Thank you for visiting nature.com. You are using a browser version with limited support for CSS. To obtain the best experience, we recommend you use a more up to date browser (or turn off compatibility mode in Internet Explorer). In the meantime, to ensure continued support, we are displaying the site without styles and JavaScript.
- View all journals
- Explore content
- About the journal
- Publish with us
- Sign up for alerts
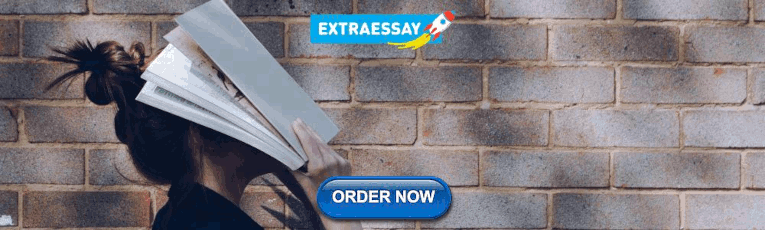
Image processing articles within Scientific Reports
Article 24 August 2024 | Open Access
Performance enhancement of deep learning based solutions for pharyngeal airway space segmentation on MRI scans
- Chattapatr Leeraha
- , Worapan Kusakunniran
- & Thanongchai Siriapisith
Article 23 August 2024 | Open Access
Machine learning approaches to detect hepatocyte chromatin alterations from iron oxide nanoparticle exposure
- Jovana Paunovic Pantic
- , Danijela Vucevic
- & Igor Pantic
Article 21 August 2024 | Open Access
An efficient segment anything model for the segmentation of medical images
- Guanliang Dong
- , Zhangquan Wang
- & Haidong Cui
Article 20 August 2024 | Open Access
A novel approach for automatic classification of macular degeneration OCT images
- Shilong Pang
- , Beiji Zou
- & Kejuan Yue
Article 18 August 2024 | Open Access
Subject-specific atlas for automatic brain tissue segmentation of neonatal magnetic resonance images
- Negar Noorizadeh
- , Kamran Kazemi
- & Ardalan Aarabi
Article 17 August 2024 | Open Access
Three layered sparse dictionary learning algorithm for enhancing the subject wise segregation of brain networks
- Muhammad Usman Khalid
- , Malik Muhammad Nauman
- & Kamran Ali
Article 14 August 2024 | Open Access
Development and performance evaluation of fully automated deep learning-based models for myocardial segmentation on T1 mapping MRI data
- Mathias Manzke
- , Simon Iseke
- & Felix G. Meinel
Article 13 August 2024 | Open Access
Haemodynamic study of left nonthrombotic iliac vein lesions: a preliminary report
- , Qijia Liu
- & Xuan Li
Cross-modality sub-image retrieval using contrastive multimodal image representations
- Eva Breznik
- , Elisabeth Wetzer
- & Nataša Sladoje
Article 11 August 2024 | Open Access
Effective descriptor extraction strategies for correspondence matching in coronary angiography images
- Hyun-Woo Kim
- , Soon-Cheol Noh
- & Si-Hyuck Kang
Article 10 August 2024 | Open Access
Lightweight safflower cluster detection based on YOLOv5
- , Tianlun Wu
- & Haiyang Chen
Article 08 August 2024 | Open Access
Primiparous sow behaviour on the day of farrowing as one of the primary contributors to the growth of piglets in early lactation
- Océane Girardie
- , Denis Laloë
- & Laurianne Canario
High-throughput image processing software for the study of nuclear architecture and gene expression
- Adib Keikhosravi
- , Faisal Almansour
- & Gianluca Pegoraro
Article 07 August 2024 | Open Access
Puzzle: taking livestock tracking to the next level
- Jehan-Antoine Vayssade
- & Mathieu Bonneau
Article 02 August 2024 | Open Access
The impact of fine-tuning paradigms on unknown plant diseases recognition
- Jiuqing Dong
- , Alvaro Fuentes
- & Dong Sun Park
Article 01 August 2024 | Open Access
AI-enhanced real-time cattle identification system through tracking across various environments
- Su Larb Mon
- , Tsubasa Onizuka
- & Thi Thi Zin
Article 31 July 2024 | Open Access
Study on lung CT image segmentation algorithm based on threshold-gradient combination and improved convex hull method
- Junbao Zheng
- , Lixian Wang
- & Abdulla Hamad Yussuf
Article 30 July 2024 | Open Access
A multibranch and multiscale neural network based on semantic perception for multimodal medical image fusion
- , Yinjie Chen
- & Mengxing Huang
Article 26 July 2024 | Open Access
Detection of diffusely abnormal white matter in multiple sclerosis on multiparametric brain MRI using semi-supervised deep learning
