360 | |
[ ] | ANN | WWTP | daily | 364 (1 year) | |
[ ] | BPNN | reservoir | No details | 400 (20 years) | |
[ ] | BPNN | NA | No details | 500 | |
[ ] | ANN | river | monthly | 500 (10 years) | |
[ ] | ANN | groundwater | 30 minutes | 818 (nearly 17 days) | |
[ ] | BPNN | river | No details | 969 | |
[ ] | MLP, RBF, GRNN | Well | No details | 975 (16 years) | |
[ ] | ANN(MLP) | stream | daily | 982 (6 months) | |
[ ] | MLP | lake | No details | 1087 (6 years) | |
[ ] | ANN | lake | No details | 1217 | |
[ ] | RBFNN, GRNN, MLR | river | No details | More than 1300 samples (6 years) | |
[ ] | RBFNN, TDNN | river | monthly | 1320 (10 years) | |
[ ] | GRNN | river | No details | 1512 (9 years) | |
[ ] | MNN | WWTP | No details | 1900 | |
[ ] | ANN(MLP), RBFNN | river | daily | 2063 (6 years) | |
[ ] | ANN | river | No details | 3001 | |
[ ] | RBFNN, ANN(MLP), MLR | upstream and downstream | daily | 2063 and 4765 samples (18 years) | |
[ ] | ANN | river | daily | more than 3000 samples (11 years) | |
[ ] | ANN | lake | 15 minutes | 6674 (86 days) | |
[ ] | ANN | river | No details | 13,800 (5 years) | |
[ ] | GRNN | river | No details | more than 32,000 samples | |
[ ] | ELM, ANN(MLP) | Open-source data | hourly | 35,064 (4 years) | |
[ ] | ANN(MLP) | power station | 10 minutes | 45,594 (2 years) | |
Recurrent | [ ] | Elman, GRNN, BPNN, MLR | river | monthly or semi-monthly | 61 |
[ ] | NARX, BPNN, MLR | river | monthly | 280 (11 years) | |
[ ] | LSTM | river | 12 hours | 460 (14months) | |
[ ] | LSTM, BPNN | lake | monthly | 657 (7 years) | |
[ ] | LSTM, RNN | Mariculture base | 5 minutes | 710 (21 days) | |
[ ] | SRU | Mariculture base | No details | 710 | |
[ ] | Elman | pond | No details | 816 (34 days) | |
[ ] | RNN, LSTM | reservoir | 5 minutes | 1440 (5 days) | |
[ ] | RNN, BPNN | river | No details | 1448 | |
[ ] | LSTM | lake | No details | 1520 | |
[ ] | LSTM, BPNN | pond | 10 minutes | 2880 (20 days) | |
[ ] | RESN | WWTP | No details | 5000 | |
[ ] | RNN | Freshwater | 10 minutes | 5006 (1 year) | |
[ ] | TLRN, RNN, TDNN | lake | 15 minutes | 13,744 (573 days) | |
[ ] | LSTM | WWTP | hourly | 23,268 (4 years) |
Category | Type | Relationship | Description |
---|---|---|---|
Univariate-Input-Itself-Output (Category 0) | Univariate | Temporal relationship | The output(s) at a specific point are learned from its own historical information |
Univariate-Input-Other(one)-Output (Category 1) | Univariate | Temporal relationship | The output(s) at a specific point are learned the historical information from other variables (one) |
Multivariate- Input-Other (multi)-Output (Category 2) | Multivariate | Temporal relationship | The output(s) at a specific point are learned the historical information from other variables (more than one) |
Multivariate-Input-Itself-Other-Output (Category 3) | Multivariate | Temporal relationship | The output(s) at a specific point are learned the historical information from both its own and other variables |
Multivariate-Input-Itself-Other (multi)-Output (Category 4) | Multivariate | Temporal relationship and spatial relationship | The output(s) at a specific point are learned the historical information from both its own and other variables (more than one) |
Categories | Methods | Comments |
---|---|---|
model-free | ad-hoc | Based on domain knowledge or casual way |
analytic | The linear and non-linear relationship between input and output | |
other | IGRA, Garson method | |
model-based | ad-hoc | e.g., trial-and-error |
stepwise | Constructive and pruning methods | |
sensitivity analysis | e.g., MCS | |
global optimization | e.g., GA |
Categories | Methods | Comments |
---|---|---|
supervised | trial-and-error | Taking the statistical properties of each subset into consideration |
temporal partitioning | Dividing the data into diel, diurnal, and nocturnal | |
M-test | The number of the data points was obtained through the winGamma software | |
unsupervised | ad-hoc | Based on domain knowledge or a casual way |
random | Divide the data randomly | |
cross-validation | e.g., K-fold cross-validation, leave-one-out cross-validation | |
stratified method | e.g., SOM |
Categories | Methods | Comments |
---|---|---|
Normalization | No details | Built-in functions in platforms |
Range scaling | The scale of each feature is in the same range | |
