- - Google Chrome
Intended for healthcare professionals
- My email alerts
- BMA member login
- Username * Password * Forgot your log in details? Need to activate BMA Member Log In Log in via OpenAthens Log in via your institution

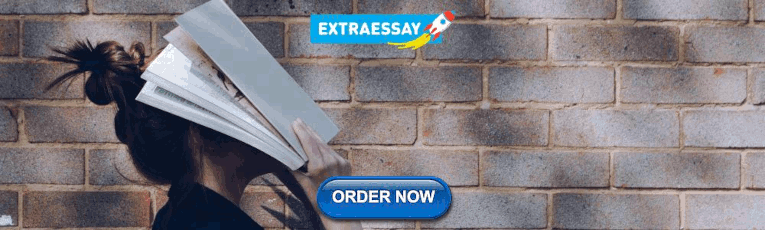
Search form
- Advanced search
- Search responses
- Search blogs
- Three techniques for...
Three techniques for integrating data in mixed methods studies
- Related content
- Peer review
- Alicia O’Cathain , professor 1 ,
- Elizabeth Murphy , professor 2 ,
- Jon Nicholl , professor 1
- 1 Medical Care Research Unit, School of Health and Related Research, University of Sheffield, Sheffield S1 4DA, UK
- 2 University of Leicester, Leicester, UK
- Correspondence to: A O’Cathain a.ocathain{at}sheffield.ac.uk
- Accepted 8 June 2010
Techniques designed to combine the results of qualitative and quantitative studies can provide researchers with more knowledge than separate analysis
Health researchers are increasingly using designs that combine qualitative and quantitative methods, and this is often called mixed methods research. 1 Integration—the interaction or conversation between the qualitative and quantitative components of a study—is an important aspect of mixed methods research, and, indeed, is essential to some definitions. 2 Recent empirical studies of mixed methods research in health show, however, a lack of integration between components, 3 4 which limits the amount of knowledge that these types of studies generate. Without integration, the knowledge yield is equivalent to that from a qualitative study and a quantitative study undertaken independently, rather than achieving a “whole greater than the sum of the parts.” 5
Barriers to integration have been identified in both health and social research. 6 7 One barrier is the absence of formal education in mixed methods research. Fortunately, literature is rapidly expanding to fill this educational gap, including descriptions of how to integrate data and findings from qualitative and quantitative methods. 8 9 In this article we outline three techniques that may help health researchers to integrate data or findings in their mixed methods studies and show how these might enhance knowledge generated from this approach.
Triangulation protocol
Researchers will often use qualitative and quantitative methods to examine different aspects of an overall research question. For example, they might use a randomised controlled trial to assess the effectiveness of a healthcare intervention and semistructured interviews with patients and health professionals to consider the way in which the intervention was used in the real world. Alternatively, they might use a survey of service users to measure satisfaction with a service and focus groups to explore views of care in more depth. Data are collected and analysed separately for each component to produce two sets of findings. Researchers will then attempt to combine these findings, sometimes calling this process triangulation. The term triangulation can be confusing because it has two meanings. 10 It can be used to describe corroboration between two sets of findings or to describe a process of studying a problem using different methods to gain a more complete picture. The latter meaning is commonly used in mixed methods research and is the meaning used here.
The process of triangulating findings from different methods takes place at the interpretation stage of a study when both data sets have been analysed separately (figure ⇓ ). Several techniques have been described for triangulating findings. They require researchers to list the findings from each component of a study on the same page and consider where findings from each method agree (convergence), offer complementary information on the same issue (complementarity), or appear to contradict each other (discrepancy or dissonance). 11 12 13 Explicitly looking for disagreements between findings from different methods is an important part of this process. Disagreement is not a sign that something is wrong with a study. Exploration of any apparent “inter-method discrepancy” may lead to a better understanding of the research question, 14 and a range of approaches have been used within health services research to explore inter-method discrepancy. 15
Point of application for three techniques for integrating data in mixed methods research
- Download figure
- Open in new tab
- Download powerpoint
The most detailed description of how to carry out triangulation is the triangulation protocol, 11 which although developed for multiple qualitative methods, is relevant to mixed methods studies. This technique involves producing a “convergence coding matrix” to display findings emerging from each component of a study on the same page. This is followed by consideration of where there is agreement, partial agreement, silence, or dissonance between findings from different components. This technique for triangulation is the only one to include silence—where a theme or finding arises from one data set and not another. Silence might be expected because of the strengths of different methods to examine different aspects of a phenomenon, but surprise silences might also arise that help to increase understanding or lead to further investigations.
The triangulation protocol moves researchers from thinking about the findings related to each method, to what Farmer and colleagues call meta-themes that cut across the findings from different methods. 11 They show a worked example of triangulation protocol, but we could find no other published example. However, similar principles were used in an iterative mixed methods study to understand patient and carer satisfaction with a new primary angioplasty service. 16 Researchers conducted semistructured interviews with 16 users and carers to explore their experiences and views of the new service. These were used to develop a questionnaire for a survey of 595 patients (and 418 of their carers) receiving either the new service or usual care. Finally, 17 of the patients who expressed dissatisfaction with aftercare and rehabilitation were followed up to explore this further in semistructured interviews. A shift of thinking to meta-themes led the researchers away from reporting the findings from the interviews, survey, and follow-up interviews sequentially to consider the meta-themes of speed and efficiency, convenience of care, and discharge and after care. The survey identified that a higher percentage of carers of patients using the new service rated the convenience of visiting the hospital as poor than those using usual care. The interviews supported this concern about the new service, but also identified that the weight carers gave to this concern was low in the context of their family member’s life being saved.
Morgan describes this move as the “third effort” because it occurs after analysis of the qualitative and the quantitative components. 17 It requires time and energy that must be planned into the study timetable. It is also useful to consider who will carry out the integration process. Farmer and colleagues require two researchers to work together during triangulation, which can be particularly important in mixed methods studies if different researchers take responsibility for the qualitative and quantitative components. 11
Following a thread
Moran-Ellis and colleagues describe a different technique for integrating the findings from the qualitative and quantitative components of a study, called following a thread. 18 They state that this takes place at the analysis stage of the research process (figure ⇑ ). It begins with an initial analysis of each component to identify key themes and questions requiring further exploration. Then the researchers select a question or theme from one component and follow it across the other components—they call this the thread. The authors do not specify steps in this technique but offer a visual model for working between datasets. An approach similar to this has been undertaken in health services research, although the researchers did not label it as such, probably because the technique has not been used frequently in the literature (box)
An example of following a thread 19
Adamson and colleagues explored the effect of patient views on the appropriate use of services and help seeking using a survey of people registered at a general practice and semistructured interviews. The qualitative (22 interviews) and quantitative components (survey with 911 respondents) took place concurrently.
The researchers describe what they call an iterative or cyclical approach to analysis. Firstly, the preliminary findings from the interviews generated a hypothesis for testing in the survey data. A key theme from the interviews concerned the self rationing of services as a responsible way of using scarce health care. This theme was then explored in the survey data by testing the hypothesis that people’s views of the appropriate use of services would explain their help seeking behaviour. However, there was no support for this hypothesis in the quantitative analysis because the half of survey respondents who felt that health services were used inappropriately were as likely to report help seeking for a series of symptoms presented in standardised vignettes as were respondents who thought that services were not used inappropriately. The researchers then followed the thread back to the interview data to help interpret this finding.
After further analysis of the interview data the researchers understood that people considered the help seeking of other people to be inappropriate, rather than their own. They also noted that feeling anxious about symptoms was considered to be a good justification for seeking care. The researchers followed this thread back into the survey data and tested whether anxiety levels about the symptoms in the standardised vignettes predicted help seeking behaviour. This second hypothesis was supported by the survey data. Following a thread led the researchers to conclude that patients who seek health care for seemingly minor problems have exceeded their thresholds for the trade-off between not using services inappropriately and any anxiety caused by their symptoms.
Mixed methods matrix
A unique aspect of some mixed methods studies is the availability of both qualitative and quantitative data on the same cases. Data from the qualitative and quantitative components can be integrated at the analysis stage of a mixed methods study (figure ⇑ ). For example, in-depth interviews might be carried out with a sample of survey respondents, creating a subset of cases for which there is both a completed questionnaire and a transcript. Cases may be individuals, groups, organisations, or geographical areas. 9 All the data collected on a single case can be studied together, focusing attention on cases, rather than variables or themes, within a study. The data can be examined in detail for each case—for example, comparing people’s responses to a questionnaire with their interview transcript. Alternatively, data on each case can be summarised and displayed in a matrix 8 9 20 along the lines of Miles and Huberman’s meta-matrix. 21 Within a mixed methods matrix, the rows represent the cases for which there is both qualitative and quantitative data, and the columns display different data collected on each case. This allows researchers to pay attention to surprises and paradoxes between types of data on a single case and then look for patterns across all cases 20 in a qualitative cross case analysis. 21
We used a mixed methods matrix to study the relation between types of team working and the extent of integration in mixed methods studies in health services research (table ⇓ ). 22 Quantitative data were extracted from the proposals, reports, and peer reviewed publications of 75 mixed methods studies, and these were analysed to describe the proportion of studies with integrated outputs such as mixed methods journal articles. Two key variables in the quantitative component were whether the study was assessed as attempting to integrate qualitative or quantitative data or findings and the type of publications produced. We conducted qualitative interviews with 20 researchers who had worked on some of these studies to explore how mixed methods research was practised, including how the team worked together.
Example of a mixed methods matrix for a study exploring the relationship between types of teams and integration between qualitative and quantitative components of studies* 22
- View inline
The shared cases between the qualitative and quantitative components were 21 mixed methods studies (because one interviewee had worked on two studies in the quantitative component). A matrix was formed with each of the 21 studies as a row. The first column of the matrix contained the study identification, the second column indicated whether integration had occurred in that project, and the third column the score for integration of publications emerging from the study. The rows were then ordered to show the most integrated cases first. This ordering of rows helped us to see patterns across rows.
The next columns were themes from the qualitative interview with a researcher from that project. For example, the first theme was about the expertise in qualitative research within the team and whether the interviewee reported this as adequate for the study. The matrix was then used in the context of the qualitative analysis to explore the issues that affected integration. In particular, it helped to identify negative cases (when someone in the analysis doesn’t fit with the conclusions the analysis is coming to) within the qualitative analysis to facilitate understanding. Interviewees reported the need for experienced qualitative researchers on mixed methods studies to ensure that the qualitative component was published, yet two cases showed that this was neither necessary nor sufficient. This pushed us to explore other factors in a research team that helped generate outputs, and integrated outputs, from a mixed methods study.
Themes from a qualitative study can be summarised to the point where they are coded into quantitative data. In the matrix (table ⇑ ), the interviewee’s perception of the adequacy of qualitative expertise on the team could have been coded as adequate=1 or not=2. This is called “quantitising” of qualitative data 23 ; coded data can then be analysed with data from the quantitative component. This technique has been used to great effect in healthcare research to identify the discrepancy between health improvement assessed using quantitative measures and with in-depth interviews in a randomised controlled trial. 24
We have presented three techniques for integration in mixed methods research in the hope that they will inspire researchers to explore what can be learnt from bringing together data from the qualitative and quantitative components of their studies. Using these techniques may give the process of integration credibility rather than leaving researchers feeling that they have “made things up.” It may also encourage researchers to describe their approaches to integration, allowing them to be transparent and helping them to develop, critique, and improve on these techniques. Most importantly, we believe it may help researchers to generate further understanding from their research.
We have presented integration as unproblematic, but it is not. It may be easier for single researchers to use these techniques than a large research team. Large teams will need to pay attention to team dynamics, considering who will take responsibility for integration and who will be taking part in the process. In addition, we have taken a technical stance here rather than paying attention to different philosophical beliefs that may shape approaches to integration. We consider that these techniques would work in the context of a pragmatic or subtle realist stance adopted by some mixed methods researchers. 25 Finally, it is important to remember that these techniques are aids to integration and are helpful only when applied with expertise.
Summary points
Health researchers are increasingly using designs which combine qualitative and quantitative methods
However, there is often lack of integration between methods
Three techniques are described that can help researchers to integrate data from different components of a study: triangulation protocol, following a thread, and the mixed methods matrix
Use of these methods will allow researchers to learn more from the information they have collected
Cite this as: BMJ 2010;341:c4587
Funding: Medical Research Council grant reference G106/1116
Competing interests: All authors have completed the unified competing interest form at www.icmje.org/coi_disclosure.pdf (available on request from the corresponding author) and declare financial support for the submitted work from the Medical Research Council; no financial relationships with commercial entities that might have an interest in the submitted work; no spouses, partners, or children with relationships with commercial entities that might have an interest in the submitted work; and no non-financial interests that may be relevant to the submitted work.
Contributors: AOC wrote the paper. JN and EM contributed to drafts and all authors agreed the final version. AOC is guarantor.
Provenance and peer review: Not commissioned; externally peer reviewed.
- ↵ Lingard L, Albert M, Levinson W. Grounded theory, mixed methods and action research. BMJ 2008 ; 337 : a567 . OpenUrl FREE Full Text
- ↵ Creswell JW, Fetters MD, Ivankova NV. Designing a mixed methods study in primary care. Ann Fam Med 2004 ; 2 : 7 -12. OpenUrl Abstract / FREE Full Text
- ↵ Lewin S, Glenton C, Oxman AD. Use of qualitative methods alongside randomised controlled trials of complex healthcare interventions: methodological study. BMJ 2009 ; 339 : b3496 . OpenUrl Abstract / FREE Full Text
- ↵ O’Cathain A, Murphy E, Nicholl J. Integration and publications as indicators of ‘yield’ from mixed methods studies. J Mix Methods Res 2007 ; 1 : 147 -63. OpenUrl CrossRef Web of Science
- ↵ Barbour RS. The case for combining qualitative and quantitative approaches in health services research. J Health Serv Res Policy 1999 ; 4 : 39 -43. OpenUrl PubMed
- ↵ O’Cathain A, Nicholl J, Murphy E. Structural issues affecting mixed methods studies in health research: a qualitative study. BMC Med Res Methodol 2009 ; 9 : 82 . OpenUrl CrossRef PubMed
- ↵ Bryman A. Barriers to integrating quantitative and qualitative research. J Mix Methods Res 2007 ; 1 : 8 -22. OpenUrl CrossRef
- ↵ Creswell JW, Plano-Clark V. Designing and conducting mixed methods research . Sage, 2007 .
- ↵ Bazeley P. Analysing mixed methods data. In: Andrew S, Halcomb EJ, eds. Mixed methods research for nursing and the health sciences . Wiley-Blackwell, 2009 :84-118.
- ↵ Sandelowski M. Triangles and crystals: on the geometry of qualitative research. Res Nurs Health 1995 ; 18 : 569 -74. OpenUrl CrossRef PubMed Web of Science
- ↵ Farmer T, Robinson K, Elliott SJ, Eyles J. Developing and implementing a triangulation protocol for qualitative health research. Qual Health Res 2006 ; 16 : 377 -94. OpenUrl Abstract / FREE Full Text
- ↵ Foster RL. Addressing the epistemologic and practical issues in multimethod research: a procedure for conceptual triangulation. Adv Nurs Sci 1997 ; 20 : 1 -12. OpenUrl PubMed
- ↵ Erzerberger C, Prein G. Triangulation: validity and empirically based hypothesis construction. Qual Quant 1997 ; 31 : 141 -54. OpenUrl CrossRef Web of Science
- ↵ Fielding NG, Fielding JL. Linking data . Sage, 1986 .
- ↵ Moffatt S, White M, Mackintosh J, Howel D. Using quantitative and qualitative data in health services research—what happens when mixed method findings conflict? BMC Health Serv Res 2006 ; 6 : 28 . OpenUrl CrossRef PubMed
- ↵ Sampson FC, O’Cathain A, Goodacre S. Is primary angioplasty an acceptable alternative to thrombolysis? Quantitative and qualitative study of patient and carer satisfaction. Health Expectations (forthcoming).
- ↵ Morgan DL. Practical strategies for combining qualitative and quantitative methods: applications to health research. Qual Health Res 1998 ; 8 : 362 -76. OpenUrl Abstract / FREE Full Text
- ↵ Moran-Ellis J, Alexander VD, Cronin A, Dickinson M, Fielding J, Sleney J, et al. Triangulation and integration: processes, claims and implications. Qualitative Research 2006 ; 6 : 45 -59. OpenUrl Abstract / FREE Full Text
- ↵ Adamson J, Ben-Shlomo Y, Chaturvedi N, Donovan J. Exploring the impact of patient views on ‘appropriate’ use of services and help seeking: a mixed method study. Br J Gen Pract 2009 ; 59 : 496 -502. OpenUrl Web of Science
- ↵ Wendler MC. Triangulation using a meta-matrix. J Adv Nurs 2001 ; 35 : 521 -5. OpenUrl CrossRef PubMed Web of Science
- ↵ Miles M, Huberman A. Qualitative data analysis: an expanded sourcebook . Sage, 1994 .
- ↵ O’Cathain A, Murphy E, Nicholl J. Multidisciplinary, interdisciplinary or dysfunctional? Team working in mixed methods research. Qual Health Res 2008 ; 18 : 1574 -85. OpenUrl Abstract / FREE Full Text
- ↵ Sandelowski M. Combining qualitative and quantitative sampling, data collection, and analysis techniques in mixed-method studies. Res Nurs Health 2000 ; 23 : 246 -55. OpenUrl CrossRef PubMed Web of Science
- ↵ Campbell R, Quilty B, Dieppe P. Discrepancies between patients’ assessments of outcome: qualitative study nested within a randomised controlled trial. BMJ 2003 ; 326 : 252 -3. OpenUrl FREE Full Text
- ↵ Mays N, Pope C. Assessing quality in qualitative research. BMJ 2000 ; 320 : 50 -2. OpenUrl FREE Full Text
- Privacy Policy

Home » Mixed Methods Research – Types & Analysis
Mixed Methods Research – Types & Analysis
Table of Contents

Mixed Methods Research
Mixed methods research is an approach to research that combines both quantitative and qualitative research methods in a single study or research project. It is a methodological approach that involves collecting and analyzing both numerical (quantitative) and narrative (qualitative) data to gain a more comprehensive understanding of a research problem.
Types of Mixed Research

There are different types of mixed methods research designs that researchers can use, depending on the research question, the available data, and the resources available. Here are some common types:
Convergent Parallel Design
This design involves collecting both qualitative and quantitative data simultaneously, analyzing them separately, and then merging the findings to draw conclusions. The qualitative and quantitative data are given equal weight, and the findings are integrated during the interpretation phase.
Sequential Explanatory Design
In this design, the researcher collects and analyzes quantitative data first, and then uses qualitative data to explain or elaborate on the quantitative findings. The researcher may use the qualitative data to clarify unexpected or contradictory results from the quantitative analysis.
Sequential Exploratory Design
This design involves collecting qualitative data first, analyzing it, and then collecting and analyzing quantitative data to confirm or refute the qualitative findings. Qualitative data are used to generate hypotheses that are tested using quantitative data.
Concurrent Triangulation Design
This design involves collecting both qualitative and quantitative data concurrently and then comparing the results to find areas of agreement and disagreement. The findings are integrated during the interpretation phase to provide a more comprehensive understanding of the research question.
Concurrent Nested Design
This design involves collecting one type of data as the primary method and then using the other type of data to elaborate or clarify the primary data. For example, a researcher may use quantitative data as the primary method and qualitative data as a secondary method to provide more context and detail.
Transformative Design
This design involves using mixed methods research to not only understand the research question but also to bring about social change or transformation. The research is conducted in collaboration with stakeholders and aims to generate knowledge that can be used to improve policies, programs, and practices.
Concurrent Embedded Design
Concurrent embedded design is a type of mixed methods research design in which one type of data is embedded within another type of data. This design involves collecting both quantitative and qualitative data simultaneously, with one type of data being the primary method and the other type of data being the secondary method. The secondary method is embedded within the primary method, meaning that it is used to provide additional information or to clarify the primary data.
Data Collection Methods
Here are some common data collection methods used in mixed methods research:
Surveys are a common quantitative data collection method used in mixed methods research. Surveys involve collecting standardized responses to a set of questions from a sample of participants. Surveys can be conducted online, in person, or over the phone.
Interviews are a qualitative data collection method that involves asking open-ended questions to gather in-depth information about a participant’s experiences, perspectives, and opinions. Interviews can be conducted in person, over the phone, or online.
Focus groups
Focus groups are a qualitative data collection method that involves bringing together a small group of participants to discuss a topic or research question. The group is facilitated by a researcher, and the discussion is recorded and analyzed for themes and patterns.
Observations
Observations are a qualitative data collection method that involves systematically watching and recording behavior in a natural setting. Observations can be structured or unstructured and can be used to gather information about behavior, interactions, and context.
Document Analysis
Document analysis is a qualitative data collection method that involves analyzing existing documents, such as reports, policy documents, or media articles. Document analysis can be used to gather information about trends, policy changes, or public attitudes.
Experimentation
Experimentation is a quantitative data collection method that involves manipulating one or more variables and measuring their effects on an outcome. Experiments can be conducted in a laboratory or in a natural setting.
Data Analysis Methods
Mixed methods research involves using both quantitative and qualitative data analysis methods to analyze data collected through different methods. Here are some common data analysis methods used in mixed methods research:
Quantitative Data Analysis
Quantitative data collected through surveys or experiments can be analyzed using statistical methods. Statistical analysis can be used to identify relationships between variables, test hypotheses, and make predictions. Common statistical methods used in quantitative data analysis include regression analysis, t-tests, ANOVA, and correlation analysis.
Qualitative Data Analysis
Qualitative data collected through interviews, focus groups, or observations can be analyzed using a variety of qualitative data analysis methods. These methods include content analysis, thematic analysis, narrative analysis, and grounded theory. Qualitative data analysis involves identifying themes and patterns in the data, interpreting the meaning of the data, and drawing conclusions based on the findings.
Integration of Data
The integration of quantitative and qualitative data involves combining the results from both types of data analysis to gain a more comprehensive understanding of the research question. Integration can involve either a concurrent or sequential approach. Concurrent integration involves analyzing quantitative and qualitative data at the same time, while sequential integration involves analyzing one type of data first and then using the results to inform the analysis of the other type of data.
Triangulation
Triangulation involves using multiple sources or types of data to validate or corroborate findings. This can involve using both quantitative and qualitative data or multiple qualitative methods. Triangulation can enhance the credibility and validity of the research findings.
Mixed Methods Meta-analysis
Mixed methods meta-analysis involves the systematic review and synthesis of findings from multiple studies that use mixed methods designs. This involves combining quantitative and qualitative data from multiple studies to gain a broader understanding of a research question.
How to conduct Mixed Methods Research
Here are some general steps for conducting mixed methods research:
- Identify the research problem: The first step is to clearly define the research problem and determine if mixed methods research is appropriate for addressing it.
- Design the study: The research design should include both qualitative and quantitative data collection and analysis methods. The specific design will depend on the research question and the purpose of the study.
- Collect data : Data collection involves collecting both qualitative and quantitative data through various methods such as surveys, interviews, observations, and document analysis.
- Analyze data: Both qualitative and quantitative data need to be analyzed separately and then integrated. Analysis methods may include coding, statistical analysis, and thematic analysis.
- Interpret results: The results of the analysis should be interpreted, taking into account both the quantitative and qualitative findings. This involves integrating the results and identifying any patterns, themes, or discrepancies.
- Draw conclusions : Based on the interpretation of the results, conclusions should be drawn that address the research question and objectives.
- Report findings: Finally, the findings should be reported in a clear and concise manner, using both quantitative and qualitative data to support the conclusions.
Applications of Mixed Methods Research
Mixed methods research can be applied to a wide range of research fields and topics, including:
- Education : Mixed methods research can be used to evaluate educational programs, assess the effectiveness of teaching methods, and investigate student learning experiences.
- Health and social sciences: Mixed methods research can be used to study health interventions, understand the experiences of patients and their families, and assess the effectiveness of social programs.
- Business and management: Mixed methods research can be used to investigate customer satisfaction, assess the impact of marketing campaigns, and analyze the effectiveness of management strategies.
- Psychology : Mixed methods research can be used to explore the experiences and perspectives of individuals with mental health issues, investigate the impact of psychological interventions, and assess the effectiveness of therapy.
- Sociology : Mixed methods research can be used to study social phenomena, investigate the experiences and perspectives of marginalized groups, and assess the impact of social policies.
- Environmental studies: Mixed methods research can be used to assess the impact of environmental policies, investigate public perceptions of environmental issues, and analyze the effectiveness of conservation strategies.
Examples of Mixed Methods Research
Here are some examples of Mixed-Methods research:
- Evaluating a school-based mental health program: A researcher might use a concurrent embedded design to evaluate a school-based mental health program. The researcher might collect quantitative data through surveys and qualitative data through interviews with students and teachers. The quantitative data might be analyzed using statistical methods, while the qualitative data might be analyzed using thematic analysis. The results of the two types of data analysis could be integrated to provide a comprehensive evaluation of the program’s effectiveness.
- Understanding patient experiences of chronic illness: A researcher might use a sequential explanatory design to investigate patient experiences of chronic illness. The researcher might collect quantitative data through surveys and then use the results of the survey to inform the selection of participants for qualitative interviews. The qualitative data might be analyzed using content analysis to identify common themes in the patients’ experiences.
- Assessing the impact of a new public transportation system : A researcher might use a concurrent triangulation design to assess the impact of a new public transportation system. The researcher might collect quantitative data through surveys and qualitative data through focus groups with community members. The results of the two types of data analysis could be triangulated to provide a more comprehensive understanding of the impact of the new transportation system on the community.
- Exploring teacher perceptions of technology integration in the classroom: A researcher might use a sequential exploratory design to investigate teacher perceptions of technology integration in the classroom. The researcher might collect qualitative data through in-depth interviews with teachers and then use the results of the interviews to develop a survey. The quantitative data might be analyzed using descriptive statistics to identify trends in teacher perceptions.
When to use Mixed Methods Research
Mixed methods research is typically used when a research question cannot be fully answered by using only quantitative or qualitative methods. Here are some common situations where mixed methods research is appropriate:
- When the research question requires a more comprehensive understanding than can be achieved by using only quantitative or qualitative methods.
- When the research question requires both an exploration of individuals’ experiences, perspectives, and attitudes, as well as the measurement of objective outcomes and variables.
- When the research question requires the examination of a phenomenon in its natural setting and context, which can be achieved by collecting rich qualitative data, as well as the generalization of findings to a larger population, which can be achieved through the use of quantitative methods.
- When the research question requires the integration of different types of data or perspectives, such as combining data collected from participants with data collected from stakeholders or experts.
- When the research question requires the validation of findings obtained through one method by using another method.
- When the research question involves studying a complex phenomenon that cannot be understood by using only one method, such as studying the impact of a policy on a community’s well-being.
- When the research question involves studying a topic that has not been well-researched, and using mixed methods can help provide a more comprehensive understanding of the topic.
Purpose of Mixed Methods Research
The purpose of mixed methods research is to provide a more comprehensive understanding of a research problem than can be obtained through either quantitative or qualitative methods alone.
Mixed methods research is particularly useful when the research problem is complex and requires a deep understanding of the context and subjective experiences of participants, as well as the ability to generalize findings to a larger population. By combining both qualitative and quantitative methods, researchers can obtain a more complete picture of the research problem and its underlying mechanisms, as well as test hypotheses and identify patterns that may not be apparent with only one method.
Overall, mixed methods research aims to provide a more holistic and nuanced understanding of the research problem, allowing researchers to draw more valid and reliable conclusions, make more informed decisions, and develop more effective interventions and policies.
Advantages of Mixed Methods Research
Mixed methods research offers several advantages over using only qualitative or quantitative research methods. Here are some of the main advantages of mixed methods research:
- Comprehensive understanding: Mixed methods research provides a more comprehensive understanding of the research problem by combining both qualitative and quantitative data, which allows for a more nuanced interpretation of the data.
- Triangulation : Mixed methods research allows for triangulation, which is the use of multiple sources of data to verify findings. This improves the validity and reliability of the research.
- Addressing limitations: Mixed methods research can address the limitations of qualitative or quantitative research by compensating for the weaknesses of each method.
- Flexibility : Mixed methods research is flexible, allowing researchers to adapt the research design and methods as needed to best address the research question.
- Validity : Mixed methods research can increase the validity of the research by using multiple methods to measure the same concept.
- Generalizability : Mixed methods research can improve the generalizability of the findings by using quantitative data to test the applicability of qualitative findings to a larger population.
- Practical applications: Mixed methods research is useful for developing practical applications, such as interventions or policies, as it provides a more comprehensive understanding of the research problem.
Limitations of Mixed Methods Research
Here are some of the main limitations of mixed methods research:
- Time-consuming: Mixed methods research can be time-consuming and may require more resources than using only one research method.
- Complex data analysis: Integrating qualitative and quantitative data can be challenging and requires specialized skills for data analysis.
- Sampling bias: Mixed methods research can be subject to sampling bias, particularly if the sampling strategies for the qualitative and quantitative components are not aligned.
- Validity and reliability: Mixed methods research requires careful attention to the validity and reliability of both the qualitative and quantitative data, as well as the integration of the two data types.
- Difficulty in balancing the two methods: Mixed methods research can be difficult to balance the qualitative and quantitative methods effectively, particularly if one method dominates the other.
- Theoretical and philosophical issues: Mixed methods research raises theoretical and philosophical questions about the compatibility of qualitative and quantitative research methods and the underlying assumptions about the nature of reality and knowledge.
About the author
Muhammad Hassan
Researcher, Academic Writer, Web developer
You may also like

Experimental Design – Types, Methods, Guide

Qualitative Research – Methods, Analysis Types...

Triangulation in Research – Types, Methods and...

Quasi-Experimental Research Design – Types...