- Benjamin C. Musall
- , Refaat E. Gabr
- & Khader M. Hasan
The integrity of the corticospinal tract and corpus callosum, and the risk of ALS: univariable and multivariable Mendelian randomization
- , Gan Zhang
- & Dongsheng Fan
Article 23 July 2024 | Open Access
Accelerating photoacoustic microscopy by reconstructing undersampled images using diffusion models
- & M. Burcin Unlu
Article 20 July 2024 | Open Access
Automated segmentation of the median nerve in patients with carpal tunnel syndrome
- Florentin Moser
- , Sébastien Muller
- & Mari Hoff
Article 18 July 2024 | Open Access
Estimating infant age from skull X-ray images using deep learning
- Heui Seung Lee
- , Jaewoong Kang
- & Bum-Joo Cho
Article 17 July 2024 | Open Access
Finite element models with automatic computed tomography bone segmentation for failure load computation
- Emile Saillard
- , Marc Gardegaront
- & Hélène Follet
Article 16 July 2024 | Open Access
Deep learning pose detection model for sow locomotion
- Tauana Maria Carlos Guimarães de Paula
- , Rafael Vieira de Sousa
- & Adroaldo José Zanella
Article 15 July 2024 | Open Access
Deep learning application of vertebral compression fracture detection using mask R-CNN
- Seungyoon Paik
- , Jiwon Park
- & Sung Won Han
Article 11 July 2024 | Open Access
Morphological classification of neurons based on Sugeno fuzzy integration and multi-classifier fusion
- , Guanglian Li
- & Haixing Song
Preoperative prediction of MGMT promoter methylation in glioblastoma based on multiregional and multi-sequence MRI radiomics analysis
- , Feng Xiao
- & Haibo Xu
Article 09 July 2024 | Open Access
Noninvasive, label-free image approaches to predict multimodal molecular markers in pluripotency assessment
- Ryutaro Akiyoshi
- , Takeshi Hase
- & Ayako Yachie
Article 08 July 2024 | Open Access
A prospective multi-center study quantifying visual inattention in delirium using generative models of the visual processing stream
- Ahmed Al-Hindawi
- , Marcela Vizcaychipi
- & Yiannis Demiris
Article 06 July 2024 | Open Access
Advancing common bean ( Phaseolus vulgaris L.) disease detection with YOLO driven deep learning to enhance agricultural AI
- Daniela Gomez
- , Michael Gomez Selvaraj
- & Ernesto Espitia
Article 05 July 2024 | Open Access
Image processing based modeling for Rosa roxburghii fruits mass and volume estimation
- Zhiping Xie
- , Junhao Wang
- & Manyu Sun
On leveraging self-supervised learning for accurate HCV genotyping
- Ahmed M. Fahmy
- , Muhammed S. Hammad
- & Walid I. Al-atabany
A semantic feature enhanced YOLOv5-based network for polyp detection from colonoscopy images
- Jing-Jing Wan
- , Peng-Cheng Zhu
- & Yong-Tao Yu
Article 03 July 2024 | Open Access
DSnet: a new dual-branch network for hippocampus subfield segmentation
- , Wangang Cheng
- & Guanghua He
Quantification of cardiac capillarization in basement-membrane-immunostained myocardial slices using Segment Anything Model
- , Xiwen Chen
- & Tong Ye
Article 02 July 2024 | Open Access
Matrix metalloproteinase 9 expression and glioblastoma survival prediction using machine learning on digital pathological images
- , Yuan Yang
- & Yunfei Zha
Article 01 July 2024 | Open Access
Generalized div-curl based regularization for physically constrained deformable image registration
- Paris Tzitzimpasis
- , Mario Ries
- & Cornel Zachiu
Multi-branch CNN and grouping cascade attention for medical image classification
- , Wenwen Yue
- & Liejun Wang
Article 25 June 2024 | Open Access
Spatial control of perilacunar canalicular remodeling during lactation
- Michael Sieverts
- , Cristal Yee
- & Claire Acevedo
Deep learning-based localization algorithms on fluorescence human brain 3D reconstruction: a comparative study using stereology as a reference
- Curzio Checcucci
- , Bridget Wicinski
- & Paolo Frasconi
Article 24 June 2024 | Open Access