Standardization | A new variable with zero mean and unit standard deviation | |
Missing data imputation | Only mentioned | Not recommend |
Deletion | Not recommend | |
Linear interpolation | The slope of the assumed line to calculate the data increment | |
Improved mean value method | Solve the breakpoint phenomenon of mean value method and linear interpolation method | |
Missing–refilling scheme | Dividing of ID and SD and using Temporal exponentially moving average to fill the missing data | |
Gap-filling | Temporal partitioning as gap-filling in order to get continuous records | |
Filling in the predicted values of the model | The missing values of predictors at time T0 are obtained by prediction values of the model at time T0 by other predictors | |
Data correct | Smoothing method | The moving average filtering can attenuate high-frequency signals |
Mean value method | Need to be corrected as a median of k data before and after | |
Data abnormal | The fixed threshold method | Setting the upper and lower threshold ranges (discard) |
Categories | Methods | Comments | Typical Examples |
---|---|---|---|
Ad-hoc | Empirical formula and trial-and-error approach | Rule 1: M is less than N minus 1 Rule 2: one range of M is equal to the sqrt of N plus O and finally plus A Rule 3: the other range of M is equal to log base 2 logarithm of N | [ ] |
Rule 4: M is equal to 5 multiplied by sqrt of N | [ ] | ||
Rule 5: M is equal to half of the sum of N and O plus square root of the number of training patterns | [ ] | ||
Rule 6: M is equal to sqrt of N plus one and finally plus A | [ ] | ||
Rule 7: M is equal to sqrt of N multiplied by O | [ ] | ||
Trial-and-error | Purely on a trial-and-error approach | [ ] | |
Stepwise trial-and-error | Stepwise trial-and-error | With each modification of the trial, a structure that is neither too complex nor too simple is building | [ ] |
Global methods | GA | Searching the solution space through simulated natural evolution | [ ] |
u GA | Introducing creep mutation in a small population | [ ] | |
IGA | Selecting excellent individuals effectively to avoid the situation of discarding by GA | [ ] | |
PSO | Excitation function does not need to be differentiable and derivable | [ ] | |
IPSO | The convergence rate and accuracy of the solution are improved | [ ] | |
ABC | More precise than PSO and GA | [ ] | |
IABC | Updating formulas just like the PSO algorithm | [ ] | |
Others | Not mentioned | Not recommend | [ ] |
Not required | Fixed structures such as GRNNs | [ ] |
Categories | Methods | Comments |
---|---|---|
Deterministic | BP algorithm(L) | Computing the direction of gradient descent |
Newton’s methods(L) | The computing tasks are implemented by Hessian matrix | |
Conjugate gradient method(L) | The search direction is carried along the conjugate direction and does not need to use Hessian matrix | |
Levenberg–Marquardt method(L) | A method, combination of BP and Newton algorithm, use Jacobian matrix to do the computing tasks | |
The Quasi-Newton method(L) | It is applied to the situation of that Jacobian matrix or Hessian matrix is difficult or even impossible to compute | |
BFGS | A Quasi-Newton method implemented by the built-in function in R | |
TRAINLM | A gradient descent with momentum and Levenberg–Marquardt backpropagation | |
Global optimization | See | |
Stochastic methods | Bayesian methods | Prediction limits can be obtained |
Adam optimization method | It implemented a reverse gradient update with the value obtained by Mini batch data | |
Emerging methods | Online learning algorithm | Quickly adjust the model in real time |
Share and Cite
Chen, Y.; Song, L.; Liu, Y.; Yang, L.; Li, D. A Review of the Artificial Neural Network Models for Water Quality Prediction. Appl. Sci. 2020 , 10 , 5776. https://doi.org/10.3390/app10175776
Chen Y, Song L, Liu Y, Yang L, Li D. A Review of the Artificial Neural Network Models for Water Quality Prediction. Applied Sciences . 2020; 10(17):5776. https://doi.org/10.3390/app10175776
Chen, Yingyi, Lihua Song, Yeqi Liu, Ling Yang, and Daoliang Li. 2020. "A Review of the Artificial Neural Network Models for Water Quality Prediction" Applied Sciences 10, no. 17: 5776. https://doi.org/10.3390/app10175776
Article Metrics
Article access statistics, further information, mdpi initiatives, follow mdpi.
