
An official website of the United States government
The .gov means it’s official. Federal government websites often end in .gov or .mil. Before sharing sensitive information, make sure you’re on a federal government site.
The site is secure. The https:// ensures that you are connecting to the official website and that any information you provide is encrypted and transmitted securely.
- Publications
- Account settings
- My Bibliography
- Collections
- Citation manager
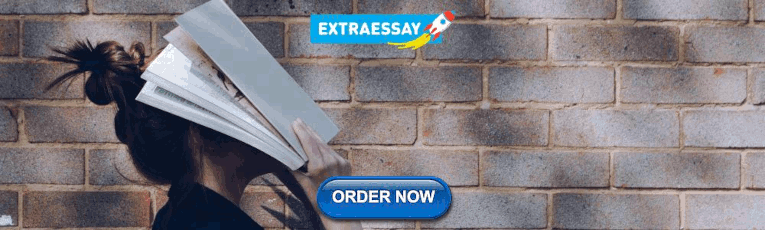
Save citation to file
Email citation, add to collections.
- Create a new collection
- Add to an existing collection
Add to My Bibliography
Your saved search, create a file for external citation management software, your rss feed.
- Search in PubMed
- Search in NLM Catalog
- Add to Search
Heart brain axis in health and disease: role of innate and adaptive immunity
Affiliations.
- 1 Institute of Biomedical Research of Barcelona (IIBB), Spanish National Research Council (CSIC), Barcelona, Spain.
- 2 DZHK (German Center for Cardiovascular Research), partner site Munich.
- 3 Institute for Stroke and Dementia Research (ISD), University Medical Center Munich, 81377 Munich, Germany.
- 4 Munich Cluster for Systems Neurology (SyNergy), Munich, Germany.
- PMID: 39180327
- DOI: 10.1093/cvr/cvae185
The importance of the brain-heart interaction has been increasingly recognized as a critical physiological axis that is altered in disease. In this review, we explore the intricate relationship between the central nervous system and cardiovascular health, focusing particularly on immunological mechanisms that influence the course of both neurological and cardiovascular diseases. While previous studies have established a key role of the autonomic nervous system in linking brain and the heart, more recent studies have expanded our understanding of the multifaceted inter-organ interactions. As such, circulating mediators include immune cells of the adaptive and innate immune system and their secreted immunogenic factors have come into the focus as mediators along this bidirectional communication. Hence, in this review we briefly discuss the contribution of the autonomic nervous system and then focus on innate and adaptive immune mechanisms along the heart-to-brain and brain-to-heart axes, illustrating how cardiovascular diseases affect cognitive functions and how brain pathologies lead to cardiac complications.
© The Author(s) 2024. Published by Oxford University Press on behalf of the European Society of Cardiology. All rights reserved. For commercial re-use, please contact [email protected] for reprints and translation rights for reprints. All other permissions can be obtained through our RightsLink service via the Permissions link on the article page on our site—for further information please contact [email protected].
PubMed Disclaimer
Related information
Linkout - more resources, full text sources.
- Silverchair Information Systems
Research Materials
- NCI CPTC Antibody Characterization Program
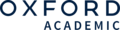
- Citation Manager
NCBI Literature Resources
MeSH PMC Bookshelf Disclaimer
The PubMed wordmark and PubMed logo are registered trademarks of the U.S. Department of Health and Human Services (HHS). Unauthorized use of these marks is strictly prohibited.
Thank you for visiting nature.com. You are using a browser version with limited support for CSS. To obtain the best experience, we recommend you use a more up to date browser (or turn off compatibility mode in Internet Explorer). In the meantime, to ensure continued support, we are displaying the site without styles and JavaScript.
- View all journals
- Explore content
- About the journal
- Publish with us
- Sign up for alerts
- Open access
- Published: 12 November 2020
Early and accurate detection and diagnosis of heart disease using intelligent computational model
- Yar Muhammad 1 ,
- Muhammad Tahir 1 ,
- Maqsood Hayat 1 &
- Kil To Chong 2
Scientific Reports volume 10 , Article number: 19747 ( 2020 ) Cite this article
27k Accesses
82 Citations
Metrics details
- Cardiovascular diseases
- Computational biology and bioinformatics
- Health care
- Heart failure
Heart disease is a fatal human disease, rapidly increases globally in both developed and undeveloped countries and consequently, causes death. Normally, in this disease, the heart fails to supply a sufficient amount of blood to other parts of the body in order to accomplish their normal functionalities. Early and on-time diagnosing of this problem is very essential for preventing patients from more damage and saving their lives. Among the conventional invasive-based techniques, angiography is considered to be the most well-known technique for diagnosing heart problems but it has some limitations. On the other hand, the non-invasive based methods, like intelligent learning-based computational techniques are found more upright and effectual for the heart disease diagnosis. Here, an intelligent computational predictive system is introduced for the identification and diagnosis of cardiac disease. In this study, various machine learning classification algorithms are investigated. In order to remove irrelevant and noisy data from extracted feature space, four distinct feature selection algorithms are applied and the results of each feature selection algorithm along with classifiers are analyzed. Several performance metrics namely: accuracy, sensitivity, specificity, AUC, F1-score, MCC, and ROC curve are used to observe the effectiveness and strength of the developed model. The classification rates of the developed system are examined on both full and optimal feature spaces, consequently, the performance of the developed model is boosted in case of high variated optimal feature space. In addition, P-value and Chi-square are also computed for the ET classifier along with each feature selection technique. It is anticipated that the proposed system will be useful and helpful for the physician to diagnose heart disease accurately and effectively.
Similar content being viewed by others
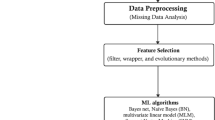
Analyzing the impact of feature selection methods on machine learning algorithms for heart disease prediction
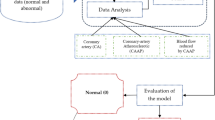
An active learning machine technique based prediction of cardiovascular heart disease from UCI-repository database
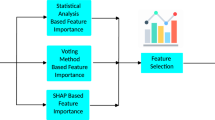
Finding the influential clinical traits that impact on the diagnosis of heart disease using statistical and machine-learning techniques
Introduction.
Heart disease is considered one of the most perilous and life snatching chronic diseases all over the world. In heart disease, normally the heart fails to supply sufficient blood to other parts of the body to accomplish their normal functionality 1 . Heart failure occurs due to blockage and narrowing of coronary arteries. Coronary arteries are responsible for the supply of blood to the heart itself 2 . A recent survey reveals that the United States is the most affected country by heart disease where the ratio of heart disease patients is very high 3 . The most common symptoms of heart disease include physical body weakness, shortness of breath, feet swollen, and weariness with associated signs, etc. 4 . The risk of heart disease may be increased by the lifestyle of a person like smoking, unhealthy diet, high cholesterol level, high blood pressure, deficiency of exercise and fitness, etc. 5 . Heart disease has several types in which coronary artery disease (CAD) is the common one that can lead to chest pain, stroke, and heart attack. The other types of heart disease include heart rhythm problems, congestive heart failure, congenital heart disease (birth time heart disease), and cardiovascular disease (CVD). Initially, traditional investigation techniques were used for the identification of heart disease, however, they were found complex 6 . Owing to the non-availability of medical diagnosing tools and medical experts specifically in undeveloped countries, diagnosis and cure of heart disease are very complex 7 . However, the precise and appropriate diagnosis of heart disease is very imperative to prevent the patient from more damage 8 . Heart disease is a fatal disease that rapidly increases in both economically developed and undeveloped countries. According to a report generated by the World Health Organization (WHO), an average of 17.90 million humans died from CVD in 2016. This amount represents approximately 30% of all global deaths. According to a report, 0.2 million people die from heart disease annually in Pakistan. Every year, the number of victimizing people is rapidly increasing. European Society of Cardiology (ESC) has published a report in which 26.5 million adults were identified having heart disease and 3.8 million were identified each year. About 50–55% of heart disease patients die within the initial 1–3 years, and the cost of heart disease treatment is about 4% of the overall healthcare annual budget 9 .
Conventional invasive-based methods used for the diagnosis of heart disease which were based on the medical history of a patient, physical test results, and investigation of related symptoms by the doctors 10 . Among the conventional methods, angiography is considered one of the most precise technique for the identification of heart problems. Conversely, angiography has some drawbacks like high cost, various side effects, and strong technological knowledge 11 . Conventional methods often lead to imprecise diagnosis and take more time due to human mistakes. In addition, it is a very expensive and computational intensive approach for the diagnosis of disease and takes time in assessment 12 .
To overcome the issues in conventional invasive-based methods for the identification of heart disease, researchers attempted to develop different non-invasive smart healthcare systems based on predictive machine learning techniques namely: Support Vector Machine (SVM), K-Nearest Neighbor (KNN), Naïve Bayes (NB), and Decision Tree (DT), etc. 13 . As a result, the death ratio of heart disease patients has been decreased 14 . In literature, the Cleveland heart disease dataset is extensively utilized by the researchers 15 , 16 .
In this regard, Robert et al . 17 have used a logistic regression classification algorithm for heart disease detection and obtained an accuracy of 77.1%. Similarly, Wankhade et al . 18 have used a multi-layer perceptron (MLP) classifier for heart disease diagnosis and attained accuracy of 80%. Likewise, Allahverdi et al . 19 have developed a heart disease classification system in which they integrated neural networks with an artificial neural network and attained an accuracy of 82.4%. In a sequel, Awang et al . 20 have used NB and DT for the diagnosis and prediction of heart disease and achieved reasonable results in terms of accuracy. They achieved an accuracy of 82.7% with NB and 80.4% with DT. Oyedodum and Olaniye 21 have proposed a three-phase system for the prediction of heart disease using ANN. Das and Turkoglu 22 have proposed an ANN ensemble-based predictive model for the prediction of heart disease. Similarly, Paul and Robin 23 have used the adaptive fuzzy ensemble method for the prediction of heart disease. Likewise, Tomov et al. 24 have introduced a deep neural network for heart disease prediction and his proposed model performed well and produced good outcomes. Further, Manogaran and Varatharajan 25 have introduced the concept of a hybrid recommendation system for diagnosing heart disease and their model has given considerable results. Alizadehsani et al . 26 have developed a non-invasive based model for the prediction of coronary artery disease and showed some good results regarding the accuracy and other performance assessment metrics. Amin et al . 27 have proposed a framework of a hybrid system for the identification of cardiac disease, using machine learning, and attained an accuracy of 86.0%. Similarly, Mohan et al . 28 have proposed another intelligent system that integrates RF with a linear model for the prediction of heart disease and achieved the classification accuracy of 88.7%. Likewise, Liaqat et al . 29 have developed an expert system that uses stacked SVM for the prediction of heart disease and obtained 91.11% classification accuracy on selected features.
The contribution of the current work is to introduce an intelligent medical decision system for the diagnosis of heart disease based on contemporary machine learning algorithms. In this study, 10 different nature of machine learning classification algorithms such as Logistic Regression (LR), Decision Tree (DT), Naïve Bayes (NB), Random Forest (RF), Artificial Neural Network (ANN), etc. are implemented in order to select the best model for timely and accurate detection of heart disease at an early stage. Four feature selection algorithms, Fast Correlation-Based Filter Solution (FCBF), minimal redundancy maximal relevance (mRMR), Least Absolute Shrinkage and Selection Operator (LASSO), and Relief have been used for selecting the vital and more correlated features that have truly reflect the motif of the desired target. Our developed system has been trained and tested on the Cleveland (S 1 ) and Hungarian (S 2 ) heart disease datasets which are available online on the UCI machine learning repository. All the processing and computations were performed using Anaconda IDE. Python has been used as a tool for implementing all the classifiers. The main packages and libraries used include pandas, NumPy, matplotlib, sci-kit learn (sklearn), and seaborn. The main contribution of our proposed work is given below:
The performance of all classifiers has been tested on full feature spaces in terms of all performance evaluation matrices specifically accuracy.
The performances of the classifiers are tested on selected feature spaces, selected through various feature selection algorithms mentioned above.
The research study recommends that which feature selection algorithm is feasible with which classification algorithm for developing a high-level intelligence system for the diagnosing of heart disease patients.
The rest of the paper is organized as: “ Results and discussion ” section represents the results and discussion, “ Material and methods ” section describes the material and methods used in this paper. Finally, we conclude our proposed research work in “ Conclusion ” section.
Results and discussion
This section of the paper discusses the experimental results of various contemporary classification algorithms. At first, the performance of all used classification models i.e. K-Nearest Neighbors (KNN), Decision Tree (DT), Extra-Tree Classifier (ETC), Random Forest (RF), Logistic Regression (LR), Naïve Bayes (NB), Artificial Neural Network (ANN), Support Vector Machine (SVM), Adaboost (AB), and Gradient Boosting (GB) along with full feature space is evaluated. After that, four feature selection algorithms (FSA): Fast Correlation-Based Filter (FCBF), Minimal Redundancy Maximal Relevance (mRMR), Least Absolute Shrinkage and Selection Operator (LASSO), and Relief are applied to select the prominent and high variant features from feature space. Furthermore, the selected feature spaces are provided to classification algorithms as input to analyze the significance of feature selection techniques. The cross-validation techniques i.e. k-fold (10-fold) are applied on both the full and selected feature spaces to analyze the generalization power of the proposed model. Various performance evaluation metrics are implemented for measuring the performances of the classification models.
Classifiers’ predictive outcomes on full feature space
The experimental outcomes of the applied classification algorithms on the full feature space of the two benchmark datasets by using 10-fold cross-validation (CV) techniques are shown in Tables 1 and 2 , respectively.