Tongue image fusion and analysis of thermal and visible images in diabetes mellitus using machine learning techniques
- Usharani Thirunavukkarasu
- , Snekhalatha Umapathy
- & Tahani Jaser Alahmadi
Article 22 June 2024 | Open Access
YOLOv8-CML: a lightweight target detection method for color-changing melon ripening in intelligent agriculture
- Guojun Chen
- , Yongjie Hou
- & Lei Cao
Article 21 June 2024 | Open Access
Performance evaluation of the digital morphology analyser Sysmex DI-60 for white blood cell differentials in abnormal samples
- , Yingying Diao
- & Hong Luan
Article 20 June 2024 | Open Access
Machine-learning-guided recognition of α and β cells from label-free infrared micrographs of living human islets of Langerhans
- Fabio Azzarello
- , Francesco Carli
- & Francesco Cardarelli
Article 10 June 2024 | Open Access
Fast and robust feature-based stitching algorithm for microscopic images
- Fatemeh Sadat Mohammadi
- , Hasti Shabani
- & Mojtaba Zarei
Article 09 June 2024 | Open Access
A deep image classification model based on prior feature knowledge embedding and application in medical diagnosis
- , Jiangxing Wu
- & Yihua Cheng
Article 07 June 2024 | Open Access
Estimation of the amount of pear pollen based on flowering stage detection using deep learning
- , Takefumi Hiraguri
- & Yoshihiro Takemura
Article 29 May 2024 | Open Access
Remote sensing image dehazing using generative adversarial network with texture and color space enhancement
- , Tie Zhong
- & Chunming Wu
A novel approach to craniofacial analysis using automated 3D landmarking of the skull
- Franziska Wilke
- , Harold Matthews
- & Susan Walsh
Browse broader subjects
- Computational biology and bioinformatics
Quick links
- Explore articles by subject
- Guide to authors
- Editorial policies

EDITORIAL article
Editorial: current trends in image processing and pattern recognition.
- PAMI Research Lab, Computer Science, University of South Dakota, Vermillion, SD, United States
Editorial on the Research Topic Current Trends in Image Processing and Pattern Recognition
Technological advancements in computing multiple opportunities in a wide variety of fields that range from document analysis ( Santosh, 2018 ), biomedical and healthcare informatics ( Santosh et al., 2019 ; Santosh et al., 2021 ; Santosh and Gaur, 2021 ; Santosh and Joshi, 2021 ), and biometrics to intelligent language processing. These applications primarily leverage AI tools and/or techniques, where topics such as image processing, signal and pattern recognition, machine learning and computer vision are considered.
With this theme, we opened a call for papers on Current Trends in Image Processing & Pattern Recognition that exactly followed third International Conference on Recent Trends in Image Processing & Pattern Recognition (RTIP2R), 2020 (URL: http://rtip2r-conference.org ). Our call was not limited to RTIP2R 2020, it was open to all. Altogether, 12 papers were submitted and seven of them were accepted for publication.
In Deshpande et al. , authors addressed the use of global fingerprint features (e.g., ridge flow, frequency, and other interest/key points) for matching. With Convolution Neural Network (CNN) matching model, which they called “Combination of Nearest-Neighbor Arrangement Indexing (CNNAI),” on datasets: FVC2004 and NIST SD27, their highest rank-I identification rate of 84.5% was achieved. Authors claimed that their results can be compared with the state-of-the-art algorithms and their approach was robust to rotation and scale. Similarly, in Deshpande et al. , using the exact same datasets, exact same set of authors addressed the importance of minutiae extraction and matching by taking into low quality latent fingerprint images. Their minutiae extraction technique showed remarkable improvement in their results. As claimed by the authors, their results were comparable to state-of-the-art systems.