Observational Research – Methods and Guide

Phenomenology – Methods, Examples and Guide
Have a language expert improve your writing
Run a free plagiarism check in 10 minutes, generate accurate citations for free.
- Knowledge Base
Methodology
- Mixed Methods Research | Definition, Guide & Examples
Mixed Methods Research | Definition, Guide & Examples
Published on August 13, 2021 by Tegan George . Revised on June 22, 2023.
Mixed methods research combines elements of quantitative research and qualitative research in order to answer your research question . Mixed methods can help you gain a more complete picture than a standalone quantitative or qualitative study, as it integrates benefits of both methods.
Mixed methods research is often used in the behavioral, health, and social sciences, especially in multidisciplinary settings and complex situational or societal research.
- To what extent does the frequency of traffic accidents ( quantitative ) reflect cyclist perceptions of road safety ( qualitative ) in Amsterdam?
- How do student perceptions of their school environment ( qualitative ) relate to differences in test scores ( quantitative ) ?
- How do interviews about job satisfaction at Company X ( qualitative ) help explain year-over-year sales performance and other KPIs ( quantitative ) ?
- How can voter and non-voter beliefs about democracy ( qualitative ) help explain election turnout patterns ( quantitative ) in Town X?
- How do average hospital salary measurements over time (quantitative) help to explain nurse testimonials about job satisfaction (qualitative) ?
Table of contents
When to use mixed methods research, mixed methods research designs, advantages of mixed methods research, disadvantages of mixed methods research, other interesting articles, frequently asked questions.
Mixed methods research may be the right choice if your research process suggests that quantitative or qualitative data alone will not sufficiently answer your research question. There are several common reasons for using mixed methods research:
- Generalizability : Qualitative research usually has a smaller sample size , and thus is not generalizable. In mixed methods research, this comparative weakness is mitigated by the comparative strength of “large N,” externally valid quantitative research.
- Contextualization: Mixing methods allows you to put findings in context and add richer detail to your conclusions. Using qualitative data to illustrate quantitative findings can help “put meat on the bones” of your analysis.
- Credibility: Using different methods to collect data on the same subject can make your results more credible. If the qualitative and quantitative data converge, this strengthens the validity of your conclusions. This process is called triangulation .
As you formulate your research question , try to directly address how qualitative and quantitative methods will be combined in your study. If your research question can be sufficiently answered via standalone quantitative or qualitative analysis, a mixed methods approach may not be the right fit.
But mixed methods might be a good choice if you want to meaningfully integrate both of these questions in one research study.
Keep in mind that mixed methods research doesn’t just mean collecting both types of data; you need to carefully consider the relationship between the two and how you’ll integrate them into coherent conclusions.
Mixed methods can be very challenging to put into practice, and comes with the same risk of research biases as standalone studies, so it’s a less common choice than standalone qualitative or qualitative research.
Prevent plagiarism. Run a free check.
There are different types of mixed methods research designs . The differences between them relate to the aim of the research, the timing of the data collection , and the importance given to each data type.
As you design your mixed methods study, also keep in mind:
- Your research approach ( inductive vs deductive )
- Your research questions
- What kind of data is already available for you to use
- What kind of data you’re able to collect yourself.
Here are a few of the most common mixed methods designs.
Convergent parallel
In a convergent parallel design, you collect quantitative and qualitative data at the same time and analyze them separately. After both analyses are complete, compare your results to draw overall conclusions.
- On the qualitative side, you analyze cyclist complaints via the city’s database and on social media to find out which areas are perceived as dangerous and why.
- On the quantitative side, you analyze accident reports in the city’s database to find out how frequently accidents occur in different areas of the city.
In an embedded design, you collect and analyze both types of data at the same time, but within a larger quantitative or qualitative design. One type of data is secondary to the other.
This is a good approach to take if you have limited time or resources. You can use an embedded design to strengthen or supplement your conclusions from the primary type of research design.
Explanatory sequential
In an explanatory sequential design, your quantitative data collection and analysis occurs first, followed by qualitative data collection and analysis.
You should use this design if you think your qualitative data will explain and contextualize your quantitative findings.
Exploratory sequential
In an exploratory sequential design, qualitative data collection and analysis occurs first, followed by quantitative data collection and analysis.
You can use this design to first explore initial questions and develop hypotheses . Then you can use the quantitative data to test or confirm your qualitative findings.
“Best of both worlds” analysis
Combining the two types of data means you benefit from both the detailed, contextualized insights of qualitative data and the generalizable , externally valid insights of quantitative data. The strengths of one type of data often mitigate the weaknesses of the other.
For example, solely quantitative studies often struggle to incorporate the lived experiences of your participants, so adding qualitative data deepens and enriches your quantitative results.
Solely qualitative studies are often not very generalizable, only reflecting the experiences of your participants, so adding quantitative data can validate your qualitative findings.
Method flexibility
Mixed methods are less tied to disciplines and established research paradigms. They offer more flexibility in designing your research, allowing you to combine aspects of different types of studies to distill the most informative results.
Mixed methods research can also combine theory generation and hypothesis testing within a single study, which is unusual for standalone qualitative or quantitative studies.
Mixed methods research is very labor-intensive. Collecting, analyzing, and synthesizing two types of data into one research product takes a lot of time and effort, and often involves interdisciplinary teams of researchers rather than individuals. For this reason, mixed methods research has the potential to cost much more than standalone studies.
Differing or conflicting results
If your analysis yields conflicting results, it can be very challenging to know how to interpret them in a mixed methods study. If the quantitative and qualitative results do not agree or you are concerned you may have confounding variables , it can be unclear how to proceed.
Due to the fact that quantitative and qualitative data take two vastly different forms, it can also be difficult to find ways to systematically compare the results, putting your data at risk for bias in the interpretation stage.
If you want to know more about statistics , methodology , or research bias , make sure to check out some of our other articles with explanations and examples.
- Degrees of freedom
- Null hypothesis
- Discourse analysis
- Control groups
- Non-probability sampling
- Quantitative research
- Inclusion and exclusion criteria
Research bias
- Rosenthal effect
- Implicit bias
- Cognitive bias
- Selection bias
- Negativity bias
- Status quo bias
Quantitative research deals with numbers and statistics, while qualitative research deals with words and meanings.
Quantitative methods allow you to systematically measure variables and test hypotheses . Qualitative methods allow you to explore concepts and experiences in more detail.
In mixed methods research , you use both qualitative and quantitative data collection and analysis methods to answer your research question .
Data collection is the systematic process by which observations or measurements are gathered in research. It is used in many different contexts by academics, governments, businesses, and other organizations.
Triangulation in research means using multiple datasets, methods, theories and/or investigators to address a research question. It’s a research strategy that can help you enhance the validity and credibility of your findings.
Triangulation is mainly used in qualitative research , but it’s also commonly applied in quantitative research . Mixed methods research always uses triangulation.
These are four of the most common mixed methods designs :
- Convergent parallel: Quantitative and qualitative data are collected at the same time and analyzed separately. After both analyses are complete, compare your results to draw overall conclusions.
- Embedded: Quantitative and qualitative data are collected at the same time, but within a larger quantitative or qualitative design. One type of data is secondary to the other.
- Explanatory sequential: Quantitative data is collected and analyzed first, followed by qualitative data. You can use this design if you think your qualitative data will explain and contextualize your quantitative findings.
- Exploratory sequential: Qualitative data is collected and analyzed first, followed by quantitative data. You can use this design if you think the quantitative data will confirm or validate your qualitative findings.
Cite this Scribbr article
If you want to cite this source, you can copy and paste the citation or click the “Cite this Scribbr article” button to automatically add the citation to our free Citation Generator.
George, T. (2023, June 22). Mixed Methods Research | Definition, Guide & Examples. Scribbr. Retrieved September 2, 2024, from https://www.scribbr.com/methodology/mixed-methods-research/
Is this article helpful?
Tegan George
Other students also liked, writing strong research questions | criteria & examples, what is quantitative research | definition, uses & methods, what is qualitative research | methods & examples, "i thought ai proofreading was useless but..".
I've been using Scribbr for years now and I know it's a service that won't disappoint. It does a good job spotting mistakes”
Qualitative Data Analysis
- Choosing QDA software
- Free QDA tools
- Transcription tools
- Social media research
- Mixed and multi-method research
- Network Diagrams
- Publishing qualitative data
- Student specialists
General Information
For assistance, please submit a request . You can also reach us via the chat below, email [email protected] , or join Discord server .
If you've met with us before, tell us how we're doing .
Stay in touch by signing up for our Data Services newsletter .
Service Desk and Chat
Bobst Library , 5th floor
Staffed Hours: Summer 2024
Mondays: 12pm - 5pm Tuesdays: 12pm - 5pm Wednesdays: 12pm - 5pm Thursdays: 12pm - 5pm Fridays: 12pm - 5pm
Data Services closes for winter break at the end of the day on Friday, Dec. 22, 2023. We will reopen on Wednesday, Jan. 3, 2024.
Learning Mixed Methods
- Start-to-Finish Mixed Methods with MAXQDA
- Tutorials and Videos
- Suggested literature: Schoonenboom, J., Johnson, R.B. How to Construct a Mixed Methods Research Design. Köln Z Soziol 69, 107–131 (2017). https://doi.org/10.1007/s11577-017-0454-1 First step: Justify your research plan previously. Why is it relevant to integrate qualitative and quantitative methods for answering your research objectives? Then, select a research design and appropriate methods to gather information.
- Suggested resource: MAXQDA Document Variables tab Second Step: organize your qualitative sources of information with relevant variables. Afterward, identify interviews with the associated date and place, sociodemographic information of interviewees. Identify to which part of the study corresponds the information gathered, exploratory, triangulation, or confirmatory.
- Suggested resource Data Services Data Management Planning Third step: If you are dealing with large sets of text or numeric information. Use data cleaning and codebook procedures.
- Suggested resource: QDA resources at Data Services Fourth Step: Conduct the relevant Qualitative Analysis. Write preliminary Conclusions.
- Suggested literature: Cox V. (2017) Exploratory Data Analysis. In: Translating Statistics to Make Decisions. Apress, Berkeley, CA. https://doi.org/10.1007/978-1-4842-2256-0_3 Fifth Step: Conduct an in-depth exploratory analysis in the case of your quantitative data. Write preliminary conclusions.
- Suggested resource: NYU Data Services Quantitative resources Sixth Step: Do the quantitative relevant analysis. Write preliminary conclusions.
- Suggested resource: General Information about Mixed Methods in MAXQDA. Seventh Step: Quantify themes/codes in your qualitative analysis and do an exploratory analysis according to variables. Write preliminary conclusions.
- Suggested resource: Exporting Data to Excel and SPSS with MAXQDA Eight step: if relevant, export themes and variables to do a quantitative analysis from qualitative data. Write preliminary conclusions.
- Suggested literature: Fielding, N. G. (2012). Triangulation and Mixed Methods Designs: Data Integration With New Research Technologies. Journal of Mixed Methods Research, 6(2), 124–136. https://doi.org/10.1177/1558689812437101 Ninth Step: compare the conclusions from each analysis by seeking similarities and differences.
- Suggested literature: Bergman, M. M. (2008). 7 quality of inferences in mixed methods research: calling for an integrative framework. In Bergman, M. M. (Ed.), Advances in mixed methods research (pp. 101-119). SAGE Publications Ltd, https://www.doi.org/10. Tenth step: conclude according to validity and reliability criteria that match your research paradigm.
- Telling a Complete Story with Qualitative and Mixed Methods Research - A conversation with Dr. John W. Creswell A Sage video interview with one of the leading scholars in Mixed Methods.
- SAGE project planner A step-by-step decision-making process for planning your research project.
- Sage Methods Maps A map for choosing qualitative and quantitative research designs and analysis.
- MAXQDA’s Mixed Methods Features A brief overview of MAXQDA's Mixed-methods functions
- Introduction to Mixed Methods Functions MAXQDA NYU Data services presentation about the Mixed Methods functions in MAXQDA.
- Creswell, John W., "Steps in Conducting a Scholarly Mixed Methods Study" (2013). DBER Speaker Series. 48. https://digitalcommons.unl.edu/dberspeakers/48 A primer to mixed methods research designs and workflows.
- Johnson, R. B., Onwuegbuzie, A. J., & Turner, L. A. (2007). Toward a definition of mixed methods research. Journal of mixed methods research, 1(2), 112-133. The purpose of this article is to examine definitions of mixed methods.
- DeCuir-Gunby, J., & Schutz, P. (2017). Developing a mixed methods proposal: A practical guide for beginning researchers. SAGE Publications, Inc. https://www.doi.org/10.4135/9781483399980 An introductory guide for making mixed-methods proposals
- Schoonenboom, J. (2018). Designing mixed methods research by mixing and merging methodologies: A 13-step model. American Behavioral Scientist, 62(7), 998-1015. In this article, a 13-step model is presented that researchers can follow in designing their own multimethodology research.
- Tashakkori, A., & Teddlie, C. (2010). SAGE handbook of mixed methods in social & behavioral research (2nd ed.). SAGE Publications, Inc. https://www.doi.org/10.4135/9781506335193 A book about different approaches in mixed-methods research.
- Seawright, J., & Gerring, J. (2008). Case selection techniques in case study research: A menu of qualitative and quantitative options. Political research quarterly, 61(2), 294-308. A qualitative and quantitative guide to case selection
- << Previous: Social media research
- Next: Network Diagrams >>
- Last Updated: Aug 27, 2024 5:40 PM
- URL: https://guides.nyu.edu/QDA
- What is mixed methods research?
Last updated
20 February 2023
Reviewed by
Miroslav Damyanov
Short on time? Get an AI generated summary of this article instead
By blending both quantitative and qualitative data, mixed methods research allows for a more thorough exploration of a research question. It can answer complex research queries that cannot be solved with either qualitative or quantitative research .
Analyze your mixed methods research
Dovetail streamlines analysis to help you uncover and share actionable insights
Mixed methods research combines the elements of two types of research: quantitative and qualitative.
Quantitative data is collected through the use of surveys and experiments, for example, containing numerical measures such as ages, scores, and percentages.
Qualitative data involves non-numerical measures like beliefs, motivations, attitudes, and experiences, often derived through interviews and focus group research to gain a deeper understanding of a research question or phenomenon.
Mixed methods research is often used in the behavioral, health, and social sciences, as it allows for the collection of numerical and non-numerical data.
- When to use mixed methods research
Mixed methods research is a great choice when quantitative or qualitative data alone will not sufficiently answer a research question. By collecting and analyzing both quantitative and qualitative data in the same study, you can draw more meaningful conclusions.
There are several reasons why mixed methods research can be beneficial, including generalizability, contextualization, and credibility.
For example, let's say you are conducting a survey about consumer preferences for a certain product. You could collect only quantitative data, such as how many people prefer each product and their demographics. Or you could supplement your quantitative data with qualitative data, such as interviews and focus groups , to get a better sense of why people prefer one product over another.
It is important to note that mixed methods research does not only mean collecting both types of data. Rather, it also requires carefully considering the relationship between the two and method flexibility.
You may find differing or even conflicting results by combining quantitative and qualitative data . It is up to the researcher to then carefully analyze the results and consider them in the context of the research question to draw meaningful conclusions.
When designing a mixed methods study, it is important to consider your research approach, research questions, and available data. Think about how you can use different techniques to integrate the data to provide an answer to your research question.
- Mixed methods research design
A mixed methods research design is an approach to collecting and analyzing both qualitative and quantitative data in a single study.
Mixed methods designs allow for method flexibility and can provide differing and even conflicting results. Examples of mixed methods research designs include convergent parallel, explanatory sequential, and exploratory sequential.
By integrating data from both quantitative and qualitative sources, researchers can gain valuable insights into their research topic . For example, a study looking into the impact of technology on learning could use surveys to measure quantitative data on students' use of technology in the classroom. At the same time, interviews or focus groups can provide qualitative data on students' experiences and opinions.
- Types of mixed method research designs
Researchers often struggle to put mixed methods research into practice, as it is challenging and can lead to research bias. Although mixed methods research can reveal differences or conflicting results between studies, it can also offer method flexibility.
Designing a mixed methods study can be broken down into four types: convergent parallel, embedded, explanatory sequential, and exploratory sequential.
Convergent parallel
The convergent parallel design is when data collection and analysis of both quantitative and qualitative data occur simultaneously and are analyzed separately. This design aims to create mutually exclusive sets of data that inform each other.
For example, you might interview people who live in a certain neighborhood while also conducting a survey of the same people to determine their satisfaction with the area.
Embedded design
The embedded design is when the quantitative and qualitative data are collected simultaneously, but the qualitative data is embedded within the quantitative data. This design is best used when you want to focus on the quantitative data but still need to understand how the qualitative data further explains it.
For instance, you may survey students about their opinions of an online learning platform and conduct individual interviews to gain further insight into their responses.
Explanatory sequential design
In an explanatory sequential design, quantitative data is collected first, followed by qualitative data. This design is used when you want to further explain a set of quantitative data with additional qualitative information.
An example of this would be if you surveyed employees at a company about their satisfaction with their job and then conducted interviews to gain more information about why they responded the way they did.
Exploratory sequential design
The exploratory sequential design collects qualitative data first, followed by quantitative data. This type of mixed methods research is used when the goal is to explore a topic before collecting any quantitative data.
An example of this could be studying how parents interact with their children by conducting interviews and then using a survey to further explore and measure these interactions.
Integrating data in mixed methods studies can be challenging, but it can be done successfully with careful planning.
No matter which type of design you choose, understanding and applying these principles can help you draw meaningful conclusions from your research.
- Strengths of mixed methods research
Mixed methods research designs combine the strengths of qualitative and quantitative data, deepening and enriching qualitative results with quantitative data and validating quantitative findings with qualitative data. This method offers more flexibility in designing research, combining theory generation and hypothesis testing, and being less tied to disciplines and established research paradigms.
Take the example of a study examining the impact of exercise on mental health. Mixed methods research would allow for a comprehensive look at the issue from different angles.
Researchers could begin by collecting quantitative data through surveys to get an overall view of the participants' levels of physical activity and mental health. Qualitative interviews would follow this to explore the underlying dynamics of participants' experiences of exercise, physical activity, and mental health in greater detail.
Through a mixed methods approach, researchers could more easily compare and contrast their results to better understand the phenomenon as a whole.
Additionally, mixed methods research is useful when there are conflicting or differing results in different studies. By combining both quantitative and qualitative data, mixed methods research can offer insights into why those differences exist.
For example, if a quantitative survey yields one result while a qualitative interview yields another, mixed methods research can help identify what factors influence these differences by integrating data from both sources.
Overall, mixed methods research designs offer a range of advantages for studying complex phenomena. They can provide insight into different elements of a phenomenon in ways that are not possible with either qualitative or quantitative data alone. Additionally, they allow researchers to integrate data from multiple sources to gain a deeper understanding of the phenomenon in question.
- Challenges of mixed methods research
Mixed methods research is labor-intensive and often requires interdisciplinary teams of researchers to collaborate. It also has the potential to cost more than conducting a stand alone qualitative or quantitative study .
Interpreting the results of mixed methods research can be tricky, as it can involve conflicting or differing results. Researchers must find ways to systematically compare the results from different sources and methods to avoid bias.
For example, imagine a situation where a team of researchers has employed an explanatory sequential design for their mixed methods study. After collecting data from both the quantitative and qualitative stages, the team finds that the two sets of data provide differing results. This could be challenging for the team, as they must now decide how to effectively integrate the two types of data in order to reach meaningful conclusions. The team would need to identify method flexibility and be strategic when integrating data in order to draw meaningful conclusions from the conflicting results.
- Advanced frameworks in mixed methods research
Mixed methods research offers powerful tools for investigating complex processes and systems, such as in health and healthcare.
Besides the three basic mixed method designs—exploratory sequential, explanatory sequential, and convergent parallel—you can use one of the four advanced frameworks to extend mixed methods research designs. These include multistage, intervention, case study , and participatory.
This framework mixes qualitative and quantitative data collection methods in stages to gather a more nuanced view of the research question. An example of this is a study that first has an online survey to collect initial data and is followed by in-depth interviews to gain further insights.
Intervention
This design involves collecting quantitative data and then taking action, usually in the form of an intervention or intervention program. An example of this could be a research team who collects data from a group of participants, evaluates it, and then implements an intervention program based on their findings .
This utilizes both qualitative and quantitative research methods to analyze a single case. The researcher will examine the specific case in detail to understand the factors influencing it. An example of this could be a study of a specific business organization to understand the organizational dynamics and culture within the organization.
Participatory
This type of research focuses on the involvement of participants in the research process. It involves the active participation of participants in formulating and developing research questions, data collection, and analysis.
An example of this could be a study that involves forming focus groups with participants who actively develop the research questions and then provide feedback during the data collection and analysis stages.
The flexibility of mixed methods research designs means that researchers can choose any combination of the four frameworks outlined above and other methodologies , such as convergent parallel, explanatory sequential, and exploratory sequential, to suit their particular needs.
Through this method's flexibility, researchers can gain multiple perspectives and uncover differing or even conflicting results when integrating data.
When it comes to integration at the methods level, there are four approaches.
Connecting involves collecting both qualitative and quantitative data during different phases of the research.
Building involves the collection of both quantitative and qualitative data within a single phase.
Merging involves the concurrent collection of both qualitative and quantitative data.
Embedding involves including qualitative data within a quantitative study or vice versa.
- Techniques for integrating data in mixed method studies
Integrating data is an important step in mixed methods research designs. It allows researchers to gain further understanding from their research and gives credibility to the integration process. There are three main techniques for integrating data in mixed methods studies: triangulation protocol, following a thread, and the mixed methods matrix.
Triangulation protocol
This integration method combines different methods with differing or conflicting results to generate one unified answer.
For example, if a researcher wanted to know what type of music teenagers enjoy listening to, they might employ a survey of 1,000 teenagers as well as five focus group interviews to investigate this. The results might differ; the survey may find that rap is the most popular genre, whereas the focus groups may suggest rock music is more widely listened to.
The researcher can then use the triangulation protocol to come up with a unified answer—such as that both rap and rock music are popular genres for teenage listeners.
Following a thread
This is another method of integration where the researcher follows the same theme or idea from one method of data collection to the next.
A research design that follows a thread starts by collecting quantitative data on a specific issue, followed by collecting qualitative data to explain the results. This allows whoever is conducting the research to detect any conflicting information and further look into the conflicting information to understand what is really going on.
For example, a researcher who used this research method might collect quantitative data about how satisfied employees are with their jobs at a certain company, followed by qualitative interviews to investigate why job satisfaction levels are low. They could then use the results to explore any conflicting or differing results, allowing them to gain a deeper understanding of job satisfaction at the company.
By following a thread, the researcher can explore various research topics related to the original issue and gain a more comprehensive view of the issue.
Mixed methods matrix
This technique is a visual representation of the different types of mixed methods research designs and the order in which they should be implemented. It enables researchers to quickly assess their research design and adjust it as needed.
The matrix consists of four boxes with four different types of mixed methods research designs: convergent parallel, explanatory sequential, exploratory sequential, and method flexibility.
For example, imagine a researcher who wanted to understand why people don't exercise regularly. To answer this question, they could use a convergent parallel design, collecting both quantitative (e.g., survey responses) and qualitative (e.g., interviews) data simultaneously.
If the researcher found conflicting results, they could switch to an explanatory sequential design and collect quantitative data first, then follow up with qualitative data if needed. This way, the researcher can make adjustments based on their findings and integrate their data more effectively.
Mixed methods research is a powerful tool for understanding complex research topics. Using qualitative and quantitative data in one study allows researchers to understand their subject more deeply.
Mixed methods research designs such as convergent parallel, explanatory sequential, and exploratory sequential provide method flexibility, enabling researchers to collect both types of data while avoiding the limitations of either approach alone.
However, it's important to remember that mixed methods research can produce differing or even conflicting results, so it's important to be aware of the potential pitfalls and take steps to ensure that data is being correctly integrated. If used effectively, mixed methods research can offer valuable insight into topics that would otherwise remain largely unexplored.
What is an example of mixed methods research?
An example of mixed methods research is a study that combines quantitative and qualitative data. This type of research uses surveys, interviews, and observations to collect data from multiple sources.
Which sampling method is best for mixed methods?
It depends on the research objectives, but a few methods are often used in mixed methods research designs. These include snowball sampling, convenience sampling, and purposive sampling. Each method has its own advantages and disadvantages.
What is the difference between mixed methods and multiple methods?
Mixed methods research combines quantitative and qualitative data in a single study. Multiple methods involve collecting data from different sources, such as surveys and interviews, but not necessarily combining them into one analysis. Mixed methods offer greater flexibility but can lead to differing or conflicting results when integrating data.
Should you be using a customer insights hub?
Do you want to discover previous research faster?
Do you share your research findings with others?
Do you analyze research data?
Start for free today, add your research, and get to key insights faster
Editor’s picks
Last updated: 18 April 2023
Last updated: 27 February 2023
Last updated: 22 August 2024
Last updated: 5 February 2023
Last updated: 16 August 2024
Last updated: 9 March 2023
Last updated: 30 April 2024
Last updated: 12 December 2023
Last updated: 11 March 2024
Last updated: 4 July 2024
Last updated: 6 March 2024
Last updated: 5 March 2024
Last updated: 13 May 2024
Latest articles
Related topics, .css-je19u9{-webkit-align-items:flex-end;-webkit-box-align:flex-end;-ms-flex-align:flex-end;align-items:flex-end;display:-webkit-box;display:-webkit-flex;display:-ms-flexbox;display:flex;-webkit-flex-direction:row;-ms-flex-direction:row;flex-direction:row;-webkit-box-flex-wrap:wrap;-webkit-flex-wrap:wrap;-ms-flex-wrap:wrap;flex-wrap:wrap;-webkit-box-pack:center;-ms-flex-pack:center;-webkit-justify-content:center;justify-content:center;row-gap:0;text-align:center;max-width:671px;}@media (max-width: 1079px){.css-je19u9{max-width:400px;}.css-je19u9>span{white-space:pre;}}@media (max-width: 799px){.css-je19u9{max-width:400px;}.css-je19u9>span{white-space:pre;}} decide what to .css-1kiodld{max-height:56px;display:-webkit-box;display:-webkit-flex;display:-ms-flexbox;display:flex;-webkit-align-items:center;-webkit-box-align:center;-ms-flex-align:center;align-items:center;}@media (max-width: 1079px){.css-1kiodld{display:none;}} build next, decide what to build next, log in or sign up.
Get started for free

An official website of the United States government
The .gov means it’s official. Federal government websites often end in .gov or .mil. Before sharing sensitive information, make sure you’re on a federal government site.
The site is secure. The https:// ensures that you are connecting to the official website and that any information you provide is encrypted and transmitted securely.
- Publications
- Account settings
Preview improvements coming to the PMC website in October 2024. Learn More or Try it out now .
- Advanced Search
- Journal List