The experimental results demonstrated that the ET classifier performed quite well in terms of all performance evaluation metrics compared to the other classifiers using 10-fold CV. ET achieved 92.09% accuracy, 91.82% sensitivity, 92.38% specificity, 97.92% AUC, 92.84% Precision, 0.92 F1-Score and 0.84 MCC. The specificity indicates that the diagnosed test was negative and the individual doesn't have the disease. While the sensitivity indicates the diagnostic test was positive and the patient has heart disease. In the case of the KNN classification model, multiple experiments were accomplished by considering various values for k i.e. k = 3, 5, 7, 9, 13, and 15, respectively. Consequently, KNN has shown the best performance at value k = 7 and achieved a classification accuracy of 85.55%, 85.93% sensitivity, 85.17% specificity, 95.64% AUC, 86.09% Precision, 0.86 F1-Score, and 0.71 MCC. Similarly, DT classifier has achieved accuracy of 86.82%, 89.73% sensitivity, 83.76% specificity, 91.89% AUC, 85.40% Precision, 0.87 F1-Score, and 0.73 MCC. Likewise, GB classifier has yielded accuracy of 91.34%, 90.32% sensitivity, 91.52% specificity, 96.87% AUC, 92.14% Precision, 0.92 F1-Score, and 0.83 MCC. After empirically evaluating the success rates of all classifiers, it is observed that ET Classifier out-performed among all the used classification algorithms in terms of accuracy, sensitivity, and specificity. Whereas, NB shows the lowest performance in terms of accuracy, sensitivity, and specificity. The ROC curve of all classification algorithms on full feature space is represented in Fig. 1 .
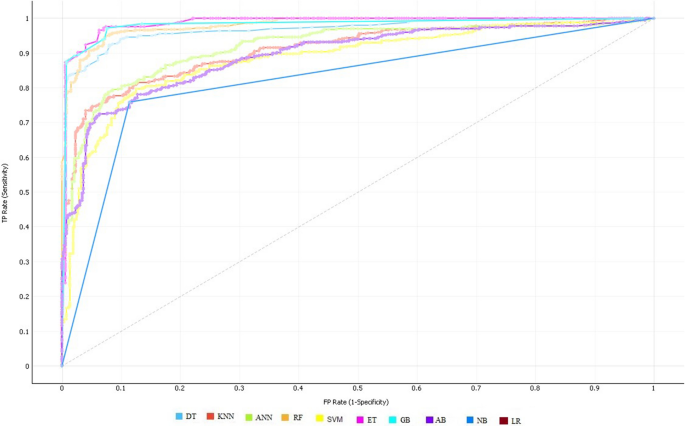
ROC curves of all classifiers on full feature space using 10-fold cross-validation on S 1 .
In the case of dataset S 2 , composed of 1025 total instances in which 525 belong to the positive class and 500 instances of having negative class, again ET has obtained quite well results compared to other classifiers using a 10-fold cross-validation test, which are 96.74% accuracy, 96.36 sensitivity, 97.40% specificity, and 0.93 MCC as shown in Table 2 .
Classifiers’ predictive outcomes on selected feature space
Fcbf feature selection technique.
FCBF feature selection technique is applied to select the best subset of feature space. In this attempt, various length of subspaces is generated and tested. Finally, the best results are achieved by classification algorithms on the subset of feature space (n = 6) using a 10-fold CV. Table 3 shows various performance measures of classifiers executed on the selected features space of FCBF.
Table 3 demonstrates that the ET classifier obtained quite good results including accuracy of 94.14%, 94.29% sensitivity, and specificity of 93.98%. In contrast, NB reported the lowest performance compared to the other classification algorithms. The performance of classification algorithms is also illustrated in Fig. 2 by using ROC curves.
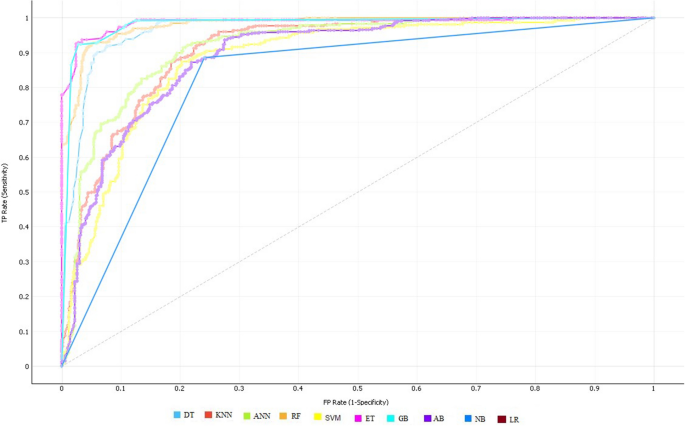
ROC curve of all classifiers on selected features by FCBF feature selection algorithm.
mRMR feature selection technique
mRMR feature selection technique is used in order to select a subset of features that enhance the performance of classifiers. The best results reported on a subset of n = 6 of feature space which is shown in Table 4 .
In the case of mRMR, still, the success rates of the ET classifier are well in terms of all performance evaluation metrics compared to the other classifiers. ET has attained 93.42% accuracy, 93.92% sensitivity, and specificity of 93.88%. In contrast, NB has achieved the lowest outcomes which are 81.84% accuracy. Figure 3 shows the ROC curve of all ten classifiers using the mRMR feature selection algorithm.
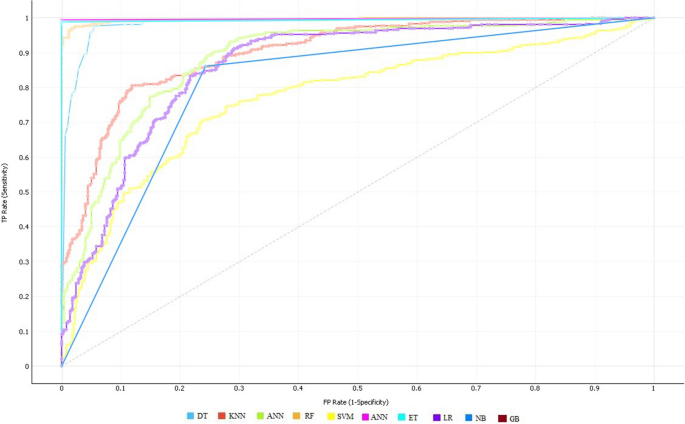
ROC curve of all classifiers on selected features using the mRMR feature selection algorithm.
LASSO feature selection technique
In order to choose the optimal feature space which not only reduces computational cost but also progresses the performance of the classifiers, LASSO feature selection technique is applied. After performing various experiments on different subsets of feature space, the best results are still noted on the subspace of (n = 6). The predicted outcomes of the best-selected feature space are reported in Table 5 using the 10-fold CV.
Table 5 demonstrated that the predicted outcomes of the ET classifier are considerable and better compared to the other classifiers. ET has achieved 89.36% accuracy, 88.21% sensitivity, and specificity of 90.58%. Likewise, GB has yielded the second-best result which is the accuracy of 88.47%, 89.54% sensitivity, and specificity of 87.37%. Whereas, LR has performed worse results and achieved 80.77% accuracy, 83.46% sensitivity, and specificity of 77.95%. ROC curves of the classifiers are shown in Fig. 4 .
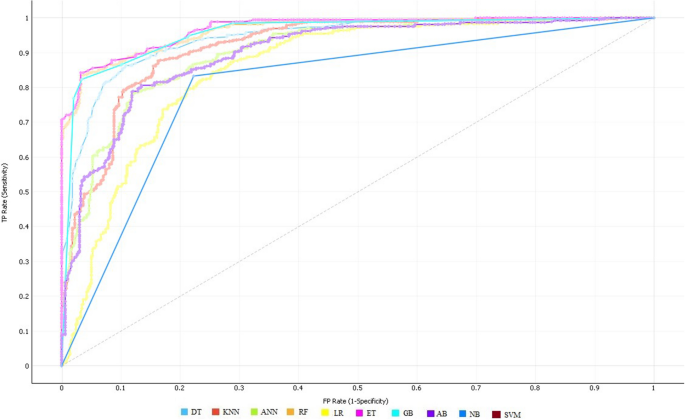
ROC curve of all classifiers on selected feature space using the LASSO feature selection algorithm.
Relief feature selection technique
In a sequel, another feature selection technique Relief is applied to investigate the performance of classifiers on different sub-feature spaces by using the wrapper method. After empirically analyzing the results of the classifiers on a different subset of feature spaces, it is observed that the performance of classifiers is outstanding on the sub-space of length (n = 6). The results of the optimal feature space on the 10-fold CV technique are listed in Table 6 .
Again, the ET classifier performed outstandingly in terms of all performance evaluation metrics as compared to other classifiers. ET has obtained an accuracy of 94.41%, 94.93% sensitivity, and specificity of 94.89%. In contrast, NB has shown the lowest performance and achieved 80.29% accuracy, 81.93% sensitivity, and specificity of 78.55%. The ROC curves of the classifiers are demonstrated in Fig. 5 .
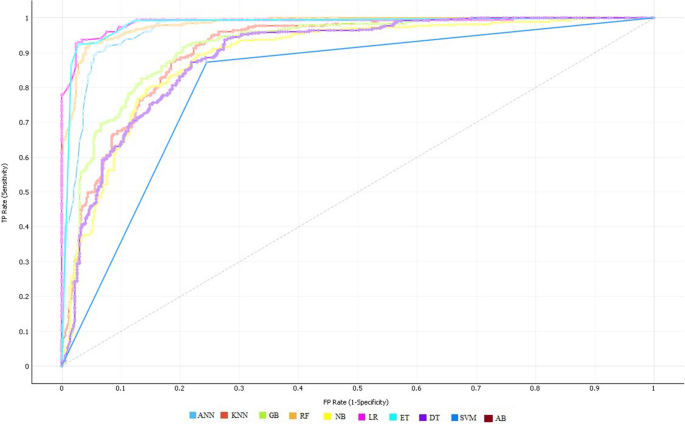
ROC curve of all classifiers on selected features selected by the Relief feature selection algorithm.
After executing classification algorithms along with full and selected feature spaces in order to select the optimal algorithm for the operational engine, the empirical results have revealed that ET performed well not only on all feature space but also on optimal selected feature space among all the used classification algorithms. Furthermore, the ET classifier obtained quite promising accuracy in the case of the Relief feature selection technique which is 94.41%. Overall, the performance of ET is reported better in terms of most of the measures while other classifiers have shown good results in one measure while worse in other measures. In addition, the performance of the ET classifier is also evaluated on a 10-fold CV in combination with different sub-feature spaces of varying length starting from 1 to 12 with a step size of 1 to check the stability and discrimination power of the classifier as described in 30 . Doing so will assist the readers to have a better understanding of the impact, of the number of selected features on the performance of the classifiers. The same process is repeated for another dataset i.e. S 2 (Hungarian heart disease dataset) as well, to know the impact of selected features on the classification performance.
Tables 7 and 8 shows the performance of the ET classifier using 10-fold CV in combination with different feature sub-spaces starting from 1 to 12 with a step size of 1. The experimental results show that the performance of the ET classifier is affected significantly by using the varying length of sub-feature spaces. Finally, it is concluded that all these achievements are ascribed with the best selection of Relief feature selection technique which not only reduces the feature space but also enhances the predictive power of classifiers. In addition, the ET classifier has also played a quite promising role in these achievements because it has clearly and precisely learned the motif of the target class and reflected it truly. In addition, the performance of the ET classifier is also evaluated on 5-fold and 7-fold CV in combination with different sub-spaces of length 5 and 7 to check the stability and discrimination power of the classifier. It is also tested on another dataset S 2 (Hungarian heart disease dataset). The results are shown in supplementary materials .
In Table 9 , P-value and Chi-Square values are also computed for the ET classifier in combination with the optimal feature spaces of different feature selection techniques.
Performance comparison with existing models
Further, a comparative study of the developed system is conducted with other states of the art machine learning approaches discussed in the literature. Table 10 represents, a brief description and classification accuracies of those approaches. The results demonstrate that our proposed model success rate is high compared to existing models in the literature.
Material and methods
The subsections represent the materials and the methods that are used in this paper.
The first and rudimentary step of developing an intelligent computational model is to construct or develop a problem-related dataset that truly and effectively reflects the pattern of the target class. Well organized and problem-related dataset has a high influence on the performance of the computational model. Looking at the significance of the dataset, two datasets i.e. the Cleveland heart disease dataset S 1 and Hungarian heart disease dataset (S 2 ) are used, which are available online at the University of California Irvine (UCI) machine learning repository and UCI Kaggle repository, and various researchers have used it for conducting their research studies 28 , 31 , 32 . The S1 consists of 304 instances, where each instance has distinct 13 attributes along with the target labels and are selected for training. The dataset is composed of two classes, presence or absence of heart disease. The S 2 is composed of 1025 instances in which 525 instances belong to positive class while the rest of 500 instances have negative class. The description of attributes of both the datasets is the same, and both have similar attributes. The complete description and information of the datasets with 13 attributes are given in Table 11 .
Proposed system methodology
The main theme of the developed system is to identify heart problems in human beings. In this study, four distant feature selection techniques namely: FCBF, mRMR, Relief, and LASSO are applied on the provided dataset in order to remove noisy, redundant features and select variant features, consequently may cause of enhancing the performance of the proposed model. Various machine learning classification algorithms are used in this study which include, KNN, DT, ETC, RF, LR, NB, ANN, SVM, AB, and GB. Different evaluation metrics are computed to assess the performance of classification algorithms. The methodology of the proposed system is carried out in five stages which include dataset preprocessing, selection of features, cross-validation technique, classification algorithms, and performance evaluation of classifiers. The framework of the proposed system is illustrated in Fig. 6 .
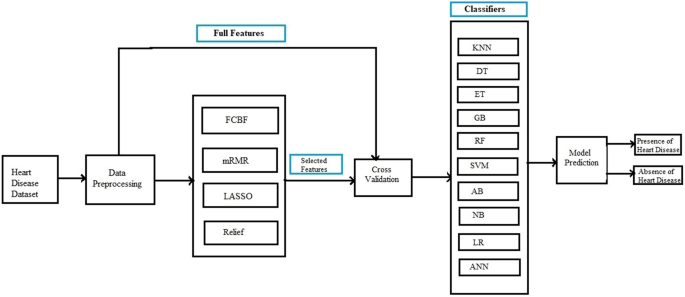
An Intelligent Hybrid Framework for the prediction of heart disease.
Preprocessing of data
Data preprocessing is the process of transforming raw data into meaningful patterns. It is very crucial for a good representation of data. Various preprocessing approaches such as missing values removal, standard scalar, and Min–Max scalar are used on the dataset in order to make it more effective for classification.