In Gornale et al. , authors extracted distinguishing features that were geometrically distorted or transformed by taking Hu’s Invariant Moments into account. With this, authors focused on early detection and gradation of Knee Osteoarthritis, and they claimed that their results were validated by ortho surgeons and rheumatologists.
In Tamilmathi and Chithra , authors introduced a new deep learned quantization-based coding for 3D airborne LiDAR point cloud image. In their experimental results, authors showed that their model compressed an image into constant 16-bits of data and decompressed with approximately 160 dB of PSNR value, 174.46 s execution time with 0.6 s execution speed per instruction. Authors claimed that their method can be compared with previous algorithms/techniques in case we consider the following factors: space and time.
In Tamilmathi and Chithra , authors carefully inspected possible signs of plant leaf diseases. They employed the concept of feature learning and observed the correlation and/or similarity between symptoms that are related to diseases, so their disease identification is possible.
In Das Chagas Silva Araujo et al. , authors proposed a benchmark environment to compare multiple algorithms when one needs to deal with depth reconstruction from two-event based sensors. In their evaluation, a stereo matching algorithm was implemented, and multiple experiments were done with multiple camera settings as well as parameters. Authors claimed that this work could be considered as a benchmark when we consider robust evaluation of the multitude of new techniques under the scope of event-based stereo vision.
In Steffen et al. ; Gornale et al. , authors employed handwritten signature to better understand the behavioral biometric trait for document authentication/verification, such letters, contracts, and wills. They used handcrafter features such as LBP and HOG to extract features from 4,790 signatures so shallow learning can efficiently be applied. Using k-NN, decision tree and support vector machine classifiers, they reported promising performance.
Author Contributions
The author confirms being the sole contributor of this work and has approved it for publication.
Conflict of Interest
The author declares that the research was conducted in the absence of any commercial or financial relationships that could be construed as a potential conflict of interest.
Publisher’s Note
All claims expressed in this article are solely those of the authors and do not necessarily represent those of their affiliated organizations, or those of the publisher, the editors and the reviewers. Any product that may be evaluated in this article, or claim that may be made by its manufacturer, is not guaranteed or endorsed by the publisher.
Santosh, KC, Antani, S., Guru, D. S., and Dey, N. (2019). Medical Imaging Artificial Intelligence, Image Recognition, and Machine Learning Techniques . United States: CRC Press . ISBN: 9780429029417. doi:10.1201/9780429029417
CrossRef Full Text | Google Scholar
Santosh, KC, Das, N., and Ghosh, S. (2021). Deep Learning Models for Medical Imaging, Primers in Biomedical Imaging Devices and Systems . United States: Elsevier . eBook ISBN: 9780128236505.
Google Scholar
Santosh, KC (2018). Document Image Analysis - Current Trends and Challenges in Graphics Recognition . United States: Springer . ISBN 978-981-13-2338-6. doi:10.1007/978-981-13-2339-3
Santosh, KC, and Gaur, L. (2021). Artificial Intelligence and Machine Learning in Public Healthcare: Opportunities and Societal Impact . Spain: SpringerBriefs in Computational Intelligence Series . ISBN: 978-981-16-6768-8. doi:10.1007/978-981-16-6768-8
Santosh, KC, and Joshi, A. (2021). COVID-19: Prediction, Decision-Making, and its Impacts, Book Series in Lecture Notes on Data Engineering and Communications Technologies . United States: Springer Nature . ISBN: 978-981-15-9682-7. doi:10.1007/978-981-15-9682-7
Keywords: artificial intelligence, computer vision, machine learning, image processing, signal processing, pattern recocgnition
Citation: Santosh KC (2021) Editorial: Current Trends in Image Processing and Pattern Recognition. Front. Robot. AI 8:785075. doi: 10.3389/frobt.2021.785075
Received: 28 September 2021; Accepted: 06 October 2021; Published: 09 December 2021.
Edited and reviewed by:
Copyright © 2021 Santosh. This is an open-access article distributed under the terms of the Creative Commons Attribution License (CC BY). The use, distribution or reproduction in other forums is permitted, provided the original author(s) and the copyright owner(s) are credited and that the original publication in this journal is cited, in accordance with accepted academic practice. No use, distribution or reproduction is permitted which does not comply with these terms.