Language: English | German
How to Construct a Mixed Methods Research Design
Wie man ein mixed methods-forschungs-design konstruiert, judith schoonenboom.
1 Institut für Bildungswissenschaft, Universität Wien, Sensengasse 3a, 1090 Wien, Austria
R. Burke Johnson
2 Department of Professional Studies, University of South Alabama, UCOM 3700, 36688-0002 Mobile, AL USA
This article provides researchers with knowledge of how to design a high quality mixed methods research study. To design a mixed study, researchers must understand and carefully consider each of the dimensions of mixed methods design, and always keep an eye on the issue of validity. We explain the seven major design dimensions: purpose, theoretical drive, timing (simultaneity and dependency), point of integration, typological versus interactive design approaches, planned versus emergent design, and design complexity. There also are multiple secondary dimensions that need to be considered during the design process. We explain ten secondary dimensions of design to be considered for each research study. We also provide two case studies showing how the mixed designs were constructed.
Zusammenfassung
Der Beitrag gibt einen Überblick darüber, wie das Forschungsdesign bei Mixed Methods-Studien angelegt sein sollte. Um ein Mixed Methods-Forschungsdesign aufzustellen, müssen Forschende sorgfältig alle Dimensionen von Methodenkombinationen abwägen und von Anfang an auf die Güte und damit verbundene etwaige Probleme achten. Wir erklären und diskutieren die für Forschungsdesigns relevanten sieben Dimensionen von Methodenkombinationen: Untersuchungsziel, Rolle von Theorie im Forschungsprozess, Timing (Simultanität und Abhängigkeit), Schnittstellen, an denen Integration stattfindet, systematische vs. interaktive Design-Ansätze, geplante vs. emergente Designs und Komplexität des Designs. Es gibt außerdem zahlreiche sekundäre Dimensionen, die bei der Aufstellung des Forschungsdesigns berücksichtigt werden müssen, von denen wir zehn erklären. Der Beitrag schließt mit zwei Fallbeispielen ab, anhand derer konkret gezeigt wird, wie Mixed Methods-Forschungsdesigns aufgestellt werden können.
What is a mixed methods design?
This article addresses the process of selecting and constructing mixed methods research (MMR) designs. The word “design” has at least two distinct meanings in mixed methods research (Maxwell 2013 ). One meaning focuses on the process of design; in this meaning, design is often used as a verb. Someone can be engaged in designing a study (in German: “eine Studie konzipieren” or “eine Studie designen”). Another meaning is that of a product, namely the result of designing. The result of designing as a verb is a mixed methods design as a noun (in German: “das Forschungsdesign” or “Design”), as it has, for example, been described in a journal article. In mixed methods design, both meanings are relevant. To obtain a strong design as a product, one needs to carefully consider a number of rules for designing as an activity. Obeying these rules is not a guarantee of a strong design, but it does contribute to it. A mixed methods design is characterized by the combination of at least one qualitative and one quantitative research component. For the purpose of this article, we use the following definition of mixed methods research (Johnson et al. 2007 , p. 123):
Mixed methods research is the type of research in which a researcher or team of researchers combines elements of qualitative and quantitative research approaches (e. g., use of qualitative and quantitative viewpoints, data collection, analysis, inference techniques) for the broad purposes of breadth and depth of understanding and corroboration.
Mixed methods research (“Mixed Methods” or “MM”) is the sibling of multimethod research (“Methodenkombination”) in which either solely multiple qualitative approaches or solely multiple quantitative approaches are combined.
In a commonly used mixed methods notation system (Morse 1991 ), the components are indicated as qual and quan (or QUAL and QUAN to emphasize primacy), respectively, for qualitative and quantitative research. As discussed below, plus (+) signs refer to concurrent implementation of components (“gleichzeitige Durchführung der Teilstudien” or “paralleles Mixed Methods-Design”) and arrows (→) refer to sequential implementation (“Sequenzielle Durchführung der Teilstudien” or “sequenzielles Mixed Methods-Design”) of components. Note that each research tradition receives an equal number of letters (four) in its abbreviation for equity. In this article, this notation system is used in some depth.
A mixed methods design as a product has several primary characteristics that should be considered during the design process. As shown in Table 1 , the following primary design “dimensions” are emphasized in this article: purpose of mixing, theoretical drive, timing, point of integration, typological use, and degree of complexity. These characteristics are discussed below. We also provide some secondary dimensions to consider when constructing a mixed methods design (Johnson and Christensen 2017 ).
List of Primary and Secondary Design Dimensions
1. Purpose | Untersuchungsziel |
2. Theoretical drive | Rolle von Theorie im Forschungsprozess |
3. Timing (simultaneity and dependence) | Timing (Simultanität und Abhängigkeit) |
4. Point of integration | Schnittstellen, an denen Integration stattfindet (Integrations-Schnittstellen) |
5. Typological vs. interactive design approach | Systematischer vs. interaktiver Design-Ansatz |
6. Planned vs. emergent design | Geplante vs. emergente Designs |
7. Complexity | Komplexität des Designs |
1. Phenomenon | Untersuchungsgegenstand |
2. Social scientific theory | Ertrag für die sozialwissenschaftliche Theorie (Theoretischer Ertrag) |
3. Ideological drive | Praktische Relevanz |
4. Combination of sampling methods | Kombinierte Stichprobenstrategien |
5. Degree to which the research participants will be similar or different | Grad der (Un)Ähnlichkeit der Forschungsteilnehmenden |
6. Degree to which the researchers on the research team will be similar or different | Grad der (Un)Ähnlichkeit der Forschenden |
7. Type of implementation setting | Untersuchungskontext |
8. Degree to which the methods similar or different | Grad der (Un)Ähnlichkeit der Untersuchungsmethoden |
9. Validity criteria and strategies | Gütekriterien und -strategien |
10. Full study vs. multiple studies | Einzelstudie vs. verschiedene Studien |
On the basis of these dimensions, mixed methods designs can be classified into a mixed methods typology or taxonomy. In the mixed methods literature, various typologies of mixed methods designs have been proposed (for an overview see Creswell and Plano Clark 2011 , p. 69–72).
The overall goal of mixed methods research, of combining qualitative and quantitative research components, is to expand and strengthen a study’s conclusions and, therefore, contribute to the published literature. In all studies, the use of mixed methods should contribute to answering one’s research questions.
Ultimately, mixed methods research is about heightened knowledge and validity. The design as a product should be of sufficient quality to achieve multiple validities legitimation (Johnson and Christensen 2017 ; Onwuegbuzie and Johnson 2006 ), which refers to the mixed methods research study meeting the relevant combination or set of quantitative, qualitative, and mixed methods validities in each research study.
Given this goal of answering the research question(s) with validity, a researcher can nevertheless have various reasons or purposes for wanting to strengthen the research study and its conclusions. Following is the first design dimension for one to consider when designing a study: Given the research question(s), what is the purpose of the mixed methods study?
A popular classification of purposes of mixed methods research was first introduced in 1989 by Greene, Caracelli, and Graham, based on an analysis of published mixed methods studies. This classification is still in use (Greene 2007 ). Greene et al. ( 1989 , p. 259) distinguished the following five purposes for mixing in mixed methods research:
1. Triangulation seeks convergence, corroboration, correspondence of results from different methods; 2. Complementarity seeks elaboration, enhancement, illustration, clarification of the results from one method with the results from the other method; 3. Development seeks to use the results from one method to help develop or inform the other method, where development is broadly construed to include sampling and implementation, as well as measurement decisions; 4. Initiation seeks the discovery of paradox and contradiction, new perspectives of frameworks, the recasting of questions or results from one method with questions or results from the other method; 5. Expansion seeks to extend the breadth and range of inquiry by using different methods for different inquiry components.
In the past 28 years, this classification has been supplemented by several others. On the basis of a review of the reasons for combining qualitative and quantitative research mentioned by the authors of mixed methods studies, Bryman ( 2006 ) formulated a list of more concrete rationales for performing mixed methods research (see Appendix). Bryman’s classification breaks down Greene et al.’s ( 1989 ) categories into several aspects, and he adds a number of additional aspects, such as the following:
(a) Credibility – refers to suggestions that employing both approaches enhances the integrity of findings. (b) Context – refers to cases in which the combination is justified in terms of qualitative research providing contextual understanding coupled with either generalizable, externally valid findings or broad relationships among variables uncovered through a survey. (c) Illustration – refers to the use of qualitative data to illustrate quantitative findings, often referred to as putting “meat on the bones” of “dry” quantitative findings. (d) Utility or improving the usefulness of findings – refers to a suggestion, which is more likely to be prominent among articles with an applied focus, that combining the two approaches will be more useful to practitioners and others. (e) Confirm and discover – this entails using qualitative data to generate hypotheses and using quantitative research to test them within a single project. (f) Diversity of views – this includes two slightly different rationales – namely, combining researchers’ and participants’ perspectives through quantitative and qualitative research respectively, and uncovering relationships between variables through quantitative research while also revealing meanings among research participants through qualitative research. (Bryman, p. 106)
Views can be diverse (f) in various ways. Some examples of mixed methods design that include a diversity of views are:
- Iteratively/sequentially connecting local/idiographic knowledge with national/general/nomothetic knowledge;
- Learning from different perspectives on teams and in the field and literature;
- Achieving multiple participation, social justice, and action;
- Determining what works for whom and the relevance/importance of context;
- Producing interdisciplinary substantive theory, including/comparing multiple perspectives and data regarding a phenomenon;
- Juxtaposition-dialogue/comparison-synthesis;
- Breaking down binaries/dualisms (some of both);
- Explaining interaction between/among natural and human systems;
- Explaining complexity.
The number of possible purposes for mixing is very large and is increasing; hence, it is not possible to provide an exhaustive list. Greene et al.’s ( 1989 ) purposes, Bryman’s ( 2006 ) rationales, and our examples of a diversity of views were formulated as classifications on the basis of examination of many existing research studies. They indicate how the qualitative and quantitative research components of a study relate to each other. These purposes can be used post hoc to classify research or a priori in the design of a new study. When designing a mixed methods study, it is sometimes helpful to list the purpose in the title of the study design.
The key point of this section is for the researcher to begin a study with at least one research question and then carefully consider what the purposes for mixing are. One can use mixed methods to examine different aspects of a single research question, or one can use separate but related qualitative and quantitative research questions. In all cases, the mixing of methods, methodologies, and/or paradigms will help answer the research questions and make improvements over a more basic study design. Fuller and richer information will be obtained in the mixed methods study.
Theoretical drive
In addition to a mixing purpose, a mixed methods research study might have an overall “theoretical drive” (Morse and Niehaus 2009 ). When designing a mixed methods study, it is occasionally helpful to list the theoretical drive in the title of the study design. An investigation, in Morse and Niehaus’s ( 2009 ) view, is focused primarily on either exploration-and-description or on testing-and-prediction. In the first case, the theoretical drive is called “inductive” or “qualitative”; in the second case, it is called “deductive” or “quantitative”. In the case of mixed methods, the component that corresponds to the theoretical drive is referred to as the “core” component (“Kernkomponente”), and the other component is called the “supplemental” component (“ergänzende Komponente”). In Morse’s notation system, the core component is written in capitals and the supplemental component is written in lowercase letters. For example, in a QUAL → quan design, more weight is attached to the data coming from the core qualitative component. Due to the decisive character of the core component, the core component must be able to stand on its own, and should be implemented rigorously. The supplemental component does not have to stand on its own.
Although this distinction is useful in some circumstances, we do not advise to apply it to every mixed methods design. First, Morse and Niehaus contend that the supplemental component can be done “less rigorously” but do not explain which aspects of rigor can be dropped. In addition, the idea of decreased rigor is in conflict with one key theme of the present article, namely that mixed methods designs should always meet the criterion of multiple validities legitimation (Onwuegbuzie and Johnson 2006 ).
The idea of theoretical drive as explicated by Morse and Niehaus has been criticized. For example, we view a theoretical drive as a feature not of a whole study, but of a research question, or, more precisely, of an interpretation of a research question. For example, if one study includes multiple research questions, it might include several theoretical drives (Schoonenboom 2016 ).
Another criticism of Morse and Niehaus’ conceptualization of theoretical drive is that it does not allow for equal-status mixed methods research (“Mixed Methods Forschung, bei der qualitative und quantitative Methoden die gleiche Bedeutung haben” or “gleichrangige Mixed Methods-Designs”), in which both the qualitative and quantitative component are of equal value and weight; this same criticism applies to Morgan’s ( 2014 ) set of designs. We agree with Greene ( 2015 ) that mixed methods research can be integrated at the levels of method, methodology, and paradigm. In this view, equal-status mixed methods research designs are possible, and they result when both the qualitative and the quantitative components, approaches, and thinking are of equal value, they take control over the research process in alternation, they are in constant interaction, and the outcomes they produce are integrated during and at the end of the research process. Therefore, equal-status mixed methods research (that we often advocate) is also called “interactive mixed methods research”.
Mixed methods research can have three different drives, as formulated by Johnson et al. ( 2007 , p. 123):
Qualitative dominant [or qualitatively driven] mixed methods research is the type of mixed research in which one relies on a qualitative, constructivist-poststructuralist-critical view of the research process, while concurrently recognizing that the addition of quantitative data and approaches are likely to benefit most research projects. Quantitative dominant [or quantitatively driven] mixed methods research is the type of mixed research in which one relies on a quantitative, postpositivist view of the research process, while concurrently recognizing that the addition of qualitative data and approaches are likely to benefit most research projects. (p. 124) The area around the center of the [qualitative-quantitative] continuum, equal status , is the home for the person that self-identifies as a mixed methods researcher. This researcher takes as his or her starting point the logic and philosophy of mixed methods research. These mixed methods researchers are likely to believe that qualitative and quantitative data and approaches will add insights as one considers most, if not all, research questions.
We leave it to the reader to decide if he or she desires to conduct a qualitatively driven study, a quantitatively driven study, or an equal-status/“interactive” study. According to the philosophies of pragmatism (Johnson and Onwuegbuzie 2004 ) and dialectical pluralism (Johnson 2017 ), interactive mixed methods research is very much a possibility. By successfully conducting an equal-status study, the pragmatist researcher shows that paradigms can be mixed or combined, and that the incompatibility thesis does not always apply to research practice. Equal status research is most easily conducted when a research team is composed of qualitative, quantitative, and mixed researchers, interacts continually, and conducts a study to address one superordinate goal.
Timing: simultaneity and dependence
Another important distinction when designing a mixed methods study relates to the timing of the two (or more) components. When designing a mixed methods study, it is usually helpful to include the word “concurrent” (“parallel”) or “sequential” (“sequenziell”) in the title of the study design; a complex design can be partially concurrent and partially sequential. Timing has two aspects: simultaneity and dependence (Guest 2013 ).
Simultaneity (“Simultanität”) forms the basis of the distinction between concurrent and sequential designs. In a sequential design , the quantitative component precedes the qualitative component, or vice versa. In a concurrent design , both components are executed (almost) simultaneously. In the notation of Morse ( 1991 ), concurrence is indicated by a “+” between components (e. g., QUAL + quan), while sequentiality is indicated with a “→” (QUAL → quan). Note that the use of capital letters for one component and lower case letters for another component in the same design suggest that one component is primary and the other is secondary or supplemental.
Some designs are sequential by nature. For example, in a conversion design, qualitative categories and themes might be first obtained by collection and analysis of qualitative data, and then subsequently quantitized (Teddlie and Tashakkori 2009 ). Likewise, with Greene et al.’s ( 1989 ) initiation purpose, the initiation strand follows the unexpected results that it is supposed to explain. In other cases, the researcher has a choice. It is possible, e. g., to collect interview data and survey data of one inquiry simultaneously; in that case, the research activities would be concurrent. It is also possible to conduct the interviews after the survey data have been collected (or vice versa); in that case, research activities are performed sequentially. Similarly, a study with the purpose of expansion can be designed in which data on an effect and the intervention process are collected simultaneously, or they can be collected sequentially.
A second aspect of timing is dependence (“Abhängigkeit”) . We call two research components dependent if the implementation of the second component depends on the results of data analysis in the first component. Two research components are independent , if their implementation does not depend on the results of data analysis in the other component. Often, a researcher has a choice to perform data analysis independently or not. A researcher could analyze interview data and questionnaire data of one inquiry independently; in that case, the research activities would be independent. It is also possible to let the interview questions depend upon the outcomes of the analysis of the questionnaire data (or vice versa); in that case, research activities are performed dependently. Similarly, the empirical outcome/effect and process in a study with the purpose of expansion might be investigated independently, or the process study might take the effect/outcome as given (dependent).
In the mixed methods literature, the distinction between sequential and concurrent usually refers to the combination of concurrent/independent and sequential/dependent, and to the combination of data collection and data analysis. It is said that in a concurrent design, the data collection and data analysis of both components occurs (almost) simultaneously and independently, while in a sequential design, the data collection and data analysis of one component take place after the data collection and data analysis of the other component and depends on the outcomes of the other component.
In our opinion, simultaneity and dependence are two separate dimensions. Simultaneity indicates whether data collection is done concurrent or sequentially. Dependence indicates whether the implementation of one component depends upon the results of data analysis of the other component. As we will see in the example case studies, a concurrent design could include dependent data analysis, and a sequential design could include independent data analysis. It is conceivable that one simultaneously conducts interviews and collects questionnaire data (concurrent), while allowing the analysis focus of the interviews to depend on what emerges from the survey data (dependence).
Dependent research activities include a redirection of subsequent research inquiry. Using the outcomes of the first research component, the researcher decides what to do in the second component. Depending on the outcomes of the first research component, the researcher will do something else in the second component. If this is so, the research activities involved are said to be sequential-dependent, and any component preceded by another component should appropriately build on the previous component (see sequential validity legitimation ; Johnson and Christensen 2017 ; Onwuegbuzie and Johnson 2006 ).
It is under the purposive discretion of the researcher to determine whether a concurrent-dependent design, a concurrent-independent design, a sequential-dependent design, or a sequential-dependent design is needed to answer a particular research question or set of research questions in a given situation.
Point of integration
Each true mixed methods study has at least one “point of integration” – called the “point of interface” by Morse and Niehaus ( 2009 ) and Guest ( 2013 ) –, at which the qualitative and quantitative components are brought together. Having one or more points of integration is the distinguishing feature of a design based on multiple components. It is at this point that the components are “mixed”, hence the label “mixed methods designs”. The term “mixing”, however, is misleading, as the components are not simply mixed, but have to be integrated very carefully.
Determining where the point of integration will be, and how the results will be integrated, is an important, if not the most important, decision in the design of mixed methods research. Morse and Niehaus ( 2009 ) identify two possible points of integration: the results point of integration and the analytical point of integration.
Most commonly, integration takes place in the results point of integration . At some point in writing down the results of the first component, the results of the second component are added and integrated. A joint display (listing the qualitative and quantitative findings and an integrative statement) might be used to facilitate this process.
In the case of an analytical point of integration , a first analytical stage of a qualitative component is followed by a second analytical stage, in which the topics identified in the first analytical stage are quantitized. The results of the qualitative component ultimately, and before writing down the results of the analytical phase as a whole, become quantitative; qualitizing also is a possible strategy, which would be the converse of this.
Other authors assume more than two possible points of integration. Teddlie and Tashakkori ( 2009 ) distinguish four different stages of an investigation: the conceptualization stage, the methodological experimental stage (data collection), the analytical experimental stage (data analysis), and the inferential stage. According to these authors, in all four stages, mixing is possible, and thus all four stages are potential points or integration.
However, the four possible points of integration used by Teddlie and Tashakkori ( 2009 ) are still too coarse to distinguish some types of mixing. Mixing in the experiential stage can take many different forms, for example the use of cognitive interviews to improve a questionnaire (tool development), or selecting people for an interview on the basis of the results of a questionnaire (sampling). Extending the definition by Guest ( 2013 ), we define the point of integration as “any point in a study where two or more research components are mixed or connected in some way”. Then, the point of integration in the two examples of this paragraph can be defined more accurately as “instrument development”, and “development of the sample”.
It is at the point of integration that qualitative and quantitative components are integrated. Some primary ways that the components can be connected to each other are as follows:
(1) merging the two data sets, (2) connecting from the analysis of one set of data to the collection of a second set of data, (3) embedding of one form of data within a larger design or procedure, and (4) using a framework (theoretical or program) to bind together the data sets (Creswell and Plano Clark 2011 , p. 76).
More generally, one can consider mixing at any or all of the following research components: purposes, research questions, theoretical drive, methods, methodology, paradigm, data, analysis, and results. One can also include mixing views of different researchers, participants, or stakeholders. The creativity of the mixed methods researcher designing a study is extensive.
Substantively, it can be useful to think of integration or mixing as comparing and bringing together two (or more) components on the basis of one or more of the purposes set out in the first section of this article. For example, it is possible to use qualitative data to illustrate a quantitative effect, or to determine whether the qualitative and the quantitative component yield convergent results ( triangulation ). An integrated result could also consist of a combination of a quantitatively established effect and a qualitative description of the underlying process . In the case of development, integration consists of an adjustment of an, often quantitative, for example, instrument or model or interpretation, based on qualitative assessments by members of the target group.
A special case is the integration of divergent results. The power of mixed methods research is its ability to deal with diversity and divergence. In the literature, we find two kinds of strategies for dealing with divergent results. A first set of strategies takes the detected divergence as the starting point for further analysis, with the aim to resolve the divergence. One possibility is to carry out further research (Cook 1985 ; Greene and Hall 2010 ). Further research is not always necessary. One can also look for a more comprehensive theory, which is able to account for both the results of the first component and the deviating results of the second component. This is a form of abduction (Erzberger and Prein 1997 ).
A fruitful starting point in trying to resolve divergence through abduction is to determine which component has resulted in a finding that is somehow expected, logical, and/or in line with existing research. The results of this research component, called the “sense” (“Lesart”), are subsequently compared to the results of the other component, called the “anti-sense” (“alternative Lesart”), which are considered dissonant, unexpected, and/or contrary to what had been found in the literature. The aim is to develop an overall explanation that fits both the sense and the anti-sense (Bazeley and Kemp 2012 ; Mendlinger and Cwikel 2008 ). Finally, a reanalysis of the data can sometimes lead to resolving divergence (Creswell and Plano Clark 2011 ).
Alternatively, one can question the existence of the encountered divergence. In this regard, Mathison ( 1988 ) recommends determining whether deviating results shown by the data can be explained by knowledge about the research and/or knowledge of the social world. Differences between results from different data sources could also be the result of properties of the methods involved, rather than reflect differences in reality (Yanchar and Williams 2006 ). In general, the conclusions of the individual components can be subjected to an inference quality audit (Teddlie and Tashakkori 2009 ), in which the researcher investigates the strength of each of the divergent conclusions. We recommend that researchers first determine whether there is “real” divergence, according to the strategies mentioned in the last paragraph. Next, an attempt can be made to resolve cases of “true” divergence, using one or more of the methods mentioned in this paragraph.
Design typology utilization
As already mentioned in Sect. 1, mixed methods designs can be classified into a mixed methods typology or taxonomy. A typology serves several purposes, including the following: guiding practice, legitimizing the field, generating new possibilities, and serving as a useful pedagogical tool (Teddlie and Tashakkori 2009 ). Note, however, that not all types of typologies are equally suitable for all purposes. For generating new possibilities, one will need a more exhaustive typology, while a useful pedagogical tool might be better served by a non-exhaustive overview of the most common mixed methods designs. Although some of the current MM design typologies include more designs than others, none of the current typologies is fully exhaustive. When designing a mixed methods study, it is often useful to borrow its name from an existing typology, or to construct a superior and nuanced clear name when your design is based on a modification of one or more of the designs.
Various typologies of mixed methods designs have been proposed. Creswell and Plano Clark’s ( 2011 ) typology of some “commonly used designs” includes six “major mixed methods designs”. Our summary of these designs runs as follows:
- Convergent parallel design (“paralleles Design”) (the quantitative and qualitative strands of the research are performed independently, and their results are brought together in the overall interpretation),
- Explanatory sequential design (“explanatives Design”) (a first phase of quantitative data collection and analysis is followed by the collection of qualitative data, which are used to explain the initial quantitative results),
- Exploratory sequential design (“exploratives Design”) (a first phase of qualitative data collection and analysis is followed by the collection of quantitative data to test or generalize the initial qualitative results),
- Embedded design (“Einbettungs-Design”) (in a traditional qualitative or quantitative design, a strand of the other type is added to enhance the overall design),
- Transformative design (“politisch-transformatives Design”) (a transformative theoretical framework, e. g. feminism or critical race theory, shapes the interaction, priority, timing and mixing of the qualitative and quantitative strand),
- Multiphase design (“Mehrphasen-Design”) (more than two phases or both sequential and concurrent strands are combined over a period of time within a program of study addressing an overall program objective).
Most of their designs presuppose a specific juxtaposition of the qualitative and quantitative component. Note that the last design is a complex type that is required in many mixed methods studies.
The following are our adapted definitions of Teddlie and Tashakkori’s ( 2009 ) five sets of mixed methods research designs (adapted from Teddlie and Tashakkori 2009 , p. 151):
- Parallel mixed designs (“paralleles Mixed-Methods-Design”) – In these designs, one has two or more parallel quantitative and qualitative strands, either with some minimal time lapse or simultaneously; the strand results are integrated into meta-inferences after separate analysis are conducted; related QUAN and QUAL research questions are answered or aspects of the same mixed research question is addressed.
- Sequential mixed designs (“sequenzielles Mixed-Methods-Design”) – In these designs, QUAL and QUAN strands occur across chronological phases, and the procedures/questions from the later strand emerge/depend/build on on the previous strand; the research questions are interrelated and sometimes evolve during the study.
- Conversion mixed designs (“Transfer-Design” or “Konversionsdesign”) – In these parallel designs, mixing occurs when one type of data is transformed to the other type and then analyzed, and the additional findings are added to the results; this design answers related aspects of the same research question,
- Multilevel mixed designs (“Mehrebenen-Mixed-Methods-Design”) – In these parallel or sequential designs, mixing occurs across multiple levels of analysis, as QUAN and QUAL data are analyzed and integrated to answer related aspects of the same research question or related questions.
- Fully integrated mixed designs (“voll integriertes Mixed-Methods-Design”) – In these designs, mixing occurs in an interactive manner at all stages of the study. At each stage, one approach affects the formulation of the other, and multiple types of implementation processes can occur. For example, rather than including integration only at the findings/results stage, or only across phases in a sequential design, mixing might occur at the conceptualization stage, the methodological stage, the analysis stage, and the inferential stage.
We recommend adding to Teddlie and Tashakkori’s typology a sixth design type, specifically, a “hybrid” design type to include complex combinations of two or more of the other design types. We expect that many published MM designs will fall into the hybrid design type.
Morse and Niehaus ( 2009 ) listed eight mixed methods designs in their book (and suggested that authors create more complex combinations when needed). Our shorthand labels and descriptions (adapted from Morse and Niehaus 2009 , p. 25) run as follows:
- QUAL + quan (inductive-simultaneous design where, the core component is qualitative and the supplemental component is quantitative)
- QUAL → quan (inductive-sequential design, where the core component is qualitative and the supplemental component is quantitative)
- QUAN + qual (deductive-simultaneous design where, the core component is quantitative and the supplemental component is qualitative)
- QUAN → qual (deductive-sequential design, where the core component is quantitative and the supplemental component is qualitative)
- QUAL + qual (inductive-simultaneous design, where both components are qualitative; this is a multimethod design rather than a mixed methods design)
- QUAL → qual (inductive-sequential design, where both components are qualitative; this is a multimethod design rather than a mixed methods design)
- QUAN + quan (deductive-simultaneous design, where both components are quantitative; this is a multimethod design rather than a mixed methods design)
- QUAN → quan (deductive-sequential design, where both components are quantitative; this is a multimethod design rather than a mixed methods design).
Notice that Morse and Niehaus ( 2009 ) included four mixed methods designs (the first four designs shown above) and four multimethod designs (the second set of four designs shown above) in their typology. The reader can, therefore, see that the design notation also works quite well for multimethod research designs. Notably absent from Morse and Niehaus’s book are equal-status or interactive designs. In addition, they assume that the core component should always be performed either concurrent with or before the supplemental component.
Johnson, Christensen, and Onwuegbuzie constructed a set of mixed methods designs without these limitations. The resulting mixed methods design matrix (see Johnson and Christensen 2017 , p. 478) contains nine designs, which we can label as follows (adapted from Johnson and Christensen 2017 , p. 478):
- QUAL + QUAN (equal-status concurrent design),
- QUAL + quan (qualitatively driven concurrent design),
- QUAN + qual (quantitatively driven concurrent design),
- QUAL → QUAN (equal-status sequential design),
- QUAN → QUAL (equal-status sequential design),
- QUAL → quan (qualitatively driven sequential design),
- qual → QUAN (quantitatively driven sequential design),
- QUAN → qual (quantitatively driven sequential design), and
- quan → QUAL (qualitatively driven sequential design).
The above set of nine designs assumed only one qualitative and one quantitative component. However, this simplistic assumption can be relaxed in practice, allowing the reader to construct more complex designs. The Morse notation system is very powerful. For example, here is a three-stage equal-status concurrent-sequential design:
The key point here is that the Morse notation provides researchers with a powerful language for depicting and communicating the design constructed for a specific research study.
When designing a mixed methods study, it is sometimes helpful to include the mixing purpose (or characteristic on one of the other dimensions shown in Table 1 ) in the title of the study design (e. g., an explanatory sequential MM design, an exploratory-confirmatory MM design, a developmental MM design). Much more important, however, than a design name is for the author to provide an accurate description of what was done in the research study, so the reader will know exactly how the study was conducted. A design classification label can never replace such a description.
The common complexity of mixed methods design poses a problem to the above typologies of mixed methods research. The typologies were designed to classify whole mixed methods studies, and they are basically based on a classification of simple designs. In practice, many/most designs are complex. Complex designs are sometimes labeled “complex design”, “multiphase design”, “fully integrated design”, “hybrid design” and the like. Because complex designs occur very often in practice, the above typologies are not able to classify a large part of existing mixed methods research any further than by labeling them “complex”, which in itself is not very informative about the particular design. This problem does not fully apply to Morse’s notation system, which can be used to symbolize some more complex designs.
Something similar applies to the classification of the purposes of mixed methods research. The classifications of purposes mentioned in the “Purpose”-section, again, are basically meant for the classification of whole mixed methods studies. In practice, however, one single study often serves more than one purpose (Schoonenboom et al. 2017 ). The more purposes that are included in one study, the more difficult it becomes to select a design on the basis of the purpose of the investigation, as advised by Greene ( 2007 ). Of all purposes involved, then, which one should be the primary basis for the design? Or should the design be based upon all purposes included? And if so, how? For more information on how to articulate design complexity based on multiple purposes of mixing, see Schoonenboom et al. ( 2017 ).
It should be clear to the reader that, although much progress has been made in the area of mixed methods design typologies, the problem remains in developing a single typology that is effective in comprehensively listing a set of designs for mixed methods research. This is why we emphasize in this article the importance of learning to build on simple designs and construct one’s own design for one’s research questions. This will often result in a combination or “hybrid” design that goes beyond basic designs found in typologies, and a methodology section that provides much more information than a design name.
Typological versus interactive approaches to design
In the introduction, we made a distinction between design as a product and design as a process. Related to this, two different approaches to design can be distinguished: typological/taxonomic approaches (“systematische Ansätze”), such as those in the previous section, and interactive approaches (“interaktive Ansätze”) (the latter were called “dynamic” approaches by Creswell and Plano Clark 2011 ). Whereas typological/taxonomic approaches view designs as a sort of mold, in which the inquiry can be fit, interactive approaches (Maxwell 2013 ) view design as a process, in which a certain design-as-a-product might be the outcome of the process, but not its input.
The most frequently mentioned interactive approach to mixed methods research is the approach by Maxwell and Loomis ( 2003 ). Maxwell and Loomis distinguish the following components of a design: goals, conceptual framework, research question, methods, and validity. They argue convincingly that the most important task of the researcher is to deliver as the end product of the design process a design in which these five components fit together properly. During the design process, the researcher works alternately on the individual components, and as a result, their initial fit, if it existed, tends to get lost. The researcher should therefore regularly check during the research and continuing design process whether the components still fit together, and, if not, should adapt one or the other component to restore the fit between them. In an interactive approach, unlike the typological approach, design is viewed as an interactive process in which the components are continually compared during the research study to each other and adapted to each other.
Typological and interactive approaches to mixed methods research have been presented as mutually exclusive alternatives. In our view, however, they are not mutually exclusive. The interactive approach of Maxwell is a very powerful tool for conducting research, yet this approach is not specific to mixed methods research. Maxwell’s interactive approach emphasizes that the researcher should keep and monitor a close fit between the five components of research design. However, it does not indicate how one should combine qualitative and quantitative subcomponents within one of Maxwell’s five components (e. g., how one should combine a qualitative and a quantitative method, or a qualitative and a quantitative research question). Essential elements of the design process, such as timing and the point of integration are not covered by Maxwell’s approach. This is not a shortcoming of Maxwell’s approach, but it indicates that to support the design of mixed methods research, more is needed than Maxwell’s model currently has to offer.
Some authors state that design typologies are particularly useful for beginning researchers and interactive approaches are suited for experienced researchers (Creswell and Plano Clark 2011 ). However, like an experienced researcher, a research novice needs to align the components of his or her design properly with each other, and, like a beginning researcher, an advanced researcher should indicate how qualitative and quantitative components are combined with each other. This makes an interactive approach desirable, also for beginning researchers.
We see two merits of the typological/taxonomic approach . We agree with Greene ( 2007 ), who states that the value of the typological approach mainly lies in the different dimensions of mixed methods that result from its classifications. In this article, the primary dimensions include purpose, theoretical drive, timing, point of integration, typological vs. interactive approaches, planned vs. emergent designs, and complexity (also see secondary dimensions in Table 1 ). Unfortunately, all of these dimensions are not reflected in any single design typology reviewed here. A second merit of the typological approach is the provision of common mixed methods research designs, of common ways in which qualitative and quantitative research can be combined, as is done for example in the major designs of Creswell and Plano Clark ( 2011 ). Contrary to other authors, however, we do not consider these designs as a feature of a whole study, but rather, in line with Guest ( 2013 ), as a feature of one part of a design in which one qualitative and one quantitative component are combined. Although one study could have only one purpose, one point of integration, et cetera, we believe that combining “designs” is the rule and not the exception. Therefore, complex designs need to be constructed and modified as needed, and during the writing phase the design should be described in detail and perhaps given a creative and descriptive name.
Planned versus emergent designs
A mixed methods design can be thought out in advance, but can also arise during the course of the conduct of the study; the latter is called an “emergent” design (Creswell and Plano Clark 2011 ). Emergent designs arise, for example, when the researcher discovers during the study that one of the components is inadequate (Morse and Niehaus 2009 ). Addition of a component of the other type can sometimes remedy such an inadequacy. Some designs contain an emergent component by their nature. Initiation, for example, is the further exploration of unexpected outcomes. Unexpected outcomes are by definition not foreseen, and therefore cannot be included in the design in advance.
The question arises whether researchers should plan all these decisions beforehand, or whether they can make them during, and depending on the course of, the research process. The answer to this question is twofold. On the one hand, a researcher should decide beforehand which research components to include in the design, such that the conclusion that will be drawn will be robust. On the other hand, developments during research execution will sometimes prompt the researcher to decide to add additional components. In general, the advice is to be prepared for the unexpected. When one is able to plan for emergence, one should not refrain from doing so.
Dimension of complexity
Next, mixed methods designs are characterized by their complexity. In the literature, simple and complex designs are distinguished in various ways. A common distinction is between simple investigations with a single point of integration versus complex investigations with multiple points of integration (Guest 2013 ). When designing a mixed methods study, it can be useful to mention in the title whether the design of the study is simple or complex. The primary message of this section is as follows: It is the responsibility of the researcher to create more complex designs when needed to answer his or her research question(s) .
Teddlie and Tashakkori’s ( 2009 ) multilevel mixed designs and fully integrated mixed designs are both complex designs, but for different reasons. A multilevel mixed design is more complex ontologically, because it involves multiple levels of reality. For example, data might be collected both at the levels of schools and students, neighborhood and households, companies and employees, communities and inhabitants, or medical practices and patients (Yin 2013 ). Integration of these data does not only involve the integration of qualitative and quantitative data, but also the integration of data originating from different sources and existing at different levels. Little if any published research has discussed the possible ways of integrating data obtained in a multilevel mixed design (see Schoonenboom 2016 ). This is an area in need of additional research.
The fully-integrated mixed design is more complex because it contains multiple points of integration. As formulated by Teddlie and Tashakkori ( 2009 , p. 151):
In these designs, mixing occurs in an interactive manner at all stages of the study. At each stage, one approach affects the formulation of the other, and multiple types of implementation processes can occur.
Complexity, then, not only depends on the number of components, but also on the extent to which they depend on each other (e. g., “one approach affects the formulation of the other”).
Many of our design dimensions ultimately refer to different ways in which the qualitative and quantitative research components are interdependent. Different purposes of mixing ultimately differ in the way one component relates to, and depends upon, the other component. For example, these purposes include dependencies, such as “x illustrates y” and “x explains y”. Dependencies in the implementation of x and y occur to the extent that the design of y depends on the results of x (sequentiality). The theoretical drive creates dependencies, because the supplemental component y is performed and interpreted within the context and the theoretical drive of core component x. As a general rule in designing mixed methods research, one should examine and plan carefully the ways in which and the extent to which the various components depend on each other.
The dependence among components, which may or may not be present, has been summarized by Greene ( 2007 ). It is seen in the distinction between component designs (“Komponenten-Designs”), in which the components are independent of each other, and integrated designs (“integrierte Designs”), in which the components are interdependent. Of these two design categories, integrated designs are the more complex designs.
Secondary design considerations
The primary design dimensions explained above have been the focus of this article. There are a number of secondary considerations for researchers to also think about when they design their studies (Johnson and Christensen 2017 ). Now we list some secondary design issues and questions that should be thoughtfully considered during the construction of a strong mixed methods research design.
- Phenomenon: Will the study be addressing (a) the same part or different parts of one phenomenon? (b) different phenomena?, or (c) the phenomenon/phenomena from different perspectives? Is the phenomenon (a) expected to be unique (e. g., historical event, particular group)?, (b) something expected to be part of a more regular and predictable phenomenon, or (c) a complex mixture of these?
- Social scientific theory: Will the study generate a new substantive theory, test an already constructed theory, or achieve both in a sequential arrangement? Or is the researcher not interested in substantive theory based on empirical data?
- Ideological drive: Will the study have an explicitly articulated ideological drive (e. g., feminism, critical race paradigm, transformative paradigm)?
- Combination of sampling methods: What specific quantitative sampling method(s) will be used? What specific qualitative sampling methods(s) will be used? How will these be combined or related?
- Degree to which the research participants will be similar or different: For example, participants or stakeholders with known differences of perspective would provide participants that are quite different.
- Degree to which the researchers on the research team will be similar or different: For example, an experiment conducted by one researcher would be high on similarity, but the use of a heterogeneous and participatory research team would include many differences.
- Implementation setting: Will the phenomenon be studied naturalistically, experimentally, or through a combination of these?
- Degree to which the methods similar or different: For example, a structured interview and questionnaire are fairly similar but administration of a standardized test and participant observation in the field are quite different.
- Validity criteria and strategies: What validity criteria and strategies will be used to address the defensibility of the study and the conclusions that will be drawn from it (see Chapter 11 in Johnson and Christensen 2017 )?
- Full study: Will there be essentially one research study or more than one? How will the research report be structured?
Two case studies
The above design dimensions are now illustrated by examples. A nice collection of examples of mixed methods studies can be found in Hesse-Biber ( 2010 ), from which the following examples are taken. The description of the first case example is shown in Box 1.
Box 1
Summary of Roth ( 2006 ), research regarding the gender-wage gap within Wall Street securities firms. Adapted from Hesse-Biber ( 2010 , pp. 457–458)
Louise Marie Roth’s research, Selling Women Short: Gender and Money on Wall Street ( 2006 ), tackles gender inequality in the workplace. She was interested in understanding the gender-wage gap among highly performing Wall Street MBAs, who on the surface appeared to have the same “human capital” qualifications and were placed in high-ranking Wall Street securities firms as their first jobs. In addition, Roth wanted to understand the “structural factors” within the workplace setting that may contribute to the gender-wage gap and its persistence over time. […] Roth conducted semistructured interviews, nesting quantitative closed-ended questions into primarily qualitative in-depth interviews […] In analyzing the quantitative data from her sample, she statistically considered all those factors that might legitimately account for gendered differences such as number of hours worked, any human capital differences, and so on. Her analysis of the quantitative data revealed the presence of a significant gender gap in wages that remained unexplained after controlling for any legitimate factors that might otherwise make a difference. […] Quantitative findings showed the extent of the wage gap while providing numerical understanding of the disparity but did not provide her with an understanding of the specific processes within the workplace that might have contributed to the gender gap in wages. […] Her respondents’ lived experiences over time revealed the hidden inner structures of the workplace that consist of discriminatory organizational practices with regard to decision making in performance evaluations that are tightly tied to wage increases and promotion.
This example nicely illustrates the distinction we made between simultaneity and dependency. On the two aspects of the timing dimension, this study was a concurrent-dependent design answering a set of related research questions. The data collection in this example was conducted simultaneously, and was thus concurrent – the quantitative closed-ended questions were embedded into the qualitative in-depth interviews. In contrast, the analysis was dependent, as explained in the next paragraph.
One of the purposes of this study was explanation: The qualitative data were used to understand the processes underlying the quantitative outcomes. It is therefore an explanatory design, and might be labelled an “explanatory concurrent design”. Conceptually, explanatory designs are often dependent: The qualitative component is used to explain and clarify the outcomes of the quantitative component. In that sense, the qualitative analysis in the case study took the outcomes of the quantitative component (“the existence of the gender-wage gap” and “numerical understanding of the disparity”), and aimed at providing an explanation for that result of the quantitative data analysis , by relating it to the contextual circumstances in which the quantitative outcomes were produced. This purpose of mixing in the example corresponds to Bryman’s ( 2006 ) “contextual understanding”. On the other primary dimensions, (a) the design was ongoing over a three-year period but was not emergent, (b) the point of integration was results, and (c) the design was not complex with respect to the point of integration, as it had only one point of integration. Yet, it was complex in the sense of involving multiple levels; both the level of the individual and the organization were included. According to the approach of Johnson and Christensen ( 2017 ), this was a QUAL + quan design (that was qualitatively driven, explanatory, and concurrent). If we give this study design a name, perhaps it should focus on what was done in the study: “explaining an effect from the process by which it is produced”. Having said this, the name “explanatory concurrent design” could also be used.
The description of the second case example is shown in Box 2.
Box 2
Summary of McMahon’s ( 2007 ) explorative study of the meaning, role, and salience of rape myths within the subculture of college student athletes. Adapted from Hesse-Biber ( 2010 , pp. 461–462)
Sarah McMahon ( 2007 ) wanted to explore the subculture of college student athletes and specifically the meaning, role, and salience of rape myths within that culture. […] While she was looking for confirmation between the quantitative ([structured] survey) and qualitative (focus groups and individual interviews) findings, she entered this study skeptical of whether or not her quantitative and qualitative findings would mesh with one another. McMahon […] first administered a survey [instrument] to 205 sophomore and junior student athletes at one Northeast public university. […] The quantitative data revealed a very low acceptance of rape myths among this student population but revealed a higher acceptance of violence among men and individuals who did not know a survivor of sexual assault. In the second qualitative (QUAL) phase, “focus groups were conducted as semi-structured interviews” and facilitated by someone of the same gender as the participants (p. 360). […] She followed this up with a third qualitative component (QUAL), individual interviews, which were conducted to elaborate on themes discovered in the focus groups and determine any differences in students’ responses between situations (i. e., group setting vs. individual). The interview guide was designed specifically to address focus group topics that needed “more in-depth exploration” or clarification (p. 361). The qualitative findings from the focus groups and individual qualitative interviews revealed “subtle yet pervasive rape myths” that fell into four major themes: “the misunderstanding of consent, the belief in ‘accidental’ and fabricated rape, the contention that some women provoke rape, and the invulnerability of female athletes” (p. 363). She found that the survey’s finding of a “low acceptance of rape myths … was contradicted by the findings of the focus groups and individual interviews, which indicated the presence of subtle rape myths” (p. 362).
On the timing dimension, this is an example of a sequential-independent design. It is sequential, because the qualitative focus groups were conducted after the survey was administered. The analysis of the quantitative and qualitative data was independent: Both were analyzed independently, to see whether they yielded the same results (which they did not). This purpose, therefore, was triangulation. On the other primary dimensions, (a) the design was planned, (b) the point of integration was results, and (c) the design was not complex as it had only one point of integration, and involved only the level of the individual. The author called this a “sequential explanatory” design. We doubt, however, whether this is the most appropriate label, because the qualitative component did not provide an explanation for quantitative results that were taken as given. On the contrary, the qualitative results contradicted the quantitative results. Thus, a “sequential-independent” design, or a “sequential-triangulation” design or a “sequential-comparative” design would probably be a better name.
Notice further that the second case study had the same point of integration as the first case study. The two components were brought together in the results. Thus, although the case studies are very dissimilar in many respects, this does not become visible in their point of integration. It can therefore be helpful to determine whether their point of extension is different. A point of extension is the point in the research process at which the second (or later) component comes into play. In the first case study, two related, but different research questions were answered, namely the quantitative question “How large is the gender-wage gap among highly performing Wall Street MBAs after controlling for any legitimate factors that might otherwise make a difference?”, and the qualitative research question “How do structural factors within the workplace setting contribute to the gender-wage gap and its persistence over time?” This case study contains one qualitative research question and one quantitative research question. Therefore, the point of extension is the research question. In the second case study, both components answered the same research question. They differed in their data collection (and subsequently in their data analysis): qualitative focus groups and individual interviews versus a quantitative questionnaire. In this case study, the point of extension was data collection. Thus, the point of extension can be used to distinguish between the two case studies.
Summary and conclusions
The purpose of this article is to help researchers to understand how to design a mixed methods research study. Perhaps the simplest approach is to design is to look at a single book and select one from the few designs included in that book. We believe that is only useful as a starting point. Here we have shown that one often needs to construct a research design to fit one’s unique research situation and questions.
First, we showed that there are there are many purposes for which qualitative and quantitative methods, methodologies, and paradigms can be mixed. This must be determined in interaction with the research questions. Inclusion of a purpose in the design name can sometimes provide readers with useful information about the study design, as in, e. g., an “explanatory sequential design” or an “exploratory-confirmatory design”.
The second dimension is theoretical drive in the sense that Morse and Niehaus ( 2009 ) use this term. That is, will the study have an inductive or a deductive drive, or, we added, a combination of these. Related to this idea is whether one will conduct a qualitatively driven, a quantitatively driven, or an equal-status mixed methods study. This language is sometimes included in the design name to communicate this characteristic of the study design (e. g., a “quantitatively driven sequential mixed methods design”).
The third dimension is timing , which has two aspects: simultaneity and dependence. Simultaneity refers to whether the components are to be implemented concurrently, sequentially, or a combination of these in a multiphase design. Simultaneity is commonly used in the naming of a mixed methods design because it communicates key information. The second aspect of timing, dependence , refers to whether a later component depends on the results of an earlier component, e. g., Did phase two specifically build on phase one in the research study? The fourth design dimension is the point of integration, which is where the qualitative and quantitative components are brought together and integrated. This is an essential dimension, but it usually does not need to be incorporated into the design name.
The fifth design dimension is that of typological vs. interactive design approaches . That is, will one select a design from a typology or use a more interactive approach to construct one’s own design? There are many typologies of designs currently in the literature. Our recommendation is that readers examine multiple design typologies to better understand the design process in mixed methods research and to understand what designs have been identified as popular in the field. However, when a design that would follow from one’s research questions is not available, the researcher can and should (a) combine designs into new designs or (b) simply construct a new and unique design. One can go a long way in depicting a complex design with Morse’s ( 1991 ) notation when used to its full potential. We also recommend that researchers understand the process approach to design from Maxwell and Loomis ( 2003 ), and realize that research design is a process and it needs, oftentimes, to be flexible and interactive.
The sixth design dimension or consideration is whether a design will be fully specified during the planning of the research study or if the design (or part of the design) will be allowed to emerge during the research process, or a combination of these. The seventh design dimension is called complexity . One sort of complexity mentioned was multilevel designs, but there are many complexities that can enter designs. The key point is that good research often requires the use of complex designs to answer one’s research questions. This is not something to avoid. It is the responsibility of the researcher to learn how to construct and describe and name mixed methods research designs. Always remember that designs should follow from one’s research questions and purposes, rather than questions and purposes following from a few currently named designs.
In addition to the six primary design dimensions or considerations, we provided a set of additional or secondary dimensions/considerations or questions to ask when constructing a mixed methods study design. Our purpose throughout this article has been to show what factors must be considered to design a high quality mixed methods research study. The more one knows and thinks about the primary and secondary dimensions of mixed methods design the better equipped one will be to pursue mixed methods research.
Acknowledgments
Open access funding provided by University of Vienna.
Biographies
1965, Dr., Professor of Empirical Pedagogy at University of Vienna, Austria. Research Areas: Mixed Methods Design, Philosophy of Mixed Methods Research, Innovation in Higher Education, Design and Evaluation of Intervention Studies, Educational Technology. Publications: Mixed methods in early childhood education. In: M. Fleer & B. v. Oers (Eds.), International handbook on early childhood education (Vol. 1). Dordrecht, The Netherlands: Springer 2017; The multilevel mixed intact group analysis: A mixed method to seek, detect, describe and explain differences between intact groups. Journal of Mixed Methods Research 10, 2016; The realist survey: How respondents’ voices can be used to test and revise correlational models. Journal of Mixed Methods Research 2015. Advance online publication.
1957, PhD, Professor of Professional Studies at University of South Alabama, Mobile, Alabama USA. Research Areas: Methods of Social Research, Program Evaluation, Quantitative, Qualitative and Mixed Methods, Philosophy of Social Science. Publications: Research methods, design and analysis. Boston, MA 2014 (with L. Christensen and L. Turner); Educational research: Quantitative, qualitative and mixed approaches. Los Angeles, CA 2017 (with L. Christensen); The Oxford handbook of multimethod and mixed methods research inquiry. New York, NY 2015 (with S. Hesse-Biber).
Bryman’s ( 2006 ) scheme of rationales for combining quantitative and qualitative research 1
- Triangulation or greater validity – refers to the traditional view that quantitative and qualitative research might be combined to triangulate findings in order that they may be mutually corroborated. If the term was used as a synonym for integrating quantitative and qualitative research, it was not coded as triangulation.
- Offset – refers to the suggestion that the research methods associated with both quantitative and qualitative research have their own strengths and weaknesses so that combining them allows the researcher to offset their weaknesses to draw on the strengths of both.
- Completeness – refers to the notion that the researcher can bring together a more comprehensive account of the area of enquiry in which he or she is interested if both quantitative and qualitative research are employed.
- Process – quantitative research provides an account of structures in social life but qualitative research provides sense of process.
- Different research questions – this is the argument that quantitative and qualitative research can each answer different research questions but this item was coded only if authors explicitly stated that they were doing this.
- Explanation – one is used to help explain findings generated by the other.
- Unexpected results – refers to the suggestion that quantitative and qualitative research can be fruitfully combined when one generates surprising results that can be understood by employing the other.
- Instrument development – refers to contexts in which qualitative research is employed to develop questionnaire and scale items – for example, so that better wording or more comprehensive closed answers can be generated.
- Sampling – refers to situations in which one approach is used to facilitate the sampling of respondents or cases.
- Credibility – refer s to suggestions that employing both approaches enhances the integrity of findings.
- Context – refers to cases in which the combination is rationalized in terms of qualitative research providing contextual understanding coupled with either generalizable, externally valid findings or broad relationships among variables uncovered through a survey.
- Illustration – refers to the use of qualitative data to illustrate quantitative findings, often referred to as putting “meat on the bones” of “dry” quantitative findings.
- Utility or improving the usefulness of findings – refers to a suggestion, which is more likely to be prominent among articles with an applied focus, that combining the two approaches will be more useful to practitioners and others.
- Confirm and discover – this entails using qualitative data to generate hypotheses and using quantitative research to test them within a single project.
- Diversity of views – this includes two slightly different rationales – namely, combining researchers’ and participants’ perspectives through quantitative and qualitative research respectively, and uncovering relationships between variables through quantitative research while also revealing meanings among research participants through qualitative research.
- Enhancement or building upon quantitative/qualitative findings – this entails a reference to making more of or augmenting either quantitative or qualitative findings by gathering data using a qualitative or quantitative research approach.
- Other/unclear.
- Not stated.
1 Reprinted with permission from “Integrating quantitative and qualitative research: How is it done?” by Alan Bryman ( 2006 ), Qualitative Research, 6, pp. 105–107.
Contributor Information
Judith Schoonenboom, Email: [email protected] .
R. Burke Johnson, Email: ude.amabalahtuos@nosnhojb .
- Bazeley, Pat, Lynn Kemp Mosaics, triangles, and DNA: Metaphors for integrated analysis in mixed methods research. Journal of Mixed Methods Research. 2012; 6 :55–72. doi: 10.1177/1558689811419514. [ CrossRef ] [ Google Scholar ]
- Bryman A. Integrating quantitative and qualitative research: how is it done? Qualitative Research. 2006; 6 :97–113. doi: 10.1177/1468794106058877. [ CrossRef ] [ Google Scholar ]
- Cook TD. Postpositivist critical multiplism. In: Shotland RL, Mark MM, editors. Social science and social policy. Beverly Hills: SAGE; 1985. pp. 21–62. [ Google Scholar ]
- Creswell JW, Plano Clark VL. Designing and conducting mixed methods research. 2. Los Angeles: SAGE; 2011. [ Google Scholar ]
- Erzberger C, Prein G. Triangulation: Validity and empirically-based hypothesis construction. Quality and Quantity. 1997; 31 :141–154. doi: 10.1023/A:1004249313062. [ CrossRef ] [ Google Scholar ]
- Greene JC. Mixed methods in social inquiry. San Francisco: Jossey-Bass; 2007. [ Google Scholar ]
- Greene JC. Preserving distinctions within the multimethod and mixed methods research merger. Sharlene Hesse-Biber and R. Burke Johnson. New York: Oxford University Press; 2015. [ Google Scholar ]
- Greene JC, Valerie J, Caracelli, Graham WF. Toward a conceptual framework for mixed-method evaluation designs. Educational Evaluation and Policy Analysis. 1989; 11 :255–274. doi: 10.3102/01623737011003255. [ CrossRef ] [ Google Scholar ]
- Greene JC, Hall JN. Dialectics and pragmatism. In: Tashakkori A, Teddlie C, editors. SAGE handbook of mixed methods in social & behavioral research. 2. Los Angeles: SAGE; 2010. pp. 119–167. [ Google Scholar ]
- Guest, Greg Describing mixed methods research: An alternative to typologies. Journal of Mixed Methods Research. 2013; 7 :141–151. doi: 10.1177/1558689812461179. [ CrossRef ] [ Google Scholar ]
- Hesse-Biber S. Qualitative approaches to mixed methods practice. Qualitative Inquiry. 2010; 16 :455–468. doi: 10.1177/1077800410364611. [ CrossRef ] [ Google Scholar ]
- Johnson BR. Dialectical pluralism: A metaparadigm whose time has come. Journal of Mixed Methods Research. 2017; 11 :156–173. doi: 10.1177/1558689815607692. [ CrossRef ] [ Google Scholar ]
- Johnson BR, Christensen LB. Educational research: Quantitative, qualitative, and mixed approaches. 6. Los Angeles: SAGE; 2017. [ Google Scholar ]
- Johnson BR, Onwuegbuzie AJ. Mixed methods research: a research paradigm whose time has come. Educational Researcher. 2004; 33 (7):14–26. doi: 10.3102/0013189X033007014. [ CrossRef ] [ Google Scholar ]
- Johnson BR, Onwuegbuzie AJ, Turner LA. Toward a definition of mixed methods research. Journal of Mixed Methods Research. 2007; 1 :112–133. doi: 10.1177/1558689806298224. [ CrossRef ] [ Google Scholar ]
- Mathison S. Why triangulate? Educational Researcher. 1988; 17 :13–17. doi: 10.3102/0013189X017002013. [ CrossRef ] [ Google Scholar ]
- Maxwell JA. Qualitative research design: An interactive approach. 3. Los Angeles: SAGE; 2013. [ Google Scholar ]
- Maxwell, Joseph A., and Diane M. Loomis. 2003. Mixed methods design: An alternative approach. In Handbook of mixed methods in social & behavioral research , Eds. Abbas Tashakkori and Charles Teddlie, 241–271. Thousand Oaks: Sage.
- McMahon S. Understanding community-specific rape myths: Exploring student athlete culture. Affilia. 2007; 22 :357–370. doi: 10.1177/0886109907306331. [ CrossRef ] [ Google Scholar ]
- Mendlinger S, Cwikel J. Spiraling between qualitative and quantitative data on women’s health behaviors: A double helix model for mixed methods. Qualitative Health Research. 2008; 18 :280–293. doi: 10.1177/1049732307312392. [ PubMed ] [ CrossRef ] [ Google Scholar ]
- Morgan DL. Integrating qualitative and quantitative methods: a pragmatic approach. Los Angeles: Sage; 2014. [ Google Scholar ]
- Morse JM. Approaches to qualitative-quantitative methodological triangulation. Nursing Research. 1991; 40 :120–123. doi: 10.1097/00006199-199103000-00014. [ PubMed ] [ CrossRef ] [ Google Scholar ]
- Morse JM, Niehaus L. Mixed method design: Principles and procedures. Walnut Creek: Left Coast Press; 2009. [ Google Scholar ]
- Onwuegbuzie AJ, Burke Johnson R. The “validity” issue in mixed research. Research in the Schools. 2006; 13 :48–63. [ Google Scholar ]
- Roth LM. Selling women short: Gender and money on Wall Street. Princeton: Princeton University Press; 2006. [ Google Scholar ]
- Schoonenboom J. The multilevel mixed intact group analysis: a mixed method to seek, detect, describe and explain differences between intact groups. Journal of Mixed Methods Research. 2016; 10 :129–146. doi: 10.1177/1558689814536283. [ CrossRef ] [ Google Scholar ]
- Schoonenboom, Judith, R. Burke Johnson, and Dominik E. Froehlich. 2017, in press. Combining multiple purposes of mixing within a mixed methods research design. International Journal of Multiple Research Approaches .
- Teddlie CB, Tashakkori A. Foundations of mixed methods research: Integrating quantitative and qualitative approaches in the social and behavioral sciences. Los Angeles: Sage; 2009. [ Google Scholar ]
- Yanchar SC, Williams DD. Reconsidering the compatibility thesis and eclecticism: Five proposed guidelines for method use. Educational Researcher. 2006; 35 (9):3–12. doi: 10.3102/0013189X035009003. [ CrossRef ] [ Google Scholar ]
- Yin RK. Case study research: design and methods. 5. Los Angeles: SAGE; 2013. [ Google Scholar ]
- Search Menu
Sign in through your institution
- Browse content in Arts and Humanities
- Browse content in Archaeology
- Anglo-Saxon and Medieval Archaeology
- Archaeological Methodology and Techniques
- Archaeology by Region
- Archaeology of Religion
- Archaeology of Trade and Exchange
- Biblical Archaeology
- Contemporary and Public Archaeology
- Environmental Archaeology
- Historical Archaeology
- History and Theory of Archaeology
- Industrial Archaeology
- Landscape Archaeology
- Mortuary Archaeology
- Prehistoric Archaeology
- Underwater Archaeology
- Zooarchaeology
- Browse content in Architecture
- Architectural Structure and Design
- History of Architecture
- Residential and Domestic Buildings
- Theory of Architecture
- Browse content in Art
- Art Subjects and Themes
- History of Art
- Industrial and Commercial Art
- Theory of Art
- Biographical Studies
- Byzantine Studies
- Browse content in Classical Studies
- Classical History
- Classical Philosophy
- Classical Mythology
- Classical Numismatics
- Classical Literature
- Classical Reception
- Classical Art and Architecture
- Classical Oratory and Rhetoric
- Greek and Roman Epigraphy
- Greek and Roman Law
- Greek and Roman Papyrology
- Greek and Roman Archaeology
- Late Antiquity
- Religion in the Ancient World
- Social History
- Digital Humanities
- Browse content in History
- Colonialism and Imperialism
- Diplomatic History
- Environmental History
- Genealogy, Heraldry, Names, and Honours
- Genocide and Ethnic Cleansing
- Historical Geography
- History by Period
- History of Emotions
- History of Agriculture
- History of Education
- History of Gender and Sexuality
- Industrial History
- Intellectual History
- International History
- Labour History
- Legal and Constitutional History
- Local and Family History
- Maritime History
- Military History
- National Liberation and Post-Colonialism
- Oral History
- Political History
- Public History
- Regional and National History
- Revolutions and Rebellions
- Slavery and Abolition of Slavery
- Social and Cultural History
- Theory, Methods, and Historiography
- Urban History
- World History
- Browse content in Language Teaching and Learning
- Language Learning (Specific Skills)
- Language Teaching Theory and Methods
- Browse content in Linguistics
- Applied Linguistics
- Cognitive Linguistics
- Computational Linguistics
- Forensic Linguistics
- Grammar, Syntax and Morphology
- Historical and Diachronic Linguistics
- History of English
- Language Acquisition
- Language Evolution
- Language Reference
- Language Variation
- Language Families
- Lexicography
- Linguistic Anthropology
- Linguistic Theories
- Linguistic Typology
- Phonetics and Phonology
- Psycholinguistics
- Sociolinguistics
- Translation and Interpretation
- Writing Systems
- Browse content in Literature
- Bibliography
- Children's Literature Studies
- Literary Studies (European)
- Literary Studies (Eco-criticism)
- Literary Studies (Asian)
- Literary Studies (American)
- Literary Studies (Romanticism)
- Literary Studies (Modernism)
- Literary Studies - World
- Literary Studies (1500 to 1800)
- Literary Studies (19th Century)
- Literary Studies (20th Century onwards)
- Literary Studies (African American Literature)
- Literary Studies (British and Irish)
- Literary Studies (Early and Medieval)
- Literary Studies (Fiction, Novelists, and Prose Writers)
- Literary Studies (Gender Studies)
- Literary Studies (Graphic Novels)
- Literary Studies (History of the Book)
- Literary Studies (Plays and Playwrights)
- Literary Studies (Poetry and Poets)
- Literary Studies (Postcolonial Literature)
- Literary Studies (Queer Studies)
- Literary Studies (Science Fiction)
- Literary Studies (Travel Literature)
- Literary Studies (War Literature)
- Literary Studies (Women's Writing)
- Literary Theory and Cultural Studies
- Mythology and Folklore
- Shakespeare Studies and Criticism
- Browse content in Media Studies
- Browse content in Music
- Applied Music
- Dance and Music
- Ethics in Music
- Ethnomusicology
- Gender and Sexuality in Music
- Medicine and Music
- Music Cultures
- Music and Religion
- Music and Media
- Music and Culture
- Music Education and Pedagogy
- Music Theory and Analysis
- Musical Scores, Lyrics, and Libretti
- Musical Structures, Styles, and Techniques
- Musicology and Music History
- Performance Practice and Studies
- Race and Ethnicity in Music
- Sound Studies
- Browse content in Performing Arts
- Browse content in Philosophy
- Aesthetics and Philosophy of Art
- Epistemology
- Feminist Philosophy
- History of Western Philosophy
- Metaphysics
- Moral Philosophy
- Non-Western Philosophy
- Philosophy of Science
- Philosophy of Mind
- Philosophy of Perception
- Philosophy of Language
- Philosophy of Religion
- Philosophy of Action
- Philosophy of Law
- Philosophy of Mathematics and Logic
- Practical Ethics
- Social and Political Philosophy
- Browse content in Religion
- Biblical Studies
- Christianity
- East Asian Religions
- History of Religion
- Judaism and Jewish Studies
- Qumran Studies
- Religion and Education
- Religion and Health
- Religion and Politics
- Religion and Science
- Religion and Law
- Religion and Art, Literature, and Music
- Religious Studies
- Browse content in Society and Culture
- Cookery, Food, and Drink
- Cultural Studies
- Customs and Traditions
- Ethical Issues and Debates
- Hobbies, Games, Arts and Crafts
- Natural world, Country Life, and Pets
- Popular Beliefs and Controversial Knowledge
- Sports and Outdoor Recreation
- Technology and Society
- Travel and Holiday
- Visual Culture
- Browse content in Law
- Arbitration
- Browse content in Company and Commercial Law
- Commercial Law
- Company Law
- Browse content in Comparative Law
- Systems of Law
- Competition Law
- Browse content in Constitutional and Administrative Law
- Government Powers
- Judicial Review
- Local Government Law
- Military and Defence Law
- Parliamentary and Legislative Practice
- Construction Law
- Contract Law
- Browse content in Criminal Law
- Criminal Procedure
- Criminal Evidence Law
- Sentencing and Punishment
- Employment and Labour Law
- Environment and Energy Law
- Browse content in Financial Law
- Banking Law
- Insolvency Law
- History of Law
- Human Rights and Immigration
- Intellectual Property Law
- Browse content in International Law
- Private International Law and Conflict of Laws
- Public International Law
- IT and Communications Law
- Jurisprudence and Philosophy of Law
- Law and Politics
- Law and Society
- Browse content in Legal System and Practice
- Courts and Procedure
- Legal Skills and Practice
- Legal System - Costs and Funding
- Primary Sources of Law
- Regulation of Legal Profession
- Medical and Healthcare Law
- Browse content in Policing
- Criminal Investigation and Detection
- Police and Security Services
- Police Procedure and Law
- Police Regional Planning
- Browse content in Property Law
- Personal Property Law
- Restitution
- Study and Revision
- Terrorism and National Security Law
- Browse content in Trusts Law
- Wills and Probate or Succession
- Browse content in Medicine and Health
- Browse content in Allied Health Professions
- Arts Therapies
- Clinical Science
- Dietetics and Nutrition
- Occupational Therapy
- Operating Department Practice
- Physiotherapy
- Radiography
- Speech and Language Therapy
- Browse content in Anaesthetics
- General Anaesthesia
- Browse content in Clinical Medicine
- Acute Medicine
- Cardiovascular Medicine
- Clinical Genetics
- Clinical Pharmacology and Therapeutics
- Dermatology
- Endocrinology and Diabetes
- Gastroenterology
- Genito-urinary Medicine
- Geriatric Medicine
- Infectious Diseases
- Medical Toxicology
- Medical Oncology
- Pain Medicine
- Palliative Medicine
- Rehabilitation Medicine
- Respiratory Medicine and Pulmonology
- Rheumatology
- Sleep Medicine
- Sports and Exercise Medicine
- Clinical Neuroscience
- Community Medical Services
- Critical Care
- Emergency Medicine
- Forensic Medicine
- Haematology
- History of Medicine
- Browse content in Medical Dentistry
- Oral and Maxillofacial Surgery
- Paediatric Dentistry
- Restorative Dentistry and Orthodontics
- Surgical Dentistry
- Browse content in Medical Skills
- Clinical Skills
- Communication Skills
- Nursing Skills
- Surgical Skills
- Medical Ethics
- Medical Statistics and Methodology
- Browse content in Neurology
- Clinical Neurophysiology
- Neuropathology
- Nursing Studies
- Browse content in Obstetrics and Gynaecology
- Gynaecology
- Occupational Medicine
- Ophthalmology
- Otolaryngology (ENT)
- Browse content in Paediatrics
- Neonatology
- Browse content in Pathology
- Chemical Pathology
- Clinical Cytogenetics and Molecular Genetics
- Histopathology
- Medical Microbiology and Virology
- Patient Education and Information
- Browse content in Pharmacology
- Psychopharmacology
- Browse content in Popular Health
- Caring for Others
- Complementary and Alternative Medicine
- Self-help and Personal Development
- Browse content in Preclinical Medicine
- Cell Biology
- Molecular Biology and Genetics
- Reproduction, Growth and Development
- Primary Care
- Professional Development in Medicine
- Browse content in Psychiatry
- Addiction Medicine
- Child and Adolescent Psychiatry
- Forensic Psychiatry
- Learning Disabilities
- Old Age Psychiatry
- Psychotherapy
- Browse content in Public Health and Epidemiology
- Epidemiology
- Public Health
- Browse content in Radiology
- Clinical Radiology
- Interventional Radiology
- Nuclear Medicine
- Radiation Oncology
- Reproductive Medicine
- Browse content in Surgery
- Cardiothoracic Surgery
- Gastro-intestinal and Colorectal Surgery
- General Surgery
- Neurosurgery
- Paediatric Surgery
- Peri-operative Care
- Plastic and Reconstructive Surgery
- Surgical Oncology
- Transplant Surgery
- Trauma and Orthopaedic Surgery
- Vascular Surgery
- Browse content in Science and Mathematics
- Browse content in Biological Sciences
- Aquatic Biology
- Biochemistry
- Bioinformatics and Computational Biology
- Developmental Biology
- Ecology and Conservation
- Evolutionary Biology
- Genetics and Genomics
- Microbiology
- Molecular and Cell Biology
- Natural History
- Plant Sciences and Forestry
- Research Methods in Life Sciences
- Structural Biology
- Systems Biology
- Zoology and Animal Sciences
- Browse content in Chemistry
- Analytical Chemistry
- Computational Chemistry
- Crystallography
- Environmental Chemistry
- Industrial Chemistry
- Inorganic Chemistry
- Materials Chemistry
- Medicinal Chemistry
- Mineralogy and Gems
- Organic Chemistry
- Physical Chemistry
- Polymer Chemistry
- Study and Communication Skills in Chemistry
- Theoretical Chemistry
- Browse content in Computer Science
- Artificial Intelligence
- Computer Architecture and Logic Design
- Game Studies
- Human-Computer Interaction
- Mathematical Theory of Computation
- Programming Languages
- Software Engineering
- Systems Analysis and Design
- Virtual Reality
- Browse content in Computing
- Business Applications
- Computer Security
- Computer Games
- Computer Networking and Communications
- Digital Lifestyle
- Graphical and Digital Media Applications
- Operating Systems
- Browse content in Earth Sciences and Geography
- Atmospheric Sciences
- Environmental Geography
- Geology and the Lithosphere
- Maps and Map-making
- Meteorology and Climatology
- Oceanography and Hydrology
- Palaeontology
- Physical Geography and Topography
- Regional Geography
- Soil Science
- Urban Geography
- Browse content in Engineering and Technology
- Agriculture and Farming
- Biological Engineering
- Civil Engineering, Surveying, and Building
- Electronics and Communications Engineering
- Energy Technology
- Engineering (General)
- Environmental Science, Engineering, and Technology
- History of Engineering and Technology
- Mechanical Engineering and Materials
- Technology of Industrial Chemistry
- Transport Technology and Trades
- Browse content in Environmental Science
- Applied Ecology (Environmental Science)
- Conservation of the Environment (Environmental Science)
- Environmental Sustainability
- Environmentalist Thought and Ideology (Environmental Science)
- Management of Land and Natural Resources (Environmental Science)
- Natural Disasters (Environmental Science)
- Nuclear Issues (Environmental Science)
- Pollution and Threats to the Environment (Environmental Science)
- Social Impact of Environmental Issues (Environmental Science)
- History of Science and Technology
- Browse content in Materials Science
- Ceramics and Glasses
- Composite Materials
- Metals, Alloying, and Corrosion
- Nanotechnology
- Browse content in Mathematics
- Applied Mathematics
- Biomathematics and Statistics
- History of Mathematics
- Mathematical Education
- Mathematical Finance
- Mathematical Analysis
- Numerical and Computational Mathematics
- Probability and Statistics
- Pure Mathematics
- Browse content in Neuroscience
- Cognition and Behavioural Neuroscience
- Development of the Nervous System
- Disorders of the Nervous System
- History of Neuroscience
- Invertebrate Neurobiology
- Molecular and Cellular Systems
- Neuroendocrinology and Autonomic Nervous System
- Neuroscientific Techniques
- Sensory and Motor Systems
- Browse content in Physics
- Astronomy and Astrophysics
- Atomic, Molecular, and Optical Physics
- Biological and Medical Physics
- Classical Mechanics
- Computational Physics
- Condensed Matter Physics
- Electromagnetism, Optics, and Acoustics
- History of Physics
- Mathematical and Statistical Physics
- Measurement Science
- Nuclear Physics
- Particles and Fields
- Plasma Physics
- Quantum Physics
- Relativity and Gravitation
- Semiconductor and Mesoscopic Physics
- Browse content in Psychology
- Affective Sciences
- Clinical Psychology
- Cognitive Psychology
- Cognitive Neuroscience
- Criminal and Forensic Psychology
- Developmental Psychology
- Educational Psychology
- Evolutionary Psychology
- Health Psychology
- History and Systems in Psychology
- Music Psychology
- Neuropsychology
- Organizational Psychology
- Psychological Assessment and Testing
- Psychology of Human-Technology Interaction
- Psychology Professional Development and Training
- Research Methods in Psychology
- Social Psychology
- Browse content in Social Sciences
- Browse content in Anthropology
- Anthropology of Religion
- Human Evolution
- Medical Anthropology
- Physical Anthropology
- Regional Anthropology
- Social and Cultural Anthropology
- Theory and Practice of Anthropology
- Browse content in Business and Management
- Business Strategy
- Business Ethics
- Business History
- Business and Government
- Business and Technology
- Business and the Environment
- Comparative Management
- Corporate Governance
- Corporate Social Responsibility
- Entrepreneurship
- Health Management
- Human Resource Management
- Industrial and Employment Relations
- Industry Studies
- Information and Communication Technologies
- International Business
- Knowledge Management
- Management and Management Techniques
- Operations Management
- Organizational Theory and Behaviour
- Pensions and Pension Management
- Public and Nonprofit Management
- Social Issues in Business and Management
- Strategic Management
- Supply Chain Management
- Browse content in Criminology and Criminal Justice
- Criminal Justice
- Criminology
- Forms of Crime
- International and Comparative Criminology
- Youth Violence and Juvenile Justice
- Development Studies
- Browse content in Economics
- Agricultural, Environmental, and Natural Resource Economics
- Asian Economics
- Behavioural Finance
- Behavioural Economics and Neuroeconomics
- Econometrics and Mathematical Economics
- Economic Systems
- Economic History
- Economic Methodology
- Economic Development and Growth
- Financial Markets
- Financial Institutions and Services
- General Economics and Teaching
- Health, Education, and Welfare
- History of Economic Thought
- International Economics
- Labour and Demographic Economics
- Law and Economics
- Macroeconomics and Monetary Economics
- Microeconomics
- Public Economics
- Urban, Rural, and Regional Economics
- Welfare Economics
- Browse content in Education
- Adult Education and Continuous Learning
- Care and Counselling of Students
- Early Childhood and Elementary Education
- Educational Equipment and Technology
- Educational Strategies and Policy
- Higher and Further Education
- Organization and Management of Education
- Philosophy and Theory of Education
- Schools Studies
- Secondary Education
- Teaching of a Specific Subject
- Teaching of Specific Groups and Special Educational Needs
- Teaching Skills and Techniques
- Browse content in Environment
- Applied Ecology (Social Science)
- Climate Change
- Conservation of the Environment (Social Science)
- Environmentalist Thought and Ideology (Social Science)
- Management of Land and Natural Resources (Social Science)
- Natural Disasters (Environment)
- Pollution and Threats to the Environment (Social Science)
- Social Impact of Environmental Issues (Social Science)
- Sustainability
- Browse content in Human Geography
- Cultural Geography
- Economic Geography
- Political Geography
- Browse content in Interdisciplinary Studies
- Communication Studies
- Museums, Libraries, and Information Sciences
- Browse content in Politics
- African Politics
- Asian Politics
- Chinese Politics
- Comparative Politics
- Conflict Politics
- Elections and Electoral Studies
- Environmental Politics
- Ethnic Politics
- European Union
- Foreign Policy
- Gender and Politics
- Human Rights and Politics
- Indian Politics
- International Relations
- International Organization (Politics)
- Irish Politics
- Latin American Politics
- Middle Eastern Politics
- Political Methodology
- Political Communication
- Political Philosophy
- Political Sociology
- Political Behaviour
- Political Economy
- Political Institutions
- Political Theory
- Politics and Law
- Politics of Development
- Public Administration
- Public Policy
- Qualitative Political Methodology
- Quantitative Political Methodology
- Regional Political Studies
- Russian Politics
- Security Studies
- State and Local Government
- UK Politics
- US Politics
- Browse content in Regional and Area Studies
- African Studies
- Asian Studies
- East Asian Studies
- Japanese Studies
- Latin American Studies
- Middle Eastern Studies
- Native American Studies
- Scottish Studies
- Browse content in Research and Information
- Research Methods
- Browse content in Social Work
- Addictions and Substance Misuse
- Adoption and Fostering
- Care of the Elderly
- Child and Adolescent Social Work
- Couple and Family Social Work
- Direct Practice and Clinical Social Work
- Emergency Services
- Human Behaviour and the Social Environment
- International and Global Issues in Social Work
- Mental and Behavioural Health
- Social Justice and Human Rights
- Social Policy and Advocacy
- Social Work and Crime and Justice
- Social Work Macro Practice
- Social Work Practice Settings
- Social Work Research and Evidence-based Practice
- Welfare and Benefit Systems
- Browse content in Sociology
- Childhood Studies
- Community Development
- Comparative and Historical Sociology
- Disability Studies
- Economic Sociology
- Gender and Sexuality
- Gerontology and Ageing
- Health, Illness, and Medicine
- Marriage and the Family
- Migration Studies
- Occupations, Professions, and Work
- Organizations
- Population and Demography
- Race and Ethnicity
- Social Theory
- Social Movements and Social Change
- Social Research and Statistics
- Social Stratification, Inequality, and Mobility
- Sociology of Religion
- Sociology of Education
- Sport and Leisure
- Urban and Rural Studies
- Browse content in Warfare and Defence
- Defence Strategy, Planning, and Research
- Land Forces and Warfare
- Military Administration
- Military Life and Institutions
- Naval Forces and Warfare
- Other Warfare and Defence Issues
- Peace Studies and Conflict Resolution
- Weapons and Equipment