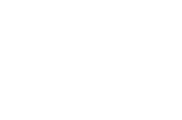
Subscribe to receive issue release notifications and newsletters from MDPI journals
On the Search of the Optimum Method for Water Quality Prediction Using Machine Learning Techniques Combined with Advanced Sampling Techniques
20 Pages Posted: 3 Sep 2024
Rahmi Fadhilah
Institut Teknologi Sepuluh Nopember
Heri Kuswanto
affiliation not provided to SSRN
Dedy Dwi Prastyo
Handling Imbalanced datasets is a significant challenge in water quality assessment. This study evaluates the performance of three machine learning models Naïve Bayes (NB), Extreme Gradient Boosting (XGBoost), and Random Forest (RF) on imbalanced water quality datasets. Various sampling strategies, including Random Undersampling (RUS), Rapidly Converging Gibbs Sampler (RACOG) and a combined RACOG-RUS approach, were employed to enhance model performance. The analysis shows notable variation in model accuracy and F1 scores depending on the sampling method and wheter feature selection was applied. XGBoost with RACOG achieved the highest performance without feature selection (accuracy: 0,958), while Naïve Bayes with RUS performed exceptionally well (accuracy: 0,986; F1 Score: 0,979). With feature selection, XGBoost with RACOG outperformed other models, reaching an F1 score of 0,982 and accuracy (0,606). These findings highlight the importance of advanced sampling techniques and feature selection in enhancing machine learning models for water quality classification. The method used:Apply advanced sampling techniques to handle imbalanced datasets effectively;Evaluated model performance with and without feature selection to identify the best approach;Enhance classification accuracy for better water quality assessment.
Keywords: Water Quality Classification, Imbalanced Data Handling, Random Forest, Naïve Bayes, XGBoost, Random Under Sampling (RUS), Rapidly Converging Gibbs Sampler (RACOG), RACOG-RUS
Suggested Citation: Suggested Citation
Institut Teknologi Sepuluh Nopember ( email )
Surabaya, 60111 Indonesia
Heri Kuswanto (Contact Author)
Affiliation not provided to ssrn ( email ).
No Address Available
Do you have a job opening that you would like to promote on SSRN?
Paper statistics, related ejournals, environmental data analysis ejournal.
Subscribe to this fee journal for more curated articles on this topic
IEEE Account
- Change Username/Password
- Update Address
Purchase Details
- Payment Options
- Order History
- View Purchased Documents
Profile Information
- Communications Preferences
- Profession and Education
- Technical Interests
- US & Canada: +1 800 678 4333
- Worldwide: +1 732 981 0060
- Contact & Support
- About IEEE Xplore
- Accessibility
- Terms of Use
- Nondiscrimination Policy
- Privacy & Opting Out of Cookies
A not-for-profit organization, IEEE is the world's largest technical professional organization dedicated to advancing technology for the benefit of humanity. © Copyright 2024 IEEE - All rights reserved. Use of this web site signifies your agreement to the terms and conditions.
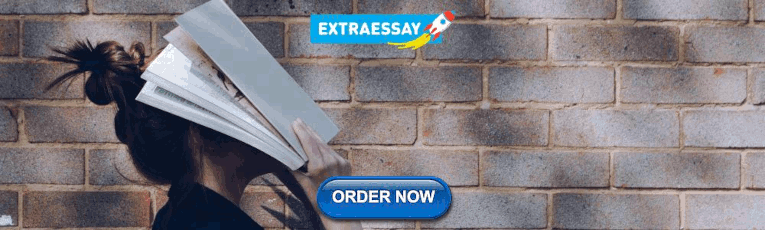
IMAGES
VIDEO
COMMENTS
Estimating water quality has been one of the significant challenges faced by the world in recent decades. This paper presents a water quality prediction model utilizing the principal component regression technique. Firstly, the water quality index (WQI) is calculated using the weighted arithmetic index method.
Water quality prediction, a well-established field with broad implications across various sectors, is thoroughly examined in this comprehensive review. Through an exhaustive analysis of over 170 studies conducted in the last five years, we focus on the application of machine learning for predicting water quality. The review begins by presenting the latest methodologies for acquiring water ...
International Journal of Scientific & Engineering Research, V olume 8, Issue 9, October-2022. ISSN 2229-5518. W ater Quality Prediction Using Machine Learning. Classification Algorithm. Michael ...
Chen, K. et al. Comparative analysis of surface water quality prediction performance and identification of key water parameters using different machine learning models based on big data. Water Res ...
1. Introduction. Ensuring the safety and quality of drinking water is a critical concern for water infrastructure management (Assembly, 2015; Tortajada, 2020).Water quality monitoring plays a vital role in achieving this objective by facilitating the detection and mitigation of potential risks, thereby ensuring the delivery of clean and safe water to consumers (Li et al., 2022; Mondejar et al ...
Here we (1) describe the challenges in water-quality sciences that DL can help to resolve, (2) review opportunities for DL in water quality prediction, particularly in addressing data scarcity and ...
The articles reviewed in this review study were selected to cover experiments focused specifically on water quality prediction. We found 83 research articles as shown in Table 1 and Fig. 1.Most of these articles were published in the last 5 years as shown in Fig. 2.Additionally, we selected to review these articles because they used various input parameters to predict the water quality as ...