Feature selection algorithms
Feature selection technique selects the optimal features sub-space among all the features in a dataset. It is very crucial because sometimes, the classification performance degrades due to irrelevant features in the dataset. The feature selection technique improves the performance of classification algorithms and also reduces their execution time. In this research study, four feature selection techniques are used and are listed below:
Fast correlation-based filter (FCBF): FCBF feature selection algorithm follows a sequential search strategy. It first selects full features and then uses symmetric uncertainty for measuring the dependencies of the features on each other and how they affect the target output label. After this, it selects the most important features using the backward sequential search strategy. FCBF outperforms on high dimensional datasets. Table 12 shows the results of the selected features (n = 6) by using the FCBF feature selection algorithm. Each attribute is given a weight based on its importance. According to the FCBF feature selection technique, the most important features are THA and CPT as shown in Table 12 . The ranking that the FCBF gives to all the features of the dataset is shown in Fig. 7 .
Minimal redundancy maximal relevance (mRMR): mRMR uses the heuristic approach for selecting the most vital features that have minimum redundancy and maximum relevance. It selects those features which are useful and relevant to the target. As it follows a heuristic approach so, it checks one feature at a time and then computes its pairwise redundancy with the other features. The mRMR feature selection algorithm is not suitable for high domain feature problems 33 . The results of selected features by the mRMR feature selection algorithm (n = 6) are listed in Table 13 . In addition, among these attributes, PES and CPT have the highest score. Figure 7 describes the attributes ranking given by the mRMR feature selection algorithm to all attributes in the feature space.
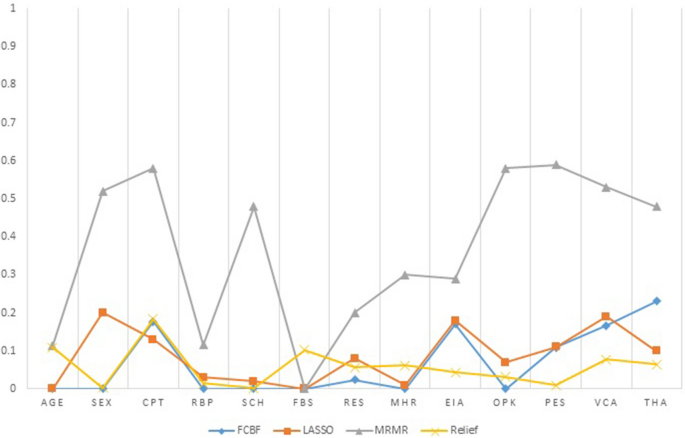
Features ranking by four feature selection algorithms (FCBF, LASSO, mRMR, Relief).
Least absolute shrinkage and selection operator (LASSO) LASSO selects features based on updating the absolute value of the features coefficient. In updating the features coefficient values, zero becoming values are removed from the features subset. LASSO outperforms with low feature coefficient values. The features having high coefficient values will be selected in the subset of features and the rest will be eliminated. Moreover, some irrelevant features with higher coefficient values may be selected and are included in the subset of features 30 . Table 14 represents the six most profound attributes which have a great correlation with the target and their scores selected by the LASSO feature selection algorithm. Figure 7 represents the important features and their scoring values given by the LASSO feature selection algorithm.
Relief feature selection algorithm Relief utilizes the concept of instance-based learning which allocates weight to each attribute based on its significance. The weight of each attribute demonstrates its capability to differentiate among class values. Attributes are rated by weights, and those attributes whose weight is exceeding a user-specified cutoff, are chosen as the final subset 34 . The relief feature selection algorithm selects the most significant attributes which have more effect on the target 35 . The algorithm operates by selecting instances randomly from the training samples. The nearest instance of the same class (nearest hit) and opposite class (nearest miss) is identified for each sampled instance. The weight of an attribute is updated according to how well its values differentiate between the sampled instance and its nearest miss and hit. If an attribute discriminates amongst instances from different classes and has the same value for instances of the same class, it will get a high weight.
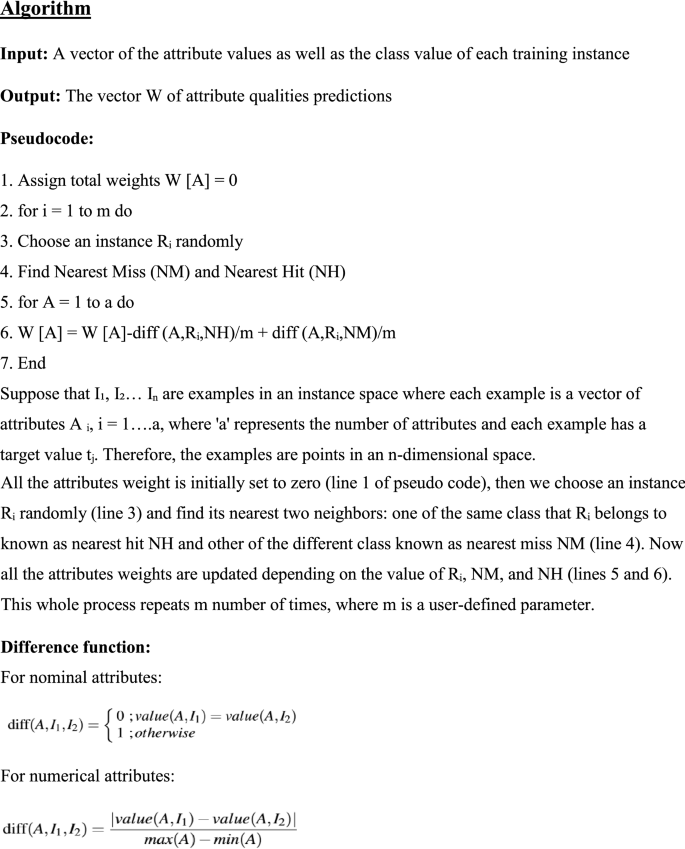
The weight updating of attributes works on a simple idea (line 6). That if instance R i and NH have dissimilar value (i.e. the diff value is large), that means the attribute splits two instances with the same class which is not worthwhile, and thus we reduce the attributes weight. On the other hand, if the instance R i and NM have a distinct value that means the attribute separates the two instances with a different class, which is desirable. The six most important features selected by the Relief algorithm are listed in descending order in Table 15 . Based on weight values the most vital features are CPT and Age. Figure 7 demonstrates the important features and their ranking given by the Relief feature selection algorithm.
Machine learning classification algorithms
Various machine learning classification algorithms are investigated for early detection of heart disease, in this study. Each classification algorithm has its significance and the importance is reported varied from application to application. In this paper, 10 distant nature of classification algorithms namely: KNN, DT, ET, GB, RF, SVM, AB, NB, LR, and ANN are applied to select the best and generalize prediction model.
Classifier validation method
Validation of the prediction model is an essential step in machine learning processes. In this paper, the K-Fold cross-validation method is applied to validating the results of the above-mentioned classification models.
K-fold cross validation (CV)
In K-Fold CV, the whole dataset is split into k equal parts. The (k-1) parts are utilized for training and the rest is used for the testing at each iteration. This process continues for k-iteration. Various researchers have used different values of k for CV. Here k = 10 is used for experimental work because it produces good results. In tenfold CV, 90% of data is utilized for training the model and the remaining 10% of data is used for the testing of the model at each iteration. At last, the mean of the results of each step is taken which is the final result.
Performance evaluation metrics
For measuring the performance of the classification algorithms used in this paper, various evaluation matrices have been implemented including accuracy, sensitivity, specificity, f1-score, recall, Mathew Correlation-coefficient (MCC), AUC-score, and ROC curve. All these measures are calculated from the confusion matrix described in Table 16 .
In confusion matrix True Negative (TN) shows that the patient has not heart disease and the model also predicts the same i.e. a healthy person is correctly classified by the model.
True Positive (TP) represents that the patient has heart disease and the model also predicts the same result i.e. a person having heart disease is correctly classified by the model.
False Positive (FP) demonstrates that the patient has not heart disease but the model predicted that the patient has i.e. a healthy person is incorrectly classified by the model. This is also called a type-1 error.
False Negative (FN) notifies that the patient has heart disease but the model predicted that the patient has not i.e. a person having heart disease is incorrectly classified by the model. This is also called a type-2 error.
Accuracy Accuracy of the classification model shows the overall performance of the model and can be calculated by the formula given below:
Specificity specificity is a ratio of the recently classified healthy people to the total number of healthy people. It means the prediction is negative and the person is healthy. The formula for calculating specificity is given as follows:
Sensitivity Sensitivity is the ratio of recently classified heart patients to the total patients having heart disease. It means the model prediction is positive and the person has heart disease. The formula for calculating sensitivity is given below:
Precision: Precision is the ratio of the actual positive score and the positive score predicted by the classification model/algorithm. Precision can be calculated by the following formula:
F1-score F1 is the weighted measure of both recall precision and sensitivity. Its value ranges between 0 and 1. If its value is one then it means the good performance of the classification algorithm and if its value is 0 then it means the bad performance of the classification algorithm.
MCC It is a correlation coefficient between the actual and predicted results. MCC gives resulting values between − 1 and + 1. Where − 1 represents the completely wrong prediction of the classifier.0 means that the classifier generates random prediction and + 1 represents the ideal prediction of the classification models. The formula for calculating MCC values is given below:
Finally, we will examine the predictability of the machine learning classification algorithms with the help of the receiver optimistic curve (ROC) which represents a graphical demonstration of the performance of ML classifiers. The area under the curve (AUC) describes the ROC of a classifier and the performance of the classification algorithms is directly linked with AUC i.e. larger the value of AUC greater will be the performance of the classification algorithm.
In this study, 10 different machine learning classification algorithms namely: LR, DT, NB, RF, ANN, KNN, GB, SVM, AB, and ET are implemented in order to select the best model for early and accurate detection of heart disease. Four feature selection algorithms such as FCBF, mRMR, LASSO, and Relief have been used to select the most vital and correlated features that truly reflect the motif of the desired target. Our developed intelligent computational model has been trained and tested on two datasets i.e. Cleveland (S1) and Hungarian (S2) heart disease datasets. Python has been used as a tool for implementation and simulating the results of all the utilized classification algorithms.
The performance of all classification models has been tested in terms of various performance metrics on full feature space as well as selected feature spaces, selected through various feature selection algorithms. This research study recommends that which feature selection algorithm is feasible with which classification model for developing a high-level intelligent system for the diagnosis of a patient having heart disease. From simulation results, it is observed that ET is the best classifier while relief is the optimal feature selection algorithm. In addition, P-value and Chi-square are also computed for the ET classifier along with each feature selection algorithm. It is anticipated that the proposed system will be useful and helpful for the doctors and other care-givers to diagnose a patient having heart disease accurately and effectively at the early stages.
Heart disease is one of the most devastating and fatal chronic diseases that rapidly increase in both economically developed and undeveloped countries and causes death. This damage can be reduced considerably if the patient is diagnosed in the early stages and proper treatment is provided to her. In this paper, we developed an intelligent predictive system based on contemporary machine learning algorithms for the prediction and diagnosis of heart disease. The developed system was checked on two datasets i.e. Cleveland (S1) and Hungarian (S2) heart disease datasets. The developed system was trained and tested on full features and optimal features as well. Ten classification algorithms including, KNN, DT, RF, NB, SVM, AB, ET, GB, LR, and ANN, and four feature selection algorithms such as FCBF, mRMR, LASSO, and Relief are used. The feature selection algorithm selects the most significant features from the feature space, which not only reduces the classification errors but also shrink the feature space. To assess the performance of classification algorithms various performance evaluation metrics were used such as accuracy, sensitivity, specificity, AUC, F1-score, MCC, and ROC curve. The classification accuracies of the top two classification algorithms i.e. ET and GB on full features were 92.09% and 91.34% respectively. After applying feature selection algorithms, the classification accuracy of ET with the relief feature selection algorithm increases from 92.09 to 94.41%. The accuracy of GB increases from 91.34 to 93.36% with the FCBF feature selection algorithm. So, the ET classifier with the relief feature selection algorithm performs excellently. P-value and Chi-square are also computed for the ET classifier with each feature selection technique. The future work of this research study is to use more optimization techniques, feature selection algorithms, and classification algorithms to improve the performance of the predictive system for the diagnosis of heart disease.
Bui, A. L., Horwich, T. B. & Fonarow, G. C. Epidemiology and risk profile of heart failure. Nat. Rev. Cardiol. 8 , 30 (2011).
Article PubMed Google Scholar
Polat, K. & Güneş, S. Artificial immune recognition system with fuzzy resource allocation mechanism classifier, principal component analysis, and FFT method based new hybrid automated identification system for classification of EEG signals. Expert Syst. Appl. 34 , 2039–2048 (2010).
Article Google Scholar
Heidenreich, P. A. et al. Forecasting the future of cardiovascular disease in the United States: A policy statement from the American Heart Association. Circulation 123 , 933–944 (2011).
Durairaj, M. & Ramasamy, N. A comparison of the perceptive approaches for preprocessing the data set for predicting fertility success rate. Int. J. Control Theory Appl. 9 , 255–260 (2016).
Google Scholar
Das, R., Turkoglu, I. & Sengur, A. Effective diagnosis of heart disease through neural networks ensembles. Expert Syst. Appl. 36 , 7675–7680 (2012).
Allen, L. A. et al. Decision making in advanced heart failure: A scientific statement from the American Heart Association. Circulation 125 , 1928–1952 (2014).
Yang, H. & Garibaldi, J. M. A hybrid model for automatic identification of risk factors for heart disease. J. Biomed. Inform. 58 , S171–S182 (2015).
Article PubMed PubMed Central Google Scholar
Alizadehsani, R., Hosseini, M. J., Sani, Z. A., Ghandeharioun, A. & Boghrati, R. In 2012 IEEE 12th International Conference on Data Mining Workshops. 9–16 (IEEE, New York).
Arabasadi, Z., Alizadehsani, R., Roshanzamir, M., Moosaei, H. & Yarifard, A. A. Computer aided decision making for heart disease detection using hybrid neural network-Genetic algorithm. Comput. Methods Programs Biomed. 141 , 19–26 (2017).
Samuel, O. W., Asogbon, G. M., Sangaiah, A. K., Fang, P. & Li, G. An integrated decision support system based on ANN and Fuzzy_AHP for heart failure risk prediction. Expert Syst. Appl. 68 , 163–172 (2017).
Patil, S. B. & Kumaraswamy, Y. Intelligent and effective heart attack prediction system using data mining and artificial neural network. Eur. J. Sci. Res. 31 , 642–656 (2009).