- < Previous chapter
- Next chapter >

4 “Fourth Floor”: Mixed Methods Data Analysis
- Published: September 2015
- Cite Icon Cite
- Permissions Icon Permissions
This chapter covers the final three steps of the nine-step process for conducting mixed methods research in social work. It begins by describing how to prepare qualitative and quantitative data for analysis, then provides some helpful techniques for efficiently coding and analyzing qualitative data. A basic description of how to analyze quantitative data is provided, as well as how it can be used to provide descriptive statistics (such as crosstabs, frequencies, and distributions), measures of central tendency, measures of variability, and measures of association. The chapter also briefly discusses how to maximize rigor and the relevance of analysis procedures for analyzing qualitative and quantitative data. The chapter concludes with some useful tips on the actual “mixing” of mixed methods research, the various points in time during which mixing can occur, and the ways data can be integrated and interpreted.
Personal account
- Sign in with email/username & password
- Get email alerts
- Save searches
- Purchase content
- Activate your purchase/trial code
- Add your ORCID iD
Institutional access
Sign in with a library card.
- Sign in with username/password
- Recommend to your librarian
- Institutional account management
- Get help with access
Access to content on Oxford Academic is often provided through institutional subscriptions and purchases. If you are a member of an institution with an active account, you may be able to access content in one of the following ways:
IP based access
Typically, access is provided across an institutional network to a range of IP addresses. This authentication occurs automatically, and it is not possible to sign out of an IP authenticated account.
Choose this option to get remote access when outside your institution. Shibboleth/Open Athens technology is used to provide single sign-on between your institution’s website and Oxford Academic.
- Click Sign in through your institution.
- Select your institution from the list provided, which will take you to your institution's website to sign in.
- When on the institution site, please use the credentials provided by your institution. Do not use an Oxford Academic personal account.
- Following successful sign in, you will be returned to Oxford Academic.
If your institution is not listed or you cannot sign in to your institution’s website, please contact your librarian or administrator.
Enter your library card number to sign in. If you cannot sign in, please contact your librarian.
Society Members
Society member access to a journal is achieved in one of the following ways:
Sign in through society site
Many societies offer single sign-on between the society website and Oxford Academic. If you see ‘Sign in through society site’ in the sign in pane within a journal:
- Click Sign in through society site.
- When on the society site, please use the credentials provided by that society. Do not use an Oxford Academic personal account.
If you do not have a society account or have forgotten your username or password, please contact your society.
Sign in using a personal account
Some societies use Oxford Academic personal accounts to provide access to their members. See below.
A personal account can be used to get email alerts, save searches, purchase content, and activate subscriptions.
Some societies use Oxford Academic personal accounts to provide access to their members.
Viewing your signed in accounts
Click the account icon in the top right to:
- View your signed in personal account and access account management features.
- View the institutional accounts that are providing access.
Signed in but can't access content
Oxford Academic is home to a wide variety of products. The institutional subscription may not cover the content that you are trying to access. If you believe you should have access to that content, please contact your librarian.
For librarians and administrators, your personal account also provides access to institutional account management. Here you will find options to view and activate subscriptions, manage institutional settings and access options, access usage statistics, and more.
Our books are available by subscription or purchase to libraries and institutions.
Month: | Total Views: |
---|---|
October 2022 | 5 |
November 2022 | 20 |
December 2022 | 12 |
January 2023 | 16 |
February 2023 | 16 |
March 2023 | 22 |
April 2023 | 21 |
May 2023 | 29 |
June 2023 | 13 |
July 2023 | 13 |
August 2023 | 13 |
September 2023 | 31 |
October 2023 | 21 |
November 2023 | 27 |
December 2023 | 16 |
January 2024 | 24 |
February 2024 | 18 |
March 2024 | 26 |
April 2024 | 40 |
May 2024 | 25 |
June 2024 | 17 |
July 2024 | 10 |
August 2024 | 17 |
- About Oxford Academic
- Publish journals with us
- University press partners
- What we publish
- New features
- Open access
- Rights and permissions
- Accessibility
- Advertising
- Media enquiries
- Oxford University Press
- Oxford Languages
- University of Oxford
Oxford University Press is a department of the University of Oxford. It furthers the University's objective of excellence in research, scholarship, and education by publishing worldwide
- Copyright © 2024 Oxford University Press
- Cookie settings
- Cookie policy
- Privacy policy
- Legal notice
This Feature Is Available To Subscribers Only
Sign In or Create an Account
This PDF is available to Subscribers Only
For full access to this pdf, sign in to an existing account, or purchase an annual subscription.
The Use of Mixed Methods in Research
- Reference work entry
- First Online: 13 January 2019
- Cite this reference work entry
- Kate A. McBride 2 ,
- Freya MacMillan 3 ,
- Emma S. George 4 &
- Genevieve Z. Steiner 5
2825 Accesses
10 Citations
Mixed methods research is becoming increasingly popular and is widely acknowledged as a means of achieving a more complex understanding of research problems. Combining both the in-depth, contextual views of qualitative research with the broader generalizations of larger population quantitative approaches, mixed methods research can be used to produce a rigorous and credible source of data. Using this methodology, the same core issue is investigated through the collection, analysis, and interpretation of both types of data within one study or a series of studies. Multiple designs are possible and can be guided by philosophical assumptions. Both qualitative and quantitative data can be collected simultaneously or sequentially (in any order) through a multiphase project. Integration of the two data sources then occurs with consideration is given to the weighting of both sources; these can either be equal or one can be prioritized over the other. Designed as a guide for novice mixed methods researchers, this chapter gives an overview of the historical and philosophical roots of mixed methods research. We also provide a practical overview of its application in health research as well as pragmatic considerations for those wishing to undertake mixed methods research.
This is a preview of subscription content, log in via an institution to check access.
Access this chapter
Subscribe and save.
- Get 10 units per month
- Download Article/Chapter or eBook
- 1 Unit = 1 Article or 1 Chapter
- Cancel anytime
- Available as PDF
- Read on any device
- Instant download
- Own it forever
- Available as EPUB and PDF
- Durable hardcover edition
- Dispatched in 3 to 5 business days
- Free shipping worldwide - see info
Tax calculation will be finalised at checkout
Purchases are for personal use only
Institutional subscriptions
Similar content being viewed by others
The Nature of Mixed Methods Research
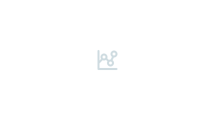
An Introduction to Qualitative and Mixed Methods Study Designs in Health Research
Appleton JV, King L. Journeying from the philosophical contemplation of constructivism to the methodological pragmatics of health services research. J Adv Nurs. 2002;40(6):641–8.
Article Google Scholar
Baba CT, Oliveira IM, Silva AEF, Vieira LM, Cerri NC, Florindo AA, de Oliveira Gomes GA. Evaluating the impact of a walking program in a disadvantaged area: using the RE-AIM framework by mixed methods. BMC Public Health. 2017;17(1):709. https://doi.org/10.1186/s12889-017-4698-5 .
Bryman A. The research question in social research: what is its role? Int J Soc Res Methodol. 2007;10(1):5–20. https://doi.org/10.1080/13645570600655282 .
Bryman A. The end of the paradigm wars. In: Alasuutari P, Bickman L, Brannen J, editors. The Sage handbook of social research methods. Thousand Oaks: Sage; 2008. p. 13–25.
Google Scholar
Caracelli VJ, Greene JC. Data-analysis strategies for mixed-method evaluation designs. Educ Eval Policy Anal. 1993;15(2):195–207. https://doi.org/10.3102/01623737015002195 .
Castro FG, Kellison JG, Boyd SJ, Kopak A. A methodology for conducting integrative mixed methods research and data analyses. J Mixed Methods Res. 2010;4(4):342–60. https://doi.org/10.1177/1558689810382916 .
Center for Innovation in Teaching in Research. Choosing a mixed methods design. 2017. Retrieved 15 Nov 2017 from https://cirt.gcu.edu/research/developmentresources/research_ready/mixed_methods/choosing_design .
Creswell JW, Plano Clark VL. Designing and conducting mixed methods research. 3rd ed. Thousand Oaks: Sage; 2018.
Curry LA, Krumholz HM, O’Cathain A, Clark VLP, Cherlin E, Bradley EH. Mixed methods in biomedical and health services research. Circ-Cardiovasc Qual Outcomes. 2013;6(1):119–23. https://doi.org/10.1161/circoutcomes.112.967885 .
Denscombe M. Communities of practice: a research paradigm for the mixed methods approach. J Mixed Methods Res. 2008;2(3):270–83.
Doyle L, Brady A-M, Byrne G. An overview of mixed methods research. J Res Nurs. 2009;14(2):175–85.
Foster NE, Bishop A, Bartlam B, Ogollah R, Barlas P, Holden M, … Young J. Evaluating acupuncture and standard carE for pregnant women with back pain (EASE back): a feasibility study and pilot randomised trial. Health Technol Assess. 2016;20(33):1–236. https://doi.org/10.3310/hta20330 .
Haider AH, Schneider EB, Kodadek LM, Adler RR, Ranjit A, Torain M, … Lau BD. Emergency department query for patient-centered approaches to sexual orientation and gender identity the EQUALITY study. JAMA Intern Med. 2017;177(6):819–828. https://doi.org/10.1001/jamainternmed.2017.0906 .
Harding KE, Taylor NF, Bowers B, Stafford M, Leggat SG. Clinician and patient perspectives of a new model of triage in a community rehabilitation program that reduced waiting time: a qualitative analysis. Aust Health Rev. 2013;37(3):324–30. https://doi.org/10.1071/ah13033 .
Hoddinott P, Britten J, Prescott GJ, Tappin D, Ludbrook A, Godden DJ. Effectiveness of policy to provide breastfeeding groups ( BIG) for pregnant and breastfeeding mothers in primary care: cluster randomised controlled trial. Br Med J. 2009;338:a3026, 10. https://doi.org/10.1136/bmj.a3026 .
Ivankova NV, Creswell JW, Stick SL. Using mixed-methods sequential explanatory design: from theory to practice. Field Methods. 2006;18(1):3–20. https://doi.org/10.1177/1525822x05282260 .
Johnson B, Gray R. A history of philosophical and theoretical issues for mixed methods research. In: Tashakkori A, Teddlie C, editors. Sage handbook of mixed methods in social and behavioral research. 2nd ed. Thousand Oaks: Sage; 2010. p. 69–94.
Chapter Google Scholar
Johnson BR, Onwuegbuzie AJ. Mixed methods research: a research paradigm whose time has come. Educ Res. 2004;33(7):14–26. https://doi.org/10.3102/0013189x033007014 .
Keeney S, McKenna H, Fleming P, McIlfatrick S. Attitudes to cancer and cancer prevention: what do people aged 35–54 years think? Eur J Cancer Care. 2010;19(6):769–77. https://doi.org/10.1111/j.1365-2354.2009.01137.x .
McBride KA, Ballinger ML, Schlub TE, Young MA, Tattersall MHN, Kirk J, et al. Psychosocial morbidity in TP53 mutation carriers: is whole-body cancer screening beneficial? Familial Cancer. 2017;16(3):423–32. https://doi.org/10.1007/s10689-016-9964-7 .
Mertens DM. Transformative mixed methods: addressing inequities. Am Behav Sci. 2012;56(6):802–13. https://doi.org/10.1177/0002764211433797 .
Moffatt S, White M, Mackintosh J, Howel D. Using quantitative and qualitative data in health services research – what happens when mixed method findings conflict? BMC Health Serv Res. 2006;6:28. https://doi.org/10.1186/1472-6963-6-28 .
Morse JM. Approaches to qualitative-quantitative methodological triangulation. Nurs Res. 1991a;40(2):120–3.
Morse JM. Principles of mixed methods and multimethod research design. In: Tashakkori A, Teddilie C, editors. SAGE handbook of mixed methods in social and behavioral research. Thousand Oaks: Sage; 1991b.
Mutrie N, Doolin O, Fitzsimons CF, Grant PM, Granat M, Grealy M, et al. Increasing older adults’ walking through primary care: results of a pilot randomized controlled trial. Fam Pract. 2012;29(6):633–42. https://doi.org/10.1093/fampra/cms038 .
Newman I, Ridenour C, Newman C, De Marco G. A typology of research purposes and its relationship to mixed methods in social and behavioral research. Thousand Oaks: Sage; 2003. p. 167–88.
O’Cathain A, Murphy E, Nicholl J. The quality of mixed methods studies in health services research. J Health Serv Res Policy. 2008;13(2):92–8. https://doi.org/10.1258/jhsrp.2007.007074 .
Onwuegbuzie AJ, Collins KMT. A typology of mixed methods sampling designs in social science research. Qual Rep. 2007;12(2):281–316.
Plano Clark VL, Badiee M. Research questions in mixed methods research. In: Tashakkori A, Teddlie C, editors. SAGE handbook of mixed methods in social and behavioral research. 2nd ed. Thousand Oaks: Sage; 2010. p. 275–304.
Prades, J., Algara, M., Espinas, J. A., Farrus, B., Arenas, M., Reyes, V., . . . Borras, J. M. (2017). Understanding variations in the use of hypofractionated radiotherapy and its specific indications for breast cancer: a mixed-methods study. Radiother Oncol, 123(1), 22–28. doi: https://doi.org/10.1016/j.radonc.2017.01.014 .
Tariq S, Woodman J. Using mixed methods in health research. JRSM Short Rep. 2013; 4 (6):1–8. https://doi.org/10.1177/2042533313479197 .
Tashakkori A, Creswell JW. Editorial: the new era of mixed methods. J Mixed Methods Res. 2007;1(1):3–7. https://doi.org/10.1177/2345678906293042 .
Tashakkori A, Teddlie C. The past and future of mixed methods research: from data triangulation to mixed model designs. In: Tashakkori A, Teddlie C, editors. Handbook of mixed methods in social & behavioral research. Thousand Oaks: Sage; 2003. p. 671–701.
Teddlie C, Tashakkori A. Foundations of mixed methods research: integrating quantitative and qualitative approaches in the social and behavioral sciences. Thousand Oaks: Sage; 2009.
Teddlie C, Yu F. Mixed methods sampling a typology with examples. J Mixed Methods Res. 2007;1(1):77–100. https://doi.org/10.1177/2345678906292430 .
Wellard SJ, Rasmussen B, Savage S, Dunning T. Exploring staff diabetes medication knowledge and practices in regional residential care: triangulation study. J Clin Nurs. 2013;22(13–14):1933–40. https://doi.org/10.1111/jocn.12043 .
Zhang W. Mixed methods application in health intervention research: a multiple case study. Int J Mult Res Approaches. 2014;8(1):24–35. https://doi.org/10.5172/mra.2014.8.1.24 .
Download references
Author information
Authors and affiliations.
School of Medicine and Translational Health Research Institute, Western Sydney University, Sydney, NSW, Australia
Kate A. McBride
School of Science and Health and Translational Health Research Institute (THRI), Western Sydney University, Penrith, NSW, Australia
Freya MacMillan
School of Science and Health, Western Sydney University, Sydney, NSW, Australia
Emma S. George
NICM and Translational Health Research Institute (THRI), Western Sydney University, Penrith, NSW, Australia
Genevieve Z. Steiner
You can also search for this author in PubMed Google Scholar
Corresponding author
Correspondence to Kate A. McBride .
Editor information
Editors and affiliations.
School of Science and Health, Western Sydney University, Penrith, NSW, Australia
Pranee Liamputtong
Rights and permissions
Reprints and permissions
Copyright information
© 2019 Springer Nature Singapore Pte Ltd.
About this entry
Cite this entry.
McBride, K.A., MacMillan, F., George, E.S., Steiner, G.Z. (2019). The Use of Mixed Methods in Research. In: Liamputtong, P. (eds) Handbook of Research Methods in Health Social Sciences. Springer, Singapore. https://doi.org/10.1007/978-981-10-5251-4_97
Download citation
DOI : https://doi.org/10.1007/978-981-10-5251-4_97
Published : 13 January 2019
Publisher Name : Springer, Singapore
Print ISBN : 978-981-10-5250-7
Online ISBN : 978-981-10-5251-4
eBook Packages : Social Sciences Reference Module Humanities and Social Sciences Reference Module Business, Economics and Social Sciences
Share this entry
Anyone you share the following link with will be able to read this content:
Sorry, a shareable link is not currently available for this article.
Provided by the Springer Nature SharedIt content-sharing initiative
- Publish with us
Policies and ethics
- Find a journal
- Track your research
Mixed Methods Data Analysis: Qualitative Analysis and Data Transformation
- Conference: European Survey Research Association

- Universität Stuttgart
Discover the world's research
- 25+ million members
- 160+ million publication pages
- 2.3+ billion citations
- Recruit researchers
- Join for free
- Login Email Tip: Most researchers use their institutional email address as their ResearchGate login Password Forgot password? Keep me logged in Log in or Continue with Google Welcome back! Please log in. Email · Hint Tip: Most researchers use their institutional email address as their ResearchGate login Password Forgot password? Keep me logged in Log in or Continue with Google No account? Sign up
Log in using your username and password
- Search More Search for this keyword Advanced search
- Latest content
- Current issue
- Write for Us
- BMJ Journals
You are here
- Volume 20, Issue 3
- Mixed methods research: expanding the evidence base
- Article Text
- Article info
- Citation Tools
- Rapid Responses
- Article metrics