Water quality is very dominant for humans, animals, plants, industries, and the environment. In the last decades, the quality of water has been impacted by contamination and pollution. In this paper, the challenge is to anticipate Water Quality Index (WQI) and Water Quality Classification (WQC), such that WQI is a vital indicator for water validity. In this study, parameters optimization and ...
Water, an invaluable and non-renewable resource, plays an indispensable role in human survival and societal development. Accurate forecasting of water quality involves early identification of future pollutant concentrations and water quality indices, enabling evidence-based decision-making and targeted environmental interventions. The emergence of advanced computational technologies ...
The presented research underscores the transformative impact of machine learning on water quality prediction in coastal areas. Our review on the limitations of current models, the need for diverse datasets, and the consideration of evolving environmental conditions points to avenues for future research. 5. Conclusions.
The application of machine learning in surface water quality research has become a hotspot [16, 17]. A series of surface water quality prediction and analysis methods have been developed (Table 1). Many efforts have been devoted to optimizing machine learning models and improving their prediction accuracy.
decision-making in environmental policy, resource management, and urban. planning. In this context, the application of m achine learning techniques offers a. promising avenue to enhance the pre ...
Water is the most crucial resource of life and it is necessary for the survival of all living creatures including human beings. The survival of business and agriculture depends on freshwater. An essential step in managing freshwater assets is the evaluation of the quality of the water. Before using water for anything, including drinking, chemical spraying (pesticides, etc.), or animal ...
Water pollution seriously endangers people's lives and restricts the sustainable development of the economy. Water quality prediction is essential for early warning and prevention of water pollution. However, the nonlinear characteristics of water quality data make it challenging to accurately predicted by traditional methods. Recently, the methods based on deep learning can better deal with ...
Additionally, these models can incorporate other environmental factors. and meteorological data, thereby improving the accuracy and reliability of water quality. prediction. However, machine ...
Abstract: Water quality prediction is a research hotspot in the field of ecological environment, which is of great significance to the prevention of water pollution and the construction of automatic water quality monitoring network. The accuracy of prediction model results will affect the scientificity and correctness of applied engineering projects, as well as the accuracy of water pollution ...
During these processes, two scenarios were introduced: Scenario 1 and Scenario 2. Scenario 1 constructs a prediction model for water quality parameters at every station, while Scenario 2 develops a prediction model on the basis of the value of the same parameter at the previous station (upstream). Both the scenarios are based on the value of ...
In addition, reliable predictions of water quality are also the best evidence that can help policy makers to make good decisions before disaster strikes (Lu and Ma 2020). In this research paper, our goal is to suggest a new model for prediction water quality based on machine learning algorithms and with minimal parameters. In addition, the ...
Water quality prediction plays an important role in environmental monitoring, ecosystem sustainability, and aquaculture. Traditional prediction methods cannot capture the nonlinear and non-stationarity of water quality well. In recent years, the rapid development of artificial neural networks (ANNs) has made them a hotspot in water quality prediction. We have conducted extensive investigation ...
This paper reviews the published research results relating to water quality evaluation and prediction. Moreover, the paper classifies and compares the applied big data analytics approaches and big data based prediction models for water quality assessment. Furthermore, the paper also discusses the future research needs and challenges.
These findings highlight the importance of advanced sampling techniques and feature selection in enhancing machine learning models for water quality classification. The method used:Apply advanced sampling techniques to handle imbalanced datasets effectively;Evaluated model performance with and without feature selection to identify the best ...
Taking a step further, this paper explores Automated Deep Learning, which is a new research domain. This paper straddles perfectly built DL models and automated DL models. With the same baseline data, the authors intend to explore the potential of automated processing, as well as the shortcomings in it. ... The water quality prediction using ...
The increase in pollution influences the quantity and quality of water, which results high risk on health and other issues for human as well as for living organisms on the planet. Hence, evaluating and monitoring the quality of water, and its prediction become crucial and applicable area for research in the current scenario.
Water Quality Research Journal 1 February 2018; 53 (1): 3-13. doi: ... The aim of this study is the prediction of water quality components using artificial intelligence (AI) techniques including MLP, SVM, and group method of data handling (GMDH). ... In this part of the paper, the results of prediction of the internal relations between the ...
This review paper explores the latest developments in IoT-based automated water monitoring systems, focusing on their role in predicting and managing water quality in aquaponic systems.
1.Introduction. Surface water is a non-renewable resource that is important in the daily life of human beings (Chen et al., 2020).Predicting the trend of water quality in a watershed is necessary for ensuring that water quality remains within manageable limits (Kut et al., 2019, Peng et al., 2020).The task of predicting water quality has become more complex due to water quality is affected by ...