Vanisree, K. & Singaraju, J. Decision support system for congenital heart disease diagnosis based on signs and symptoms using neural networks. Int. J. Comput. Appl. 19 , 6–12 (2015).
B. Edmonds. In Proceedings of AISB Symposium on Socially Inspired Computing 1–12 (Hatfield, 2005).
Methaila, A., Kansal, P., Arya, H. & Kumar, P. Early heart disease prediction using data mining techniques. Comput. Sci. Inf. Technol. J. https://doi.org/10.5121/csit.2014.4807 (2014).
Samuel, O. W., Asogbon, G. M., Sangaiah, A. K., Fang, P. & Li, G. An integrated decision support system based on ANN and Fuzzy_AHP for heart failure risk prediction. Expert Syst. Appl. 68 , 163–172 (2018).
Nazir, S., Shahzad, S., Mahfooz, S. & Nazir, M. Fuzzy logic based decision support system for component security evaluation. Int. Arab J. Inf. Technol. 15 , 224–231 (2018).
Detrano, R. et al. International application of a new probability algorithm for the diagnosis of coronary artery disease. Am. J. Cardiol. 64 , 304–310 (2009).
Gudadhe, M., Wankhade, K. & Dongre, S. In 2010 International Conference on Computer and Communication Technology (ICCCT) , 741–745 (IEEE, New York).
Kahramanli, H. & Allahverdi, N. Design of a hybrid system for the diabetes and heart diseases. Expert Syst. Appl. 35 , 82–89 (2013).
Palaniappan, S. & Awang, R. In 2012 IEEE/ACS International Conference on Computer Systems and Applications 108–115 (IEEE, New York).
Olaniyi, E. O., Oyedotun, O. K. & Adnan, K. Heart diseases diagnosis using neural networks arbitration. Int. J. Intel. Syst. Appl. 7 , 72 (2015).
Das, R., Turkoglu, I. & Sengur, A. Effective diagnosis of heart disease through neural networks ensembles. Expert Syst. Appl. 36 , 7675–7680 (2011).
Paul, A. K., Shill, P. C., Rabin, M. R. I. & Murase, K. Adaptive weighted fuzzy rule-based system for the risk level assessment of heart disease. Applied Intelligence 48 , 1739–1756 (2018).
Tomov, N.-S. & Tomov, S. On deep neural networks for detecting heart disease. arXiv:1808.07168 (2018).
Manogaran, G., Varatharajan, R. & Priyan, M. Hybrid recommendation system for heart disease diagnosis based on multiple kernel learning with adaptive neuro-fuzzy inference system. Multimedia Tools Appl. 77 , 4379–4399 (2018).
Alizadehsani, R. et al. Non-invasive detection of coronary artery disease in high-risk patients based on the stenosis prediction of separate coronary arteries. Comput. Methods Programs Biomed. 162 , 119–127 (2018).
Haq, A. U., Li, J. P., Memon, M. H., Nazir, S. & Sun, R. A hybrid intelligent system framework for the prediction of heart disease using machine learning algorithms. Mobile Inf. Syst. 2018 , 3860146. https://doi.org/10.1155/2018/3860146 (2018).
Mohan, S., Thirumalai, C. & Srivastava, G. Effective heart disease prediction using hybrid machine learning techniques. IEEE Access 7 , 81542–81554 (2019).
Ali, L. et al. An optimized stacked support vector machines based expert system for the effective prediction of heart failure. IEEE Access 7 , 54007–54014 (2019).
Peng, H., Long, F. & Ding, C. Feature selection based on mutual information criteria of max-dependency, max-relevance, and min-redundancy. IEEE Trans. Pattern Anal. Mach. Intell. 27 (8), 1226–1238 (2005).
Palaniappan, S. & Awang, R. In 2008 IEEE/ACS International Conference on Computer Systems and Applications 108–115 (IEEE, New York).
Ali, L., Niamat, A., Golilarz, N. A., Ali, A. & Xingzhong, X. An expert system based on optimized stacked support vector machines for effective diagnosis of heart disease. IEEE Access (2019).
Pérez, N. P., López, M. A. G., Silva, A. & Ramos, I. Improving the Mann-Whitney statistical test for feature selection: An approach in breast cancer diagnosis on mammography. Artif. Intell. Med. 63 , 19–31 (2015).
Tibshirani, R. Regression shrinkage and selection via the lasso: A retrospective. J. R. Stat. Soc. Ser. B Stat. Methodol. 73 , 273–282 (2011).
Article MathSciNet Google Scholar
Peng, H., Long, F. & Ding, C. Feature selection based on mutual information criteria of max-dependency, max-relevance, and min-redundancy. IEEE Trans. Pattern Anal. Mach. Intell. 27 , 1226–1238 (2012).
de Silva, A. M. & Leong, P. H. Grammar-Based Feature Generation for Time-Series Prediction (Springer, Berlin, 2015).
Book Google Scholar
Download references
Acknowledgements
This research was supported by the Brain Research Program of the National Research Foundation (NRF) funded by the Korean government (MSIT) (No. NRF-2017M3C7A1044815).
Author information
Authors and affiliations.
Department of Computer Science, Abdul Wali Khan University Mardan, Mardan, 23200, KP, Pakistan
Yar Muhammad, Muhammad Tahir & Maqsood Hayat
Department of Electronic and Information Engineering, Jeonbuk National University, Jeonju, 54896, South Korea
Kil To Chong
You can also search for this author in PubMed Google Scholar
Contributions
All authors have equal contributions.
Corresponding authors
Correspondence to Maqsood Hayat or Kil To Chong .
Ethics declarations
Competing interests.
The authors declare no competing interests.
Additional information
Publisher's note.
Springer Nature remains neutral with regard to jurisdictional claims in published maps and institutional affiliations.
Supplementary information
Supplementary information., rights and permissions.
Open Access This article is licensed under a Creative Commons Attribution 4.0 International License, which permits use, sharing, adaptation, distribution and reproduction in any medium or format, as long as you give appropriate credit to the original author(s) and the source, provide a link to the Creative Commons licence, and indicate if changes were made. The images or other third party material in this article are included in the article's Creative Commons licence, unless indicated otherwise in a credit line to the material. If material is not included in the article's Creative Commons licence and your intended use is not permitted by statutory regulation or exceeds the permitted use, you will need to obtain permission directly from the copyright holder. To view a copy of this licence, visit http://creativecommons.org/licenses/by/4.0/ .
Reprints and permissions
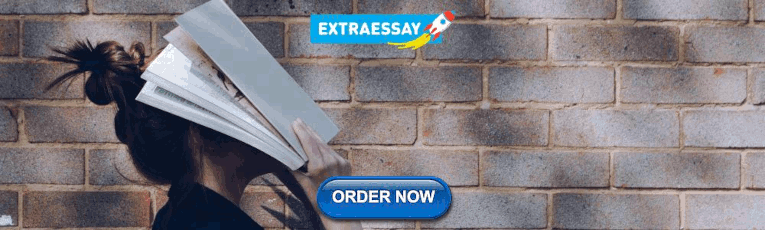
About this article
Cite this article.
Muhammad, Y., Tahir, M., Hayat, M. et al. Early and accurate detection and diagnosis of heart disease using intelligent computational model. Sci Rep 10 , 19747 (2020). https://doi.org/10.1038/s41598-020-76635-9
Download citation
Received : 03 April 2020
Accepted : 28 October 2020
Published : 12 November 2020
DOI : https://doi.org/10.1038/s41598-020-76635-9
Share this article
Anyone you share the following link with will be able to read this content:
Sorry, a shareable link is not currently available for this article.
Provided by the Springer Nature SharedIt content-sharing initiative
This article is cited by
Comprehensive evaluation and performance analysis of machine learning in heart disease prediction.
- Halah A. Al-Alshaikh
- Abeer A. AlSanad
Scientific Reports (2024)
Heart Disease Prediction Using Weighted K-Nearest Neighbor Algorithm
- Khalidou Abdoulaye Barry
- Youness Manzali
- Mohamed Elfar
Operations Research Forum (2024)
Future prediction for precautionary measures associated with heart-related issues based on IoT prototype
- Ganesh Keshaorao Yenurkar
- Aniket Pathade
Multimedia Tools and Applications (2024)
An improved machine learning-based prediction framework for early detection of events in heart failure patients using mHealth
- Deepak Kumar
- Keerthiveena Balraj
- Anurag S. Rathore
Health and Technology (2024)
Identification and classification of pneumonia disease using a deep learning-based intelligent computational framework
- Lanying Tang
Neural Computing and Applications (2023)
By submitting a comment you agree to abide by our Terms and Community Guidelines . If you find something abusive or that does not comply with our terms or guidelines please flag it as inappropriate.
Quick links
- Explore articles by subject
- Guide to authors
- Editorial policies
Sign up for the Nature Briefing newsletter — what matters in science, free to your inbox daily.


An official website of the United States government
Here’s how you know
Official websites use .gov A .gov website belongs to an official government organization in the United States.
Secure .gov websites use HTTPS A lock ( A locked padlock ) or https:// means you’ve safely connected to the .gov website. Share sensitive information only on official, secure websites.
- Heart-Healthy Living
- High Blood Pressure
- Sickle Cell Disease
- Sleep Apnea
- Information & Resources on COVID-19
- The Heart Truth®
- Learn More Breathe Better®
- Blood Diseases & Disorders Education Program
- Publications and Resources
- Clinical Trials
- Blood Disorders and Blood Safety
- Sleep Science and Sleep Disorders
- Lung Diseases
- Health Disparities and Inequities
- Heart and Vascular Diseases
- Precision Medicine Activities
- Obesity, Nutrition, and Physical Activity
- Population and Epidemiology Studies
- Women’s Health
- Research Topics
- All Science A-Z
- Grants and Training Home
- Policies and Guidelines
- Funding Opportunities and Contacts
- Training and Career Development
- Email Alerts
- NHLBI in the Press
- Research Features
- Ask a Scientist
- Past Events
- Upcoming Events
- Mission and Strategic Vision
- Divisions, Offices and Centers
- Advisory Committees
- Budget and Legislative Information
- Jobs and Working at the NHLBI
- Contact and FAQs
- NIH Sleep Research Plan
- < Back To Research Topics
Coronary Heart Disease Research
Language switcher.
For almost 75 years, the NHLBI has been at the forefront of improving the nation’s health and reducing the burden of heart and vascular diseases . Heart disease, including coronary heart disease, remains the leading cause of death in the United States. However, the rate of heart disease deaths has declined by 70% over the past 50 years, thanks in part to NHLBI-funded research. Many current studies funded by the NHLBI focus on discovering genetic associations and finding new ways to prevent and treat the onset of coronary heart disease and associated medical conditions.

NHLBI research that really made a difference
The NHLBI supports a wide range of long-term studies to understand the risk factors of coronary heart disease. These ongoing studies, among others, have led to many discoveries that have increased our understanding of the causes of cardiovascular disease among different populations, helping to shape evidence-based clinical practice guidelines.
- Risk factors that can be changed: The NHLBI Framingham Heart Study (FHS) revealed that cardiovascular disease is caused by modifiable risk factors such as smoking, high blood pressure , obesity , high cholesterol levels, and physical inactivity. It is why, in routine physicals, healthcare providers check for high blood pressure, high cholesterol, unhealthy eating patterns, smoking, physical inactivity, and unhealthy weight. The FHS found that cigarette smoking increases the risk of heart disease. Researchers also showed that cardiovascular disease can affect people differently depending on sex or race, underscoring the need to address health disparities.
- Risk factors for Hispanic/Latino adults: The Hispanic Community Health Study/Study of Latinos (HCHS/SOL) found that heart disease risk factors are widespread among Hispanic/Latino adults in the United States , with 80% of men and 71% of women having at least one risk factor. Researchers also used HCHS/SOL genetic data to explore genes linked with central adiposity (the tendency to have excess body fat around the waist) in Hispanic/Latino adults. Before this study, genes linked with central adiposity, a risk factor for coronary heart disease, had been identified in people of European ancestry. These results showed that those genes also predict central adiposity for Hispanic/Latino communities. Some of the genes identified were more common among people with Mexican or Central/South American ancestry, while others were more common among people of Caribbean ancestry.
- Risk factors for African Americans: The Jackson Heart Study (JHS) began in 1997 and includes more than 5,300 African American men and women in Jackson, Mississippi. It has studied genetic and environmental factors that raise the risk of heart problems, especially high blood pressure, coronary heart disease, heart failure , stroke , and peripheral artery disease (PAD) . Researchers discovered a gene variant in African American individuals that doubles the risk of heart disease. They also found that even small spikes in blood pressure can lead to a higher risk of death. A community engagement component of the JHS is putting 20 years of the study’s findings into action by turning traditional gathering places, such as barbershops and churches, into health information hubs.
- Risk factors for American Indians: The NHLBI actively supports the Strong Heart Study , a long-term study that began in 1988 to examine cardiovascular disease and its risk factors among American Indian men and women. The Strong Heart Study is one of the largest epidemiological studies of American Indian people ever undertaken. It involves a partnership with 12 Tribal Nations and has followed more than 8,000 participants, many of whom live in low-income rural areas of Arizona, Oklahoma, and the Dakotas. Cardiovascular disease remains the leading cause of death for American Indian people. Yet the prevalence and severity of cardiovascular disease among American Indian people has been challenging to study because of the small sizes of the communities, as well as the relatively young age, cultural diversity, and wide geographic distribution of the population. In 2019, the NHLBI renewed its commitment to the Strong Heart Study with a new study phase that includes more funding for community-driven pilot projects and a continued emphasis on training and development. Read more about the goals and key findings of the Strong Heart Study.
Current research funded by the NHLBI
Within our Division of Cardiovascular Sciences , the Atherothrombosis and Coronary Artery Disease Branch of its Adult and Pediatric Cardiac Research Program and the Center for Translation Research and Implementation Science oversee much of our funded research on coronary heart disease.
Research funding
Find funding opportunities and program contacts for research on coronary heart disease.