- Allison Shorten 1 ,
- Joanna Smith 2
- 1 School of Nursing , University of Alabama at Birmingham , USA
- 2 Children's Nursing, School of Healthcare , University of Leeds , UK
- Correspondence to Dr Allison Shorten, School of Nursing, University of Alabama at Birmingham, 1720 2nd Ave South, Birmingham, AL, 35294, USA; [email protected]; ashorten{at}uab.edu
https://doi.org/10.1136/eb-2017-102699
Statistics from Altmetric.com
Request permissions.
If you wish to reuse any or all of this article please use the link below which will take you to the Copyright Clearance Center’s RightsLink service. You will be able to get a quick price and instant permission to reuse the content in many different ways.
Introduction
‘Mixed methods’ is a research approach whereby researchers collect and analyse both quantitative and qualitative data within the same study. 1 2 Growth of mixed methods research in nursing and healthcare has occurred at a time of internationally increasing complexity in healthcare delivery. Mixed methods research draws on potential strengths of both qualitative and quantitative methods, 3 allowing researchers to explore diverse perspectives and uncover relationships that exist between the intricate layers of our multifaceted research questions. As providers and policy makers strive to ensure quality and safety for patients and families, researchers can use mixed methods to explore contemporary healthcare trends and practices across increasingly diverse practice settings.
What is mixed methods research?
Mixed methods research requires a purposeful mixing of methods in data collection, data analysis and interpretation of the evidence. The key word is ‘mixed’, as an essential step in the mixed methods approach is data linkage, or integration at an appropriate stage in the research process. 4 Purposeful data integration enables researchers to seek a more panoramic view of their research landscape, viewing phenomena from different viewpoints and through diverse research lenses. For example, in a randomised controlled trial (RCT) evaluating a decision aid for women making choices about birth after caesarean, quantitative data were collected to assess knowledge change, levels of decisional conflict, birth choices and outcomes. 5 Qualitative narrative data were collected to gain insight into women’s decision-making experiences and factors that influenced their choices for mode of birth. 5
In contrast, multimethod research uses a single research paradigm, either quantitative or qualitative. Data are collected and analysed using different methods within the same paradigm. 6 7 For example, in a multimethods qualitative study investigating parent–professional shared decision-making regarding diagnosis of suspected shunt malfunction in children, data collection included audio recordings of admission consultations and interviews 1 week post consultation, with interactions analysed using conversational analysis and the framework approach for the interview data. 8
What are the strengths and challenges in using mixed methods?
Selecting the right research method starts with identifying the research question and study aims. A mixed methods design is appropriate for answering research questions that neither quantitative nor qualitative methods could answer alone. 4 9–11 Mixed methods can be used to gain a better understanding of connections or contradictions between qualitative and quantitative data; they can provide opportunities for participants to have a strong voice and share their experiences across the research process, and they can facilitate different avenues of exploration that enrich the evidence and enable questions to be answered more deeply. 11 Mixed methods can facilitate greater scholarly interaction and enrich the experiences of researchers as different perspectives illuminate the issues being studied. 11
The process of mixing methods within one study, however, can add to the complexity of conducting research. It often requires more resources (time and personnel) and additional research training, as multidisciplinary research teams need to become conversant with alternative research paradigms and different approaches to sample selection, data collection, data analysis and data synthesis or integration. 11
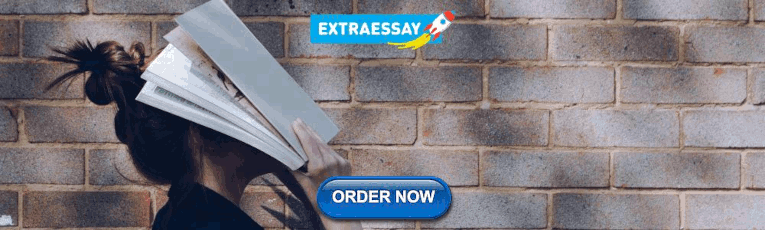
What are the different types of mixed methods designs?
Mixed methods research comprises different types of design categories, including explanatory, exploratory, parallel and nested (embedded) designs. 2 Table 1 summarises the characteristics of each design, the process used and models of connecting or integrating data. For each type of research, an example was created to illustrate how each study design might be applied to address similar but different nursing research aims within the same general nursing research area.
- View inline
Types of mixed methods designs*
What should be considered when evaluating mixed methods research?
When reading mixed methods research or writing a proposal using mixed methods to answer a research question, the six questions below are a useful guide 12 :
Does the research question justify the use of mixed methods?
Is the method sequence clearly described, logical in flow and well aligned with study aims?
Is data collection and analysis clearly described and well aligned with study aims?
Does one method dominate the other or are they equally important?
Did the use of one method limit or confound the other method?
When, how and by whom is data integration (mixing) achieved?
For more detail of the evaluation guide, refer to the McMaster University Mixed Methods Appraisal Tool. 12 The quality checklist for appraising published mixed methods research could also be used as a design checklist when planning mixed methods studies.
- Elliot AE , et al
- Creswell JW ,
- Plano ClarkV L
- Greene JC ,
- Caracelli VJ ,
- Ivankova NV
- Shorten A ,
- Shorten B ,
- Halcomb E ,
- Cheater F ,
- Bekker H , et al
- Tashakkori A ,
- Creswell JW
- 12. ↵ National Collaborating Centre for Methods and Tools . Appraising qualitative, quantitative, and mixed methods studies included in mixed studies reviews: the MMAT . Hamilton, ON : BMJ Publishing Group , 2015 . http://www.nccmt.ca/resources/search/232 (accessed May 2017) .
Competing interests None declared.
Provenance and peer review Commissioned; internally peer reviewed.
Read the full text or download the PDF:
Qualitative Quantitative Mixed Methods
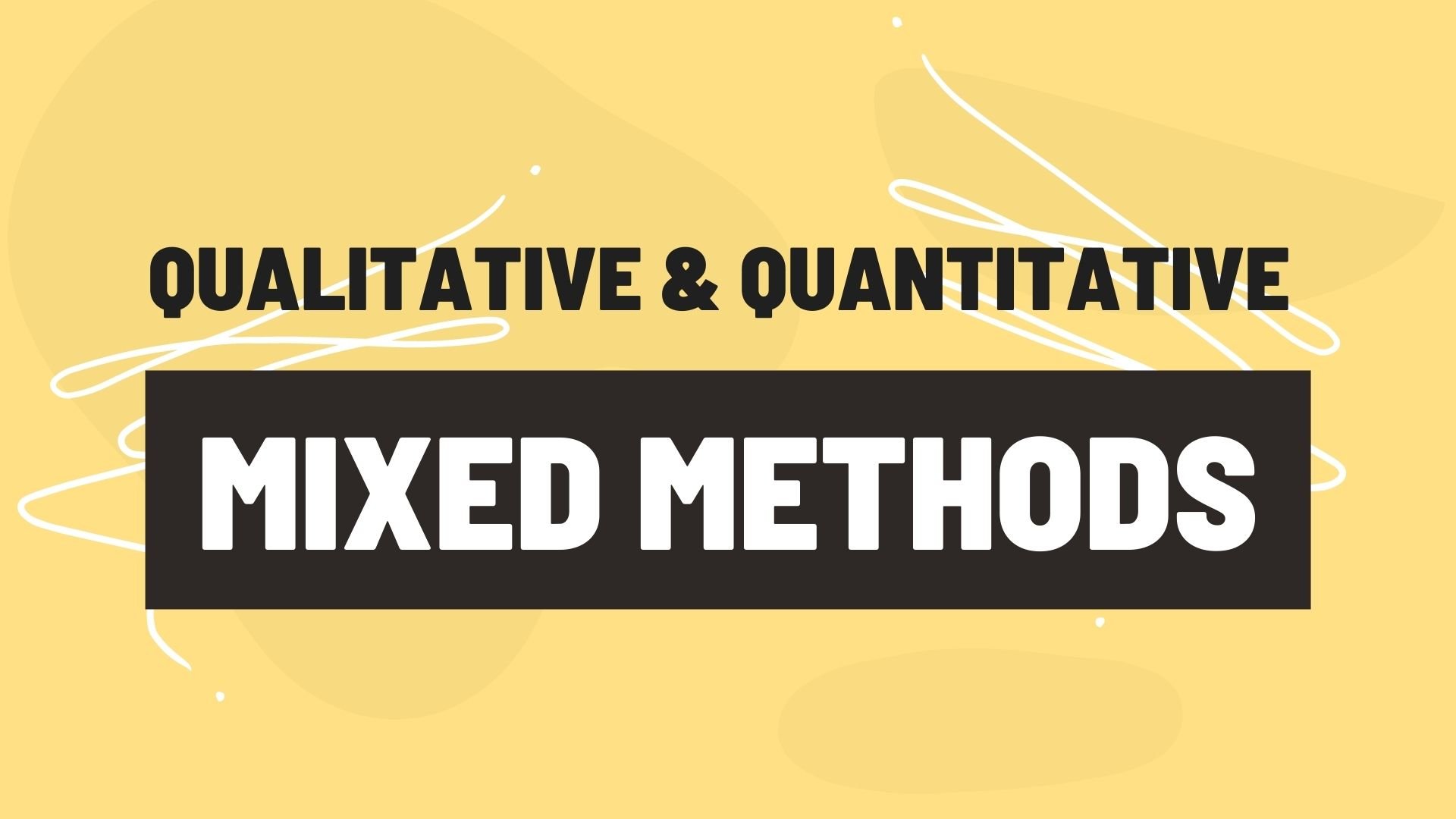
This is part of our Essential Guide to Coding Qualitative Data | Start a Free Trial of Delve | Take Our Free Online Qualitative Data Analysis Course
What is mixed methods research?
Mixed methods research is a research method that combines and integrates qualitative and quantitative research methods in a single research study. It involves collecting and analyzing qualitative and quantitative data to understand a phenomenon better and answer the research questions.
The central premise of using mixed methods research is that it makes the most of the strengths of each data type while neutralizing their weaknesses. Researchers combine qualitative and quantitative methods to expand their evidence, improve the credibility of their findings, and illustrate the results from one method with the results from the other one.
What are the core mixed methods research designs?
The three core mixed methods research designs are convergent design, explanatory sequential design, and exploratory sequential design. Let’s take a look at these three types of mixed methods research designs:
1. Convergent design
Convergent design is a type of mixed methods research in which you collect quantitative and qualitative data simultaneously and analyze them separately. After the analysis, you then combine or compare the results to draw a conclusion. Convergent design is used when there is a need to compare statistical results with qualitative findings to understand the research problem better. Researchers also use this mixed qualitative and quantitative research methods design to validate and illustrate qualitative findings with quantitative results.
2. Explanatory sequential design
Explanatory sequential design is a type of mixed methods research in which you first collect and analyze quantitative data and then follow the results up with a qualitative phase. In this approach, researchers implement the qualitative phase to explain initial quantitative results in more depth.
3. Exploratory sequential design
Exploratory sequential design is a type of mixed methods research design in which you collect and analyze qualitative data and then follow up your results with a quantitative phase. This mixed qualitative and quantitative research methods design aims to explore a phenomenon before deciding which variables you need to measure quantitatively.
What are the steps for conducting mixed methods research?
There is no clear-cut procedure for conducting a study using mixed methods. However, you can conduct mixed methods research by following the steps below:
Determine whether mixed methods research is appropriate
The first step is to determine whether mixed methods research is appropriate for answering your questions and offer the best kind of evidence you need for your research study.
Determine your purpose
Determine the purpose you want to achieve by using mixed methods research design. Is it to use the results from one data type to corroborate, expand, develop, or complement the other?
Select the most appropriate design
Select the most appropriate design out of the three types of mixed methods research designs.
Convergent design is suitable when you need both qualitative and quantitative data, have limited time to collect the data, and must gather the required data in one visit.
Explanatory design is appropriate if the research problem is quantitatively oriented and the variables and instruments required for the research are available.
Exploratory design is best when the variables are unknown and the instruments needed are not available. This is also the best mixed methods research approach to use if there is no guiding theory for the study.
Collect qualitative and quantitative data.
Collect the qualitative and quantitative data you’ll analyze. Check our our guide to qualitative methods to learn about different ways to collect qualitative data.
Analyze the collected data.
Analyze the collected data. You can use the Delve qualitative data analysis tool to quickly and rigorously analyze your qualitative data. You can then export the data into spreadsheets to analyzed it along with your quantitative data.
Write the research report
Validate and interpret the data and write your research report.
Mixed methods research examples
Here is a real world mixed methods research example to help you better understand this research approach.
In Newman, Shell, Ming, Jianping, and Mass’ paper on adolescent alcohol use , the researchers used the mixed methods research approach to understand the characteristics of adolescents who drink and don’t drink alcohol. The researchers utilized exploratory sequential design. They started by collecting and analyzing qualitative data sourced through in-depth interviews, discussions, and observations to develop a clear view of the behaviour from the participants’ perspective.
Then the researchers used the qualitative findings to design survey instruments used to collect quantitative data to explore the behavior in more detail and with a bigger sample. Using mixed methods research, the researchers gained a better understanding of this health-related behaviour and provided insights on how to reduce alcohol-related risks among young adults.
Qualitative analysis doesn't have to be overwhelming
Take delve's free online course to learn how to find themes and patterns in your qualitative data. get started here..
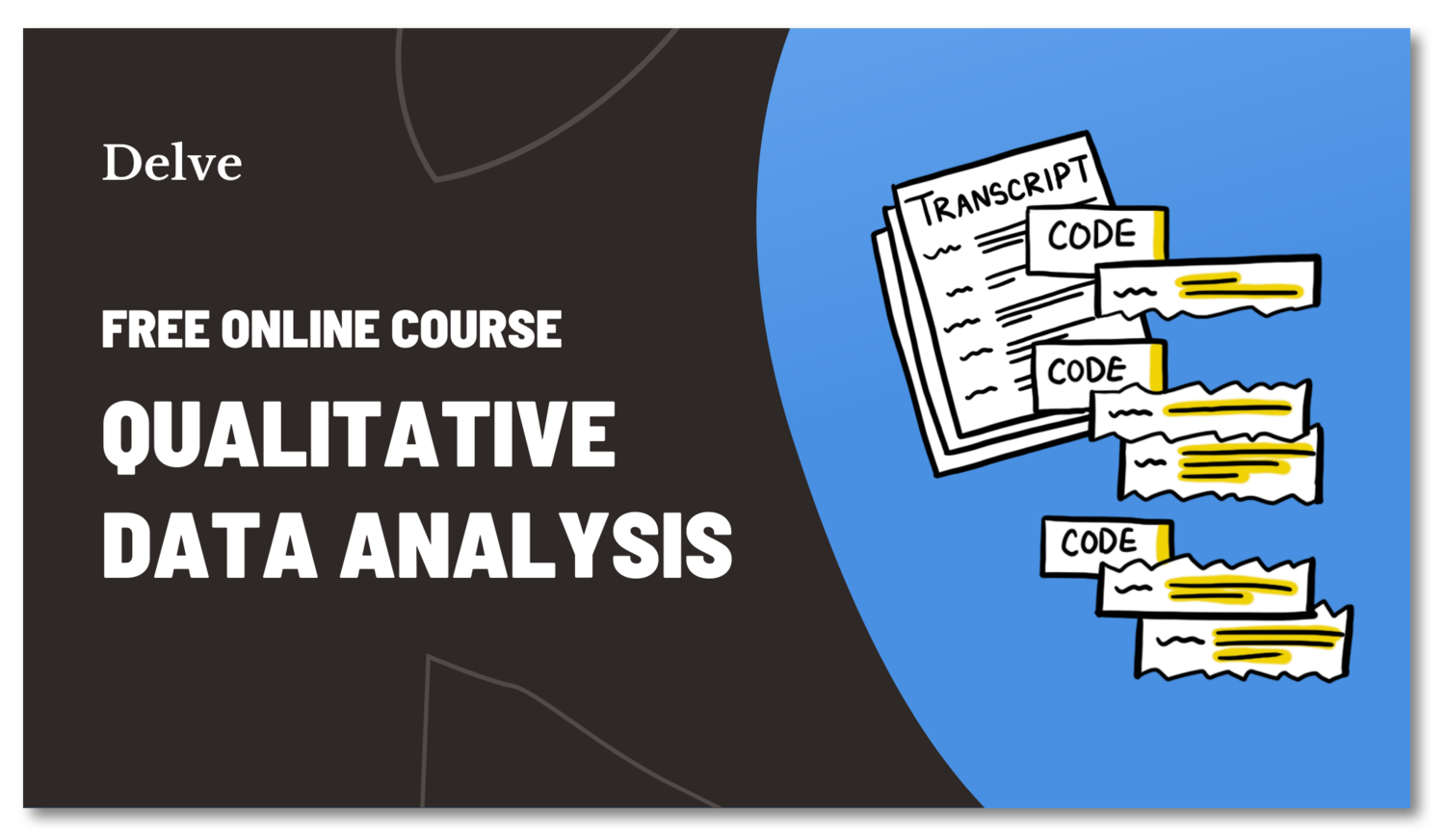
Advantages of mixed methods research
The main benefit of using mixed methods research is that you get the best of both qualitative and quantitative research methods. This approach allows you to use the strength of one data type to mitigate the weakness of the other.
Mixed qualitative and quantitative research methods are less tied to established research paradigms, so they are flexible to use.
Mixed methods research allows you to tackle a broad range of research questions. This is because you are not limited to a research method.
This approach helps researchers get stronger evidence to collaborate their findings.
Mixed methods research gives researchers a complete understanding of the topic or phenomenon under study.
Disadvantages of mixed methods research
Mixed methods research requires collecting and analyzing two types of data, so it is labor-intensive and time-consuming.
Results from mixed methods research can be difficult to interpret if you get different results from the two data types.
Mixed methods research requires you to understand multiple methods and how to combine them appropriately.
Try Delve Qualitative Analysis Tool
The Delve qualitative analysis tool can streamline the code for your qualitative data and help you to analyze data. Start a free trial of Delve today!
References:
Newman, I; Shell, D; Ming, Q; Jianping, X; Maas, M. (2006). Adolescent Alchohol Use: Mixed Methods Research Approach . Educational Psychology Papers and Publications.
Johnson, R; Schoonenboom, J. (2017). How to Construct a Mixed Methods Research Design . Kolner Z Soz Sozpsychol.
Molina-Azorin, F. (2016). Mixed methods research: An opportunity to improve our studies and our research skills . European Journal of Management and Business Economics.
Cite this blog post:
Delve, Ho, L., & Limpaecher, A. (2022a, March 23). Qualitative Quantitative Mixed Methods https://delvetool.com/blog/mixedmethods
- Open access
- Published: 02 September 2024
Clinical supervisor’s experiences of peer group clinical supervision during COVID-19: a mixed methods study
- Owen Doody ORCID: orcid.org/0000-0002-3708-1647 1 ,
- Kathleen Markey ORCID: orcid.org/0000-0002-3024-0828 1 ,
- James Turner ORCID: orcid.org/0000-0002-8360-1420 2 ,
- Claire O. Donnell ORCID: orcid.org/0000-0003-2386-7048 1 &
- Louise Murphy ORCID: orcid.org/0000-0003-2381-3963 1
BMC Nursing volume 23 , Article number: 612 ( 2024 ) Cite this article
Metrics details
Providing positive and supportive environments for nurses and midwives working in ever-changing and complex healthcare services is paramount. Clinical supervision is one approach that nurtures and supports professional guidance, ethical practice, and personal development, which impacts positively on staff morale and standards of care delivery. In the context of this study, peer group clinical supervision provides allocated time to reflect and discuss care provided and facilitated by clinical supervisors who are at the same grade/level as the supervisees.
To explore the clinical supervisor’s experiences of peer group clinical supervision a mixed methods study design was utilised within Irish health services (midwifery, intellectual disability, general, mental health). The Manchester Clinical Supervision Scale was used to survey clinical supervisors ( n = 36) and semi-structured interviews ( n = 10) with clinical supervisors were conducted. Survey data were analysed through SPSS and interview data were analysed utilising content analysis. The qualitative and quantitative data’s reporting rigour was guided by the CROSS and SRQR guidelines.
Participants generally had a positive encounter when providing clinical supervision. They highly appreciated the value of clinical supervision and expressed a considerable degree of contentment with the supervision they provided to supervisees. The advantages of peer group clinical supervision encompass aspects related to self (such as confidence, leadership, personal development, and resilience), service and organisation (including a positive working environment, employee retention, and safety), and patient care (involving critical thinking and evaluation, patient safety, adherence to quality standards, and elevated levels of care).
There are many benefits of peer group clinical supervision at an individual, service, organisation, and patient level. Nevertheless, there is a need to address a lack of awareness and misconceptions surrounding clinical supervision to create an environment and culture conducive to realising its full potential. It is crucial that clinical supervision be accessible to nurses and midwives of all grades across all healthcare services, with national planning to address capacity and sustainability.
Peer Review reports
Within a dynamic healthcare system, nurses and midwives face growing demands, underscoring the necessity for ongoing personal and professional development. This is essential to improve the effectiveness and efficiency of care delivery for patients, families, and societies. Despite the increased emphasis on increasing the quality and safety of healthcare services and delivery, there is evidence highlighting declining standards of nursing and midwifery care [ 1 ]. The recent focus on re-affirming and re-committing to core values guiding nursing and midwifery practice is encouraging such as compassion, care and commitment [ 2 ], competence, communication, and courage [ 3 ]. However, imposing value statements in isolation is unlikely to change behaviours and greater consideration needs to be given to ways in which compassion, care, and commitment are nurtured and ultimately applied in daily practice. Furthermore, concerns have been raised about global staff shortages [ 4 ], the evidence suggesting several contributing factors such as poor workforce planning [ 5 ], job dissatisfaction [ 6 ], and healthcare migration [ 7 ]. Without adequate resources and staffing, compromising standards of care and threats to patient safety will be imminent therefore the importance of developing effective strategies for retaining competent registered nurses and midwives is paramount in today’s climate of increased staff shortages [ 4 ]. Clinical supervision serves as a means to facilitate these advancements and has been linked to heightened job satisfaction, enhanced staff retention, improved staff effectiveness, and effective clinical governance, by aiding in quality improvements, risk management, and heightened accountability [ 8 ].
Clinical supervision is a key component of professional practice and while the aim is largely known, there is no universally accepted definition of clinical supervision [ 8 ]. Clinical supervision is a structured process where clinicians are allowed protected time to reflect on their practice within a supportive environment and with the purpose of developing high-quality clinical care [ 9 ]. Recent literature published on clinical supervision [ 8 , 9 , 10 , 11 , 12 , 13 , 14 , 15 , 16 ] highlights the advantages and merits of clinical supervision. However, there are challenges also identified such as a lack of consensus regarding the meaning and goal, implementation issues, variations in approaches in its operationalisation, and an absence of research evidence on its effectiveness. Duration and experience in clinical supervision link to positive benefits [ 8 ], but there is little evidence of how clinical supervision altered individual behaviours and practices. This is reinforced by Kuhne et al., [ 15 ] who emphasise that satisfaction rather than effectiveness is more commonly examined. It is crucial to emphasise that reviews have pinpointed that clinical supervision lowers the risks of adverse patient outcomes [ 9 ] and demonstrates enhancements in the execution of certain care processes. Peer group clinical supervision is a form of clinical supervision whereby two or more practitioners engage in a supervision or consultation process to improve their professional practice [ 17 ]. There is limited evidence regarding peer group clinical supervision and research on the experiences of peer clinical supervision and stakeholders is needed [ 13 ]. In Ireland, peer group clinical supervision has been recommended and guidelines have been developed [ 18 ]. In the Irish context, peer clinical supervision is where both clinical supervisees and clinical supervisors are peers at the same level/grade. However, greater evidence is required to inform future decisions on the implementation of peer group clinical supervision and the purpose of this study is to explore clinical supervisors’ experiences of peer group clinical supervision. As the focus is on peer group supervisors and utilising mixed methods the experiences of the other stakeholders were investigated and reported separately.
A mixed methods approach was used (survey and semi-structured interviews) to capture clinical supervisor’s experiences of clinical supervision. The study adhered to the Consensus-Based Checklist for Reporting of Survey Studies guidelines [ 19 ] (Supplementary File S1 ) and Standards for Reporting Qualitative Research guidelines [ 20 ] (Supplementary File S2 ).
Participants
This study was conducted with participants who successfully completed a professionally credited award: clinical supervision module run by a university in Ireland (74 clinical supervisors across 5 programmes over 3 years). The specific selection criteria for participants were that they were registered nurses/midwives delivering peer group clinical supervision within the West region of Ireland. The specific exclusion criteria were as follows: (1) nurses and midwives who haven’t finished the clinical supervision module at the University, (2) newly appointed peer group clinical supervisors who have yet to establish their groups and initiate the delivery of peer group clinical supervision.
Measures and procedures
The Manchester Clinical Supervision Scale-26 was used to survey participants in February/March 2022 and measure the peer group clinical supervisors’ overall experiences of facilitating peer group clinical supervision. The Manchester Clinical Supervision Scale-26 is a validated 26-item self-report questionnaire with a Likert-type (1–5) scale ranging from strongly disagree (1) to strongly agree (5) [ 21 ]. The Manchester Clinical Supervision Scale-26 measures the efficiency of and satisfaction with supervision, to investigate the skills acquisition aspect of clinical supervision and its effect on the quality of clinical care [ 21 ]. The instrument consists of two main sections to measure three (normative, restorative, and formative) dimensions of clinical supervision utilising six sub-scales: (1) trust and rapport, (2) supervisor advice/support, (3) improved care/skills, (4) importance/value of clinical supervision, (5) finding time, (6) personal issues/reflections and a total score for the Manchester Clinical Supervision Scale-26 is also calculated. Section two consisted of the demographic section of the questionnaire and was tailored to include eight demographic questions concerning the supervisor’s demographics, supervisee characteristics, and characteristics of clinical supervision sessions. There were also two open field questions on the Manchester Clinical Supervision Scale-26 (model of clinical supervision used and any other comments about experience of peer group clinical supervision). The main question about participants’ experiences with peer clinical supervision was “What was your experience of peer clinical supervision?” This was gathered through individual semi-structured interviews lasting between 20 and 45 min, in March/April 2022 (Supplementary file 3 ).
Ethical considerations
Health service institutional review boards of two University hospitals approved this study (Ref: 091/19 and Ref: C.A. 2199). Participants were recruited after receiving a full explanation of the study’s purpose and procedure and all relevant information. Participants were aware of potential risks and benefits and could withdraw from the study, or the survey could be stopped at any time. Informed consent was recorded, and participant identities were protected by using a pseudonym to protect anonymity.
Data analysis method
Survey data was analysed using the data analysis software package Statistical Package for the Social Sciences, version 26 (SPSS Inc., Chicago, Il, USA). Descriptive analysis was undertaken to summarise responses to all items and categorical variables (nominal and ordinal) were analysed using frequencies to detail the number and percentage of responses to each question. Scores on the Manchester Clinical Supervision Scale-26 were reverse scored for 9 items (Q1-Q6, Q8, Q20,21) and total scores for each of the six sub-scales were calculated by adding the scores for each item. Raw scores for the individual sub-scales varied in range from 0 to 20 and these raw scores were then converted to percentages which were used in addition to the raw scores for each sub-scale to describe and summarise the results of the Manchester Clinical Supervision Scale-26. Cronbach’s alpha coefficient was undertaken with the 26 questions included within the Manchester Clinical Supervision Scale-26 and more importantly with each of the dimensions in the Manchester Clinical Supervision Scale-26. The open-ended questions on the Manchester Clinical Supervision Scale-26 and interviews were analysed using content analysis guided by Colorafi and Evans [ 22 ] and categories were generated using their eight steps, (1) creating a coding framework, (2) adding codes and memos, (3) applying the first level of coding, (4) categorising codes and applying the second level of coding, (5) revising and redefining the codes, (6) adding memos, (7) visualising data and (8) representing the data.
Research rigour
To ensure the validity and rigour of this study the researchers utilised the Manchester Clinical Supervision Scale-26 a recognised clinical supervision tool with good reliability and wide usage. Interviews were recorded, transcribed, and verified by four participants, data were collected until no new components appeared, data collection methods and analysis procedures were described, and the authors’ biases were minimised throughout the research process. The Manchester Clinical Supervision Scale-26 instrument internal consistency reliability was assessed which was overall good (α = 0.878) with individual subscale also good e.g., normative domain 0.765, restorative domain 0.864, and formative domain 0.900. Reporting rigour was demonstrated using the Consensus-Based Checklist for Reporting of Survey Studies guidelines [ 19 ] and Standards for Reporting Qualitative Research guidelines [ 20 ].
Quantitative data
Participant and clinical supervision characteristics.
Thirty-six of the fifty-two (69.2%) peer group clinical supervisors working across a particular region of Ireland responded to the Manchester Clinical Supervision Scale-26 survey online via Qualtrics. Table 1 identifies the demographics of the sample who were predominantly female (94.4%) with a mean age of 44.7 years (SD. 7.63).
Peer group clinical supervision session characteristics (Table 2 ) highlight over half of peer group clinical supervisors ( n = 20, 55.6%) had been delivering peer group clinical supervision for less than one year and were mainly delivered to female supervisees ( n = 28, 77.8%). Most peer group clinical supervision sessions took place monthly ( n = 32, 88.9%) for 31–60 min ( n = 27, 75%).
Manchester Clinical Supervision Scale-26 results
Participants generally viewed peer group clinical supervision as effective (Table 3 ), the total mean Manchester Clinical Supervision Scale-26 score among all peer group clinical supervisors was 76.47 (SD. 12.801) out of 104, Surpassing the clinical supervision threshold score of 73, which was established by the developers of the Manchester Clinical Supervision Scale-26 as the benchmark indicating proficient clinical supervision provision [ 21 ]. Of the three domains; normative, formative, and restorative, the restorative domain scored the highest (mean 28.56, SD. 6.67). The mean scores compare favourably to that of the Manchester Clinical Supervision Scale-26 benchmark data and suggest that the peer group clinical supervisors were satisfied with both the level of support, encouragement, and guidance they provided and the level of trust/rapport they had developed during the peer group clinical supervision sessions. 83.3% ( n = 30) of peer group clinical supervisors reported being either very satisfied ( n = 12, 33.3%) or moderately satisfied ( n = 18, 50%) with the peer group clinical supervision they currently delivered. Within the peer group clinical supervisor’s supervisee related issues ( n = 17, 47.2%), work environment-related issues ( n = 16, 44.4%), staff-related issues ( n = 15, 41.7%) were reported as the most frequent issues, with patient/client related issues being less frequent ( n = 8, 22.2%). The most identified model used to facilitate peer group clinical supervision was the Proctors model ( n = 8, 22.22%), which was followed by group ( n = 2, 5.55%), peer ( n = 2, 5.55%), and a combination of the seven-eyed model of clinical supervision and Proctors model ( n = 1, 2.77%) with some not sure what model they used ( n = 2, 5.553%) and 58.33% ( n = 21) did not report what model they used.
Survey open-ended question
‘Please enter any additional comments , which are related to your current experience of delivering Peer Group Clinical Supervision.’ There were 22 response comments to this question, which represented 61.1% of the 36 survey respondents, which were analysed using content analysis guided by Colorafi & Evans [ 22 ]. Three categories were generated. These included: personal value/benefit of peer group clinical supervision, challenges with facilitating peer group clinical supervision, and new to peer group clinical supervision.
The first category ‘personal value/benefit of peer group clinical supervision’ highlighted positive experiences of both receiving and providing peer group clinical supervision. Peer group clinical supervisors reported that they enjoyed the sessions and found them both worthwhile and beneficial for both the group and them as peer group clinical supervisors in terms of creating a trusted supportive group environment and motivation to develop. Peer group clinical supervision was highlighted as very important for the peer group clinical supervisors working lives and they hoped that there would be more uptake from all staff. One peer group clinical supervisor expressed that external clinical supervision was a ‘lifeline’ to shaping their supervisory journey to date.
The second category ‘challenges with facilitating peer group clinical supervision’, identified time constraints, lack of buy-in/support from management, staff shortages, lack of commitment by supervisees, and COVID-19 pandemic restrictions and related sick leave, as potential barriers to facilitating peer group clinical supervision. COVID-19 was perceived to have a negative impact on peer group clinical supervision sessions due to staff shortages, which resulted in difficulties for supervisees attending the sessions during work time. Peer group clinical supervisors felt that peer group clinical supervision was not supported by management and there was limited ‘buy-in’ at times. There was also a feeling expressed that peer group clinical supervision was in its infancy, as COVID-19 and its related restrictions impacted on this by either slowing down the process of commencing peer group clinical supervision in certain areas or having to move online. However, more recently improvements in managerial support and supervisee engagement with the peer group clinical supervision process are noted.
The final category ‘new to peer group clinical supervision’ highlighted that some peer group clinical supervisors were new to the process of providing peer group clinical supervision and some felt that this survey was not a true reflection of their experience of delivering peer group clinical supervision, as they were not fully established yet as clinical supervisors due to the impact of COVID-19. Peer group clinical supervisors identified that while they were new to providing peer group clinical supervision, they were enjoying it and that it was a learning curve for them.
Qualitative data
The qualitative phase explored peer group clinical supervisors’ ( n = 10) own experiences of preparation received and experiences of being a peer group clinical supervisor. Three themes were identified through data analysis, building the foundations, enacting engagement and actions, and realities (Table 4 ).
Building the foundations
This theme highlights the importance of prior knowledge, awareness, and training but also the recruitment process and education in preparing peer group clinical supervisors.
Knowledge and awareness
Participant’s prior knowledge and awareness of peer group clinical supervision was mixed with some reporting having little or no knowledge of clinical supervision.
I’m 20 years plus trained as a nurse , and I had no awareness of clinical supervision beforehand , I really hadn’t got a clue what all of this was about , so it was a very new concept to me (Bernie) .
Others were excited about peer group clinical supervision and while they could see the need they were aware that there may be limited awareness of the value and process of clinical supervision among peers.
I find that there’s great enthusiasm and passion for clinical supervision as it’s a great support mechanism for staff in practice , however , there’s a lack of awareness of clinical supervision (Jane) .
Recruitment
Some participants highlighted that the recruitment process to become a peer group clinical supervisor was vague in some organisations with an unclear and non-transparent process evident where people were chosen by the organisation’s management rather than self-selecting interested parties.
It was just the way the training was put to the people , they were kind of nominated and told they were going and there was a lot of upset over that , so they ended up in some not going at all (Ailbhe) .
In addition, the recruitment process was seen as top loaded where senior grades of staff were chosen, and this limited staff nurse grade opportunities where there was a clear need for peer group clinical supervisors and support.
We haven’t got down to the ground level like you know we’ve done the directors , we’ve done the CNM3s the CNM2s we are at the CNM1s , so we need to get down to the staff nurse level so the nurses at the direct frontline are left out and aren’t receiving supervision because we don’t have them trained (Bernie) .
Training and education
Participants valued the training and education provided but there was a clear sense of ‘imposter syndrome’ for some peer group clinical supervisors starting out. Participants questioned their qualifications, training duration, and confidence to undertake the role of peer group clinical supervisor.
Because it is group supervision and I know that you know they say that we are qualified to do supervision and you know we’re now qualified clinical supervisors but I’m not sure that a three-month module qualifies you to be at the top of your game (Maria) .
Participants when engaged in the peer group clinical supervisor educational programme did find it beneficial and the true benefit was the actual re-engagement in education and published evidence along with the mix of nursing and midwifery practice areas.
I found it very beneficial , I mean I hadn’t been engaged in education here in a while , so it was great to be back in that field and you know with the literature that’s big (Claire) .
Enacting engagement and actions
This theme highlights the importance of forming the groups, getting a clear message out, setting the scene, and grounding the group.
Forming the groups
Recruitment for the group was of key importance to the peer group clinical supervisor and they all sent out a general invitation to form their group. Some supervisors used invitation letters or posters in addition to a general email and this was effective in recruiting supervisees.
You’re reaching out to people , I linked in with the ADoN and I put together a poster and circulated that I wasn’t ‘cherry picking , and I set up a meeting through Webex so people could get a sense of what it was if they were on the fence about it or unsure if it was for them (Karen) .
In forming the peer clinical supervision groups consideration needs to be given to the actual number of supervisees and participants reported four to six supervisees as ideal but that number can alter due to attendance.
The ideal is having five or six consistent people and that they all come on board and that you get the dynamics of the group and everything working (Claire) .
Getting a clear message out
Within the recruitment process, it was evident that there was a limited and often misguided understanding or perception of peer group clinical supervision.
Greater awareness of what actually clinical supervision is , people misjudge it as a supervision where someone is appraising you , when in fact it is more of a support mechanism , I think peer support is the key element that needs to be brought out (Jane) .
Given the lack of clarity and understanding regarding peer group clinical supervision, the participants felt strongly that further clarity is needed and that the focus needs to be on the support it offers to self, practice, and the profession.
Clinical supervision to me is clinical leadership (Jane) .
Setting the scene and grounding the group
In the initial phase of the group coming together the aspect of setting the scene and grounding the group was seen as important. A key aspect of this process was establishing the ground rules which not only set the boundaries and gave structure but also ensured the adoption of principles of trust, confidentiality, and safety.
We start with the ground rules , they give us structure it’s our contract setting out the commitment the expectation for us all , and the confidentiality as that’s so important to the trust and safety and building the relationships (Brid) .
Awareness of group dynamics is important in this process along with awareness of the group members (supervisees) as to their role and expectations.
I reiterate the role of each person in relation to confidentiality and the relationship that they would have with each other within the group and the group is very much aware that it is based on respect for each person’s point of view people may have a fear of contributing to the group and setting the ground rules is important (Jane) .
To ground the group, peer group clinical supervisors saw the importance of being present and allowing oneself to be in the room. This was evident in the time allocated at the start of each session to allow ‘grounding’ to occur in the form of techniques such as a short meditation, relaxation, or deep breathing.
At the start , I do a bit of relaxation and deep breathing , and I saw that with our own external supervisor how she settled us into place so very much about connecting with your body and you’ve arrived , then always come in with the contract in my first sentence , remember today you know we’re in a confidential space , of course , you can take away information , but the only information you will take from today is your own information and then the respect aspect (Mary Rose) .
This settling in and grounding was seen as necessary for people to feel comfortable and engage in the peer group clinical supervision process where they could focus, be open, converse, and be aware of their role and the role of peer group clinical supervision.
People have to be open, open about their practice and be willing to learn and this can only occur by sharing, clinical supervision gives us the space to do it in a space where we know we will be respected, and we can trust (Claire) .
This theme highlights the importance of the peer group clinical supervisors’ past experiences, delivering peer group clinical supervision sessions, responding to COVID-19, personal and professional development, and future opportunities.
Past experiences
Past experiences of peer group clinical supervisors were not always positive and for one participant this related to the lack of ground rules or focus of the sessions and the fact it was facilitated by a non-nurse.
In the past , I suppose I would have found it very frustrating as a participant because I just found that it was going round in circles , people moaning and you know it wasn’t very solution focused so I came from my situation where I was very frustrated with clinical supervision , it was facilitated by somebody that was non-nursing then it wasn’t very , there wasn’t the ground rules , it was very loose (Caroline) .
However, many did not have prior experience of peer group clinical supervision. Nonetheless, through the education and preparation received, there was a sense of commitment to embrace the concept, practice, and philosophy.
I did not really have any exposure or really much information on clinical supervision , but it has opened my eyes , and as one might say I am now a believer (Brid) .
Delivering peer group clinical supervision
In delivering peer group clinical supervision, participants felt supervisees were wary, as they did not know what peer group clinical supervision was, and they had focused more on the word supervision which was misleading to them. Nonetheless, the process was challenging, and buy-in was questioned at an individual and managerial level.
Buy-in wasn’t great I think now of course people will blame the pandemic , but this all happened before the pandemic , there didn’t seem to be you know , the same support from management that I would have expected so I kind of understood it in a way because then there wasn’t the same real respect from the practitioners either (Mary Rose) .
From the peer group clinical supervisor’s perspective, they were all novices in delivering/facilitating peer group clinical supervision sessions, and the support of the external clinical supervisors, and their own peer group clinical supervision sessions were invaluable along with a clinical supervision model.
Having supervision myself was key and something that is vital and needed , we all need to look at our practice and how we work it’s no good just facilitating others without being part of the process yourself but for me I would say the three principles of clinical supervision , you know the normative , formative and restorative , I keep hammering that home and bring that in regularly and revisit the contract and I have to do that often you know (Claire) .
All peer group clinical supervisors commented on the preparation for their peer group clinical supervision sessions and the importance of them having the right frame of mind and that often they needed to read over their course work and published evidence.
I want everybody to have a shared voice and you know that if one person , there is something that somebody feels very strongly and wants to talk about it that they e-mail in advance like we don’t have a set agenda but that’s agreed from the participant at the start (Caroline) .
To assist this, the peer group clinical supervisors noted the importance of their own peer group clinical supervision, the support of their peers, and external clinical supervisors. This preparation in an unpredictable situation can be difficult but drawing on one’s experience and the experience within the group can assist in navigating beyond unexpected situations.
I utilise the models of clinical supervision and this helps guide me , I am more of a facilitator of the group we are experts in our own area and our own role but you can only be an expert if you take the time to examine your practice and how you operate in your role (Brid) .
All clinical supervisors noted that the early sessions can be superficial, and the focus can be on other practice or management issues, but as time moves on and people become more engaged and involved it becomes easier as their understanding of supervision becomes clearer. In addition, there may be hesitancy and people may have difficulty opening up with certain people in the group and this is a reality that can put people off.
Initially there was so much managerial bashing and I think through supervision , I began to kind of think , I need the pillars of supervision , the governance , bringing more knowledge and it shifted everything in the room , trying to marry it with all the tensions that people have (Mary Rose) .
For some clinical supervisors, there were expected and unexpected challenges for them as clinical supervisors in terms of the discussions veering off course and expectations of their own ability.
The other big challenge is when they go off , how do you bring him back , you know when they veer off and you’re expected to be a peer , but you have to try and recoil that you have to get the balance with that right (Mary Rose) .
While peer group clinical supervision is accepted and seen as a valuable process by the peer group clinical supervisors, facilitating peer group supervision with people known to you can be difficult and may affect the process.
I’d love to supervise a group where I actually don’t know the people , I don’t know the dynamics within the group , and I’d love to see what it would be like in a group (Bernie) .
Of concern to clinical supervisors was the aspect of non-attendance and while there may be valid reasons such as COVID-19 the absence of a supervisee for several sessions can affect the group dynamics, especially if the supervisee has only engaged with early group sessions.
One of the ones that couldn’t attend because of COVID and whatever , but she’s coming to the next one and I just feel there’s a lot of issues in her area and I suppose I’m mindful that I don’t want that sort of thing to seep in , so I suppose it’s just for me just to keep reiterating the ground rules and the boundaries , that’s something I just have to manage as a facilitator , but what if they don’t attend how far will the group have progressed before she attends (Caroline) .
Responding to COVID-19
The advent of COVID-19 forced peer group clinical supervisors to find alternative means of providing peer group clinical supervision sessions which saw the move from face-to-face to online sessions. The online transition was seen as seamless for many established groups while others struggled to deliver sessions.
With COVID we did online for us it was fine because we were already formed (Corina) .
While the transition may have been positive many clinical supervisors came across issues because they were using an online format that would not be present in the face-to-face session.
We did have a session where somebody was in the main office and they have a really loud booming voice and they were saying stuff that was not appropriate to say outside of clinical supervision and I was like are you in the office can you lower it down a bit can you put your headphones on (Maria) .
However, two peer group clinical supervisors ceased or hasted the progress of rolling out peer group clinical supervision sessions mainly due to redeployment and staff availability.
With COVID it just had to be canceled here , it’s just the whole thing was canceled so it was very , very difficult for people (Mary Rose) .
It was clear from clinical supervisors that online sessions were appropriate but that they felt they were only appropriate for existing established groups that have had the opportunity to build relationships, develop trust, embed the ground rules, and create the space for open communication and once established a combined approach would be appropriate.
Since we weren’t as established as a group , not everybody knew each other it would be difficult to establish that so we would hold off/reschedule , obviously COVID is a major one but also I suppose if you have an established group now , and again , you could go to a remote one , but I felt like since we weren’t established as a group it would be difficult to develop it in that way (Karen) .
Within practice COVID-19 took priority and other aspects such as peer group clinical supervision moved lower down on the priority list for managers but not for the clinical supervisors even where redeployment occurred.
With COVID all the practical side , if one of the managers is dealing with an outbreak , they won’t be attending clinical supervision , because that has to be prioritised , whereas we’ve prioritised clinical supervision (Maria) .
The valuing of peer group clinical supervision was seen as important by clinical supervisors, and they saw it as particularly needed during COVID-19 as staff were dealing with many personal and professional issues.
During the height of COVID , we had to take a bit of a break for four months as things were so demanding at work for people but then I realised that clinical supervision was needed and started back up and they all wanted to come back (Brid) .
Having peer group clinical supervision during COVID-19 supported staff and enabled the group to form supportive relationships.
COVID has impacted over the last two years in every shape and they needed the supervision and the opportunity to have a safe supportive space and it gelled the group I think as we all were there for each other (Claire) .
While COVID-19 posed many challenges it also afforded clinical supervisors and supervisees the opportunity for change and to consider alternative means of running peer group clinical supervision sessions. This change resulted in online delivery and in reflecting on both forms of delivery (face-to-face and online) clinical supervisors saw the benefit in both. Face-to-face was seen as being needed to form the group and then the group could move online once the group was established with an occasional periodic face-to-face session to maintain motivation commitment and reinforce relationships and support.
Online formats can be effective if the group is already established or the group has gone through the storming and forming phase and the ground rules have been set and trust built , then I don’t see any problem with a blended online version of clinical supervision , and I think it will be effective (Jane) .
Personal and professional development
Growth and development were evident from peer group clinical supervisors’ experiences and this growth and development occurred at a personal, professional, and patient/client level. This development also produced an awakening and valuing of one’s passion for self and their profession.
I suppose clinical supervision is about development I can see a lot of development for me and my supervisees , you know personally and professionally , it’s the support really , clinical supervision can reinvigorate it’s very exciting and a great opportunity for nursing to support each other and in care provision (Claire) .
A key to the peer group clinical supervisor’s development was the aspect of transferable skills and the confidence they gained in fulfilling their role.
All of these skills that you learn are transferable and I am a better manager because of clinical supervision (Maria) .
The confidence and skills gained translated into the clinical supervisor’s own practice as a clinical practitioner and clinical supervisor but they were also realistic in predicting the impact on others.
I have empowered my staff , I empower them to use their voice and I give my supervisees a voice and hope they take that with them (Corina) .
Fundamental to the development process was the impact on care itself and while this cannot always be measured or identified, the clinical supervisors could see that care and support of the individual practitioner (supervisee) translated into better care for the patient/client.
Care is only as good as the person delivering it and what they know , how they function and what energy and passion they have , and clinical supervision gives the person support to begin to understand their practice and how and why they do things in a certain way and when they do that they can begin to question and even change their way of doing something (Brid) .
Future opportunities
Based on the clinical supervisor’s experiences there was a clear need identified regarding valuing and embedded peer group clinical supervision within nursing/midwifery practice.
There has to be an emphasis placed on supervision it needs to be part of the fabric of a service and valued by all in that service , we should be asking why is it not available if it’s not there but there is some work first on promoting it and people knowing what it actually is and address the misconceptions (Claire) .
While such valuing and buy-in are important, it is not to say that all staff need to have peer group clinical supervision so as to allow for personal choice. In addition, to value peer group clinical supervision it needs to be evident across all staffing grades and one could question where the best starting point is.
While we should not mandate that all staff do clinical supervision it should become embedded within practice more and I suppose really to become part of our custom and practice and be across all levels of staff (Brid) .
When peer group clinical supervision is embedded within practice then it should be custom and practice, where it is included in all staff orientations and is nationally driven.
I suppose we need to be driving it forward at the coal face at induction , at orientation and any development for the future will have to be driven by the NMPDUs or nationally (Ailbhe) .
A formalised process needs to address the release of peer group clinical supervisors but also the necessity to consider the number of peer group clinical supervisors at a particular grade.
The issue is release and the timeframe as they have a group but they also have their external supervision so you have to really work out how much time you’re talking about (Maria) .
Vital within the process of peer group clinical supervision is receiving peer group clinical supervision and peer support and this needs to underpin good peer group clinical supervision practice.
Receiving peer group supervision helps me , there are times where I would doubt myself , it’s good to have the other group that I can go to and put it out there to my own group and say , look at this , this is what we did , or this is what came up and this is how (Bernie) .
For future roll out to staff nurse/midwife grade resourcing needs to be considered as peer group clinical supervisors who were managers could see the impact of having several peer group clinical supervisors in their practice area may have on care delivery.
Facilitating groups is an issue and needs to be looked at in terms of the bigger picture because while I might be able to do a second group the question is how I would be supported and released to do so (Maria) .
While there was ambiguity regarding peer group clinical supervision there was an awareness of other disciplines availing of peer group clinical supervision, raising questions about the equality of supports available for all disciplines.
I always heard other disciplines like social workers would always have been very good saying I can’t meet you I have supervision that day and I used to think my God what’s this fabulous hour that these disciplines are getting and as a nursing staff it just wasn’t there and available (Bernie) .
To address this equity issue and the aspect of low numbers of certain grades an interdisciplinary approach within nursing and midwifery could be used or a broader interdisciplinary approach across all healthcare professionals. An interdisciplinary or across-services approach was seen as potentially fruitful.
I think the value of interprofessional or interdisciplinary learning is key it addresses problem-solving from different perspectives that mix within the group is important for cross-fertilisation and embedding the learning and developing the experience for each participant within the group (Jane) .
As we move beyond COVID-19 and into the future there is a need to actively promote peer group clinical supervision and this would clarify what peer group clinical supervision actually is, its uptake and stimulate interest.
I’d say it’s like promoting vaccinations if you could do a roadshow with people , I think that would be very beneficial , and to launch it , like you have a launch an official launch behind it (Mary Rose) .
The advantages of peer group clinical supervision highlighted in this study pertain to self-enhancement (confidence, leadership, personal development, resilience), organisational and service-related aspects (positive work environment, staff retention, safety), and professional patient care (critical thinking and evaluation, patient safety, adherence to quality standards, elevated care standards). These findings align with broader literature that acknowledges various areas, including self-confidence and facilitation [ 23 ], leadership [ 24 ], personal development [ 25 ], resilience [ 26 ], positive/supportive working environment [ 27 ], staff retention [ 28 ], sense of safety [ 29 ], critical thinking and evaluation [ 30 ], patient safety [ 31 ], quality standards [ 32 ] and increased standards of care [ 33 ].
In this study, peer group clinical supervision appeared to contribute to the alleviation of stress and anxiety. Participants recognised the significance of these sessions, where they could openly discuss and reflect on professional situations both emotionally and rationally. Central to these discussions was the creation of a safe, trustworthy, and collegial environment, aligning with evidence in the literature [ 34 ]. Clinical supervision provided a platform to share resources (information, knowledge, and skills) and address issues while offering mutual support [ 35 ]. The emergence of COVID-19 has stressed the significance of peer group clinical supervision and support for the nursing/midwifery workforce [ 36 ], highlighting the need to help nurses/midwifes preserve their well-being and participate in collaborative problem-solving. COVID-19 impacted and disrupted clinical supervision frequency, duration and access [ 37 ]. What was evident during COVID-19 was the stress and need for support for staff and given the restorative or supportive functions of clinical supervision it is a mechanism of support. However, clinical supervisors need support themselves to be able to better meet the supervisee’s needs [ 38 ].
The value of peer group clinical supervision in nurturing a conducive working environment cannot be overstated, as it indorses the understanding and adherence to workplace policies by empowering supervisees to understand the importance and rationale behind these policies [ 39 ]. This becomes vital in a continuously changing healthcare landscape, where guidelines and policies may be subject to change, especially in response to situations such as COVID-19. In an era characterised by international workforce mobility and a shortage of healthcare professionals, a supportive and positive working environment through the provision of peer group clinical supervision can positively influence staff retention [ 40 ], enhance job satisfaction [ 41 ], and mitigate burnout [ 42 ]. A critical aspect of the peer group clinical supervision process concerns providing staff the opportunity to reflect, step back, problem-solve and generate solutions. This, in turn, ensures critical thinking and evaluation within clinical supervision, focusing on understanding the issues and context, and problem-solving to draw constructive lessons for the future [ 30 ]. Research has determined a link between clinical supervision and improvements in the quality and standards of care [ 31 ]. Therefore, peer group clinical supervision plays a critical role in enhancing patient safety by nurturing improved communication among staff, facilitating reflection, promoting greater self-awareness, promoting the exchange of ideas, problem-solving, and facilitating collective learning from shared experiences.
Starting a group arose as a foundational aspect emphasised in this study. The creation of the environment through establishing ground rules, building relationships, fostering trust, displaying respect, and upholding confidentiality was evident. Vital to this process is the recruitment of clinical supervisees and deciding the suitable group size, with a specific emphasis on addressing individuals’ inclination to engage, their knowledge and understanding of peer group clinical supervision, and dissipating any lack of awareness or misconceptions regarding peer group supervision. Furthermore, the educational training of peer group clinical supervisors and the support from external clinical supervisors played a vital role in the rollout and formation of peer group clinical supervision. The evidence stresses the significance of an open and safe environment, wherein supervisees feel secure and trust their supervisor. In such an environment, they can effectively reflect on practice and related issues [ 41 ]. This study emphasises that the effectiveness of peer group supervision is more influenced by the process than the content. Clinical supervisors utilised the process to structure their sessions, fostering energy and interest to support their peers and cultivate new insights. For peer group clinical supervision to be effective, regularity is essential. Meetings should be scheduled in advance, allocate protected time, and take place in a private space [ 35 ]. While it is widely acknowledged that clinical supervisors need to be experts in their professional field to be credible, this study highlights that the crucial aspects of supervision lie in the quality of the relationship with the supervisor. The clinical supervisor should be supportive, caring, open, collaborative, sensitive, flexible, helpful, non-judgmental, and focused on tacit knowledge, experiential learning, and providing real-time feedback.
Critical to the success of peer group clinical supervision is the endorsement and support from management, considering the organisational culture and attitudes towards the practice of clinical supervision as an essential factor [ 43 ]. This support and buy-in are necessary at both the management and individual levels [ 28 ]. The primary obstacles to effective supervision often revolve around a lack of time and heavy workloads [ 44 ]. Clinical supervisors frequently struggle to find time amidst busy environments, impacting the flexibility and quality of the sessions [ 45 ]. Time constraints also limit the opportunity for reflection within clinical supervision sessions, leaving supervisees feeling compelled to resolve issues on their own without adequate support [ 45 ]. Nevertheless, time-related challenges are not unexpected, prompting a crucial question about the value placed on clinical supervision and its integration into the culture and fabric of the organisation or profession to make it a customary practice. Learning from experiences like those during the COVID-19 pandemic has introduced alternative ways of working, and the use of technology (such as Zoom, Microsoft Teams, Skype) may serve as a means to address time, resource, and travel issues associated with clinical supervision.
Despite clinical supervision having a long international history, persistent misconceptions require attention. Some of these include not considering clinical supervision a priority [ 46 ], perceiving it as a luxury [ 41 ], deeming it self-indulgent [ 47 ], or viewing it as mere casual conversation during work hours [ 48 ]. A significant challenge lies in the lack of a shared understanding regarding the role and purpose of clinical supervision, with past perceptions associating it with surveillance and being monitored [ 48 ]. These negative connotations often result in a lack of engagement [ 41 ]. Without encouragement and recognition of the importance of clinical supervision from management or the organisation, it is unlikely to become embedded in the organisational culture, impeding its normalisation [ 39 ].
In this study, some peer group clinical supervisors expressed feelings of being impostors and believed they lacked the knowledge, skills, and training to effectively fulfil their roles. While a deficiency in skills and competence are possible obstacles to providing effective clinical supervision [ 49 ], the peer group clinical supervisors in this study did not report such issues. Instead, their concerns were more about questioning their ability to function in the role of a peer group clinical supervisor, especially after a brief training program. The literature acknowledges a lack of training where clinical supervisors may feel unprepared and ill-equipped for their role [ 41 ]. To address these challenges, clinical supervisors need to be well-versed in professional guidelines and ethical standards, have clear roles, and understand the scope of practice and responsibilities associated with being a clinical supervisor [ 41 ].
The support provided by external clinical supervisors and the peer group clinical supervision sessions played a pivotal role in helping peer group clinical supervisors ease into their roles, gain experiential learning, and enhance their facilitation skills within a supportive structure. Educating clinical supervisors is an investment, but it should not be a one-time occurrence. Ongoing external clinical supervision for clinical supervisors [ 50 ] and continuous professional development [ 51 ] are crucial, as they contribute to the likelihood of clinical supervisors remaining in their roles. However, it is important to interpret the results of this study with caution due to the small sample size in the survey. Generalising the study results should be approached with care, particularly as the study was limited to two regions in Ireland. However, the addition of qualitative data in this mixed-methods study may have helped offset this limitation.
This study highlights the numerous advantages of peer group clinical supervision at individual, service, organisational, and patient/client levels. Success hinges on addressing the initial lack of awareness and misconceptions about peer group clinical supervision by creating the right environment and establishing ground rules. To unlock the full potential of peer group clinical supervision, it is imperative to secure management and organisational support for staff release. More crucially, there is a need for valuing and integrating peer group clinical supervision into nursing and midwifery education and practice. Making peer group clinical supervision accessible to all grades of nurses and midwives across various healthcare services is essential, necessitating strategic planning to tackle capacity and sustainability challenges.
Data availability
Data are available from the corresponding author upon request owing to privacy or ethical restrictions.
Zelenikova R, Gurkova E, Friganovic A, Uchmanowicz I, Jarosova D, Ziakova K, Plevova I, Papastavrou E. Unfinished nursing care in four central European countries. J Nurs Manage. 2020;28(8):1888–900. https://doi.org/10.1111/jonm.12896 .
Article Google Scholar
Department of Health, Office of the Chief Nursing Officer. Position paper 1: values for nurses and midwives in Ireland. Dublin: The Stationery Office; 2016.
Google Scholar
Cummings J, Bennett V. Developing the culture of compassionate care: creating a new vision for nurses, midwives and care-givers. London: Department of Health; 2012.
Both-Nwabuwe JM, Dijkstra MT, Klink A, Beersma B. Maldistribution or scarcity of nurses: the devil is in the detail. J Nurs Manage. 2018;26(2):86–93. https://doi.org/10.1111/jonm.12531 .
Squires A, Jylha V, Jun J, Ensio A, Kinnunen J. A scoping review of nursing workforce planning and forecasting research. J Nurs Manage. 2017;25:587–96. https://doi.org/10.1111/jonm.12510 .
Sasso L, Bagnasco A, Catania G, Zanini M, Aleo G, Watson R. Push and pull factors of nurses’ intention to leave. J Nurs Manage. 2019;27:946–54. https://doi.org/10.1111/jonm.12745 .
Gea-Caballero V, Castro-Sánchez E, Díaz‐Herrera MA, Sarabia‐Cobo C, Juárez‐Vela R, Zabaleta‐Del Olmo E. Motivations, beliefs, and expectations of Spanish nurses planning migration for economic reasons: a cross‐sectional, web‐based survey. J Nurs Scholarsh. 2019;51(2):178–86. https://doi.org/10.1111/jnu.12455 .
Article PubMed Google Scholar
Cutcliffe J, Sloan G, Bashaw M. A systematic review of clinical supervision evaluation studies in nursing. Int J Ment Health Nurs. 2018;27:1344–63. https://doi.org/10.1111/inm.12443 .
Snowdon DA, Hau R, Leggat SG, Taylor NF. Does clinical supervision of health professionals improve patient safety? A systematic review and meta-analysis. Int J Qual Health C. 2016;28(4):447–55. https://doi.org/10.1093/intqhc/mzw059 .
Turner J, Hill A. Implementing clinical supervision (part 1): a review of the literature. Ment Health Nurs. 2011;31(3):8–12.
Dilworth S, Higgins I, Parker V, Kelly B, Turner J. Finding a way forward: a literature review on the current debates around clinical supervision. Contemp Nurse. 2013;45(1):22–32. https://doi.org/10.5172/conu.2013.45.1.22 .
Buss N, Gonge H. Empirical studies of clinical supervision in psychiatric nursing: a systematic literature review and methodological critique. Int J Ment Health Nurs. 2009;18(4):250–64. https://doi.org/10.1111/j.1447-0349.2009.00612.x .
Pollock A, Campbell P, Deery R, Fleming M, Rankin J, Sloan G, Cheyne H. A systematic review of evidence relating to clinical supervision for nurses, midwives and allied health professionals. J Adv Nurs. 2017;73(8):1825–37. https://doi.org/10.1111/jan.13253 .
Snowdon DA, Leggat SG, Taylor NF. Does clinical supervision of healthcare professionals improve effectiveness of care and patient experience: a systematic review. BMC Health Serv Res. 2017;17(1):1–11. https://doi.org/10.1186/s12913-017-2739-5 .
Kühne F, Maas J, Wiesenthal S, Weck F. Empirical research in clinical supervision: a systematic review and suggestions for future studies. BMC Psychol. 2019;7(1):1–11. https://doi.org/10.1186/s40359-019-0327-7 .
Snowdon DA, Sargent M, Williams CM, Maloney S, Caspers K, Taylor NF. Effective clinical supervision of allied health professionals: a mixed methods study. BMC Health Serv Res. 2020;20(1):1–11. https://doi.org/10.1186/s12913-019-4873-8 .
Borders LD. Dyadic, triadic, and group models of peer supervision/consultation: what are their components, and is there evidence of their effectiveness? Clin Psychol. 2012;16(2):59–71.
Health Service Executive. Guidance document on peer group clinical supervision. Mayo: Nursing and Midwifery Planning and Development Unit Health Service Executive West Mid West; 2023.
Sharma A, Minh Duc NT, Lam Thang L, Nam T, Ng NH, Abbas SJ, Huy KS, Marušić NT, Paul A, Kwok CL. Karamouzian, M. A consensus-based checklist for reporting of survey studies (CROSS). J Gen Intern Med. 2021;36(10):3179–87. https://doi.org/10.1007/s11606-021-06737-1 .
Article PubMed PubMed Central Google Scholar
O’Brien BC, Harris IB, Beckman TJ, Reed DA, Cook DA. Standards for reporting qualitative research: a synthesis of recommendations. Acad Med. 2014;899:1245–51. https://doi.org/10.1097/ACM.0000000000000388 .
Winstanley J, White E. The MCSS-26©: revision of the Manchester Clinical Supervision Scale© using the Rasch Measurement Model. J Nurs Meas. 2011;193(2011):160–78. https://doi.org/10.1891/1061-3749.19.3.160 .
Colorafi KJ, Evans B. Qualitative descriptive methods in health science research. HERD-Health Env Res. 2016;9:16–25. https://doi.org/10.1177/1937586715614171 .
Agnew T, Vaught CC, Getz HG, Fortune J. Peer group clinical supervision program fosters confidence and professionalism. Prof Sch Couns. 2000;4(1):6–12.
Mc Carthy V, Goodwin J, Saab MM, Kilty C, Meehan E, Connaire S, O’Donovan A. Nurses and midwives’ experiences with peer-group clinical supervision intervention: a pilot study. J Nurs Manage. 2021;29:2523–33. https://doi.org/10.1111/jonm.13404 .
Rothwell C, Kehoe A, Farook SF, Illing J. Enablers and barriers to effective clinical supervision in the workplace: a rapid evidence review. BMJ Open. 2021;119:e052929. https://doi.org/10.1136/bmjopen-2021-052929 .
Francis A, Bulman C. In what ways might group clinical supervision affect the development of resilience in hospice nurses. Int J Palliat Nurs. 2019;25:387–96. https://doi.org/10.12968/ijpn.2019.25.8.387 .
Chircop Coleiro A, Creaner M, Timulak L. The good, the bad, and the less than ideal in clinical supervision: a qualitative meta-analysis of supervisee experiences. Couns Psychol Quart. 2023;36(2):189–210. https://doi.org/10.1080/09515070.2021.2023098 .
Stacey G, Cook G, Aubeeluck A, Stranks B, Long L, Krepa M, Lucre K. The implementation of resilience based clinical supervision to support transition to practice in newly qualified healthcare professionals. Nurs Educ Today. 2020;94:104564. https://doi.org/10.1016/j.nedt.2020.104564 .
Feerick A, Doyle L, Keogh B. Forensic mental health nurses’ perceptions of clinical supervision: a qualitative descriptive study. Issues Ment Health Nurs. 2021;42:682–9. https://doi.org/10.1080/01612840.2020.1843095 .
Corey G, Haynes RH, Moulton P, Muratori M. Clinical supervision in the helping professions: a practical guide. Alexandria, VA: American Counseling Association; 2021.
Sturman N, Parker M, Jorm C. Clinical supervision in general practice training: the interweaving of supervisor, trainee and patient entrustment with clinical oversight, patient safety and trainee learning. Adv Health Sci Educ. 2021;26:297–311. https://doi.org/10.1007/s10459-020-09986-7 .
Alfonsson S, Parling T, Spännargård Å, Andersson G, Lundgren T. The effects of clinical supervision on supervisees and patients in cognitive behavioral therapy: a systematic review. Cogn Behav Therapy. 2018;47(3):206–28. https://doi.org/10.1080/16506073.2017.1369559 .
Coelho M, Esteves I, Mota M, Pestana-Santos M, Santos MR, Pires R. Clinical supervision of the nurse in the community to promote quality of care provided by the caregiver: scoping review protocol. Millenium J Educ Technol Health. 2022;2:83–9. https://doi.org/10.29352/mill0218.26656 .
Toros K, Falch-Eriksen A. Structured peer group supervision: systematic case reflection for constructing new perspectives and solutions. Int Soc Work. 2022;65:1160–5. https://doi.org/10.1177/0020872820969774 .
Bifarin O, Stonehouse D. Clinical supervision: an important part of every nurse’s practice. Brit J Nurs. 2017;26(6):331–5. https://doi.org/10.12968/bjon.2017.26.6.331 .
Turner J, Simbani N, Doody O, Wagstaff C, McCarthy-Grunwald S. Clinical supervision in difficult times and at all times. Ment Health Nurs. 2022;42(1):10–3.
Martin P, Tian E, Kumar S, Lizarondo L. A rapid review of the impact of COVID-19 on clinical supervision practices of healthcare workers and students in healthcare settings. J Adv Nurs. 2022;78:3531–9. https://doi.org/10.1111/jan.15360 .
van Dam M, van Hamersvelt H, Schoonhoven L, Hoff RG, Cate OT, Marije P. Hennus. Clinical supervision under pressure: a qualitative study amongst health care professionals working on the ICU during COVID-19. Med Edu Online. 2023;28:1. https://doi.org/10.1080/10872981.2023.2231614 .
Martin P, Lizarondo L, Kumar S, Snowdon D. Impact of clinical supervision on healthcare organisational outcomes: a mixed methods systematic review. PLoS ONE. 2021;1611:e0260156. https://doi.org/10.1371/journal.pone.0260156 .
Article CAS Google Scholar
Hussein R, Salamonson Y, Hu W, Everett B. Clinical supervision and ward orientation predict new graduate nurses’ intention to work in critical care: findings from a prospective observational study. Aust Crit Care. 2019;325:397–402. https://doi.org/10.1016/j.aucc.2018.09.003 .
Love B, Sidebotham M, Fenwick J, Harvey S, Fairbrother G. Unscrambling what’s in your head: a mixed method evaluation of clinical supervision for midwives. Women Birth. 2017;30:271–81. https://doi.org/10.1016/j.wombi.2016.11.002 .
Berry S, Robertson N. Burnout within forensic psychiatric nursing: its relationship with ward environment and effective clinical supervision? J Psychiatr Ment Health Nurs. 2019;26:7–8. https://doi.org/10.1111/jpm.12538 .
Markey K, Murphy L, O’Donnell C, Turner J, Doody O. Clinical supervision: a panacea for missed care. J Nurs Manage. 2020;28:2113–7. https://doi.org/10.1111/jonm.13001 .
Brody AA, Edelman L, Siegel EO, Foster V, Bailey DE Jr., Bryant AL, Bond SM. Evaluation of a peer mentoring program for early career gerontological nursing faculty and its potential for application to other fields in nursing and health sciences. Nurs Outlook. 2016;64(4):332–8. https://doi.org/10.1016/j.outlook.2016.03.004 .
Bulman C, Forde-Johnson C, Griffiths A, Hallworth S, Kerry A, Khan S, Mills K, Sharp P. The development of peer reflective supervision amongst nurse educator colleagues: an action research project. Nurs Educ Today. 2016;45:148–55. https://doi.org/10.1016/j.nedt.2016.07.010 .
Pack M. Unsticking the stuckness’: a qualitative study of the clinical supervisory needs of early-career health social workers. Brit J Soc Work. 2015;45:1821–36. https://doi.org/10.1093/bjsw/bcu069 .
Bayliss J. Clinical supervision for palliative care. London: Quay Books; 2006.
Kenny A, Allenby A. Implementing clinical supervision for Australian rural nurses. Nurs Educ Pract. 2013;13(3):165–9. https://doi.org/10.1016/j.nepr.2012.08.009 .
MacLaren J, Stenhouse R, Ritchie D. Mental health nurses’ experiences of managing work-related emotions through supervision. J Adv Nurs. 2016;72:2423–34. https://doi.org/10.1111/jan.12995 .
Wilson HM, Davies JS, Weatherhead S. Trainee therapists’ experiences of supervision during training: a meta-synthesis. Clinl Psychol Psychother. 2016;23:340–51. https://doi.org/10.1002/cpp.1957 .
Noelker LS, Ejaz FK, Menne HL, Bagaka’s JG. Factors affecting frontline workers’ satisfaction with supervision. J Aging Health. 2009;21(1):85–101. https://doi.org/10.1177/0898264308328641 .
Download references
Acknowledgements
The research team would like to thank all participants for their collaboration, the HSE steering group members and Carmel Hoey, NMPDU Director, HSE West Mid West, Dr Patrick Glackin, NMPD Area Director, HSE West, Annette Cuddy, Director, Centre of Nurse and Midwifery Education Mayo/Roscommon; Ms Ruth Hoban, Assistant Director of Nursing and Midwifery (Prescribing), HSE West; Ms Annette Connolly, NMPD Officer, NMPDU HSE West Mid West.
The authors declare that there are no sources of funding associated with this paper.
Author information
Authors and affiliations.
Department of Nursing and Midwifery, Health Research Institute, University of Limerick, Limerick, Ireland
Owen Doody, Kathleen Markey, Claire O. Donnell & Louise Murphy
Department of Nursing and Midwifery, Sheffield Hallam University, Sheffield, UK
James Turner
You can also search for this author in PubMed Google Scholar
Contributions
OD: Conceptualization, Methodology, Formal analysis, Investigation, Writing - Original Draft, Writing - Review & Editing, Project administration, Funding acquisition. COD: Methodology, Formal analysis, Investigation, Writing - Original Draft, Writing - Review & Editing, Funding acquisition. KM: Methodology, Formal analysis, Investigation, Writing - Original Draft, Writing - Review & Editing, Funding acquisition. JT: Methodology, Formal analysis, Writing - Original Draft, Writing - Review & Editing. LM: Methodology, Formal analysis, Investigation, Writing - Original Draft, Writing - Review & Editing, Funding acquisition.
Corresponding author
Correspondence to Owen Doody .
Ethics declarations
Ethics approval and consent to participate.
This study was approved by two health service institutional review boards University Hospital Limerick (Ref: 091/19) and Galway University Hospitals (Ref: C.A. 2199). The study was conducted in accordance with the principles of the Declaration of Helsinki and all study details were fully disclosed to participants, who were assured of the voluntary nature of participation and withdrawal. The study questionnaires were coded, and identities were not disclosed to guarantee participants’ anonymity and all participants provided written informed consent before interviews.
Consent for publication
No identifying images or other personal or clinical details of participants are presented in this paper that would compromise anonymity and all participants were aware and informed through the Participant Information Leaflet that the data collected may be reported through article and/or conference publications and this was reiterated during the qualitative data collection stage.
Competing interests
The authors declare no competing interests.
Additional information
Publisher’s note.
Springer Nature remains neutral with regard to jurisdictional claims in published maps and institutional affiliations.
Electronic supplementary material
Below is the link to the electronic supplementary material.
Supplementary Material 1
Supplementary material 2, supplementary material 3, rights and permissions.
Open Access This article is licensed under a Creative Commons Attribution-NonCommercial-NoDerivatives 4.0 International License, which permits any non-commercial use, sharing, distribution and reproduction in any medium or format, as long as you give appropriate credit to the original author(s) and the source, provide a link to the Creative Commons licence, and indicate if you modified the licensed material. You do not have permission under this licence to share adapted material derived from this article or parts of it. The images or other third party material in this article are included in the article’s Creative Commons licence, unless indicated otherwise in a credit line to the material. If material is not included in the article’s Creative Commons licence and your intended use is not permitted by statutory regulation or exceeds the permitted use, you will need to obtain permission directly from the copyright holder. To view a copy of this licence, visit http://creativecommons.org/licenses/by-nc-nd/4.0/ .
Reprints and permissions
About this article
Cite this article.
Doody, O., Markey, K., Turner, J. et al. Clinical supervisor’s experiences of peer group clinical supervision during COVID-19: a mixed methods study. BMC Nurs 23 , 612 (2024). https://doi.org/10.1186/s12912-024-02283-3
Download citation
Received : 04 January 2024
Accepted : 22 August 2024
Published : 02 September 2024
DOI : https://doi.org/10.1186/s12912-024-02283-3
Share this article
Anyone you share the following link with will be able to read this content:
Sorry, a shareable link is not currently available for this article.
Provided by the Springer Nature SharedIt content-sharing initiative
- Clinical supervision
BMC Nursing
ISSN: 1472-6955
- General enquiries: [email protected]
Thank you for visiting nature.com. You are using a browser version with limited support for CSS. To obtain the best experience, we recommend you use a more up to date browser (or turn off compatibility mode in Internet Explorer). In the meantime, to ensure continued support, we are displaying the site without styles and JavaScript.
- View all journals
- Explore content
- About the journal
- Publish with us
- Sign up for alerts
- Open access
- Published: 01 September 2024
Robust identification of perturbed cell types in single-cell RNA-seq data
- Phillip B. Nicol 1 ,
- Danielle Paulson 1 ,
- Gege Qian 2 ,
- X. Shirley Liu ORCID: orcid.org/0000-0003-4736-7339 3 ,
- Rafael Irizarry ORCID: orcid.org/0000-0002-3944-4309 3 &
- Avinash D. Sahu ORCID: orcid.org/0000-0002-2193-6276 4
Nature Communications volume 15 , Article number: 7610 ( 2024 ) Cite this article
2 Altmetric
Metrics details
- Bioinformatics
- Computational models
- Data mining
- Statistical methods
- Transcriptomics
Single-cell transcriptomics has emerged as a powerful tool for understanding how different cells contribute to disease progression by identifying cell types that change across diseases or conditions. However, detecting changing cell types is challenging due to individual-to-individual and cohort-to-cohort variability and naive approaches based on current computational tools lead to false positive findings. To address this, we propose a computational tool, scDist , based on a mixed-effects model that provides a statistically rigorous and computationally efficient approach for detecting transcriptomic differences. By accurately recapitulating known immune cell relationships and mitigating false positives induced by individual and cohort variation, we demonstrate that scDist outperforms current methods in both simulated and real datasets, even with limited sample sizes. Through the analysis of COVID-19 and immunotherapy datasets, scDist uncovers transcriptomic perturbations in dendritic cells, plasmacytoid dendritic cells, and FCER1G+NK cells, that provide new insights into disease mechanisms and treatment responses. As single-cell datasets continue to expand, our faster and statistically rigorous method offers a robust and versatile tool for a wide range of research and clinical applications, enabling the investigation of cellular perturbations with implications for human health and disease.
Similar content being viewed by others
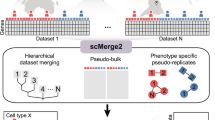
Atlas-scale single-cell multi-sample multi-condition data integration using scMerge2
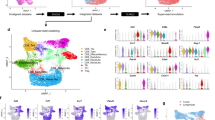
Interpretation of T cell states from single-cell transcriptomics data using reference atlases
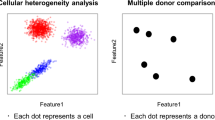
Data-driven comparison of multiple high-dimensional single-cell expression profiles
Introduction.
The advent of single-cell technologies has enabled measuring transcriptomic profiles at single-cell resolution, paving the way for the identification of subsets of cells with transcriptomic profiles that differ across conditions. These cutting-edge technologies empower researchers and clinicians to study human cell types impacted by drug treatments, infections like SARS-CoV-2, or diseases like cancer. To conduct such studies, scientists must compare single-cell RNA-seq (scRNA-seq) data between two or more groups or conditions, such as infected versus non-infected 1 , responders versus non-responders to treatment 2 , or treatment versus control in controlled experiments.
Two related but distinct classes of approaches exist for comparing conditions in single-cell data: differential abundance prediction and differential state analysis 3 . Differential abundance approaches, such as DA-seq, Milo, and Meld 4 , 5 , 6 , 7 , focus on identifying cell types with varying proportions between conditions. In contrast, differential state analysis seeks to detect predefined cell types with distinct transcriptomic profiles between conditions. In this study, we focus on the problem of differential state analysis.
Past differential state studies have relied on manual approaches involving visually inspecting data summaries to detect differences in scRNA data. Specifically, cells were clustered based on gene expression data and visualized using uniform manifold approximation (UMAP) 8 . Cell types that appeared separated between the two conditions were identified as different 1 . Another common approach is to use the number of differentially expressed genes (DEGs) as a metric for transcriptomic perturbation. However, as noted by ref. 9 , the number of DEGs depends on the chosen significance level and can be confounded by the number of cells per cell type because this influences the power of the corresponding statistical test. Additionally, this approach does not distinguish between genes with large and small (yet significant) effect sizes.
To overcome these limitations, Augur 9 uses a machine learning approach to quantify the cell-type specific separation between the two conditions. Specifically, Augur trains a classifier to predict condition labels from the expression data and then uses the area under the receiver operating characteristic (AUC) as a metric to rank cell types by their condition difference. However, Augur does not account for individual-to-individual variability (or pseudoreplication 10 ), which we show can confound the rankings of perturbed cell types.
In this study, we develop a statistical approach that quantifies transcriptomic shifts by estimating the distance (in gene expression space) between the condition means. This method, which we call scDist , introduces an interpretable metric for comparing different cell types while accounting for individual-to-individual and technical variability in scRNA-seq data using linear mixed-effect models. Furthermore, because transcriptomic profiles are high-dimensional, we develop an approximation for the between-group differences, based on a low-dimensional embedding, which results in a computationally convenient implementation that is substantially faster than Augur . We demonstrate the benefits using a COVID-19 dataset, showing that scDist can recover biologically relevant between-group differences while also controlling for sample-level variability. Furthermore, we demonstrated the utility of the scDist by jointly inferring information from five single-cell immunotherapy cohorts, revealing significant differences in a subpopulation of NK cells between immunotherapy responders and non-responders, which we validated in bulk transcriptomes from 789 patients. These results highlight the importance of accounting for individual-to-individual and technical variability for robust inference from single-cell data.
Not accounting for individual-to-individual variability leads to false positives
We used blood scRNA-seq from six healthy controls 1 (see Table 1 ), and randomly divided them into two groups of three, generating a negative control dataset in which no cell type should be detected as being different. We then applied Augur to these data. This procedure was repeated 20 times. Augur falsely identified several cell types as perturbed (Fig. 1 A). Augur quantifies differences between conditions with an AUC summary statistic, related to the amount of transcriptional separation between the two groups (AUC = 0.5 represents no difference). Across the 20 negative control repeats, 93% of the AUCs (across all cell typess) were >0.5, and red blood cells (RBCs) were identified as perturbed in all 20 trials (Fig. 1 A). This false positive result was in part due to high across-individual variability in cell types such as RBCs (Fig. 1 B).
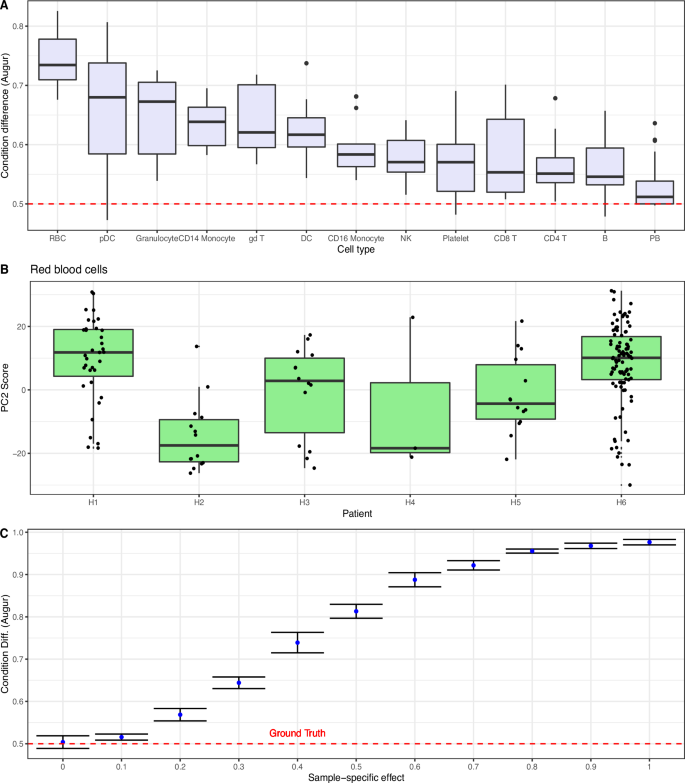
A AUCs achieved by Augur on 20 random partitions of healthy controls ( n = 6 total patients divided randomly into two groups of 3), with no expected cell type differences (dashed red line indicates the null value of 0.5). B Boxplot depicting the second PC score for red blood cells from healthy individuals, highlighting high across-individual variability (each box represents a different individual). The boxplots display the median and first/third quartiles. C AUCs achieved by Augur on simulated scRNA-seq data (10 individuals, 50 cells per individual) with no condition differences but varying patient-level variability (dashed red line indicates the ground truth value of no condition difference, AUC 0.5), illustrating the influence of individual-to-individual variability on false positive predictions. Points and error bands represent the mean ±1 SD. Source data are provided as a Source Data file.
We confirmed that individual-to-individual variation underlies false positive predictions made by Augur using a simulation. We generated simulated scRNA-seq data with no condition-level difference and varying patient-level variability (Methods). As patient-level variability increased, differences estimated by Augur also increased, converging to the maximum possible AUC of 1 (Fig. 1 C): Augur falsely interpreted individual-to-individual variability as differences between conditions.
Augur recommends that unwanted variability should be removed in a pre-processing step using batch correction software. We applied Harmony 11 to the same dataset 1 , treating each patient as a batch. We then applied Augur to the resulting batch corrected PC scores and found that several cell types still had AUCs significantly above the null value of 0.5 (Fig. S1 a). On simulated data, batch correction as a pre-processing step also leads to confounding individual-to-individual variability as condition difference (Fig. S1 b).
A model-based distance metric controls for false positives
To account for individual-to-individual variability, we modeled the vector of normalized counts with a linear mixed-effects model. Mixed models have previously been shown to be successful at adjusting for this source of variability 10 . Specifically, for a given cell type, let z i j be a length G vector of normalized counts for cell i and sample j ( G is the number of genes). We then model
where α is a vector with entries α g representing the baseline expression for gene g , x j is a binary indicator that is 0 if individual j is in the reference condition, and 1 if in the alternative condition, β is a vector with entries β g representing the difference between condition means for gene g , ω j is a random effect that represents the differences between individuals, and ε i j is a random vector (of length G ) that accounts for other sources of variability. We assume that \({{{\boldsymbol{\omega }}}}_{j}\mathop{ \sim }\limits^{{{\rm{i}}}.{{\rm{i}}}.{{\rm{d}}}}{{\mathcal{N}}}(0,{\tau }^{2}I)\) , \({{{\boldsymbol{\varepsilon }}}}_{ij}\mathop{ \sim }\limits^{{{\rm{i}}}.{{\rm{i}}}.{{\rm{d}}}}{{\mathcal{N}}}(0,{\sigma }^{2}I)\) , and that the ω j and ε i j are independent of each other.
To obtain normalized counts, we recommend defining z i j to be the vector of Pearson residuals obtained from fitting a Poisson or negative binomial GLM 12 , the normalization procedure is implemented in the scTransform function 13 . However, our proposed approach can be used with other normalization methods for which the model is appropriate.
Note that in model ( 18 ), the means for the two conditions are α and α + β , respectively. Therefore, we quantify the difference in expression profile by taking the 2 − norm of the vector β :
Here, D can be interpreted as the Euclidean distance between condition means (Fig. 2 A).
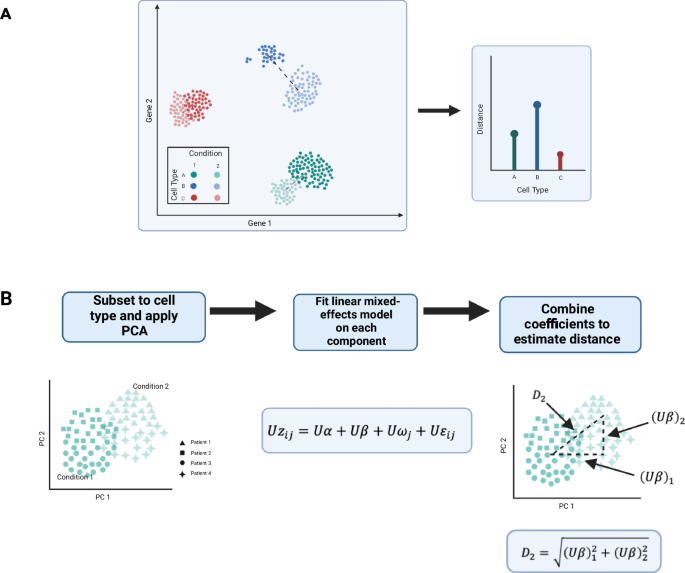
A scDist estimates the distance between condition means in high-dimensional gene expression space for each cell type. B To improve efficiency, scDist calculates the distance in a low-dimensional embedding space (derived from PCA) and employs a linear mixed-effects model to account for sample-level and other technical variability. This figure is created with Biorender.com, was released under a Creative Commons Attribution-NonCommercial-NoDerivs 4.0 International license.
Because we expected the vector of condition differences β to be sparse, we improved computational efficiency by approximating D with a singular value decomposition to find a K × G matrix U , with K much smaller than G , and
With this approximation in place, we fitted model equation ( 18 ) by replacing z i j with U z i j to obtain estimates of ( U β ) k . A challenge with estimating D K is that the maximum likelihood estimator can have a significant upward bias when the number of patients is small (as is typically the case). For this reason, we employed a post-hoc Bayesian procedure to shrink \({{(U{\boldsymbol{\beta}} )}_{k}^{2}}\) towards zero and compute a posterior distribution of D K 14 . We also provided a statistical test for the null hypothesis that D K = 0. We refer to the resulting procedure as scDist (Fig. 2 B). Technical details are provided in Methods.
We applied scDist to the negative control dataset based on blood scRNA-seq from six healthy used to show the large number of false positives reported by Augur (Fig. 1 ) and found that the false positive rate was controlled (Fig. 3 A, B). We then applied scDist to the data from the simulation study and found that, unlike Augur , the resulting distance estimate does not grow with individual-to-individual variability (Fig. 3 C). scDist also accurately estimated distances on fully simulated data (Fig. S2 ).
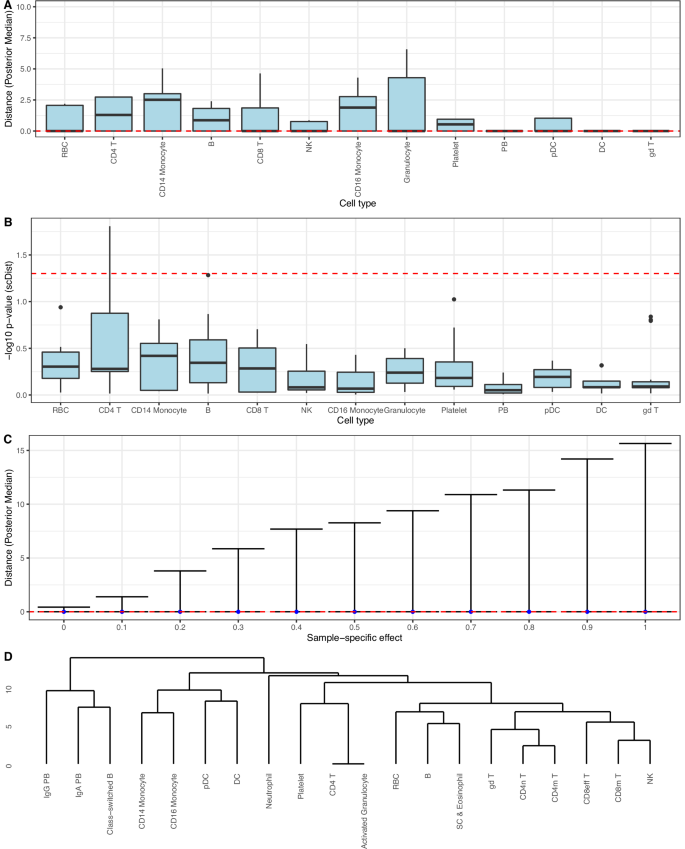
A Reanalysis of the data from Fig. 1 A using distances calculated with scDist ; the dashed red line represents ground truth value of 0. B As in A , but for p-values computed with scDist ; values above the dashed red line represent p < 0.05. C Null simulation from Fig. 1 C reanalyzed using distances calculated by scDist ; the dashed red line represents the ground truth value of 0. Points and error bands represent the mean ±1 SD. D Dendrogram generated by hierarchical clustering based on distances between pairs of cell types estimated by scDist . In all panels the boxplots display median and first/third quartiles. Source data are provided as a Source Data file.
The Euclidean distance D measures perturbation by taking the sum of squared differences across all genes. To show that this measure is biologically meaningful, we applied scDist to obtain estimated distances between pairs of known cell types in the above dataset and then applied hierarchical clustering to these distances. The resulting clustering is consistent with known relationships driven by cell lineages (Fig. 3 D). Specifically, Lymphoid cell types T and NK cells clustered together, while B cells were further apart, and Myeloid cell types DC, monocytes, and neutrophils were close to each other.