Current research on preventing coronary heart disease
- Blood cholesterol and coronary heart disease: The NHLBI supports new research into lowering the risk of coronary heart disease by reducing levels of cholesterol in the blood. High levels of blood cholesterol, especially a type called low-density lipoprotein (LDL) cholesterol, raise the risk of coronary heart disease. However, even with medicine that lowers LDL cholesterol, there is still a risk of coronary heart disease due to other proteins, called triglyceride-rich ApoB-containing lipoproteins (ApoBCLs), that circulate in the blood. Researchers are working to find innovative ways to reduce the levels of ApoBCLs, which may help prevent coronary heart disease and other cardiovascular conditions.
- Pregnancy, preeclampsia, and coronary heart disease risk: NHLBI-supported researchers are investigating the link between developing preeclampsia during pregnancy and an increased risk for heart disease over the lifespan . This project uses “omics” data – such as genomics, proteomics, and other research areas – from three different cohorts of women to define and assess preeclampsia biomarkers associated with cardiovascular health outcomes. Researchers have determined that high blood pressure during pregnancy and low birth weight are predictors of atherosclerotic cardiovascular disease in women . Ultimately, these findings can inform new preventive strategies to lower the risk of coronary heart disease.
- Community-level efforts to lower heart disease risk among African American people: The NHLBI is funding initiatives to partner with churches in order to engage with African American communities and lower disparities in heart health . Studies have found that church-led interventions reduce risk factors for coronary heart disease and other cardiovascular conditions. NHLBI-supported researchers assessed data from more than 17,000 participants across multiple studies and determined that these community-based approaches are effective in lowering heart disease risk factors .
Find more NHLBI-funded studies on preventing coronary heart disease on the NIH RePORTER.

Learn about the impact of COVID-19 on your risk of coronary heart disease.
Current research on understanding the causes of coronary heart disease
- Pregnancy and long-term heart disease: NHLBI researchers are continuing the Nulliparous Pregnancy Outcomes Study: Monitoring Mothers-to-be (nuMoM2b) study to understand the relationship between pregnancy-related problems, such as gestational hypertension, and heart problems. The study also looks at how problems during pregnancy may increase risk factors for heart disease later in life. NuMoM2b launched in 2010, and long-term studies are ongoing, with the goal of collecting high-quality data and understanding how heart disease develops in women after pregnancy.
- How coronary artery disease affects heart attack risk: NHLBI-funded researchers are investigating why some people with coronary artery disease are more at risk for heart attacks than others. Researchers have found that people with coronary artery disease who have high-risk coronary plaques are more likely to have serious cardiac events, including heart attacks. However, we do not know why some people develop high-risk coronary plaques and others do not. Researchers hope that this study will help providers better identify which people are most at risk of heart attacks before they occur.
- Genetics of coronary heart disease: The NHLBI supports studies to identify genetic variants associated with coronary heart disease . Researchers are investigating how genes affect important molecular cascades involved in the development of coronary heart disease . This deeper understanding of the underlying causes for plaque buildup and damage to the blood vessels can inform prevention strategies and help healthcare providers develop personalized treatment for people with coronary heart disease caused by specific genetic mutations.
Find more NHLBI-funded studies on understanding the causes of coronary heart disease on the NIH RePORTER.

Recent findings suggest that cholesterol-lowering treatment can lower the risk of heart disease complications in people with HIV.
Current research on treatments for coronary heart disease
- Insight into new molecular targets for treatment: NHLBI-supported researchers are investigating the role of high-density lipoprotein (HDL) cholesterol in coronary heart disease and other medical conditions . Understanding how the molecular pathways of cholesterol affect the disease mechanism for atherosclerosis and plaque buildup in the blood vessels of the heart can lead to new therapeutic approaches for the treatment of coronary heart disease. Researchers have found evidence that treatments that boost HDL function can lower systemic inflammation and slow down plaque buildup . This mechanism could be targeted to develop a new treatment approach for coronary heart disease.
- Long-term studies of treatment effectiveness: The NHLBI is supporting the International Study of Comparative Health Effectiveness with Medical and Invasive Approaches (ISCHEMIA) trial EXTENDed Follow-up (EXTEND) , which compares the long-term outcomes of an initial invasive versus conservative strategy for more than 5,000 surviving participants of the original ISCHEMIA trial. Researchers have found no difference in mortality outcomes between invasive and conservative management strategies for patients with chronic coronary heart disease after more than 3 years. They will continue to follow up with participants for up to 10 years. Researchers are also assessing the impact of nonfatal events on long-term heart disease and mortality. A more accurate heart disease risk score will be constructed to help healthcare providers deliver more precise care for their patients.
- Evaluating a new therapy for protecting new mothers: The NHLBI is supporting the Randomized Evaluation of Bromocriptine In Myocardial Recovery Therapy for Peripartum Cardiomyopathy (REBIRTH) , for determining the role of bromocriptine as a treatment for peripartum cardiomyopathy (PPCM). Previous research suggests that prolactin, a hormone that stimulates the production of milk for breastfeeding, may contribute to the development of cardiomyopathy late in pregnancy or the first several months postpartum. Bromocriptine, once commonly used in the United States to stop milk production, has shown promising results in studies conducted in South Africa and Germany. Researchers will enroll approximately 200 women across North America who have been diagnosed with PPCM and assess their heart function after 6 months.
- Impact of mental health on response to treatment: NHLBI-supported researchers are investigating how mental health conditions can affect treatment effectiveness for people with coronary heart disease. Studies show that depression is linked to a higher risk for negative outcomes from coronary heart disease. Researchers found that having depression is associated with poor adherence to medical treatment for coronary heart disease . This means that people with depression are less likely to follow through with their heart disease treatment plans, possibly contributing to their chances of experiencing worse outcomes. Researchers are also studying new ways to treat depression in patients with coronary heart disease .
Find more NHLBI-funded studies on treating coronary heart disease on the NIH RePORTER.

Researchers have found no clear difference in patient survival or heart attack risk between managing heart disease through medication and lifestyle changes compared with invasive procedures.
Coronary heart disease research labs at the NHLBI
- Laboratory of Cardiac Physiology
- Laboratory of Cardiovascular Biology
- Minority Health and Health Disparities Population Laboratory
- Social Determinants of Obesity and Cardiovascular Risk Laboratory
- Laboratory for Cardiovascular Epidemiology and Genomics
- Laboratory for Hemostasis and Platelet Biology
Related coronary heart disease programs
- In 2002, the NHLBI launched The Heart Truth® , the first federally sponsored national health education program designed to raise awareness about heart disease as the leading cause of death in women. The NHLBI and The Heart Truth® supported the creation of the Red Dress® as the national symbol for awareness about women and heart disease, and also coordinate National Wear Red Day ® and American Heart Month each February.
- The Biologic Specimen and Data Repository Information Coordinating Center (BioLINCC) facilitates access to and maximizes the scientific value of NHLBI biospecimen and data collections. A main goal is to promote the use of these scientific resources by the broader research community. BioLINCC serves to coordinate searches across data and biospecimen collections and provide an electronic means for requesting additional information and submitting requests for collections. Researchers wanting to submit biospecimen collections to the NHLBI Biorepository to share with qualified investigators may also use the website to initiate the application process.
- Our Trans-Omics for Precision Medicine (TOPMed) Program studies the ways genetic information, along with information about health status, lifestyle, and the environment, can be used to predict the best ways to prevent and treat heart, lung, blood, and sleep disorders. TOPMed specifically supports NHLBI’s Precision Medicine Activities.
- NHLBI population and epidemiology studies in different groups of people, including the Atherosclerosis Risk in Communities (ARIC) Study , the Multi-Ethnic Study of Atherosclerosis (MESA) , and the Cardiovascular Health Study (CHS) , have made major contributions to understanding the causes and prevention of heart and vascular diseases, including coronary heart disease.
- The Cardiothoracic Surgical Trials Network (CTSN) is an international clinical research enterprise that studies heart valve disease , arrhythmias , heart failure, coronary heart disease, and surgical complications. The trials span all phases of development, from early translation to completion, and have more than 14,000 participants. The trials include six completed randomized clinical trials, three large observational studies, and many other smaller studies.

Learn how heart disease may be different for women than for men.
Explore more NHLBI research on coronary heart disease
The sections above provide you with the highlights of NHLBI-supported research on coronary heart disease. You can explore the full list of NHLBI-funded studies on the NIH RePORTER .
To find more studies:
- Type your search words into the Quick Search box and press enter.
- Check Active Projects if you want current research.
- Select the Agencies arrow, then the NIH arrow, then check NHLBI .
If you want to sort the projects by budget size — from the biggest to the smallest — click on the FY Total Cost by IC column heading.
Watch CBS News
Catching up on sleep on weekends may lower risk of heart disease, research finds
By Sara Moniuszko
Edited By Allison Elyse Gualtieri
August 29, 2024 / 11:52 AM EDT / CBS News
Busy week have you catching up on sleep during the weekend? That may be good for your heart health , according to new research.
In a study set to be presented at the European Society of Cardiology Congress on Sunday, researchers found the risk of heart disease fell about 20% for people who slept in on the weekends to catch up on sleep lost earlier in the week.
The study used data from 90,903 people, whose sleep patterns were self-reported. In the study, sleep deprivation was defined as having less than seven hours of sleep per night. A total of 19,816 participants were categorized as sleep deprived and underwent a health follow-up over a decade later.
"Our results show that for the significant proportion of the population in modern society that suffers from sleep deprivation, those who have the most 'catch-up' sleep at weekends have significantly lower rates of heart disease than those with the least," co-author Zechen Liu said in a news release .
The study found no differences between men and women, but results did differ depending on how much sleep was lost.
"The association becomes even more pronounced among individuals who regularly experience inadequate sleep on weekdays," co-author Yanjun Song said in the release.
This study has not yet been published by a peer-reviewed publication, but was selected to be presented at the cardiology conference in London.
Previous research has shown poor sleep is bad for our health — but there are ways to improve sleep hygiene , including adjusting sleep schedules and environments to be primed for good rest.
Experts also suggest focusing on sleep quality over quantity. Strategies for improving sleep quality include limiting alcohol and caffeine intake, reducing screen time before bed and managing how much liquid you drink before bed.
- Heart Disease
Sara Moniuszko is a health and lifestyle reporter at CBSNews.com. Previously, she wrote for USA Today, where she was selected to help launch the newspaper's wellness vertical. She now covers breaking and trending news for CBS News' HealthWatch.
More from CBS News
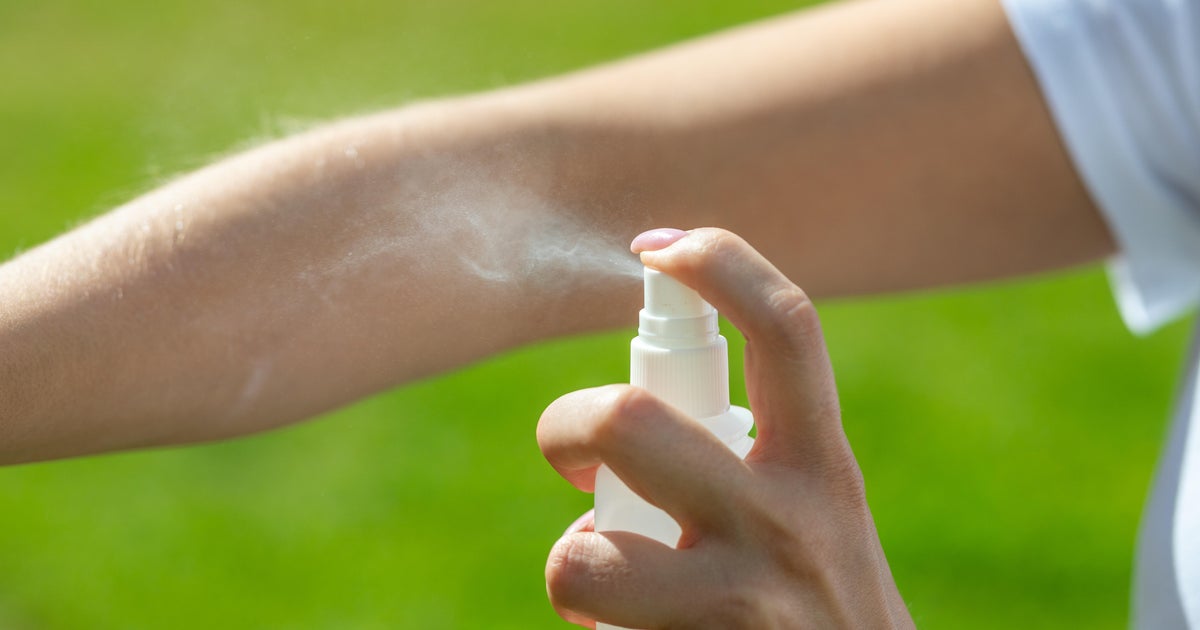
What is EEE? Mosquito-borne virus symptoms and prevention, explained
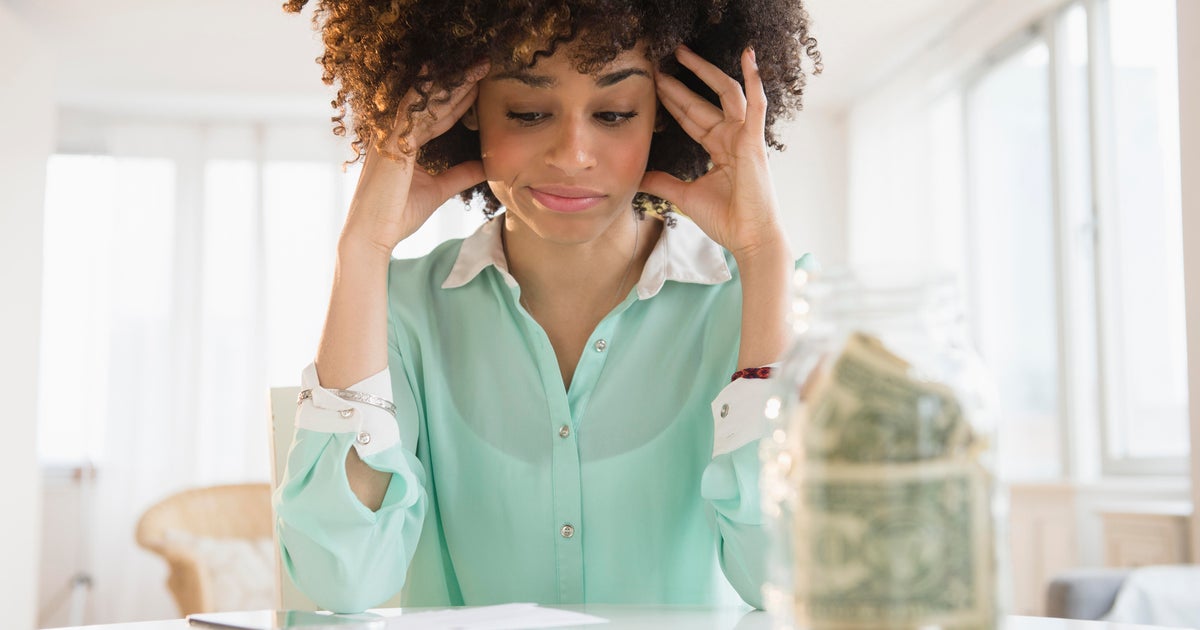
These are the financial moves Americans regret the most
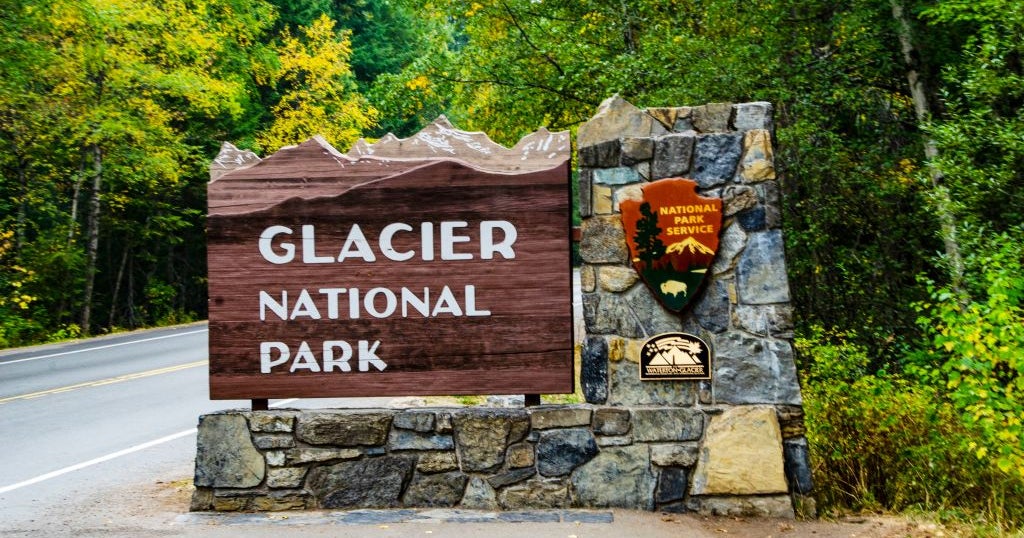
Missing climber found dead from apparent fall at Glacier National Park
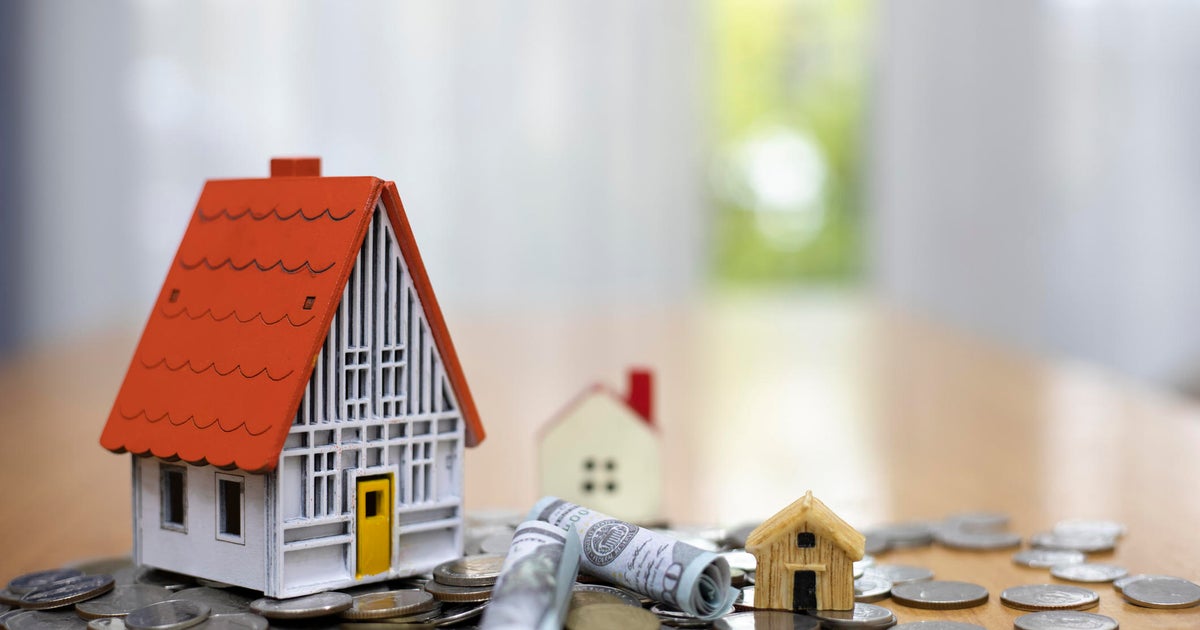
How much would a $350,000 mortgage cost per month?
Living in tree-filled neighborhoods may reduce risk of heart disease, study shows
Living in a tree-filled neighborhood may be as beneficial to the heart as regular exercise, new research shows.
Researchers at the University of Louisville designed a clinical trial that followed hundreds of people living in six low- to middle-income neighborhoods in South Louisville, Kentucky. They used blood and other samples to better understand how their heart risks changed before and after the team planted thousands of mature trees near their homes.
Results from the Green Heart Louisville Project ’s HEAL Study , released Tuesday, showed that people living in neighborhoods with twice as many trees and shrubs had lower levels of a blood marker associated with heart disease, diabetes and some types of cancer compared with those who lived in more tree-bare neighborhoods.
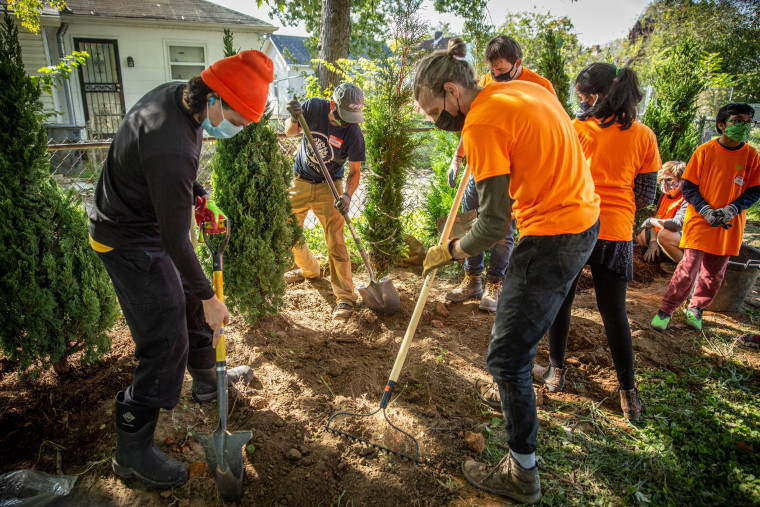
“We are trying to see if we can decrease the rates of heart disease in a community,” said Aruni Bhatnagar, a professor of medicine at the University of Louisville, who led the project.
Most previous studies showing the effects of nature on mental and physical health are observational and can’t answer whether people who live in green communities are healthier because they’re wealthier and have access to better health care.
The HEAL study was set up with a control group and an intervention, meaning something measurable that some of the participants were exposed to during the study but not before.
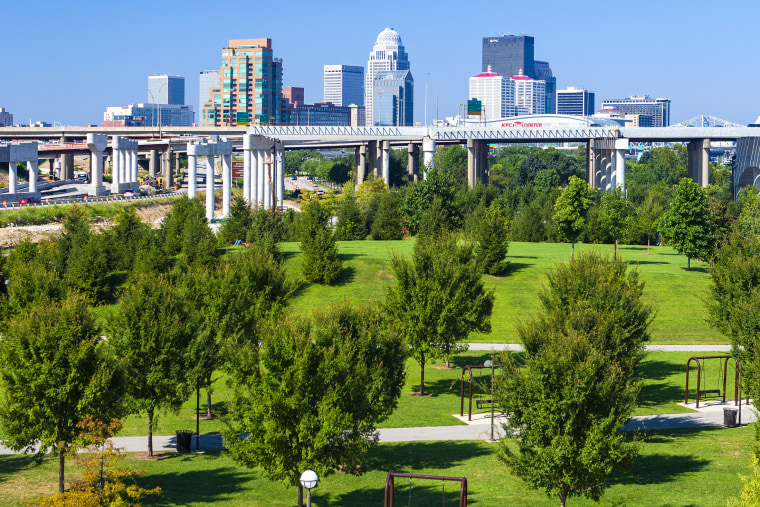
Bhatnagar and his team recruited about 750 people living in a 4-mile area of South Louisville cut by a highway. The residents were 25 to 75 years old.
Nearly 80% were white, and 60% identified as female. Half reported average household incomes of $50,000.
The researchers collected blood, urine, nail and hair samples, as well as health data, from each person before they began their intervention.
Then, from 2019 to 2022, they planted nearly 8,500 evergreen trees, 630 deciduous trees — the type that lose leaves in the fall — and 45 different types of shrubs in parts of the 4-mile study area, leaving others untouched.
Last year and this year, they took new samples from residents living in both areas.
People living in the intervention areas had 13% lower levels of high-sensitivity C-reactive protein , a blood marker associated with heart disease, including stroke, coronary artery disease and heart attack. The drop was similar to starting a regular exercise routine, Bhatnagar said.
“I wouldn’t have expected such a strong biomarker response, and that speaks to maybe something truly is causal here with how trees impact health,” said Peter James, director of the Center for Occupational and Environmental Health at the University of California, Davis School of Medicine, who wasn’t involved in the new research.
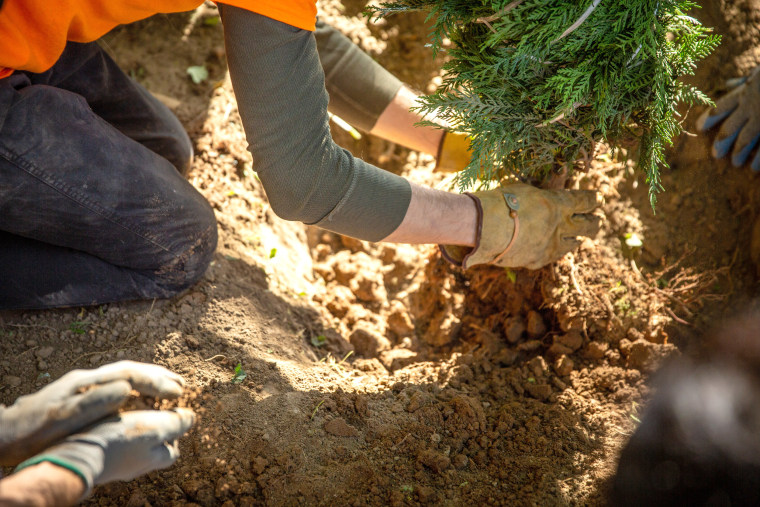
How trees can improve physical health
Previous research has shown spending time in green spaces boosts mental health .
The new study showed the connection between living among more trees and physical health.
Trees provide shade and cool the areas where they’re planted, helping quell the urban heat effect that disproportionately affects low-income neighborhoods and neighborhoods of color. Hot weather aggravates heart disease and can cause heatstroke in people without pre-existing conditions.
Trees also buffer noise, which is linked to higher rates of cardiovascular disease, James said.
“They provide areas for people to relax, exercise, and probably more importantly, socialize,” Joan Casey, an environmental epidemiologist and associate professor of environmental and occupational health sciences at the University of Washington, said in an email.
“They also replace other health-harmful land uses, like industrial sites,” she said.
Because one of the city’s major highways cuts through the study area, Bhatnagar and his team believe, trees’ ability to filter air pollution and buffer neighborhoods from constantly breathing in harmful particles could be a primary way the tree-planting intervention appeared to lower inflammation markers in people living in greened areas.
During the study, the project planted trees only in the parts of South Louisville that had the worst air quality. It took air quality samples before the project, and it is still analyzing how the new tree cover has affected pollution. It’s a complex undertaking, because air quality fluctuates based on the weather — a windy day might increase or decrease air pollution in certain areas, depending on the direction of the wind, and air pollution is worse on hotter days.
The project plans to plant trees in the control group neighborhoods in another three or four years if the intervention neighborhoods continue to show positive results. It also wants to determine whether tree cover improves sleep or children’s immune systems by encouraging outside play.
“There is no sort of ultimate proof,” Bhatnagar said. “But this is the strongest evidence of any study that’s ever been done on trees and their relationship to health.”
Growing evidence shows the importance of ensuring green spaces are equitably distributed around cities, which is currently not the case .
Casey said it’s important that city planners be careful not to create “green gentrification” when they create more equitable access to green spaces in cities — that is, when spaces such as water fronts are restored and housing prices increase as a result, making it unaffordable for current residents to continue living there once a green space is completed.
“The take-home message here is that nature is not an amenity; green spaces are not a perk for the wealthy. They are essential for us as human beings,” James said.
Kaitlin Sullivan is a contributor for NBCNews.com who has worked with NBC News Investigations. She reports on health, science and the environment and is a graduate of the Craig Newmark Graduate School of Journalism at City University of New York.

Anne Thompson is NBC News’ chief environmental affairs correspondent.
Warning: The NCBI web site requires JavaScript to function. more...

An official website of the United States government
The .gov means it's official. Federal government websites often end in .gov or .mil. Before sharing sensitive information, make sure you're on a federal government site.
The site is secure. The https:// ensures that you are connecting to the official website and that any information you provide is encrypted and transmitted securely.
- Publications
- Account settings
- Browse Titles
NCBI Bookshelf. A service of the National Library of Medicine, National Institutes of Health.
StatPearls [Internet]. Treasure Island (FL): StatPearls Publishing; 2024 Jan-.

StatPearls [Internet].