Though the scDist distance D assigns each gene an equal weight (unweighted), scDist includes an option to assign different weights w g to each gene (Methods). Weighting could be useful in situations where certain genes are known to contribute more to specific phenotypes. We conducted a simulation to study the impact of using the weighted distance. These simulations show that when a priori information is available, using the correct weighting leads to a slightly better estimation of the distance. However, incorrect weighting leads to significantly worse estimation compared to the unweighted distance (Fig. S3 ). Therefore, the unweighted distance is recommended unless strong a priori information is available.
Challenges in cell type annotations are expected to impact scDist ’s interpretation, much like it does for other methods reliant on a priori cell type annotation such as 3 , 9 . Our simulations (see Methods), reveal scDist ’s vulnerability to false-negatives when annotations are confounded by condition- or patient-specific factors. However, when clusters are annotated using data where such differences have been removed, scDist’s predictions become more reliable (Fig. S23 ). Thus, we recommend removing these confounders before annotation. As potential issues could occur when the inter-condition distance exceeds the inter-cell-type distance, scDist provides a diagnostic plot (Fig. S6 ) to compare these two distances. scDist also incorporates an additional diagnostic feature (Fig. S24 ) to identify annotation issues, utilizing a cell-type tree to evaluate cell relationships at different hierarchical levels. Inconsistencies in scDist ’s output signal potential clustering or annotation errors.
Comparison to counting the number of DEGs
We also compared scDist to the approach of counting the number of differentially expressed genes (nDEG) on pseudobulk samples 3 . Given that the statistical power to detect DEGs is heavily reliant on sample size, we hypothesized that nDEG could become a misleading measure of perturbation in single-cell data with a large variance in the number of cells per cell type. To demonstrate this, we applied both methods to resampled COVID-19 data 1 where the number of cells per cell type was artificially varied between 100 and 10,000. nDEG was highly confounded by the number of cells (Fig. 4 A), whereas the scDist distance remained relatively constant despite the varying number of cells (Fig. 4 B). When the number of subsampled cells is small, the ranking of cell types (by perturbation) was preserved by scDist but not by nDEG (Fig. S5 a–c). Additionally, scDist was over 60 times faster than nDEG since the latter requires testing all G genes as opposed to K ≪ G PCs (Fig. S4 ).
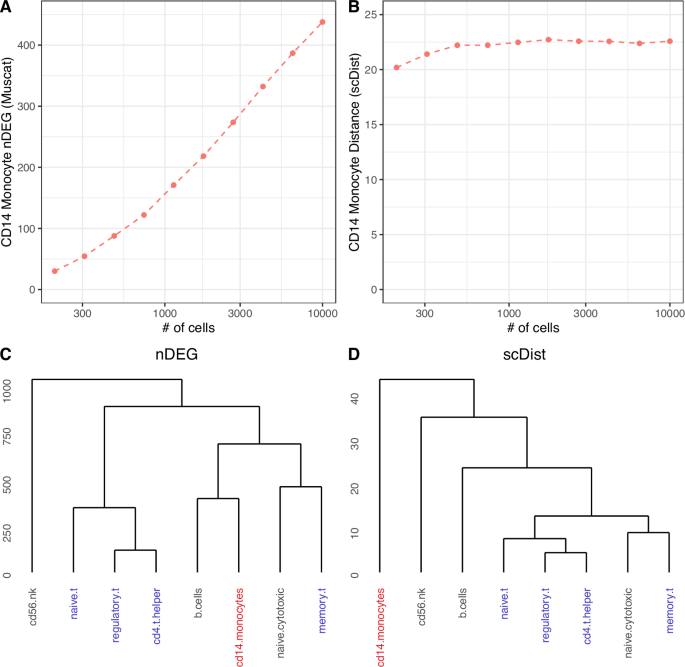
A Sampling with replacement from the COVID-19 dataset 1 to create datasets with a fixed number of cells per cell type, and then counting the number of differentially expressed genes (nDEG) for the CD14 monocytes. B Repeating the previous analysis with the scDist distance. C Comparing all pairs of cell types on the downsampled 15 dataset and applying hierarchical clustering to the pairwise perturbations. Leaves corresponding to T cells are colored blue while the leaf corresponding to monocytes is colored red. D The same analysis using the scDist distances. Source data are provided as a Source Data file.
An additional limitation of nDEG is that it does not account for the magnitude of the differential expression. We illustrated this with a simple simulation that shows the number of DEGs between two cell types can be the same (or less) despite a larger transcriptomic perturbation in gene expression space (Fig. S7 a, b). To demonstrate this on real data, we considered a dataset consisting of eight sorted immune cell types (originally from ref. 15 and combined by ref. 16 ) where scDist and nDEG were applied to all pairs of cell types, and the perturbation estimates were visualized using hierarchical clustering. Although both nDEG and scDist performed well when the sample size was balanced across cell types (Fig. S8 ), nDEG provided inconsistent results when the CD14 Monocytes were downsampled to create a heterogeneous cell type size distribution. Specifically, scDist produced the expected result of clustering the T cells together, whereas nDEG places the Monocytes in the same cluster as B and T cells (Fig. 4 C, D) despite the fact that these belong to different lineages. Thus by taking into account the magnitude of the differential expression, scDist is able to produce results more in line with known biology.
We also considered varying the number of patients on simulated data with a known ground truth. Again, the nDEG (computed using a mixed model, as recommended by ref. 10 ) increases as the number of patients increases, whereas scDist remains relatively stable (Fig. S9 a). Moreover, the correlation between the ground truth perturbation and scDist increases as the number of patients increases (Fig. S9 b). Augur was also sensitive to the number of samples and had a lower correlation with the ground truth than both nDEG and scDist .
scDist detects cell types that are different in COVID-19 patient compared to controls
We applied scDist to a large COVID-19 dataset 17 consisting of 1.4 million cells of 64 types from 284 PBMC samples from 196 individuals consisting of 171 COVID-19 patients and 25 healthy donors. The large number of samples of this dataset permitted further evaluation of our approach using real data rather than simulations. Specifically, we defined true distances between the two groups by computing the sum of squared log fold changes (across all genes) on the entire dataset and then estimated the distance on random samples of five cases versus five controls. Because Augur does not estimate distances explicitly, we assessed the two methods’ ability to accurately recapitulate the ranking of cell types based on established ground truth distances. We found that scDist recovers the rankings better than Augur (Fig. 5 A, S10 ). When the size of the subsample is increased to 15 patients per condition, the accuracy of scDist to recover the ground truth rank and distance improves further (Fig. S25 ).
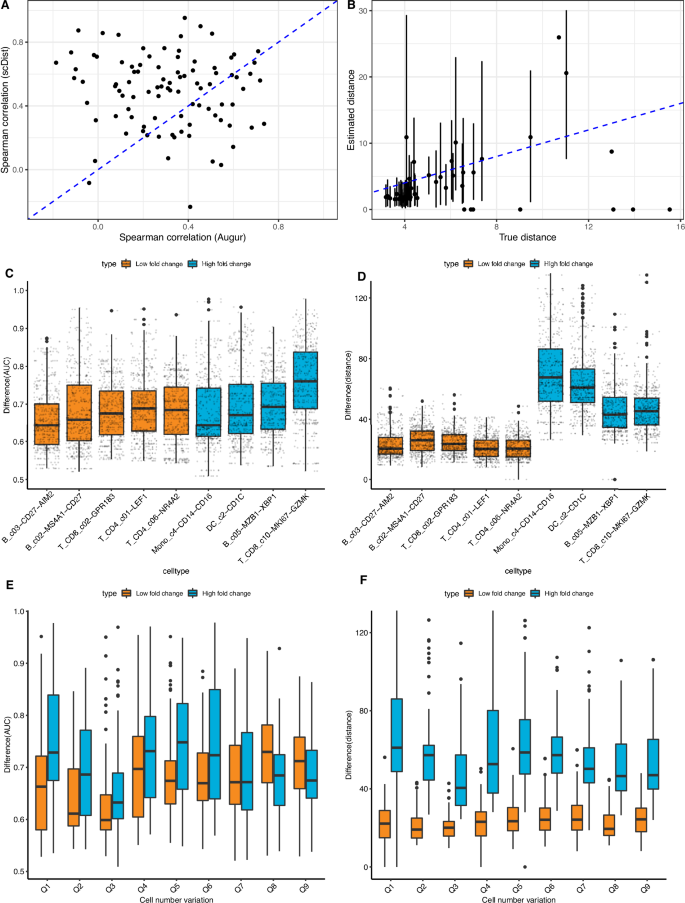
A Correlation between estimated ranks (based on subsamples of 5 cases and 5 controls) and true ranks for each method, with points above the diagonal line indicate better agreement of scDist with the true ranking. B Plot of true distance vs. distances estimated with scDist (dashed line represents y = x ). Poins and error bars represent mean, and 5/95th percentile. C AUC values achieved by Augur , where color represents likely true (blue) or false (orange) positive cell types. D Same as C , but for distances estimated with scDist . E AUC values achieved by Augur against the cell number variation in subsampled-datasets (of false positive cell types). F Same as E , but for distances estimated with scDist . In all boxplots the median and first/third quartiles are reported. For C – E , 1000 random subsamples were used. Source data are provided as a Source Data file.
To evaluate scDist ’s accuracy further, we defined a new ground truth using the entire COVID-19 dataset, consisting two groups: four cell types with differences between groups (true positives) and five cell types without differences (false positives) (Fig. S11 , Methods). We generated 1000 random samples with only five individuals per cell type and estimated group differences using both Augur and scDist . Augur failed to accurately separate the two groups (Fig. 5 C); median difference estimates of all true positive cell types, except MK167+ CD8+T, were lower than median estimates of all true negative cell types (Fig. 5 C). In contrast, scDist showed a separation between scDist estimates between the two groups (Fig. 5 D).
Single-cell data can also exhibit dramatic sample-specific variation in the number of cells of specific cell types. This imbalance can arise from differences in collection strategies, biospecimen quality, and technical effects, and can impact the reliability of methods that do not account for sample-to-sample or individual-to-individual variation. We measured the variation in cell numbers within samples by calculating the ratio of the largest sample’s cell count to the total cell counts across all samples (Methods). Augur ’s predictions were negatively impacted by this cell number variation (Figs. 5 E, S12 ), indicating its increased susceptibility to false positives when sample-specific cell number variation was present (Fig. 1 C). In contrast, scDist ’s estimates were robust to sample-specific cell number variation in single-cell data (Fig. 5 F).
To further demonstrate the advantage of statistical inference in the presence of individual-to-individual variation, we analyzed the smaller COVID-19 dataset 1 with only 13 samples. The original study 1 discovered differences between cases and controls in CD14+ monocytes through extensive manual inspection. scDist identified this same group as the most significantly perturbed cell type. scDist also identified two cell types not considered in the original study, dendritic cells (DCs) and plasmacytoid dendritic cells (pDCs) ( p = 0.01 and p = 0.04, Fig. S13 a), although pDC did not remain significant after adjusting for multiple testing. We note that DCs induce anti-viral innate and adaptive responses through antigen presentation 18 . Our finding was consistent with studies reporting that DCs and pDCs are perturbed by COVID-19 infection 19 , 20 . In contrast, Augur identified RBCs, not CD14+ monocytes, as the most perturbed cell type (Fig. S14 ). Omitting the patient with the most RBCs dropped the perturbation between infected and control cases estimated by Augur for RBCs markedly (Fig. S14 ), further suggesting that Augur predictions are clouded by patient-level variability.
scDist enables the identification of genes underlying cell-specific across-condition differences
To identify transcriptomic alteration, scDist assigns an importance score to each gene based on its contribution to the overall perturbation (Methods). We assessed this importance score for CD14+ monocytes in small COVID-19 datasets. In this cell type, scDist assigned the highest importance score to genes S100 calcium-binding protein A8 ( S100A8 ) and S100 calcium-binding protein A9 ( S100A9 ) ( p < 10 −3 , Fig. S13 b). These genes are canonical markers of inflammation 21 that are upregulated during cytokine storm. Since patients with severe COVID-19 infections often experience cytokine storms, the result suggests that S100A8/A9 upregulation in CD14+ monocyte could be a marker of the cytokine storm 22 . These two genes were reported to be upregulated in COVID-19 patients in the study of 284 samples 17 .
scDist identifies transcriptomic alterations associated with immunotherapy response
To demonstrate the real-world impact of scDist , we applied it to four published dataset used to understand patient responses to cancer immunotherapy in head and neck, bladder, and skin cancer patients, respectively 2 , 23 , 24 , 25 . We found that each individual dataset was underpowered to detect differences between responders and non-responders (Fig. S15 ). To potentially increase power, we combined the data from all cohorts (Fig. 6 A). However, we found that analyzing the combined data without accounting for cohort-specific variations led to false positives. For example, responder-non-responder differences estimated by Augur were highly correlated between pre- and post-treatments (Fig. 6 B), suggesting a confounding effect of cohort-specific variations. Furthermore, Augur predicted that most cell types were altered in both pre-treatment and post-treatment samples (AUC > 0.5 for 41 in pre-treatment and 44 in post-treatment out of a total of 49 cell types), which is potentially due to the confounding effect of cohort-specific variations.
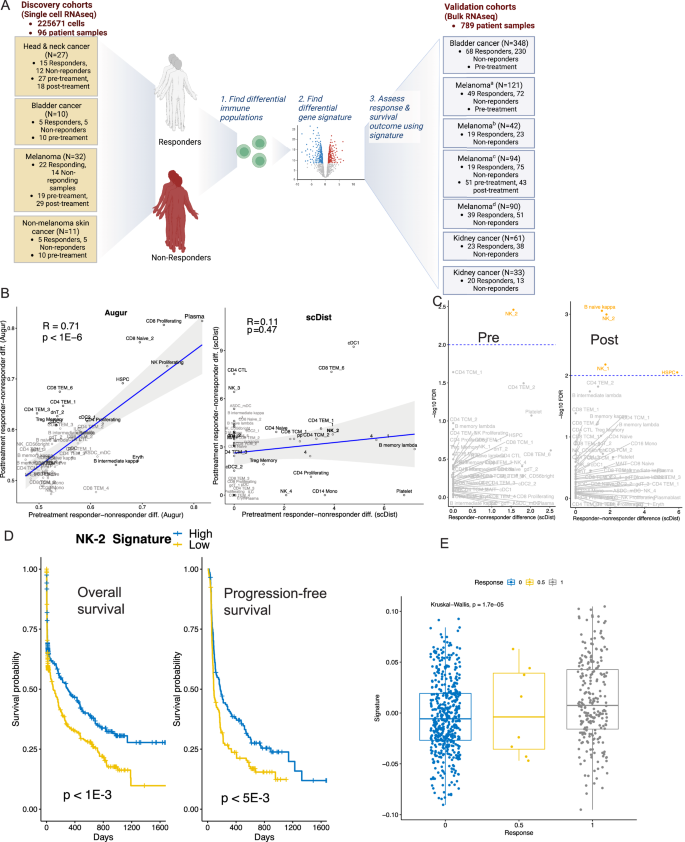
A Study design: discovery cohorts of four scRNA cohorts (cited in order as shown 2 , 23 , 24 , 25 ) identify cell-type-specific differences and a differential gene signature between responders and non-responders. This signature was evaluated in validation cohorts of six bulk RNA-seq cohorts (cited in order as shown 32 , 33 , 34 , 35 , 36 , 37 , 38 ). B Pre-treatment and post-treatment sample differences were estimated using Augur and scDist (Spearman correlation is reported on the plot). The error bars represent 95% confidence interval for the fitted linear regression line. C Significance of the estimated differences ( scDist ). D Kaplan–Meier plots display the survival differences in anti-PD-1 therapy patients, categorized by low-risk and high-risk groups using the median value of the NK-2 signature value; overall and progression-free survival is shown. E NK-2 signature levels in non-responders, partial-responders, and responders (Bulk RNA-seq cohorts). The boxplots report the median and first/third quartiles. A created with BioRender.com, released under a Creative Commons Attribution-NonCommercial-NoDerivs 4.0 International license. Source data are provided as a Source Data file.
To account for cohort-specific variations, we ran scDist including an explanatory variable to the model ( 18 ) to account for cohort effects. With this approach, distance estimates were not correlated significantly between pre- and post-treatment (Fig. 6 B). Removal of these variables re-established correlation (Fig. S16 ). scDist predicted CD4-T and CD8-T altered pre-treatment (Fig. S17 a), while NK, CD8-T, and B cells altered post-treatment (Fig. S17 b). Analysis of subtypes revealed FCER1G+NK cells (NK-2) were changed in both pre-treatment and post-treatment samples (Fig. 6 C). To validate this finding, we generated an NK-2 signature differential between responders and non-responders (Fig. S18 Methods) and evaluated these signatures in bulk RNA-seq immunotherapy cohorts, composing 789 patient samples (Fig. 6 A). We scored each of the 789 patient samples using the NK-2 differential signature (Methods). The NK-2 signature scores were significantly associated with overall and progression-free survival (Fig. 6 D) as well as radiology-based response (Fig. 6 E). We similarly evaluated the top Augur prediction. Differential signature from plasma, the top predicted cell type by Augur , did not show an association with the response or survival outcomes in 789 bulk transcriptomes (Fig. S19 , Methods).
scDist is computationally efficient
A key strength of the linear modeling framework used by scDist is that it is efficient on large datasets. For instance, on the COVID-19 dataset with 13 samples 1 , scDist completed the analysis in around 50 seconds, while Augur required 5 minutes. To better understand how runtime depends on the number of cells, we applied both methods to subsamples of the dataset that varied in size and observed that scDist was, on average, five-fold faster (Fig. S20 ). scDist is also capable of scaling to millions of cells. On simulated data, scDist required approximately 10 minutes to fit a dataset with 1,000,000 cells (Fig. S21 ). We also tested the sensitivity of scDist to the number of PCs used by comparing D K for various values of K . We observed that the estimated distances stabilize as K increases (Fig. S22 ), justifying K = 20 as a reasonable choice for most datasets.
The identification of cell types influenced by infections, treatments, or biological conditions is crucial for understanding their impact on human health and disease. We present scDist , a statistically rigorous and computationally fast method for detecting cell-type specific differences across multiple groups or conditions. By using a mixed-effects model, scDist estimates the difference between groups while quantifying the statistical uncertainty due to individual-to-individual variation and other sources of variability. We validated scDist through the unbiased recapitulation of known relationships between immune cells and demonstrated its effectiveness in mitigating false positives from patient-level and technical variations in both simulated and real datasets. Notably, scDist facilitates biological discoveries from scRNA cohorts, even when the number of individuals is limited, a common occurrence in human scRNA-seq datasets. We also pointed out how the detection of cell-type specific differences can be obscured by batch effects or other confounders and how the linear model used by our approach permits accounting for these.
Since the same expression data is used for annotation and by scDist , there are potential issues associated with “double dipping.” Our simulation highlighted this issue by showing that condition-specific effects can result in over-clustering and downward bias in the estimated distances (Methods, Fig. S23 ). Users can avoid these false negatives by using annotation approaches that can control for patient and condition-specific effects. scDist provides two diagnostic tools to help users identify potential issues in their annotation (Figs. S24 and S6 . Despite this, significant errors in clustering and annotation could cause unavoidable bias in scDist , and thus designing a cluster-free extension of scDist is an area for future work. scDist also provides a diagnostic tool that estimates distances at multiple resolutions to help users identify potential issues in their annotation (Fig. S24 ). Another point of sensitivity for scDist is the choice of the number of principal components used to estimate the distance. Although in practice we observed that the distance estimate is stable as the number of PCs varies between 20 and 50 (Fig. S22 ), an adaptive approach for selecting K could improve performance and maximize power. Finally, although Pearson residual-based normalized counts 12 , 13 is recommended input for scDist , if the data available was normalized by another, sub-optimal, approach, scDist ’s performances could be affected. A future version could adapt the model and estimation procedure so that scDist can be directly applied to the counts, and avoid potential problems introduced by normalization.
We believe that scDist will have extensive utility, as the comparison of single-cell experiments between groups is a common task across a range of research and clinical applications. In this study, we have focused on examining discrete phenotypes, such as infected versus non-infected (in COVID-19 studies) and responders vs. non-responders to checkpoint inhibitors. However, the versatility of our framework allows for extension to experiments involving continuous phenotypes or conditions, such as height, survival, and exposure levels, to name a few. As single-cell datasets continue to grow in size and complexity, scDist will enable rigorous and reliable insights into cellular perturbations with implications for human health and disease.
Normalization
Our method takes as input a normalized count matrix (with corresponding cell type annotations). We recommend using scTransform 13 to normalize, although the method is compatible with any normalization approach. Let y i j g be the UMI counts for gene 1 ≤ g ≤ G in cell i from sample j . scTransform fits the following model:
where r i j is the total number of UMI counts for the particular cell. The normalized counts are given by the Pearson residuals of the above model:
Distance in normalized expression space
In this section, we describe the inferential procedure of scDist for cases without additional covariates. However, the procedure can be generalized to the full model ( 18 ) with arbitrary covariates (design matrix) incorporating random and fixed effects, as well as nested-effect mixed models. For a given cell type, we model the G -dimensional vector of normalized counts as
where \({\boldsymbol{\alpha}},{\boldsymbol{\beta}} \in {{\mathbb{R}}}^{G}\) , x i j is a binary indicator of condition, \({\boldsymbol{\omega }}_{j} \sim {{\mathcal{N}}}(0,{\tau }^{2}{I}_{G})\) , and \({\boldsymbol{\varepsilon }}_{ij} \sim {{\mathcal{N}}}(0,{\sigma }^{2}{I}_{G})\) . The quantity of interest is the Euclidean distance between condition means α and α + β :
If \(U\in {{\mathbb{R}}}^{G\times G}\) is an orthonormal matrix, we can apply U to equation ( 6 ) to obtain the transformed model :
Since U is orthogonal, U ω j and U ε i j still have spherical normal distributions. We also have that
This means that the distance in the transformed model is the same as in the original model. As mentioned earlier, our goal is to find U such that
with K ≪ G .
Let \(Z\in {{\mathbb{R}}}^{n\times G}\) be the matrix with rows z i j (where n is the total number of cells). Intuitively, we want to choose a U such that the projection of z i j onto the first K rows of U ( \({u}_{1},\ldots,{u}_{K}\in {{\mathbb{R}}}^{G}\) ) minimizes the reconstruction error
where \(\mu \in {{\mathbb{R}}}^{G}\) is a shift vector and \(({v}_{ik})\in {{\mathbb{R}}}^{n\times K}\) is a matrix of coefficients. It can be shown that the PCA of Z yields the (orthornormal) u 1 , …, u K that minimizes this reconstruction error 26 .
Given an estimator \(\widehat{{(U{\boldsymbol{\beta}} )}_{k}}\) of ( U β ) k , a naive estimator of D K is given by taking the square root of the sum of squared estimates:
However, this estimator can have significant upward bias due to sampling variability. For instance, even if the true distance is 0, \(\widehat{{(U\beta )}_{k}}\) is unlikely to be exactly zero, and that noise becomes strictly positive when squaring.
To account for this, we apply a post-hoc Bayesian procedure to the \({\widehat{U\beta }}_{k}\) to shrink them towards zero before computing the sum of squares. In particular, we adopt the spike slab model of 14
where \({{\rm{Var}}}[\widehat{{(U{\boldsymbol{\beta}} )}_{k}}]\) is the variance of the estimator \(\widehat{{(U{\boldsymbol{\beta}} )}_{k}}\) , δ 0 is a point mass at 0, and π 0 , π 1 , … π T are mixing weights (that is, they are non-negative and sum to 1). 14 provides a fast empirical Bayes approach to estimate the mixing weights and obtain posterior samples of ( U β ) k . Then samples from the posterior of D K are obtained by applying the formula ( 12 ) to the posterior samples of ( U β ) k . We then summarize the posterior distribution by reporting the median and other quantiles. Advantage of this particular specification is that the amount of shrinkage depends on the uncertainty in the initial estimate of ( U β ) k .
We use the following procedure to obtain \({\widehat{U\beta }}_{k}\) :
Use the matrix of PCA loadings as a plug in estimator for U . Then U z i j is the vector of PC scores for cell i in sample j .
Estimate ( U β ) k by using lme4 27 to fit the model ( 6 ) using the PC scores corresponding to the k -th loading (i.e., each dimension is fit independently).
Note that only the first K rows of U need to be stored.
We are particularly interested in testing the null hypothesis of D K = 0 against the alternative D d > 0. Because the null hypothesis corresponds to ( U β ) k = 0 for all 1 ≤ k ≤ d , we can use the sum of individual Wald statistics as our test statistic:
Under the null hypothesis that ( U β ) k = 0, W k can be approximated by a \({F}_{{\nu }_{k},1}\) distribution. ν k is estimated using Satterthwaite’s approximation in lmerTest . This implies that
under the null. Moreover, the W k are independent because we have assumed that covariance matrices for the sample and cell-level noise are multiples of the identity. Equation ( 16 ) is not a known distribution but quantiles can be approximated using Monte Carlo samples. To make this precise, let W 1 , …, W M be draws from equation ( 16 ), where M = 10 5 and let W * be the value of equation ( 15 ) (i.e., the actual test statistic). Then the empirical p -value 28 is computed as
Controlling for additional covariates
Because scDist is based on a linear model, it is straightforward to control for additional covariates such as age or sex of a patient in the analysis. In particular, model ( 18 ) can be replaced with
where \({w}_{ijk}\in {\mathbb{R}}\) is the value of the k th covariate for cell i in sample j and \({\boldsymbol{\gamma }}_{k}\in {{\mathbb{R}}}^{G}\) is the corresponding gene-specific effect corresponding to the k th covariate.
Choosing the number of principal components
An important choice in scDist is the number of principal components d . If d is chosen too small, then estimation accuracy may suffer as the first few PCs may not capture enough of the distance. On the other hand, if d is chosen too large then the power may suffer as a majority of the PCs will simply be capturing random noise (and adding to degrees of freedom to the Wald statistic). Moreover, it is important that d is chosen a priori, as choosing the d that produces the lowest p values is akin to p -hacking.
If the model is correctly specified then it is reasonable to choose d = J − 1, where J is the number of samples (or patients). To see why, notice that the mean expression in sample 1 ≤ j ≤ J is
In particular, the J sample means lie on a ( J − 1)-dimensional subspace in \({{\mathbb{R}}}^{G}\) . Under the assumption that the condition difference and sample-level variability is larger than the error variance σ 2 , we should expect that the first J − 1 PC vectors capture all of the variance due to differences in sample means.
In practice, however, the model can not be expected to be correctly specified. For this reason, we find that d = 20 is a reasonable choice when the number of samples is small (as is usually the case in scRNA-seq) and d = 50 for datasets with a large number of samples. This is line with other single-cell methods, where the number of PCs retained is usually between 20 and 50.
Cell type annotation and “double dipping”
scDist takes as input an annotated list of cells. A common approach to annotate cells is to cluster based on gene expression. Since scDist also uses the gene expression data to measure the condition difference there are concerns associated with “double-dipping” or using the data twice. In particular, if the condition difference is very large and all of the data is used to cluster it is possible that the cells in the two conditions would be assigned to different clusters. In this case scDist would be unable to estimate the inter-condition distance, leading to a false negative. In other words, the issue of double dipping could cause scDist to be more conservative. Note that the opposite problem occurs when performing differential expression between two estimated clusters; in this case, the p -values corresponding to genes will be anti-conservative 29 .
To illustrate, we simulated a normalized count matrix with 4000 cells and 1000 genes in such a way that there are two “true” cell types and a true condition distance of 4 for both cell types (Fig. S23 a). To cluster (annotate) the cells, we applied k -means with various choices of k and compared results by taking the median inter-condition distance across all clusters. As the number of clusters increases, the median distance decays towards 0, which demonstrates that scDist can produce false negatives when the data is over-clustered (Fig. S23 b). To avoid this issue, one possible approach is to begin by clustering the data for only one condition and then to assign cells in the other condition by finding the nearest centroid in the existing clusters. When applied to the simulated data this approach is able to correctly estimate the condition distance even when the number of clusters k is larger than the true value.
On real data, one approach to identify possible over-clustering is to apply scDist at various cluster resolutions. We used the expression data from the small COVID-19 data 1 to construct a tree \({{\mathcal{T}}}\) with leaf nodes corresponding to the cell types in the original annotation provided by the authors (Fig. S24 , see Appendix A for a description of how the tree is estimated). At each internal node \(v\in {{\mathcal{T}}}\) , we applied scDist to the cluster containing all children of v . We can then visualize the estimated distances by plotting the tree (Fig. S24 ). Situations where the child nodes have a small distance but the parent node has a large distance could be indicative of over-clustering. For example, PB cells are almost exclusiviely found in cases (1977 cells in cases and 86 cells in controls), suggesting that it is reasonable to consider PB and B cells as a single-cell type when applying scDist .
Feature importance
To better understand the genes that drive the observed difference in the CD14+ monocytes, we define a gene importance score . For 1 ≤ k ≤ d and 1 ≤ g ≤ G , the k -th importance score for gene g is ∣ U k g ∣ β g . In other words, the importance score is the absolute value of the gene’s k -th PC loading times its expression difference between the two conditions. Note that the gene importance score is 0 if and only if β g = 0 or U k g = 0. Since the U k g are fixed and known, significance can be assigned to the gene importance score using the differential expression method used to estimate β g .
Simulated single-cell data
We test the method on data generated from model equation ( 6 ). To ensure that the “true” distance is D , we use the R package uniformly 30 to draw β from the surface of the sphere of radius D in \({{\mathbb{R}}}^{G}\) . The data in Figs. 1 C and 3 C are obtained by setting β = 0 and σ 2 = 1 and varying τ 2 between 0 and 1.
Weighted distance
By default, scDist uses the Euclidean distance D which treats each gene equally. In cases where a priori information is available about the relevance of each gene, scDist provides the option to estimate a weighted distance D w , where \(w\in {{\mathbb{R}}}^{G}\) has non-negative components and
The weighted distance can be written in matrix form by letting \(W\in {{\mathbb{R}}}^{G\times G}\) be a diagonal matrix with W g g = w g , so that
Thus, the weighted distance can be estimated by instead considered the transformed model where \(U\sqrt{W}\) is applied to each z i j . After this different transformed model is obtained, estimation and inference of D w proceeds in exactly the same way as the unweighted case.
To test the accuracy of the weighted distance estimate, we considered a simulation where each gene had only a 10% chance of having β g ≠ 0 (otherwise \({\beta }_{g} \sim {{\mathcal{N}}}(0,1)\) ). We then considered three scenarios: w g = 1 if β g ≠ 0 and w g = 0 otherwise (correct weighting), w g = 1 for all g (unweighted), and w g = 1 randomly with probability 0.1 (incorrect weights). We then quantified the performance by taking the absolute value of the error between \({\sum }_{g}{\beta }_{g}^{2}\) and the estimated distance. Figure S3 shows that correct weighting slightly outperforms unweighted scDist but random weights are significantly worse. Thus, the unweighted version of scDist should be preferred unless strong a priori information is available.
Robustness to model misspecification
The scDist model assumes that the cell-specific variance σ 2 and sample-specific variance τ 2 are shared across genes. The purpose of this assumption is to ensure that the noise in the transformed model follows a spherical normal distribution. Violations of this assumption could lead to miscalibrated standard errors and hypothesis tests but should not effect estimation. To demonstrate this, we considered simulated data where each gene has σ g ~ Gamma( r , r ) and τ g ~ Gamma( r /2, r ). As r varies, the quality of the distance estimates does not change significantly (Fig. S26 ).
Semi-simulated COVID-19 data
COVID-19 patient data for the analysis was obtained from ref. 17 , containing 1.4 million cells of 64 types from 284 PBMC samples collected from 196 individuals, including 171 COVID-19 patients and 25 healthy donors.
Ground truth
We define the ground truth as the cell-type specific transcriptomic differences between the 171 COVID-19 patients and the 25 healthy controls. Specifically, we used the following approach to define a ground truth distance:
For each gene g , we computed the log fold changes L g between COVID-19 cases and controls, with L g = E g ( C o v i d ) − E g ( C o n t r o l ), where E g denotes the log-transformed expression data \(\log (1+x)\) .
The ground truth distance is then defined as \(D={\sum }_{g}{L}_{g}^{2}\) .
Subsequently, we excluded any cell types not present in more than 10% of the samples from further analysis. For true negative cell types, we identified the top 5 with the smallest fold change and a representation of over 20,000 cells within the entire dataset. When attempting similar filtering based on cell count alone, no cell types demonstrated a sufficiently large true distance. Consequently, we chose the top four cell types with over 5000 cells as our true positives Fig. S11 .
Using the ground truth, we performed two separate simulation analyses:
1: Simulation analyses I (Fig. 5 A, B): Using one half of the dataset (712621 cells, 132 case samples, 20 control samples), we created 100 subsamples consisting of 5 cases and 5 controls. For each subsample, we applied both scDist and Augur to estimate perturbation/distance between cases and controls for each cell type. Then we computed the correlation between the ground truth ranking (ordering cells by sum of log fold changes on the whole dataset) and the ranking obtained by both methods. For scDist , we restricted to cell types that had a non-zero distance estimate in each subsample, and for Augur we restricted to cell types that had an AUC greater than 0.5 (Fig. 5 A). For Fig. 5 B, we took the mean estimated distance across subsamples for which the given cell type had a non-zero distance estimate. This is because in some subsamples a given cell type could be completely absent.
2: Simulation analyses II (Fig. 5 C–F): We subsampled the COVID-19 cohort with 284 samples (284 PBMC samples from 196 individuals: 171 with COVID-19 infection and 25 healthy controls) to create 1,000 downsampled cohorts, each containing samples from 10 individuals (5 with COVID-19 and 5 healthy controls). We randomly selected each sample from the downsampled cohort, further downsampled the number of cells for each cell type, and selected them from the original COVID-19 cohort. This downsampling procedure increases both cohort variability and cell-number variations.
Performance Evaluation in Subsampled Cohorts : We applied scDist and Augur to each subsampled cohort, comparing the results for true positive and false positive cell types. We partitioned the sampled cohorts into 10 groups based on cell-number variation, defined as the number of cells in a sample with the highest number of cells for false-negative cell types divided by the average number of cells in cell types. This procedure highlights the vulnerability of computational methods to cell number variation, particularly in negative cell types.
Analysis of immunotherapy cohorts
Data collection.
We obtained single-cell data from four cohorts 2 , 23 , 24 , 25 , including expression counts and patient response information.
Pre-processing
To ensure uniform processing and annotation across the four scRNA cohorts, we analyzed CD45+ cells (removing CD45− cells) in each cohort and annotated cells using Azimuth 31 with reference provided for CD45+ cells.
Model to account for cohort and sample variance
To account for cohort-specific and sample-specific batch effects, scDist modeled the normalized gene expression as:
Here, Z represents the normalized count matrix, X denotes the binary indicator of condition (responder = 1, non-responder = 0); γ and ω are cohort and sample-level random effects, and (1 ∣ γ : ω ) models nested effects of samples within cohorts. The inference procedure for distance, its variance, and significance for the model with multiple cohorts is analogous to the single-cohort model.
We estimated the signature in the NK-2 cell type using differential expression between responders and non-responders. To account for cohort-specific and patient-specific effects in differential expression estimation, we employed a linear mixed model described above for estimating distances, performing inference for each gene separately. The coefficient of X inferred from the linear mixed models was used as the estimate of differential expression:
Here, Z represents the normalized count matrix, X denotes the binary indicator of condition (responder = 1, non-responder = 0); γ and ω are cohort and sample-level random effects, and (1 ∣ γ : ω ) models nested effects of samples within cohorts.
Bulk RNA-seq cohorts
We obtained bulk RNA-seq data from seven cancer cohorts 32 , 33 , 34 , 35 , 36 , 37 , 38 , comprising a total of 789 patients. Within each cohort, we converted counts of each gene to TPM and normalized them to zero mean and unit standard deviation. We collected survival outcomes (both progression-free and overall) and radiologic-based responses (partial/complete responders and non-responders with stable/progressive disease) for each patient.
Evaluation of signature in bulk RNA-seq cohorts
We scored each bulk transcriptome (sample) for the signature using the strategy described in ref. 39 . Specifically, the score was defined as the Spearman correlation between the normalized expression and differential expression in the signature. We stratified patients into two groups using the median score for patient stratification. Kaplan–Meier plots were generated using these stratifications, and the significance of survival differences was assessed using the log-rank test. To demonstrate the association of signature levels with radiological response, we plotted signature levels separately for non-responders, partial-responders, and responders.
Evaluating Augur Signature in Bulk RNA-Seq Cohorts
A differential signature was derived for Augur ’s top prediction, plasma cells, using a procedure analogous to the one described above for scDist . This plasma signature was then assessed in bulk RNA-seq cohorts following the same evaluation strategy as applied to the scDist signature.
Statistics and reproducibility
No statistical method was used to predetermine sample size. No data were excluded from the analyses. The experiments were not randomized. The Investigators were not blinded to allocation during experiments and outcome assessment.
Reporting summary
Further information on research design is available in the Nature Portfolio Reporting Summary linked to this article.
Data availability
Table 1 gives a list of the datasets used in each figure, as well as details about how the datasets can be obtained. Source data are provided with this paper.
Code availability
scDist is available as an R package and can be downloaded from GitHub 40 : github.com/phillipnicol/scDist . The repository also includes scripts to replicate some of the figures and a demo of scDist using simulated data.
Wilk, A. J. et al. A single-cell atlas of the peripheral immune response in patients with severe covid-19. Nat. Med. 26 , 1070–1076 (2020).
Article PubMed PubMed Central Google Scholar
Yuen, K. C. et al. High systemic and tumor-associated il-8 correlates with reduced clinical benefit of pd-l1 blockade. Nat. Med. 26 , 693–698 (2020).
Crowell, H. L. et al. Muscat detects subpopulation-specific state transitions from multi-sample multi-condition single-cell transcriptomics data. Nat. Commun. 11 , 6077 (2020).
Article ADS PubMed PubMed Central Google Scholar
Helmink, B. A. et al. B cells and tertiary lymphoid structures promote immunotherapy response. Nature 577 , 549–555 (2020).
Zhao, J. et al. Detection of differentially abundant cell subpopulations in scrna-seq data. Proc. Natl. Acad. Sci. 118 , e2100293118 (2021).
Dann, E., Henderson, N. C., Teichmann, S. A., Morgan, M. D. & Marioni, J. C. Differential abundance testing on single-cell data using k-nearest neighbor graphs. Nat. Biotechnol. 40 , 245–253 (2022).
Article PubMed Google Scholar
Burkhardt, D. B. et al. Quantifying the effect of experimental perturbations at single-cell resolution. Nat. Biotechnol. 39 , 619–629 (2021).
McInnes, L., Healy, J. & Melville, J. Umap: uniform manifold approximation and projection for dimension reduction. arXiv https://arxiv.org/abs/1802.03426 (2018).
Skinnider, M. A. et al. Cell type prioritization in single-cell data. Nat. Biotechnol. 39 , 30–34 (2021).
Zimmerman, K. D., Espeland, M. A. & Langefeld, C. D. A practical solution to pseudoreplication bias in single-cell studies. Nat. Commun. 12 , 1–9 (2021).
Article Google Scholar
Korsunsky, I. et al. Fast, sensitive and accurate integration of single-cell data with harmony. Nat. Methods 16 , 1289–1296 (2019).
Townes, F. W., Hicks, S. C., Aryee, M. J. & Irizarry, R. A. Feature selection and dimension reduction for single-cell rna-seq based on a multinomial model. Genome Biol. 20 , 1–16 (2019).
Hafemeister, C. & Satija, R. Normalization and variance stabilization of single-cell RNA-seq data using regularized negative binomial regression. Genome Biol. 20 , 1–15 (2019).
Stephens, M. False discovery rates: a new deal. Biostatistics 18 , 275–294 (2017).
MathSciNet PubMed Google Scholar
Zheng, G. X. et al. Massively parallel digital transcriptional profiling of single cells. Nat. Commun. 8 , 14049 (2017).
Duò, A., Robinson, M. D. & Soneson, C. A systematic performance evaluation of clustering methods for single-cell RNA-seq data. F1000Res. 7 , 1141 (2018).
Ren, X. et al. Covid-19 immune features revealed by a large-scale single-cell transcriptome atlas. Cell 184 , 1895–1913 (2021).
Galati, D., Zanotta, S., Capitelli, L. & Bocchino, M. A bird’s eye view on the role of dendritic cells in sars-cov-2 infection: Perspectives for immune-based vaccines. Allergy 77 , 100–110 (2022).
Pérez-Gómez, A. et al. Dendritic cell deficiencies persist seven months after sars-cov-2 infection. Cell. Mol. Immunol. 18 , 2128–2139 (2021).
Upadhyay, A. A. et al. Trem2+ and interstitial macrophages orchestrate airway inflammation in sars-cov-2 infection in rhesus macaques. bioRxiv https://www.biorxiv.org/content/10.1101/2021.10.05.463212v1 (2021).
Wang, S. et al. S100a8/a9 in inflammation. Front. Immunol. 9 , 1298 (2018).
Mellett, L. & Khader, S. A. S100a8/a9 in covid-19 pathogenesis: impact on clinical outcomes. Cytokine Growth Factor Rev. 63 , 90–97 (2022).
Luoma, A. M. et al. Tissue-resident memory and circulating t cells are early responders to pre-surgical cancer immunotherapy. Cell 185 , 2918–2935 (2022).
Yost, K. E. et al. Clonal replacement of tumor-specific t cells following pd-1 blockade. Nat. Med. 25 , 1251–1259 (2019).
Sade-Feldman, M. et al. Defining T cell states associated with response to checkpoint immunotherapy in melanoma. Cell 175 , 998–1013 (2018).
Pearson, K. Liii. on lines and planes of closest fit to systems of points in space. Lond. Edinb. Dublin Philos. Mag. J. Sci. 2 , 559–572 (1901).
Bates, D., Mächler, M., Bolker, B. & Walker, S. Fitting linear mixed-effects models using lme4. J. Stat. Softw. 67 , 1–48 (2015).
North, B. V., Curtis, D. & Sham, P. C. A note on the calculation of empirical p values from Monte Carlo procedures. Am. J. Hum. Genet. 71 , 439–441 (2002).
Neufeld, A., Gao, L. L., Popp, J., Battle, A. & Witten, D. Inference after latent variable estimation for single-cell RNA sequencing data. arXiv https://arxiv.org/abs/2207.00554 (2022).
Laurent, S. uniformly: uniform sampling. R package version 0.2.0 https://CRAN.R-project.org/package=uniformly (2022).
Hao, Y. et al. Integrated analysis of multimodal single-cell data. Cell 184 , 3573–3587 (2021).
Mariathasan, S. et al. Tgf β attenuates tumour response to pd-l1 blockade by contributing to exclusion of T cells. Nature 554 , 544–548 (2018).
Weber, J. S. et al. Sequential administration of nivolumab and ipilimumab with a planned switch in patients with advanced melanoma (checkmate 064): an open-label, randomised, phase 2 trial. Lancet Oncol. 17 , 943–955 (2016).
Liu, D. et al. Integrative molecular and clinical modeling of clinical outcomes to pd1 blockade in patients with metastatic melanoma. Nat. Med. 25 , 1916–1927 (2019).
McDermott, D. F. et al. Clinical activity and molecular correlates of response to atezolizumab alone or in combination with bevacizumab versus sunitinib in renal cell carcinoma. Nat. Med. 24 , 749–757 (2018).
Riaz, N. et al. Tumor and microenvironment evolution during immunotherapy with nivolumab. Cell 171 , 934–949 (2017).
Miao, D. et al. Genomic correlates of response to immune checkpoint therapies in clear cell renal cell carcinoma. Science 359 , 801–806 (2018).
Van Allen, E. M. et al. Genomic correlates of response to ctla-4 blockade in metastatic melanoma. Science 350 , 207–211 (2015).
Sahu, A. et al. Discovery of targets for immune–metabolic antitumor drugs identifies estrogen-related receptor alpha. Cancer Discov. 13 , 672–701 (2023).
Nicol, P. scdist https://doi.org/10.5281/zenodo.12709683 (2024).
Download references
Acknowledgements
P.B.N. is supported by NIH T32CA009337. A.D.S. received support from R00CA248953, the Michelson Foundation, and was partially supported by the UNM Comprehensive Cancer Center Support Grant NCI P30CA118100. We express our gratitude to Adrienne M. Luoma, Shengbao Suo, and Kai W. Wucherpfennig for providing the scRNA data 23 . We also thank Zexian Zeng for assistance with downloading and accessing the bulk RNA-seq dataset.
Author information
Authors and affiliations.
Harvard University, Cambridge, MA, USA
Phillip B. Nicol & Danielle Paulson
University of California San Diego School of Medicine, San Diego, CA, USA
Dana-Farber Cancer Institute, Boston, MA, USA
X. Shirley Liu & Rafael Irizarry
University of New Mexico Comprehensive Cancer Center, Albuquerque, NM, USA
Avinash D. Sahu
You can also search for this author in PubMed Google Scholar
Contributions
P.B.N., D.P., G.Q., X.S.L., R.I., and A.D.S. conceived the study. P.B.N. and A.D.S. implemented the method and performed the experiments. P.B.N., R.I., and A.D.S. wrote the manuscript.
Corresponding authors
Correspondence to Rafael Irizarry or Avinash D. Sahu .
Ethics declarations
Competing interests.
X.S.L. conducted the work while being on the faculty at DFCI, and is currently a board member and CEO of GV20 Therapeutics. P.B.N., D.P., G.Q., R.I., and A.D.S. declare no competing interests.
Peer review
Peer review information.
Nature Communications thanks the anonymous reviewer(s) for their contribution to the peer review of this work. A peer review file is available.
Additional information
Publisher’s note Springer Nature remains neutral with regard to jurisdictional claims in published maps and institutional affiliations.
Supplementary information
Supplementary information, peer review file, reporting summary, source data, source data, rights and permissions.
Open Access This article is licensed under a Creative Commons Attribution 4.0 International License, which permits use, sharing, adaptation, distribution and reproduction in any medium or format, as long as you give appropriate credit to the original author(s) and the source, provide a link to the Creative Commons licence, and indicate if changes were made. The images or other third party material in this article are included in the article’s Creative Commons licence, unless indicated otherwise in a credit line to the material. If material is not included in the article’s Creative Commons licence and your intended use is not permitted by statutory regulation or exceeds the permitted use, you will need to obtain permission directly from the copyright holder. To view a copy of this licence, visit http://creativecommons.org/licenses/by/4.0/ .
Reprints and permissions
About this article
Cite this article.
Nicol, P.B., Paulson, D., Qian, G. et al. Robust identification of perturbed cell types in single-cell RNA-seq data. Nat Commun 15 , 7610 (2024). https://doi.org/10.1038/s41467-024-51649-3
Download citation
Received : 14 December 2023
Accepted : 09 August 2024
Published : 01 September 2024
DOI : https://doi.org/10.1038/s41467-024-51649-3
Share this article
Anyone you share the following link with will be able to read this content:
Sorry, a shareable link is not currently available for this article.
Provided by the Springer Nature SharedIt content-sharing initiative
By submitting a comment you agree to abide by our Terms and Community Guidelines . If you find something abusive or that does not comply with our terms or guidelines please flag it as inappropriate.
Quick links
- Explore articles by subject
- Guide to authors
- Editorial policies
Sign up for the Nature Briefing newsletter — what matters in science, free to your inbox daily.