Cardiovascular disease.
Edgardo Olvera Lopez ; Brian D. Ballard ; Arif Jan .
Affiliations
Last Update: August 22, 2023 .
- Continuing Education Activity
The cardiovascular system consists of the heart and its blood vessels. A wide array of problems can arise within the cardiovascular system, a few of which include endocarditis, rheumatic heart disease, and conduction system abnormalities. Cardiovascular disease, also known as heart disease, refers to the following 4 entities: coronary artery disease (CAD) which is also referred to as coronary heart disease (CHD), cerebrovascular disease, peripheral artery disease (PAD), and aortic atherosclerosis. CAD results from decreased myocardial perfusion that causes angina due to ischemia and can result in myocardial infarction (MI), and/or heart failure. It accounts for one-third to one-half of all cases of cardiovascular disease. Cerebrovascular disease is the entity associated with strokes, also termed cerebrovascular accidents, and transient ischemic attacks (TIAs). Peripheral arterial disease (PAD) is arterial disease predominantly involving the limbs that may result in claudication. Aortic atherosclerosis is the entity associated with thoracic and abdominal aneurysms. This activity reviews the evaluation and treatment of cardiovascular disease and the role of the medical team in evaluating and treating these conditions.
- Review the cause of coronary artery disease.
- Describe the pathophysiology of atherosclerosis.
- Summarize the treatment options for heart disease.
- Outline the evaluation and treatment of cardiovascular disease and the role of the medical team in evaluating and treating this condition.
- Introduction
The cardiovascular system consists of the heart and blood vessels. [1] There is a wide array of problems that may arise within the cardiovascular system, for example, endocarditis, rheumatic heart disease, abnormalities in the conduction system, among others, cardiovascular disease (CVD) or heart disease refer to the following 4 entities that are the focus of this article [2] :
- Coronary artery disease (CAD): Sometimes referred to as Coronary Heart Disease (CHD), results from decreased myocardial perfusion that causes angina, myocardial infarction (MI), and/or heart failure. It accounts for one-third to one-half of the cases of CVD.
- Cerebrovascular disease (CVD): Including stroke and transient ischemic attack (TIA)
- Peripheral artery disease (PAD): Particularly arterial disease involving the limbs that may result in claudication
- Aortic atherosclerosis: Including thoracic and abdominal aneurysms
Although CVD may directly arise from different etiologies such as emboli in a patient with atrial fibrillation resulting in ischemic stroke, rheumatic fever causing valvular heart disease, among others, addressing risks factors associated to the development of atherosclerosis is most important because it is a common denominator in the pathophysiology of CVD.
The industrialization of the economy with a resultant shift from physically demanding to sedentary jobs, along with the current consumerism and technology-driven culture that is related to longer work hours, longer commutes, and less leisure time for recreational activities, may explain the significant and steady increase in the rates of CVD during the last few decades. Specifically, physical inactivity, intake of a high-calorie diet, saturated fats, and sugars are associated with the development of atherosclerosis and other metabolic disturbances like metabolic syndrome, diabetes mellitus, and hypertension that are highly prevalent in people with CVD. [3] [2] [4] [5]
According to the INTERHEART study that included subjects from 52 countries, including high, middle, and low-income countries, 9 modifiable risks factors accounted for 90% of the risk of having a first MI: smoking, dyslipidemia, hypertension, diabetes, abdominal obesity, psychosocial factors, consumption of fruits and vegetables, regular alcohol consumption, and physical inactivity. It is important to mention that in this study 36% of the population-attributable risk of MI was accounted to smoking. [6]
Other large cohort studies like the Framingham Heart Study [7] and the Third National Health and Nutrition Examination Survey (NHANES III) [5] have also found a strong association and predictive value of dyslipidemia, high blood pressure, smoking, and glucose intolerance. Sixty percent to 90% of CHD events occurred in subjects with at least one risk factor.
These findings have been translated into health promotion programs by the American Heart Association with emphasis on seven recommendations to decrease the risk of CVD: avoiding smoking, being physically active, eating healthy, and keeping normal blood pressure, body weight, glucose, and cholesterol levels. [8] [9]
On the other hand, non-modifiable factors as family history, age, and gender have different implications. [4] [7] Family history, particularly premature atherosclerotic disease defined as CVD or death from CVD in a first-degree relative before 55 years (in males) or 65 years (in females) is considered an independent risk factor. [10] There is also suggestive evidence that the presence of CVD risk factors may differently influence gender. [4] [7] For instance, diabetes and smoking more than 20 cigarettes per day had increased CVD risk in women compared to men. [11] Prevalence of CVD increases significantly with each decade of life. [12]
The presence of HIV (human immunodeficiency virus), [13] history of mediastinal or chest wall radiation, [14] microalbuminuria, [15] , increased inflammatory markers [16] [17] have also been associated with an increased rate and incidence of CVD.
Pointing out specific diet factors like meat consumption, fiber, and coffee and their relation to CVD remains controversial due to significant bias and residual confounding encountered in epidemiological studies. [18] [19]
- Epidemiology
Cardiovascular diseases (CVD) remain among the 2 leading causes of death in the United States since 1975 with 633,842 deaths or 1 in every 4 deaths, heart disease occupied the leading cause of death in 2015 followed by 595,930 deaths related to cancer. [2] CVD is also the number 1 cause of death globally with an estimated 17.7 million deaths in 2015, according to the World Health Organization (WHO). The burden of CVD further extends as it is considered the most costly disease even ahead of Alzheimer disease and diabetes with calculated indirect costs of $237 billion dollars per year and a projected increased to $368 billion by 2035. [20]
Although the age-adjusted rate and acute mortality from MI have been declining over time, reflecting the progress in diagnosis and treatment during the last couple of decades, the risk of heart disease remains high with a calculated 50% risk by age 45 in the general population. [7] [21] The incidence significantly increases with age with some variations between genders as the incidence is higher in men at younger ages. [2] The difference in incidence narrows progressively in the post-menopausal state. [2]
- Pathophysiology
Atherosclerosis is the pathogenic process in the arteries and the aorta that can potentially cause disease as a consequence of decreased or absent blood flow from stenosis of the blood vessels. [22]
It involves multiple factors dyslipidemia, immunologic phenomena, inflammation, and endothelial dysfunction. These factors are believed to trigger the formation of fatty streak, which is the hallmark in the development of the atherosclerotic plaque [23] ; a progressive process that may occur as early as in the childhood. [24] This process comprises intimal thickening with subsequent accumulation of lipid-laden macrophages (foam cells) and extracellular matrix, followed by aggregation and proliferation of smooth muscle cells constituting the formation of the atheroma plaque. [25] As this lesions continue to expand, apoptosis of the deep layers can occur, precipitating further macrophage recruitment that can become calcified and transition to atherosclerotic plaques. [26]
Other mechanisms like arterial remodeling and intra-plaque hemorrhage play an important role in the delay and accelerated the progression of atherosclerotic CVD but are beyond the purpose of this article. [27]
- History and Physical
The clinical presentation of cardiovascular diseases can range from asymptomatic (e.g., silent ischemia, angiographic evidence of coronary artery disease without symptoms, among others) to classic presentations as when patients present with typical anginal chest pain consistent of myocardial infarction and/or those suffering from acute CVA presenting with focal neurological deficits of sudden onset. [28] [29] [28]
Historically, coronary artery disease typically presents with angina that is a pain of substernal location, described as a crushing or pressure in nature, that may radiate to the medial aspect of the left upper extremity, to the neck or the jaw and that can be associated with nausea, vomiting, palpitations, diaphoresis, syncope or even sudden death. [30] Physicians and other health care providers should be aware of possible variations in symptom presentation for these patients and maintain a high index of suspicion despite an atypical presentation, for example, dizziness and nausea as the only presenting symptoms in patients having an acute MI [31] ), particularly in people with a known history of CAD/MI and for those with the presence of CVD risk factors. [32] [33] [34] [33] [32] Additional chest pain features suggestive of ischemic etiology are the exacerbation with exercise and or activity and resolution with rest or nitroglycerin. [35]
Neurologic deficits are the hallmark of cerebrovascular disease including TIA and stroke where the key differentiating factor is the resolution of symptoms within 24 hours for patients with TIA. [36] Although the specific symptoms depend on the affected area of the brain, the sudden onset of extremity weakness, dysarthria, and facial droop are among the most commonly reported symptoms that raise concern for a diagnosis of a stroke. [37] [38] Ataxia, nystagmus and other subtle symptoms as dizziness, headache, syncope, nausea or vomiting are among the most reported symptoms with people with posterior circulation strokes challenging to correlate and that require highly suspicion in patients with risks factors. [39]
Patients with PAD may present with claudication of the limbs, described as a cramp-like muscle pain precipitated by increased blood flow demand during exercise that typically subsides with rest. [40] Severe PAD might present with color changes of the skin and changes in temperature. [41]
Most patients with thoracic aortic aneurysm will be asymptomatic, but symptoms can develop as it progresses from subtle symptoms from compression to surrounding tissues causing cough, shortness of breath or dysphonia, to the acute presentation of sudden crushing chest or back pain due to acute rupture. [42] The same is true for abdominal aortic aneurysms (AAA) that cause no symptoms in early stages to the acute presentation of sudden onset of abdominal pain or syncope from acute rupture. [43]
A thorough physical examination is paramount for the diagnosis of CVD. Starting with a general inspection to look for signs of distress as in patients with angina or with decompensated heart failure, or chronic skin changes from PAD. Carotid examination with the patient on supine position and the back at 30 degrees for the palpation and auscultation of carotid pulses, bruits and to evaluate for jugular venous pulsations on the neck is essential. Precordial examination starting with inspection, followed by palpation looking for chest wall tenderness, thrills, and identification of the point of maximal impulse should then be performed before auscultating the precordium. Heart sounds auscultation starts in the aortic area with the identification of the S1 and S2 sounds followed by characterization of murmurs if present. Paying attention to changes with inspirations and maneuvers to correctly characterize heart murmurs is encouraged. Palpating peripheral pulses with bilateral examination and comparison when applicable is an integral part of the CVD examination. [44]
Thorough clinical history and physical exam directed but not limited to the cardiovascular system are the hallmarks for the diagnosis of CVD. Specifically, a history compatible with obesity, angina, decreased exercise tolerance, orthopnea, paroxysmal nocturnal dyspnea, syncope or presyncope, and claudication should prompt the clinician to obtain a more detailed history and physical exam and, if pertinent, obtain ancillary diagnostic test according to the clinical scenario (e.g., electrocardiogram and cardiac enzymes for patients presenting with chest pain).
Besides a diagnosis prompted by clinical suspicion, most of the efforts should be oriented for primary prevention by targeting people with the presence of risk factors and treat modifiable risk factors by all available means. All patient starting at age 20 should be engaged in the discussion of CVD risk factors and lipid measurement. [9] Several calculators that use LDL-cholesterol and HDL-cholesterol levels and the presence of other risk factors calculate a 10-year or 30-year CVD score to determine if additional therapies like the use of statins and aspirin are indicated for primary prevention, generally indicated if such risk is more than ten percent. [10] Like other risk assessment tools, the use of this calculators have some limitations, and it is recommended to exert precaution when assessing patients with diabetes and familial hypercholesterolemia as their risk can be underestimated. Another limitation to their use is that people older than 79 were usually excluded from the cohorts where these calculators were formulated, and individualized approach for these populations is recommended by discussing risk and benefits of adjunctive therapies and particular consideration of life expectancy. Some experts recommend a reassessment of CVD risk every 4 to 6 years. [9]
Preventative measures like following healthy food habits, avoiding overweight and following an active lifestyle are pertinent in all patients, particularly for people with non-modifiable risk factors such as family history of premature CHD or post-menopause. [9] [8]
The use of inflammatory markers and other risk assessment methods as coronary artery calcification score (CAC) are under research and have limited applications that their use should not replace the identification of people with known risk factors, nonetheless these resources remain as promising tools in the future of primary prevention by detecting people with subclinical atherosclerosis at risk for CVD. [45]
- Treatment / Management
Management of CVD is very extensive depending on the clinical situation (catheter-directed thrombolysis for acute ischemic stroke, angioplasty for peripheral vascular disease, coronary stenting for CHD); however, patients with known CVD should be strongly educated on the need for secondary prevention by risk factor and lifestyle modification. [9] [46]
- Differential Diagnosis
- Acute pericarditis
- Angina pectoris
- Artherosclerosis
- Coronary artery vasospasm
- Dilated cardiomyopathy
- Giant cell arteritis
- Hypertension
- Hypertensive heart disease
- Kawasaki disease
- Myocarditis
The prognosis and burden of CVD have been discussed in other sections.
- Complications
The most feared complication from CVD is death and, as explained above, despite multiple discoveries in the last decades CVD remains in the top leading causes of death all over the world owing to the alarming prevalence of CVD in the population. [2] Other complications as the need for longer hospitalizations, physical disability and increased costs of care are significant and are the focus for health-care policymakers as it is believed they will continue to increase in the coming decades. [20]
For people with heart failure with reduced ejection fraction (HFreEF) of less than 35%, as the risk of life-threatening arrhythmias is exceedingly high in these patients, current guidelines recommend the implantation of an implantable-cardioverter defibrillator (ICD) for those with symptoms equivalent to a New York Heart Association (NYHA) Class II-IV despite maximal tolerated medical therapy. [47]
Strokes can leave people with severe disabling sequelae like dysarthria or aphasia, dysphagia, focal or generalized muscle weakness or paresis that can be temporal or cause permanent physical disability that may lead to a complete bedbound state due to hemiplegia with added complications secondary to immobility as is the higher risk of developing urinary tract infections and/or risk for thromboembolic events. [48] [49]
There is an increased risk of all-cause death for people with PAD compared to those without evidence of peripheral disease. [50] Chronic wounds, physical limitation, and limb ischemia are among other complications from PAD. [51]
- Consultations
An interprofessional approach that involves primary care doctors, nurses, dietitians, cardiologists, neurologists, and other specialists is likely to improve outcomes. This has been shown to be beneficial in patients with heart failure, [52] coronary disease, [53] and current investigations to assess the impact on other forms of CVD are under planning and promise encouraging results.