- Open access
- Published: 29 August 2024
Temporalities of oxytocin for labour augmentation: a mixed-methods study of time factors shaping labour practices in a busy maternity unit in Tanzania
- Monica Lauridsen Kujabi 1 , 2 ,
- Luzango Maembe 3 ,
- Daniel Nkungu 4 ,
- Nanna Maaløe 1 , 5 ,
- Brenda Sequeira D’mello 1 , 6 , 7 ,
- Jos van Roosmalen 8 , 9 ,
- Thomas van den Akker 8 , 9 ,
- Flemming Konradsen 1 , 10 ,
- Kidanto Hussein 6 ,
- Eunice Pallangyo 11 ,
- Morten Skovdal 12 &
- Jane Brandt Sørensen 1
BMC Pregnancy and Childbirth volume 24 , Article number: 563 ( 2024 ) Cite this article
Metrics details
High rates of labour augmentation with oxytocin have been found in some low- and lower-middle-income countries, causing potential perinatal harm. It is critical to understand the reasons for this overuse. Aim was to explore factors that shape practices around using oxytocin for labour augmentation in a high-volume labour ward in Dar es Salaam, Tanzania.
Mixed-methods data collection was conducted from March 2021 to February 2022, including structured observations of 234 births, 220 h of unstructured labour ward observations and 13 individual in-depth interviews with birth attendants. Thematic network analysis and descriptive statistics were used to analyse data. We used a time-lens to understand practices of oxytocin for labour augmentation in time-pressured labour wards.
Birth attendants constantly had to prioritise certain care practices over others in response to time pressure. This led to overuse of oxytocin for augmentation to ensure faster labour progression and decongestion of the, often overburdened, ward. Simultaneously, birth attendants had little time to monitor foetal and maternal condition. Surprisingly, while oxytocin was used in 146 out of 234 (62.4%) structured labour observations, only 9/234 (4.2%) women had active labour lasting more than 12 h. Correspondingly, 21/48 (43.8%) women who were augmented with oxytocin in the first stage of labour had uncomplicated labour progression at the start of augmentation. While the partograph was often not used for decision-making, timing of starting oxytocin often correlated with natural cycles of ward-rounds and shift-turnovers instead of individual women’s labour progression. This resulted in co-existence of ‘too early’ and ‘too late’ use of oxytocin. Liberal use of oxytocin for labour augmentation was facilitated by an underlying fear of prolonged labour and low alertness of oxytocin-related risks.
Conclusions
Time scarcity in the labour ward often made birth attendants deviate from clinical guidelines for labour augmentation with oxytocin. Efforts to navigate time pressure resulted in too many women with uncomplicated labour progression receiving oxytocin with little monitoring of labour. Fear of prolonged labour and low alertness to oxytocin-mediated risks were crucial drivers. These findings call for research into safety and benefits of oxytocin in low-resource settings and interventions to address congestion in labour wards to prevent using oxytocin as a time-management tool.
Peer Review reports
Oxytocin has been used since the 1950s to augment labour by stimulating contractions. Evidence, however, remains missing that oxytocin reduces caesarean section rates, although it has shown to reduce labour duration by 2 h [ 1 , 2 ]. In too high doses, oxytocin may cause harm to women and their foetuses [ 3 , 4 ]. Potential complications of its use include uterine rupture and stillbirth [ 5 ]. The required dose of oxytocin differs per individual and correct dosing requires titrating oxytocin against the woman’s contractions, whilst monitoring the foetal heart rate (FHR). Risks of oxytocin-related harm appear higher in low-resource settings with substandard monitoring [ 3 ]. Dosing in such settings often happens by visual drop count rather than electronic infusion, which is time-demanding and increases the risk of under- or overdosing. Availability of cardiotocography is rare in low-resource settings and replaced by intermittent auscultation of FHR.
It is troubling that the few studies conducted in low- and lower-middle-income countries (LLMIC) find that 89.5% of women receiving oxytocin for labour augmentation had uncomplicated labour progress, with some facilities using the drug in 80%-90% of women during birth [ 3 ]. This is much higher than the suggested 9–12% [ 6 , 7 ]. Despite dangerously high rates of oxytocin for labour augmentation in some LLMICs, few studies have explored the underlying reasons.
Qualitative studies of labour augmentation with oxytocin have identified local demands among labouring women in Nepal, India and Uganda [ 8 , 9 , 10 , 11 ]. Studies from India, Nepal, Uganda and Sierra Leone suggest that birth attendants often underestimate the risks associated with oxytocin for labour augmentation and lack of skills in diagnosing prolonged labour and timing of oxytocin for labour augmentation [ 8 , 9 , 10 , 12 ]. Finally, Khalil et al. explain how, in an Egyptian hospital, shortages of beds and hands were the main reasons for nearly universal oxytocin used for labour augmentation [ 13 ] while Van Hollen describes how oxytocin was used as “crowd control” [ 11 ]. These studies stop short of exploring such notions any further.
In alignment with previous studies suggesting that untimely and too frequent use of oxytocin to augment labour could be related to congestion, this mixed-methods study was conducted in an urban high-volume maternity unit, which historically has been among the most congested labour wards in Dar es Salaam, the largest urban centre of Tanzania and one of the fastest growing cities on the continent. The objective of this study was to investigate factors affecting practices around labour augmentation with oxytocin in a high-volume maternity unit through the lens of time. Here, time refers to the duration of labour, birth attendant-to-women ratio, and frequency, duration and timing of care practices.
This mixed-methods study forms part of the PartoMa research project, aiming at co-creating contextualised intrapartum clinical guidelines and training [ 14 , 15 ]. The study took place in a public urban maternity unit in a regional referral hospital in Dar es Salaam, Tanzania. Annual number of births was around 7,000, in 2021, much lower than the number of 16,000 in 2016 [ 16 ]. This reduction likely resulted from a policy promoting decongestion of the busiest referral facilities by re-distributing women more evenly among the facilities [ 16 ]. The Covid-19 pandemic may also have influenced the facility birth rate [ 17 ].
In the latent phase (until cervical dilatation of 4 cm), women are monitored in the antenatal ward where they often share beds with other women. In active phase (cervical dilatation 4 cm or above), they enter the labour ward. The labour ward with 16 beds is managed by two to three nurse-midwives and a varying number of nurse-midwifery students, interns, registrar doctors and obstetric specialists. Nurse-midwives mostly practice on their own. They triage women on admission, monitor and manage labour, resuscitate neonates, prepare and escort women for caesarean section, provide immediate postnatal care and escort severely ill women to the national hospital. In addition, they are responsible for cleaning up beds after birth, keeping the ward clean, filling up equipment and medication storages and documenting information in multiple registers. All these extra tasks imply that generally, 1–2 nurse-midwives provide care to the women in the 16 beds with varying occupancy. Oxytocin is administered with manual infusion lines with 5 International Units in 500 ml saline. Contractions are measured manually over ten minutes and FHR assessed using intermitted auscultation with fetoscope or doppler. The context of high-volume Tanzanian maternity wards has also been described by others [ 16 , 18 , 19 , 20 ].
Conceptual framework
To investigate the role of time, we draw on the concept of “Timescapes”. This concept was developed by sociologist Barbara Adam to highlight the interdependency between space and time [ 21 ]. The ‘Timescapes-concept’ allows us to examine how the spatial context of a busy labour ward (the ‘scapes’ part) combined with the duration of labour and timing of labour interventions (the ‘times’ part) will affect the use of oxytocin for labour augmentation. Table 1 details the clinical guidance around frequency and timing of oxytocin administration, indicating the time spent on key practices. Aiming to provide the best possible care, birth attendants tinker with care practices to make them work in their time pressured and overstretched realities. By exploring how time manifests in such a congested environment we examined the relationship between time scarcity, efforts to manage time and the influence on oxytocin for labour augmentation. Exploration of these efforts included obtaining an in-depth understanding of how tasks are prioritised and planned in a busy and under-resourced labour ward.
Data collection
Data were collected between March 15th 2021 and February 15th 2022 (Table 2 ). We combined three methods: (i) 220 h of unstructured observations and informal conversations with staff to explore labour ward practices; (ii) 13 in-depth individual semi-structured interviews (Additional file 1) to explore birth attendants’ perceptions and (iii) 234 structured observations of labours by two research assistants to quantify oxytocin practices (timing, titration etc.).
Observations were conducted equally during days, evenings and nights over three months. Extensive field notes were written during and after observations. Although being a medical doctor, MLK had no license to practice clinically within this setting and did not make clinical decisions during observations. Structured observations were carried out by two Swahili-speaking research assistants with no obstetric experience. Observed care practices were noted in pre-piloted sheets (Additional file 2). Women were included in active labour and followed till birth. Inclusion happened consecutively during each observation period and was limited to singleton cephalic pregnancies at term with positive foetal heart rate on admission. Women who were referred and women with hypertensive disorders or previous caesarean section were excluded. We aimed to study oxytocin use among a low-risk population to avoid competing factors influencing the administration of oxytocin. MLK and a Swahili-speaking research assistant conducted interviews through purposive sampling until no new topics appeared. The interview guide was adjusted after the first two interviews. Respondents included nurse-midwives ( n = 3), intern doctors ( n = 2), registrars ( n = 3) and obstetric specialists ( n = 3), all working in the labour ward at the time. Re-interviews with two respondents were conducted one year later to explore preliminary analytical findings. All interviews were conducted outside working hours and were audio-recorded, transcribed and translated ad verbatim to English by a trained translator. Translations were checked by two research assistants. To protect anonymity, staff members are referred to as respondents (R) or birth attendants throughout the text. In this manuscript, oxytocin refers to oxytocin for labour augmentation during active labour unless stated otherwise.
A thematic network analysis was carried out using a combined deductive and inductive approach where time emerged as a central theme [ 26 ]. Text was coded using NVivo (release 1.6.2) and included predefined and emerging themes. Data from observations and interviews were triangulated and basic, organising and global themes were created within the frame of temporality (Table 3 ). Descriptive statistics using IBM SPSS (version 28.0.0.0) were used to analyse data from structured observations. Rate of oxytocin for labour augmentation was based on all observed women, while a subgroup of women who received oxytocin during the first stage of labour were assessed for timing of oxytocin and monitoring of labour.
Three global, seven organising and 17 basic themes emerged from the analysis and all related to temporality (Table 3 ). Our findings are structured around the three global themes. Figure 1 shows the conceptual framework, which was developed from the results of our analysis.
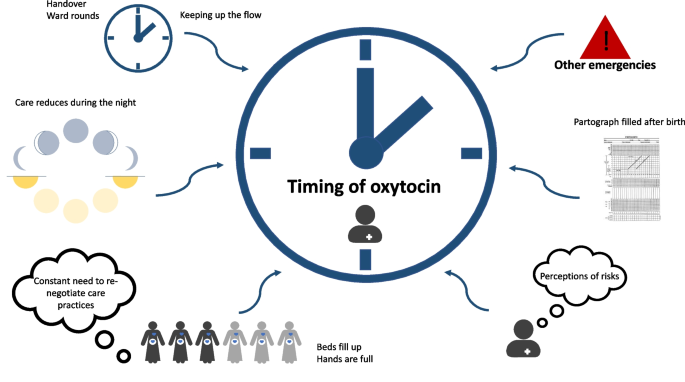
Conceptual framework on how time factors shape practices around oxytocin for labour augmentation
Use of oxytocin was often not based on labour progression or partograph. Number of women and staff, time of the day, ward-rounds and shifts turn-over created cycles with moments where oxytocin use accelerated, sometimes leaving all women on a drip, while at other times, when the ward was too busy or during nighttime, oxytocin was given too late. Perceptions related to risks of prolonged labour and oxytocin further facilitated its use
Time-pressure: the reality of an urban congested labour ward
“ It was busy, everyone was running around. Nobody kept an overview, it was impossible because the women were everywhere, constantly crying for help: “Nurse, nurse, nurse, come”. It was stressful and overwhelming. Everyone did their best and all hands were in use. Two women were pushing with assistance from students, a woman had just given birth but there was no time to clean up and help her get settled with her newborn. A foetal head was crowning in bed three, but there was no birth attendant to assist. In another bed the drip with oxytocin was running, the woman unattended. An assistant was running with birth trays and medication for prevention of haemorrhage. There were not enough suturing sets, so the intern doctor struggled to repair a bleeding tear. All hands were in use, care was limited to what was most necessary. After a few hours the labour ward became less chaotic. Exhaustion filled the room. Now the paperwork had to be filled, stocks recharged and curtains and floors cleaned for blood. Until the next wave of women filled the beds. ” (Observation notes 01.04.21).
This extract describes the extreme time scarcity in the labour ward, weighing heavily on the birth attendants, who often referred to the inability to do what was required. Time scarcity in the congested labour ward was a dominating theme throughout the study and explained to cause neglect and harm: “ It often happened that women were here for days because there was no overview of the too many women. Then we had bad outcomes, it was always like that ” (Respondent (R) 2). Neglected prolonged labour appeared to have become a symbol of the dangerously congested and time-pressured labour ward. Thus, tackling prolonged labour had become a key strategy both at management levels and in day-to-day clinical work.
Time scarcity also influenced the ability to use the partograph. Although the partograph was viewed as a useful tool by birth attendants, it was rarely used for decision-making to time labour augmentation. For example, during observations, not all vaginal examinations were plotted on the partograph: “ The nurse did not record the vaginal examination. She explained that she was not supposed to do an examination before four hours, so therefore it would be wrong to write it on the partograph (since only two hours had passed) ” (observation notes 27.03.21). Correct filling of the partograph required four-hourly examinations and half-hourly monitoring, which was often impossible. Rather than using it for decision-making, staff filled the partograph so they could not be accused of mismanagement. It was, therefore, not unusual to see nurses meticulously filling it after the woman had given birth, with FHR and contractions that they never assessed.
As Table 1 illustrates, safe and timely administration of oxytocin for labour augmentation would require one-to-one care. In this congested labour ward, one to three birth attendants had to look after up to 16 women simultaneously while also having many other tasks. Consequently, guidelines were largely unattainable and therefore constantly, either deliberately or subconsciously, negotiated and prioritised to manage in time.
Re-negotiating time: how birth attendants navigate in a busy urban labour ward
Birth attendants had found their own ways to deal with the time-pressured labour ward. This section details their time management strategies.
It was generally understood that oxytocin reduces labour duration and therefore resulted in a faster flow of labouring women through the ward. Use of oxytocin for labour augmentation was, therefore, a way to manage time by decongesting the labour ward. The necessity of applying oxytocin when the labour ward got busy and beds started to fill up, was the most frequently mentioned reason for applying oxytocin. As one birth attendant explained “ Oxytocin can be dangerous, but I believe that it is more dangerous if we have a congested labour ward where staff cannot monitor the women. We used to see much neglect at these times ” (R4). In this way, applying oxytocin was perceived as a tool to negotiate time to provide better care by shortening the duration of labour. As a response to the harmful congestion, the hospital had made an internal standard operating guideline by which nulliparous women in active labour should give birth within eight hours and multiparous women within six hours. This guideline was unanimously viewed as helpful by birth attendants. Even though limits were flexible, this practice shows that negotiation of time had become interwoven into hospital procedures, ensuring that birth attendants would keep a steady flow of birthing women.
Efforts to manage time also resulted in the dropping of several time-consuming clinical practices. For instance, structured observations showed that only 3/42 women (7.1%) had contractions and 5/42 (11.9%) had FHR measured when receiving oxytocin for augmentation (Table 4 ). One birth attendant explained: “ How is it possible to monitor contractions for ten minutes when we have so many women in labour ” (R1). Also, titration of oxytocin was often neglected. Most birth attendants knew that oxytocin had to be titrated and detailed titration plans were commonly observed in case-notes. Birth attendants had no explanation as to why it did not happen. As one doctor said “ I have never seen anyone titrating. You know it from school, but in hospitals, no, it doesn’t happen… ” (R12). Noteworthy, some birth attendants claimed to titrate oxytocin, but were rarely observed to do so. Instead, unstructured observations showed that oxytocin drips were often almost blindly (without counting drops) increased or decreased without reference to contractions and FHR. Limited titration could reflect how cumbersome this practice was without electronic infusion pumps in a busy labour ward. These deviations from clinical guidelines illustrate how birth attendants tinkered with practices around oxytocin administration to navigate time-pressure.
Birth attendants also navigated the, at times, overwhelmingly busy and unpredictable environment by adhering to the ‘natural labour ward cycles’ instead of using time-demanding surveillance cycles of individual women with reference to their partograph. Hence, labour progression was assessed mainly during ward rounds (10–12 am) and around handovers between shifts (7–8 am and 2–3 pm) or on demand (e.g., a birthing woman starting to push or a filled labour ward). It was observed on several occasions that particularly doctors were action-oriented in their management during ward rounds. They often ordered obstetric interventions (e.g., oxytocin) to enhance labour despite uncomplicated progression. When asked, one doctor explained: “ Because it can become very busy as the day progresses, we never know when we are able to come back. So many hours can pass before someone is checking the woman. If I come in the morning and again in the afternoon, I know that the women who are still here have been there too long ” (R7). This illustrates how birth attendants had found a system to navigate time and avoid delaying care.
Too early interventions also happened, because birth attendants were encouraged to hand over a “clean” labour ward (referring to a less crowded ward) for the next shift: “ We always tell them to make sure the women in their shift give birth. That is their responsibility, these women. This is how we avoid women are there during one, two or three shifts ” (R12). According to the staff, this was a helpful division because it ensured that women would not stay during several shifts. These are examples of how attempts to manage time pressure were built into existing systems. On other occasions, these natural cycles were broken because it was too busy and women were at risk of delayed care. In the morning, it sometimes happened that women had been in labour overnight without receiving appropriate interventions. For example, one woman progressed 2 cm over 17 h, without the membranes being ruptured or oxytocin given. The following day she gave birth two hours after augmentation with oxytocin was started. Surprisingly, delayed care also happened during calm moments, where care tended to be more passive and less action-oriented: “ There was only one woman in labour. The birth attendant was on his/her phone while another was sleeping. I knew that time for vaginal examination was overdue, but nobody seemed to notice ” (field notes, 28.3.21). This illustrates how the natural labour ward cycles had replaced structured timekeeping even when there was time.
An up-tempo labour ward
Table 4 presents data from 234 structured labour observations and includes background characteristics and birth outcomes. Data will be presented in this section to triangulate the qualitative findings on how oxytocin was used for labour augmentation.
The urge to speed up labour resulted in 146/234 (62.4%) women in active labour receiving oxytocin for labour augmentation (Table 4 ). Of a subgroup of women augmented in the first stage of labour, 21/48 (43.8%) had labour progressing faster than 0.5 cm per hour at the time oxytocin was applied and 5/48 (10.4%) faster than 1 cm per hour. International clinical guidelines recommend that vaginal examination should take place no more than four-hourly in uncomplicated labour. We observed, however, that 34/53 (64.1%) women received oxytocin within four hours of active labour and 15/53 (28.3%) even within two hours (Table 4 ).
Exploring substandard monitoring of women on oxytocin revealed that birth attendants had little awareness of the risks associated with oxytocin. Birth attendants used expressions like “ Why not speed up labour, if you can ” (R4) and referred to oxytocin as a way of helping women give birth faster. One birth attendant described the events of a woman with a stillborn baby: “ He [the doctor] even added an extra bottle of oxytocin [to help the baby] ” (R5). This showed the perceived harmlessness of oxytocin, which is crucial in understanding why injudicious use had developed. In contrast, experiences related to delay in care seemed to have a strong impact on birth attendants. They had daunting memories from the past when the labour ward had been busier, with women presenting from home whilst having been in labour for several days and with a dead foetus in utero. When adverse outcomes happened, prolonged labour was seen as a determinant while oxytocin was considered helpful. These perceptions persisted even though experiences of such horrifying delays mostly belonged to the past. In fact, during the structured labour observations, prolonged labour was a rare event: only 9/214 (4.2%) women were in active labour for more than 12 h, while 166/214 (77.6%) were in active labour for less than 6 h (Table 4 ). These findings crucially show how efforts to bring flow to the labour ward and avoid prolonged labour had been successful, but to an extent where oxytocin was given even in the absence of prolonged labour largely detached from individual women’s needs. What remains to be explored is what the consequences of such “up-tempo” labour ward management are in terms of caesarean sections and foetal outcomes. Four of five perinatal deaths happened in the oxytocin group (Table 4 ). It is, however, beyond the scope of this study to assess causal associations.
In the study’s high-volume, urban maternity ward with severe time scarcity and shortage of staff, birth attendants were forced to renegotiate care practices. Of the 234 women in active labour, 146 (62.4%) received oxytocin for labour augmentation, most often despite uncomplicated labour progress. Several time factors influenced oxytocin use (Fig. 1 ). The strongest driver was to fasten labour when the ward became too busy or to prevent overcrowding. In the absence of electronic monitoring devices, many women received oxytocin at unregulated doses with little monitoring. In many ways, birth attendants found augmentation of labour with oxytocin a preferred choice: it was perceived to prevent congestion and responded to the fear of prolonged labour, while there appeared to be little focus on potential oxytocin-mediated risks. Importantly, among the 234 observed women, only nine were in active labour for > 12 h (4.2%) and prolonged labour was a rare complication. This illustrates how efforts to avoid dangerous congestion and delayed care had been successful, but consequently resulted in overuse of oxytocin with little reference to individual women’s needs.
Navigating time-pressure in maternity care globally
Time pressure is not unique to this labour ward. Hospital environments globally face increasing demands for providing constantly better care without a simultaneous increase in resources [ 27 ]. High-volume understaffed hospitals are increasingly common in cities of LLMIC as urbanisation continues, and communities increasingly respond to the push for facility births [ 16 , 28 ]. As reported from Egypt and India, we argue that overburdened time-pressured mega maternity units are a crucial driver of the overuse of oxytocin and likely a driver of urban disadvantages in maternal health [ 28 ]. Reporting a 50% deficiency in health workforce, literature increasingly reports poor health outcomes in Dar es Salaam, one of the fastest-growing cities of the world [ 29 ]. This emphasises, that simply training birth attendants better will not solve overmedicalisation caused by underfunded health care systems. Referring to “tricks of the trade”, Celine et al. emphasised how medical doctors in Switzerland avoided time-consuming practices, just as birth attendants did in our study [ 30 ]. They explain how everything that takes time was seen as ‘bumps on the road’. Although effective, if time management becomes the end goal, unintended, less obvious consequences may occur. Oxytocin overuse is an example of an unintended side effect of navigating time pressure. Oxytocin has been shown to reduce labour by two hours. While such a reduction in labour duration may not seem crucial for the individual, administering oxytocin to many women could save time for care and bed space (if not considering the increased need for monitoring). This explains why excess oxytocin can be used as a time management strategy [ 11 , 13 , 31 ]. Birth attendants in our study had been successful in managing congestion and avoiding delayed care, but at a price of overmedicalisation. Recognising that increasing staff-patient ratios must be seen as a central part of the solution in a human resource crisis is therefore important.
With increasing urbanisation and the formation of mega-cities, such as Dar es Salaam, the time lens could become a crucial tool to understand care provision in these settings more broadly. This requires investigating time scarcity including health provider-to-patient ratio and the gap between guidelines and reality, as well as strategies that health providers use to navigate time pressure.
Normalisation of deviance
While time pressure appeared to be a key driver of overusing oxytocin for labour augmentation, practices remained, even when the labour ward was calm. This emphasises the complexity of deviations from the clinical guidelines [ 32 ]. Drawing on previous literature which describes the process of normalisation of deviance, three factors appear central: 1) unrealistic clinical guidelines and structures requiring deviation, 2) low alertness to harmful effects and 3) limited accountability measures [ 33 ]. These factors are elaborated in the context of labour augmentation with oxytocin [ 34 ].
First, the current guidelines for oxytocin use are cumbersome and not made for settings with a shortage of staff and no electronic devices to dose or monitor oxytocin. Therefore, birth attendants have to find alternative ways that work for them. Adaptations happen all the time in complex, imperfect hospital settings, but as Freedman emphasises, more commonly in low-resource settings [ 35 , 36 ]. The further away from reality clinical guidelines are, the harder they are to adapt, and adaptations may become unintentionally harmful [ 37 ]. When adapted, evidence from which the guidelines were originally based, may no longer be applicable. Deviant practices often start with small changes that move further away from the intended use [ 33 ]. It is likely that the current deviations in this labour ward are a result of many years of adjustment to meet continuous congestion with constantly fewer resources [ 16 ].
Second, the scarcity of evidence of adverse effects results in low alertness toward oxytocin [ 3 ]. Collectively, because of the bad experiences with prolonged labour, clinicians blamed labour duration rather than oxytocin when adverse events occurred. Behavioural scientist Soyer and psychologist Hogart unfold this well-known phenomenon in their article “Fooled by experience” [ 38 ]. Additionally, RCTs including oxytocin were small and not powered to investigate rare events which further downplays the risk of oxytocin [ 1 , 39 , 40 , 41 , 42 , 43 , 44 ].
Finally, the low focus on substandard, unrestricted and unaudited use reflects the limited accountability for oxytocin. In contrast, birth attendants were held accountable for perfectly filled partographs, where prolonged labour was not accepted. Meanwhile, oxytocin was freely available and mostly in stock in the fridge next to the labour ward. While this may increase life-saving oxytocin for third stage of labour, it may have facilitated liberal use during labour. Establishing accountability measures that hold birth attendants accountable for realistic oxytocin guidelines based on the partograph as a decision, and not a liability tool, thus, seems crucial.
Improving the use of oxytocin in this labour ward requires de-normalising its use. Consequently, we recommend addressing the congestion of urban high-volume labour wards, as this fundamentally sustains excessive oxytocin use. We further emphasise the sensitisation of oxytocin as a high-alert medication, while global evidence is crucially needed to unfold the risk profile and long-term effects of oxytocin stratified in low-, middle- and high-income countries. Meanwhile, the development of realistic oxytocin guidelines for low-resource settings is crucial for providing safer use.
A few studies from LLMIC have succeeded in reducing oxytocin use. A Tanzanian study decreased labour augmentation with oxytocin from 22 to 12% after implementing context-adjusted guidelines co-created with frontline health workers and three-monthly seminars (the PartoMa intervention) [ 7 , 45 ]. The guideline restricted oxytocin to women crossing the partograph’s action line [ 7 ]. An Indian study reported a reduction in oxytocin from 78 to 32% after implementing a coaching-based intervention using the WHO childbirth checklist which prohibits routine oxytocin for labour augmentation altogether [ 31 ]. Oxytocin, however, increased to 48% six months after the intervention had ended [ 31 ]. Finally, a study from a Palestinian hospital reported a decrease in the use of oxytocin for labour augmentation from 32.1 to 10.9% after implementing a multifaceted intervention [ 46 ]. The intervention consisted of on-the-job training 2–3 days a week, audit of practices, feedback during interactive audit meetings every 2–3 months, five training workshops and informal meetings with managers and midwives to understand barriers and providers’ opinions of the intervention [ 46 ]. Two years post-implementation the oxytocin rate, however, increased to 17% [ 46 ]. None of these interventions negatively affected caesarean section rates or birth outcomes. Concludingly, it appears that reducing use of oxytocin for labour augmentation is possible through clinical guidelines and training but contextual factors that withhold its use, such as crowded labour wards, must be tackled to make changes sustainable.
Strengths and limitations
Our mixed-methods design allowed a deeper understanding of the mechanisms which shape practices around labour augmentation with oxytocin. The primary researcher’s position as an “outsider” with obstetric experience from another setting and our diverse author group appeared crucial in recognizing and reflecting on deviant normalized practices. BSD, MA and DN have in-depth clinical experience from the study hospital ensuring findings were recognizable. Translated transcripts have limitations since nuances may have been missed in the translation process. Observations may be subject to the Hawthorne effect where birth attendants performed better than they would usually. Though assistants were not trained obstetricians and had no reason to over- or underestimate oxytocin observer’s bias cannot be ruled out. We included only women with low-risk pregnancies and quantitative findings are therefore comparable to this population primarily. Underlying perceptions and culture are specific to this labour ward with limited generalizability. Our findings are of interest to other LLMICs where congestion is an issue. Rural hospitals may experience underuse of oxytocin due to absence of staff, lack of storage capacity, stock-outs of oxytocin or low quality of oxytocin. Findings, however, are of global health concern, as unindicated oxytocin use is also increasingly observed in HIC [ 43 , 47 , 48 , 49 ].
In this labour ward, almost two out of three women in active labour received oxytocin for labour augmentation, most with uncomplicated labour progress and limited monitoring and titration. Overcrowding appeared to be the strongest driver, forcing birth attendants to keep up the flow in the busy labour ward by using oxytocin as a decongestion tool. Low alertness towards oxytocin-mediated risks, along with fear of prolonged labour, further facilitated liberal oxytocin use. Evidence-based, context-adjusted training and guidelines for prolonged labour management in time-pressured labour wards are required to avoid medically unindicated use of oxytocin. Further, myths and perceptions must be addressed to de-normalise its use. With increasing urbanisation, facility births and the formation of mega-cities, the time lens could become a crucial tool to understand the effect of time pressure and congestion on care practices in low-resource settings more broadly.
Availability of data and materials
The datasets are not publicly available due to privacy of respondents, but are available from the corresponding author on reasonable request.
Abbreviations
Low- and lower-middle-income countries
Low-income countries
High-income countries
Fetal heart rate
World Health Organisation
Bugg GJ, Siddiqui F, Thornton JG. Oxytocin versus no treatment or delayed treatment for slow progress in the first stage of spontaneous labour. Cochrane Database Syst Rev. 2013;6:CD007123.
Google Scholar
National Institute for Health and Care Excellence (NICE). Intrapartum care for healthy women and babies. NICE Clinical Guidelines. 2022; No 190.
Kujabi ML, Mikkelsen E, Housseine N, Obel J, D’Mello BS, Meyrowitsch DW, et al. Labor augmentation with oxytocin in low- and lower-middle-income countries: a systematic review and meta-analysis. AJOG global reports. 2022;2:100123.
Article PubMed PubMed Central Google Scholar
Burgod C, Pant S, Morales MM, Montaldo P, Ivain P, Elangovan R, et al. Effect of intra-partum oxytocin on neonatal encephalopathy: a systematic review and meta-analysis. BMC Pregnancy Childbirth. 2021;21:736.
World Health Organisation. WHO recommendations for augmentation of labour. WHO library; 2014. ISBN 978 92 4 150736 3.
World Health Organization. World health organization partograph in management of labour. Lancet. 1994;343:1399–404.
Maaløe N, Housseine N, Meguid T, Nielsen BB, Jensen AKG, Khamis RS, et al. Effect of locally-tailored labour management guidelines on intrahospital stillbirths and birth asphyxia at the referral hospital of Zanzibar: a quasi-experimental pre-post-study (the PartoMa study). BJOG. 2018;125:235–45.
Article PubMed Google Scholar
Deepak NN, Mirzabagi E, Koski A, Tripathi V. Knowledge, attitudes, and practices related to uterotonic drugs during childbirth in Karnataka, India: a qualitative research study. PLoS One. 2013;8:e62801.
Article CAS PubMed PubMed Central Google Scholar
Mirzabagi E, Deepak NN, Koski A, Tripathi V. Uterotonic use during childbirth in Uttar Pradesh: accounts from community members and health providers. Midwifery. 2013;29:902–10.
Koski A, Mirzabagi E, Cofie P, Tripathi V. Uterotonic use at childbirth in Ghana: a qualitative study of practices, perceptions, and knowledge among facility-based health care providers and community members. Int J Childbirth. 2014;4:25–38.
Article Google Scholar
Van Hollen C. Invoking vali: painful technologies of modern birth in south India. Med Anthropol Q. 2003;17:49–77.
Forna F, Titulaer P, Sesay S, Conteh S, Muoghalu S, Kanu P, et al. Prevalence of use of highly concentrated oxytocin or “pepper injection” in labor among clinicians undergoing emergency obstetric training in Sierra Leone. Int J Gynecol Obstet. 2020;151:450–5.
Khalil K, Cherine M, Elnoury A, Sholkamy H, Breebaart M, Hassanein N. Labor augmentation in an Egyptian teaching hospital. Int J Gynaecol Obstet. 2004;85:74–80.
Sørensen JB, Housseine N, Maaløe N, Bygbjerg IC, Pinkowski Tersbøl B, Konradsen F, et al. Scaling up locally adapted clinical practice guidelines for improving childbirth care in Tanzania: a protocol for programme theory and qualitative methods of the PartoMa scale-up study. Glob Health Action. 2022;15:2034136.
Maaløe N, Housseine N, Sørensen JB, Obel J, Sequeira DMello B, Kujabi ML, et al. Scaling up context-tailored clinical guidelines and training to improve childbirth care in urban, low-resource maternity units in Tanzania: a protocol for a stepped-wedged cluster randomized trial with embedded qualitative and economic analyses (the PartoMa scale-up study). Glob Health Action. 2022;15:2034135.
Sequeira Dmello B, Sellah Z, Magembe G, Housseine N, Maaløe N, Van Den Akker T, et al. Learning from changes concurrent with implementing a complex and dynamic intervention to improve urban maternal and perinatal health in Dar es Salaam, Tanzania, 2011–2019. BMJ Glob Health. 2021;6:e004022.
Dmello BS, Housseine N, van den Akker T, van Roosmalen J, Maaløe N. Impact of COVID-19 on maternal and child health. Lancet Glob Health. 2020;8:e1259.
Miltenburg AS, Kiritta RF, Meguid T, Sundby J, et al. Quality of care during childbirth in Tanzania: identification of areas that need improvement. Reprod Health. 2018;15:14.
Housseine N, Punt MC, Mohamed AG, Said SM, Maaløe N, Zuithoff NPA, et al. Quality of intrapartum care: direct observations in a low-resource tertiary hospital. Reprod Health. 2020;17:1–11.
Maaløe N, Housseine N, Bygbjerg IC, Meguid T, Khamis RS, Mohamed AG, et al. Stillbirths and quality of care during labour at the low resource referral hospital of Zanzibar : a case-control study. BMC Pregnancy Childbirth. 2016;16:1–12.
Adam B. Of timescapes, futurescapes and timeprints. Lüneburg Talk Web. 2008;070708:1–9.
World Health Organization. Managing complications in pregnancy and childbirth: a guide for midwives and doctors – 2nd ed. Geneva; 2017. ISBN 978-92-4-156549-3
The United Republic of Tanzania, ministry of health, community development, gender, elderly and children. Clinical practice guidelines on appropriate use of uterotonics: for post-abortion care, induction of labour, prevention and treatment of postpartum haemorrhage. 2019.
Médecins Sans Frontières. Essential obstetric and newborn care practical guide for midwives, doctors with obstetrics training and health care personnel who deal with obstetric emergencies. 2019.
World Health Organization. WHO labour care guide: user’s manual. Geneva; 2020.
Attride-Stirling J. Thematic networks: an analytic tool for qualitative research. Qual Res. 2001;1:385–405.
How can health services keep pace with the rapid growth of cities? | Healthcare Network | The Guardian. https://www.theguardian.com/sustainable-business/2017/feb/24/how-can-health-services-keep-pace-with-the-rapid-growth-of-cities . Accessed 12 Dec 2022.
Save the Children. The urban disadvantage: state of the world’s mothers. 2015.
Norris M, Klabbers G, Pembe AB, Hanson C, Baker U, Aung K, et al. A growing disadvantage of being born in an urban area? Analysing urban–rural disparities in neonatal mortality in 21 African countries with a focus on Tanzania. BMJ Glob Health. 2022;7:e007544.
Céline B, Matteo M, Michael S, Friedrich S, Vanessa K, David G, et al. Running against the clock: a qualitative study of internal medicine residents’ work experience. Swiss Med Wkly. 2022;152:w30216.
Marx Delaney M, Kalita T, Hawrusik B, Neal B, Miller K, Ketchum R, et al. Modification of oxytocin use through a coaching-based intervention based on the WHO safe childbirth checklist in Uttar Pradesh, India: a secondary analysis of a cluster randomised controlled trial. BJOG. 2021;128:2013–21.
Article CAS PubMed Google Scholar
Baartmans MC, Hooftman J, Zwaan L, van Schoten SM, Erwich JJHM, Wagner C. What can we learn from in-depth analysis of human errors resulting in diagnostic errors in the emergency department: an analysis of serious adverse event reports. J Patient Saf. 2022;18:e1135–41.
Price MR, Williams TC. When doing wrong feels so right: normalization of deviance. J Patient Saf. 2018;14:1–2.
Clark SL, Simpson KR, Knox GE, Garite TJ. Oxytocin: new perspectives on an old drug. Am J Obstet Gynecol. 2009;200:35.e1-35.e6.
The elusive quest: accountability in hospitals - Carolyn L. Wiener. https://books.google.dk/books?hl=da&lr=&id=MoeeDXObhk8C&oi=fnd&pg=PR11&ots=2Cg1RukT7F&sig=qCtxYnObmbTTmhb_8JI_hN10AMg&redir_esc=y#v=onepage&q&f=false . Accessed 28 Nov 2022.
Freedman LP. Implementation and aspiration gaps: whose view counts? Lancet. 2016;388:2068–9.
Maaløe N, Ørtved A, Sørensen J, Sequeira Dmello B, van den Akker T, Kujabi M, et al. The injustice of unfit clinical practice guidelines in low-resource realities. Lancet Glob Health. 2021;9:e875–9.
Soyer E, Hogarth R. Fooled by experience. Harv Bus Rev. 2015;May:72–7.
Berglund S, Grunewald C, Pettersson H, Cnattingius S. Severe asphyxia due to delivery-related malpractice in Sweden 1990–2005. BJOG. 2008;115:316.
Simpson K, James D. Effects of oxytocin-induced uterine hyperstimulation during labor on fetal oxygen status and fetal heart rate patterns. Am J Obstet Gynecol. 2008;199:34.e1-34.e5.
Jonsson M, Nordén S, Hanson U. Analysis of malpractice claims with a focus on oxytocin use in labour. Acta Obstet Gynecol Scand. 2007;86:315–9.
Milsom I, Ladfors L, Thiringer K, Niklasson A, Odeback A, Thornberg E. Influence of maternal, obstetric and fetal risk factors on the prevalence of birth asphyxia at term in a Swedish urban population. Acta Obstet Gynecol Scand. 2002;81:909–17.
Dalbye R, Bernitz S, Olsen IC, Zhang J, Eggebø TM, Rozsa D, et al. The labor progression study: the use of oxytocin augmentation during labor following Zhang’s guideline and the WHO partograph in a cluster randomized trial. Acta Obstet Gynecol Scand. 2019;98:1187–94.
Rossen J, Østborg T, Lindtjørn E, Schulz J, Eggebø T. Judicious use of oxytocin augmentation for the management of prolonged labor. Acta Obstet Gynecol Scand. 2016;95:355–61.
Maaløe N, Meguid T, Housseine N, Kragelund Nielsen K, van Roosmalen JJM, Bygbjerg IC, et al. Locally-adapted intrapartum clinical guidelines in Zanzibar’s tertiary hospital, United Republic of Tanzania. Lessons from the field. Bull World Health Organ. 2019;97:365–70.
Hassan S, Sundby J, Husseini A. Translating evidence into practice in childbirth: a case from the occupied Palestinian territory. Women and Birth. 2013;26:e82–9.
Selin L, Almström E, Wallin G, Berg M. Use and abuse of oxytocin for augmentation of labor. Acta Obstet Gynecol Scand. 2009;88:1352–7.
Gaudernack LC, Frøslie KF, Michelsen TM, Voldner N, Lukasse M. De-medicalization of birth by reducing the use of oxytocin for augmentation among first-time mothers - a prospective intervention study. BMC Pregnancy Childbirth. 2018;18:1–9.
Girault A, Blondel B, Goffinet F, le Ray C. Frequency and determinants of misuse of augmentation of labor in France: a population-based study. PLoS One. 2021;16:e0246729.
Download references
Acknowledgements
Dorkasi L. Mwakawanga, Assistant Lecturer, School of Nursing-Muhimbili University of Health and Allied Sciences.
Bavelyne Machumu, Kilimanjaro Clinical Research Institute.
Siah Hakim Nyange, Management and Development for Health—Temeke Regional Referral Hospital.
Funding was obtained from: Danida Research Fellowship Centre, Laerdal Foundation, Thorvald Madsens Legat, Bodil Pedersen Fonden and Reinholt W. Jorcks Fond.
The funding sources had no role in the design of the study and collection, analysis, and interpretation of data and in writing the manuscript.
Author information
Authors and affiliations.
Global Health Section, Department of Public Health, University of Copenhagen, Copenhagen, Denmark
Monica Lauridsen Kujabi, Nanna Maaløe, Brenda Sequeira D’mello, Flemming Konradsen & Jane Brandt Sørensen
Department of Gynaecology and Obstetrics, Aarhus University Hospital Skejby, Aarhus, Denmark
Monica Lauridsen Kujabi
Department of Gynaecology and Obstetrics, Mwanyanamala Regional Referral Hospital, Dar es Salaam, Tanzania
Luzango Maembe
Department of Gynaecology and Obstetrics, Morogoro Regional Referral Hospital, Morogoro, Tanzania
Daniel Nkungu
Department of Gynaecology and Obstetrics, Copenhagen University Hospital Hvidovre, Copenhagen, Denmark
Nanna Maaløe
Medical College East Africa, Aga Khan University, Dar es Salaam, Tanzania
Brenda Sequeira D’mello & Kidanto Hussein
CCBRT Hospital, Dar es Salaam, Tanzania
Brenda Sequeira D’mello
Athena Institute, VU University Amsterdam, Amsterdam, the Netherlands
Jos van Roosmalen & Thomas van den Akker
Department of Obstetrics and Gynaecology, Leiden University Medical Center, Leiden, the Netherlands
Novo Nordisk Foundation, Hellerup, Denmark
Flemming Konradsen
School of Nursing and Midwifery East Africa, Aga Khan University, Dar es Salaam, Tanzania
Eunice Pallangyo
Section of Health Services Research, Department of Public Health, University of Copenhagen, Copenhagen, Denmark
Morten Skovdal
You can also search for this author in PubMed Google Scholar
Contributions
MLK, JBS, MS, EP, NM, TvdA and FK contributed to the conception and design of the work. MLK, MS, JBS and DN contributed to the acquisition, analysis, interpretation of data. MLK drafted the manuscript. All authors substantively revised the draft. All authors have approved the submitted version (and any substantially modified version that involves the author's contribution to the study). All authors have agreed both to be personally accountable for the author’s own contributions and to ensure that questions related to the accuracy or integrity of any part of the work, even ones in which the author was not personally involved, are appropriately investigated, resolved, and the resolution documented in the literature.
Corresponding author
Correspondence to Monica Lauridsen Kujabi .
Ethics declarations
Ethics approval and consent to participate.
All respondents for interview gave their written informed consent. Informed verbal consent was obtained from women and staff during observations. The research project, including verbal consent, received ethical approval from the ethics committee of the Tanzanian National Institute for Medical Research (NIMR) through the Tanzanian National Health Research Ethics Review Sub-Committee (NatHREC) (NIMR/HQ/R.8a/Vol. IX/3324) (approval of protocol) and NIMR/HQ/P.12VOLXXXV/111 (permission to publish). Approvals were obtained from the Ministry of Health, Tanzania and facility management. Methods were performed in accordance with the Declaration of Helsinki.
Consent for publication
Not applicable.
Competing interests
The authors declare that they have no competing interests, apart from Jos van Roosmalen, who is a senior board member of BMC Pregnancy Childbirth.
Additional information
Publisher’s note.
Springer Nature remains neutral with regard to jurisdictional claims in published maps and institutional affiliations.
Supplementary Information
Supplementary material 1., supplementary material 2., rights and permissions.
Open Access This article is licensed under a Creative Commons Attribution-NonCommercial-NoDerivatives 4.0 International License, which permits any non-commercial use, sharing, distribution and reproduction in any medium or format, as long as you give appropriate credit to the original author(s) and the source, provide a link to the Creative Commons licence, and indicate if you modified the licensed material. You do not have permission under this licence to share adapted material derived from this article or parts of it. The images or other third party material in this article are included in the article’s Creative Commons licence, unless indicated otherwise in a credit line to the material. If material is not included in the article’s Creative Commons licence and your intended use is not permitted by statutory regulation or exceeds the permitted use, you will need to obtain permission directly from the copyright holder. To view a copy of this licence, visit http://creativecommons.org/licenses/by-nc-nd/4.0/ .
Reprints and permissions
About this article
Cite this article.
Kujabi, M.L., Maembe, L., Nkungu, D. et al. Temporalities of oxytocin for labour augmentation: a mixed-methods study of time factors shaping labour practices in a busy maternity unit in Tanzania. BMC Pregnancy Childbirth 24 , 563 (2024). https://doi.org/10.1186/s12884-024-06717-3
Download citation
Received : 08 February 2023
Accepted : 23 July 2024
Published : 29 August 2024
DOI : https://doi.org/10.1186/s12884-024-06717-3
Share this article
Anyone you share the following link with will be able to read this content:
Sorry, a shareable link is not currently available for this article.
Provided by the Springer Nature SharedIt content-sharing initiative
- Prolonged labour
- Low-resource
- Augmentation
- Intrapartum
- Time-pressure
- Urbanisation
BMC Pregnancy and Childbirth
ISSN: 1471-2393
- Submission enquiries: [email protected]
- General enquiries: [email protected]
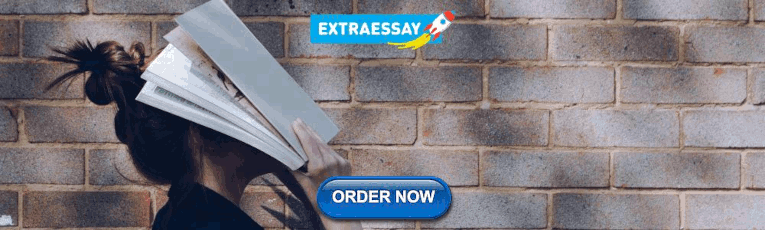
IMAGES
VIDEO
COMMENTS
Techniques designed to combine the results of qualitative and quantitative studies can provide researchers with more knowledge than separate analysis Health researchers are increasingly using designs that combine qualitative and quantitative methods, and this is often called mixed methods research.1 Integration—the interaction or conversation between the qualitative and quantitative ...
Mixed Methods Meta-analysis. Mixed methods meta-analysis involves the systematic review and synthesis of findings from multiple studies that use mixed methods designs. This involves combining quantitative and qualitative data from multiple studies to gain a broader understanding of a research question.
It has been argued that mixed methods research can be useful in nursing and health science because of the complexity of the phenomena studied. However, the integration of qualitative and quantitative approaches continues to be one of much debate and there is a need for a rigorous framework for designing and interpreting mixed methods research.
Abstract The purpose of this methodological article is to provide a primer for conducting a mixed analysis—the term used for analyzing data in mixed research. Broadly speaking, a mixed analysis involves using quantitative and quantitative data analysis techniques within the same study.
Mixed methods research combines elements of quantitative research and qualitative research in order to answer your research question. Mixed methods can help you gain a more complete picture than a standalone quantitative or qualitative study, as it integrates benefits of both methods. Mixed methods research is often used in the behavioral ...
Mixed methods is NOT simply having quantitative and qualitative data available or analyzing and presenting data findings seperately. The integration process can occur during data collection, analysis, or presentation of results.
Mixed‐methods research, or multi‐strategy designs, 1 can be defined as 'the collection, analysis and integration of both qualitative and quantitative data in a single study': 2 semi‐structured interviews and workplace measures (e.g. attendance data) might be undertaken concurrently to gain a multifaceted perspective on a particular ...
This guide provides information and guidance for researchers interested in conducting qualitative data analysis. A guide to mixing qualitative and quantitative methods and techniques for social-science research.
Certainly they use "mixed methods data analyses" (Onwuegbuzie & Teddlie, 2003) consisting of both qualitative and quantitative data analysis, but the data col-lection procedure involves collecting only qualitative data (and not quantita-tive data).
Combining methods in social scientific research has recently gained momentum through a research strand called Mixed Methods Research (MMR). This approach, which explicitly aims to offer a framework for combining methods, has rapidly spread through the social and behavioural sciences, and this article offers an analysis of the approach from a field theoretical perspective. After a brief outline ...
A mixed methods research design is an approach to collecting and analyzing both qualitative and quantitative data in a single study. Mixed methods designs allow for method flexibility and can provide differing and even conflicting results. Examples of mixed methods research designs include convergent parallel, explanatory sequential, and ...
Quantitative dominant [or quantitatively driven] mixed methods research is the type of mixed research in which one relies on a quantitative, postpositivist view of the research process, while concurrently recognizing that the addition of qualitative data and approaches are likely to benefit most research projects. (p.
The large volume of data generated by mixed methods research can create challenges in analysis and dissemination. There are various alternatives for the presentation of mixed methods projects in the literature.
Abstract. This chapter covers the final three steps of the nine-step process for conducting mixed methods research in social work. It begins by describing how to prepare qualitative and quantitative data for analysis, then provides some helpful techniques for efficiently coding and analyzing qualitative data.
Integration in mixed methods can take multiple forms. Where integration is intentional, the mix of methods might occur through iteration, blending, nesting, or embedding or through serving a common substantive (holistic) or ideological (transformative) purpose (Greene, 2007). All mixed methods research involves, as a minimum, integrating conclu ...
Mixed methods research is becoming increasingly popular and is widely acknowledged as a means of achieving a more complex understanding of research problems. Combining both the in-depth, contextual views of qualitative research with the broader generalizations of...
Quantitizing qualitative data can serve as an additional step during data analysis to broaden the perspective and collect complementary views. Examples from research illustrate how information ...
Introduction. 'Mixed methods' is a research approach whereby researchers collect and analyse both quantitative and qualitative data within the same study. 1 2 Growth of mixed methods research in nursing and healthcare has occurred at a time of internationally increasing complexity in healthcare delivery.
The theory of change (Do -> Get) model behind integrative mixed methods analysis is very simple. It is that: (1) effectively combining more than one source or type of data and/or more than one approach to analysis will deliver a gain over using a single source, type of data, or approach to analysis, and (2) effective integration of sources and ...
Mixed methods research is a research method that combines and integrates qualitative and quantitative research methods in a single research study. It involves collecting and analyzing qualitative and quantitative data to understand a phenomenon better and answer the research questions.
I am pleased to offer this media review of the Sage Handbook of Mixed Methods Research Design edited by Dr Cheryl Poth.Dr Poth is a leading Canadian expert in the field of mixed methods research. Dr Poth is a Professor in the Faculty of Education and faculty member of the Centre for Applied Research in Assessment and Measurement in Education (CRAME) at the University of Alberta.
1. mixed methods that came with making a difference in research methods through mixing different data at the various levels of research. Multimethod started in the field of anthropology and program evaluation (Denzin 1978 and Cook 1985). This write up distinguishes between the two research methods as follows; Mixed Method Research Approach.
In this video, I talk about analyzing mixed methods research data, and I specifically focus on data analysis in mixed methods sequential designs (exploratory design and explanatory design), but I ...
In this paper, we return to this data for a second analysis to answer the following research questions: ... Only through utilizing an integrative mixed-methods approach in our secondary data analysis, we were able to account for the vast spectrum of double awareness in patient cases.
To address the multidimensional challenges faced by young adult cancer survivors, 20 young adult cancer survivors participated in a rehabilitation program. Mixed methods, including a longitudinal convergent parallel design, were employed to evaluate both process and outcome issues. Data were collected simultaneously via questionnaires, physical testing, and interviews at four separate time points.
Data analysis method. Survey data was analysed using the data analysis software package Statistical Package for the Social Sciences, version 26 (SPSS Inc., Chicago, Il, USA). ... particularly as the study was limited to two regions in Ireland. However, the addition of qualitative data in this mixed-methods study may have helped offset this ...
Research method. In recent years, bibliometrics has become one of the crucial methods for analyzing literature reviews and is widely used in disciplinary and industrial intelligence analysis (Jing ...
To demonstrate this, we applied both methods to resampled COVID-19 data 1 where the number of cells per cell type was artificially varied between 100 and 10,000. nDEG was highly confounded by the ...
Lack of recognition and validation for this attraction, including in the polyamorous community, may be negatively impacting those who experience symbiosexual attraction. I conducted an integrated mixed-methods analysis of secondary data from the 2023 The Pleasure Study to learn more about symbiosexual attraction.
Methods. Mixed-methods data collection was conducted from March 2021 to February 2022, including structured observations of 234 births, 220 h of unstructured labour ward observations and 13 individual in-depth interviews with birth attendants. Thematic network analysis and descriptive statistics were used to analyse data.