- Deterrence and Patient Education
Efforts should be directed toward primary prevention by leading a healthy lifestyle, and an appropriate diet starting as early as possible with the goal of delay or avoid the initiation of atherosclerosis as it relates to the future risk of CVD. The AHA developed the concept of "ideal cardiovascular health" defined by the presence of [8] :
- Ideal health behaviors: Nonsmoking, body mass index less than 25 kg/m2, physical activity at goal levels, and the pursuit of a diet consistent with current guideline recommendations
- Ideal health factors: Untreated total cholesterol less than 200 mg/dL, untreated blood pressure less than 120/80 mm Hg, and fasting blood glucose less than 100 mg/dL) with the goal to improve the health of all Americans with an expected decrease in deaths from CVD by 20%
Specific attention should be made to people at higher risk for CVD as are people with diabetes, hypertension, hyperlipidemia, smokers, and obese patients. Risk factors modification by controlling their medical conditions, avoiding smoking, taking appropriate measures to lose weight and maintaining an active lifestyle is of extreme importance. [8] [9] [10] The recommendations on the use of statins and low-dose aspirin for primary and secondary prevention has been discussed in other sections.
- Pearls and Other Issues
Cardiovascular disease generally refers to 4 general entities: CAD, CVD, PVD, and aortic atherosclerosis.
CVD is the main cause of death globally.
Measures aimed to prevent the progression of atherosclerosis are the hallmark for primary prevention of CVD.
Risk factor and lifestyle modification are paramount in the prevention of CVD.
- Enhancing Healthcare Team Outcomes
An interprofessional and patient-oriented approach can help to improve outcomes for people with cardiovascular disease as shown in patients with heart failure (HF) who had better outcomes when the interprofessional involvement of nurses, dietitians, pharmacists, and other health professionals was used (Class 1A). [52]
Similarly, positive results were obtained in people in an intervention group who were followed by an interprofessional team comprised of pharmacists, nurses and a team of different physicians. This group had a reduction in all-cause mortality associated with CAD by 76% compared to the control group. [53] Healthcare workers should educate the public on lifestyle changes and reduce the modifiable risk factors for heart disease to a minimum.
- Review Questions
- Access free multiple choice questions on this topic.
- Comment on this article.
Atherosclerosis as a result of coronary heart disease. Contributed by National Heart, Lung and Blood Institute (NIH)
Coronary Artery Disease Pathophysiology. Coronary artery disease is usually caused by an atherosclerotic plaque that blocks the lumen of a coronary artery, typically the left anterior descending artery. Contributed by S Bhimji, MD
Disclosure: Edgardo Olvera Lopez declares no relevant financial relationships with ineligible companies.
Disclosure: Brian Ballard declares no relevant financial relationships with ineligible companies.
Disclosure: Arif Jan declares no relevant financial relationships with ineligible companies.
This book is distributed under the terms of the Creative Commons Attribution-NonCommercial-NoDerivatives 4.0 International (CC BY-NC-ND 4.0) ( http://creativecommons.org/licenses/by-nc-nd/4.0/ ), which permits others to distribute the work, provided that the article is not altered or used commercially. You are not required to obtain permission to distribute this article, provided that you credit the author and journal.
- Cite this Page Olvera Lopez E, Ballard BD, Jan A. Cardiovascular Disease. [Updated 2023 Aug 22]. In: StatPearls [Internet]. Treasure Island (FL): StatPearls Publishing; 2024 Jan-.
In this Page
Bulk download.
- Bulk download StatPearls data from FTP
Related information
- PMC PubMed Central citations
- PubMed Links to PubMed
Similar articles in PubMed
- Review De-risking primary prevention: role of imaging. [Ther Adv Cardiovasc Dis. 2021] Review De-risking primary prevention: role of imaging. Shafter AM, Shaikh K, Johanis A, Budoff MJ. Ther Adv Cardiovasc Dis. 2021 Jan-Dec; 15:17539447211051248.
- Shared and non-shared familial susceptibility of coronary heart disease, ischemic stroke, peripheral artery disease and aortic disease. [Int J Cardiol. 2013] Shared and non-shared familial susceptibility of coronary heart disease, ischemic stroke, peripheral artery disease and aortic disease. Calling S, Ji J, Sundquist J, Sundquist K, Zöller B. Int J Cardiol. 2013 Oct 3; 168(3):2844-50. Epub 2013 Apr 30.
- Stenting for peripheral artery disease of the lower extremities: an evidence-based analysis. [Ont Health Technol Assess Ser....] Stenting for peripheral artery disease of the lower extremities: an evidence-based analysis. Medical Advisory Secretariat. Ont Health Technol Assess Ser. 2010; 10(18):1-88. Epub 2010 Sep 1.
- Polyvascular disease and long-term cardiovascular outcomes in older patients with non-ST-segment-elevation myocardial infarction. [Circ Cardiovasc Qual Outcomes....] Polyvascular disease and long-term cardiovascular outcomes in older patients with non-ST-segment-elevation myocardial infarction. Subherwal S, Bhatt DL, Li S, Wang TY, Thomas L, Alexander KP, Patel MR, Ohman EM, Gibler WB, Peterson ED, et al. Circ Cardiovasc Qual Outcomes. 2012 Jul 1; 5(4):541-9. Epub 2012 Jun 19.
- Review Prevention of ventricular fibrillation, acute myocardial infarction (myocardial necrosis), heart failure, and mortality by bretylium: is ischemic heart disease primarily adrenergic cardiovascular disease? [Am J Ther. 2004] Review Prevention of ventricular fibrillation, acute myocardial infarction (myocardial necrosis), heart failure, and mortality by bretylium: is ischemic heart disease primarily adrenergic cardiovascular disease? Bacaner M, Brietenbucher J, LaBree J. Am J Ther. 2004 Sep-Oct; 11(5):366-411.
Recent Activity
- Cardiovascular Disease - StatPearls Cardiovascular Disease - StatPearls
Your browsing activity is empty.
Activity recording is turned off.
Turn recording back on
Connect with NLM
National Library of Medicine 8600 Rockville Pike Bethesda, MD 20894
Web Policies FOIA HHS Vulnerability Disclosure
Help Accessibility Careers
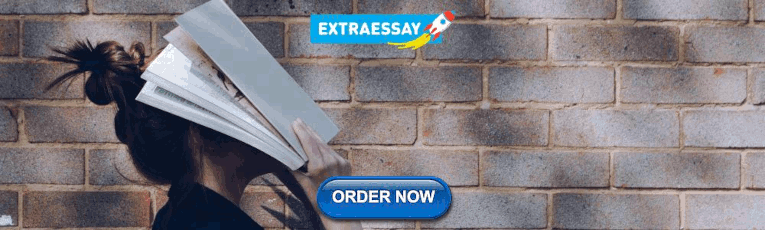
IMAGES
COMMENTS
The coronary heart disease studies were not restricted during the search by sample size because of the limited number of studies in selected countries. The study reviews the potential risk factors responsible for coronary heart disease globally. ... The selected language was English for all the research articles, and each gender was given ...
A healthy diet is one of the American Heart Association (AHA)'s "Life's Essential 8" cardiovascular health metrics, which also include favorable levels of physical activity, sleep, nicotine exposure, BMI, blood lipids, blood glucose, and blood pressure. 19 However, <1% of US adults met "ideal" criteria for the AHA's healthy diet ...
1. Introduction. Cardiovascular (CV) disease (CVD) is still a major cause of morbidity and mortality in many countries in Europe although considerable efforts have been made in recent decades to address this disease in an even more "comprehensive" approach [].The so-called "traditional" CV risk factors are arterial hypertension, dyslipidemia, diabetes, cigarette smoking, family history ...
The American Heart Association, in conjunction with the National Institutes of Health, annually reports the most up-to-date statistics related to heart disease, stroke, and cardiovascular risk factors, including core health behaviors (smoking, physical activity, diet, and weight) and health factors (cholesterol, blood pressure, and glucose control) that contribute to cardiovascular health.
Beginning with ischemic heart disease (IHD) and stroke, this article provides information on the burden of CVD, ... GBD 2019 is a multinational collaborative research study that estimates disease burden for every country in the world (1,4). The study is an ongoing effort, updated annually, and is designed to allow for consistent comparison over ...
Congenital Heart Disease 10; Mechanical Circulatory Support 7; Venous Disorders 3; By Article Category. Research 3320; Commentary 1376; Other 1308; Clinical Cases 1265; Review 1217; Media 443 ...
Introduction. Cardiovascular diseases (CVDs) have collectively remained the leading causes of death worldwide and substantially contribute to loss of health and excess health system costs. 1, 2 The Global Burden of Diseases, Injuries, and Risk Factors (GBD) Study has tracked trends in death and disability since 1990 and has provided an updated perspective on the status of cardiovascular health ...
The American Heart Association (AHA), in conjunction with the National Institutes of Health, annually reports the most up-to-date statistics related to heart disease, stroke, and cardiovascular risk factors, including core health behaviors (smoking, physical activity, nutrition, sleep, and obesity) and health factors (cholesterol, blood pressure, glucose control, and metabolic syndrome) that ...
Background: The American Heart Association, in conjunction with the National Institutes of Health, annually reports the most up-to-date statistics related to heart disease, stroke, and cardiovascular risk factors, including core health behaviors (smoking, physical activity, diet, and weight) and health factors (cholesterol, blood pressure, and glucose control) that contribute to cardiovascular ...
Cardiovascular risk factors are known to increase cardiovascular disease risk differently across various geographic regions. 2,18 Among them, high blood pressure is associated with up to 13.5% of ...
New global data analysis highlights the urgency of translating research into practice. Over the last 30 years, deaths and disability from cardiovascular disease have been steadily rising across the globe. In 2019 alone, the condition, which includes heart disease and stroke, was responsible for a staggering one-third of all deaths worldwide.
Research is revealing the causes of heart disease and what can be done to tackle the world's biggest killer. ... During the time it takes to read this brief article, ischaemic heart disease, in ...
The importance of the brain-heart interaction has been increasingly recognized as a critical physiological axis that is altered in disease. In this review, we explore the intricate relationship between the central nervous system and cardiovascular health, focusing particularly on immunological mechanisms that influence the course of both neurological and cardiovascular diseases.
As the leading funder of heart- and stroke-related research outside the U.S. government, the American Heart Association compiles an annual overview of significant scientific advances in the fight against cardiovascular disease, which claims more than 850,000 lives in the U.S. each year and is the leading cause of death and disability worldwide.
At the end of August, the European Society of Cardiology is holding its annual Congress, spotlighting innovative cardiovascular research. Cardiovascular diseases are the leading cause of death worldwide, representing 32% of all deaths in 2021. Cardiovascular care is unacceptably unequal; more than three-quarters of these deaths take place in low-income and middle-income countries (LMICs), and ...
Heart disease is a fatal human disease, rapidly increases globally in both developed and undeveloped countries and consequently, causes death. ... The research study recommends that which feature ...
The projected rise in heart disease and stroke - along with several key risk factors, including high blood pressure and obesity - is likely to triple related costs to $1.8 trillion by 2050, according to two American Heart Association presidential advisories published Tuesday in the AHA journal Circulation.
Read current medical research on risk factors, causes and prevention of heart disease, strokes, and peripheral arterial disease. What new treatment options are under development?
Heart disease, including coronary heart disease, remains the leading cause of death in the United States. However, the rate of heart disease deaths has declined by 70% over the past 50 years, thanks in part to NHLBI-funded research. Many current studies funded by the NHLBI focus on discovering genetic associations and finding new ways to ...
More Than $5.7 billion Invested. Since 1949, the American Heart Association has invested more than $5.7 billion toward scientific research, more than any other non-profit organization outside of the federal government. If you are a researcher, the AHA has resources available for you. Learn more about our varied initiatives, and how you can play ...
Recent research has harnessed advanced multi-system 18F-fluorodeoxyglucose positron emission tomography/computed ... Britton A, Brunner EJ, et al. Increased risk of coronary heart disease among individuals reporting adverse impact of stress on their health: the Whitehall II prospective cohort study. Eur Heart J. 2013; 34 (34):2697-705. 10. ...
There are several kinds of heart disease, including: cardiomyopathy: a heart muscle disease that causes the heart to become abnormally enlarged, thickened, and/or stiffened. congenital heart disease: heart disease or abnormalities in the heart's structure that are present at birth. coronary artery disease: accumulation of cholesterol-filled ...
Background—Cardiovascular disease (CVD) is the leading cause of death in the United States and is responsible for 17% of national health expenditures. As the population ages, these costs are expected to increase substantially. Methods and Results—To prepare for future cardiovascular care needs, the American Heart Association developed methodology to project future costs of care for ...
How improving sleep can mean better cardiac health 04:10. Busy week have you catching up on sleep during the weekend? That may be good for your heart health, according to new research.. In a study ...
New research suggests that sleeping in on weekends may help you catch up on lost sleep and lower your heart disease risk by up to 20%. The effects of "compensatory sleep" were more pronounced in ...
The Coronary Heart Disease Damp Phlegm and Blood Stasis Pattern Questionnaire (CHD-DPBSPQ) is the standard metric for measuring CHD-DPBSP signs and symptoms in practice and clinical research [11 - 13]. The CHD-DPBSPQ was published in 2019 by combining an assessment of the damp phlegm pattern and blood stasis pattern in CHD Patients .
Living in a tree-filled neighborhood may be as beneficial to the heart as regular exercise, new research shows. Researchers at the University of Louisville designed a clinical trial that followed ...
Heart disease and stroke could affect at least 60% of adults in U.S. by 2050 . Jun 4, 2024. An aging, more diverse population with rising cardiovascular risk factors - especially obesity and high blood pressure - will drive big increases in heart disease and stroke over the next 30 years, according to new projections.
The cardiovascular system consists of the heart and blood vessels.[1] There is a wide array of problems that may arise within the cardiovascular system, for example, endocarditis, rheumatic heart disease, abnormalities in the conduction system, among others, cardiovascular disease (CVD) or heart disease refer to the following 4 entities that are the focus of this article[2]:
As recently highlighted by the American Heart Association statement on Cardiovascular-Kidney-Metabolic syndrome, people with chronic kidney disease (CKD) are at high risk of cardiovascular disease (CVD).1 Our understanding of the multiplicative effects of CKD and CVD on mortality has led to a deeper appreciation of the urgency of studying these frequently concomitant conditions, particularly ...