*Correspondence: KC Santosh, [email protected]
Disclaimer: All claims expressed in this article are solely those of the authors and do not necessarily represent those of their affiliated organizations, or those of the publisher, the editors and the reviewers. Any product that may be evaluated in this article or claim that may be made by its manufacturer is not guaranteed or endorsed by the publisher.
Recent trends in image processing and pattern recognition
- Guest Editorial
- Published: 27 October 2020
- Volume 79 , pages 34697–34699, ( 2020 )
Cite this article
- K. C. Santosh 1 &
- Sameer K. Antani 2
2355 Accesses
8 Citations
Explore all metrics
Avoid common mistakes on your manuscript.
The Call for Papers of the special issue was initially sent out to the participants of the 2018 conference (2nd International Conference on Recent Trends in Image Processing and Pattern Recognition). To attract high quality research articles, we also accepted papers for review from outside the conference event. Of 123 submissions, 22 papers were accepted. The acceptance rate, therefore, is just under 18%.
In “Multilevel Polygonal Descriptor Matching Defined by Combining Discrete Lines and Force Histogram Concepts,” authors presented a new method to describe shapes from a set of polygonal curves using a relational descriptor. In their study, relational descriptor is the main idea of the paper.
In “An Asymmetric Cryptosystem based on the Random Weighted Singular Value Decomposition and Fractional Hartley Domain,” authors proposed an encryption system for double random phase encoding based on random weighted singular value decomposition and fractional Hartley transform domain. Authors claimed that the proposed cryptosystem is efficiently compared with singular value decomposition and truncated singular value decomposition.
In “Classification of Complex Environments using Pixel Level Fusion of Satellite Data,” authors analyzed composite land features by fusing two original hyperspectral and multispectral datasets. In their study, the fusion image technique was found to be superior to the single original image.
In “Image Dehazing using Window-based Integrated Means Filter,” authors reported that the proposed technique outperforms the state-of-the-arts in single image dehazing approaches.
In “Research on Fundus Image Registration and Fusion Method based on Nonsubsampled Contourlet and Adaptive Pulse Coupled Neural Network,” authors presented a registration and fusion method of fluorescein fundus angiography image and color fundus image that combines Nonsubsampled Contourlet (NSCT) and adaptive Pulse Coupled Neural Network (PCNN). Authors claimed that the image fusion provides an effective reference for the clinical diagnosis of fundus diseases.
In “Super Resolution of Single Depth Image based on Multi-dictionary Learning with Edge Feature Regularization,” authors focused on super resolution based on multi-dictionary learning with edge regularization model. With this, the reconstructed depth images were found to be superior with respect to the state-of-art methods.
In “A Universal Foreground Segmentation Technique using Deep Neural Network,” authors presented an idea of optical-flow details to make use of temporal information in deep neural network.
In “Removal of ‘Salt & Pepper’ Noise from Color Images using Adaptive Fuzzy Technique based on Histogram Estimation,” authors focused on the use of processing window that is based on local noise densities using fuzzy based criterion.
In “Image Retrieval by Integrating Global Correlation of Color and Intensity Histograms with Local Texture Features,” authors integrated color, intensity histograms with local state-of-the-art texture features to perform content-based image retrieval.
In “Image-based Features for Speech Signal Classification,” authors analyzed speech signal with the help of image features. Authors used the idea of computer-based image features for speech analysis.
In “ Ensembling Handcrafted Features with Deep Features: An Analytical Study for Classification of Routine Colon Cancer Histopathological Nuclei Images,” authors studied deep learning models to analyze medical histopathology: classification, segmentation, and detection.
In “Non-destructive and Cost-effective 3D Plant Growth Monitoring System in Outdoor Conditions,” authors monitored plant growth precisely with the use of mobile phone.
In “Fusion based Feature Reinforcement Component for Remote Sensing Image Object Detection,” authors employed reinforcement component (FB-FRC) to improve image classification, where two fusion strategies are proposed: a hard-fusion strategy through artificially set rules; and a soft fusion strategy by learning the fusion parameters.
In “An Improved Cuckoo Search Algorithm for Multi-level Gray-scale Image Thresholding,” authors employed computationally efficient cuckoo search algorithm.
In “Image Fuzzy Enhancement Algorithm based on Contourlet Transform Domain,” authors focused on enhancing globally the texture and edge of the image.
In “Pixel Encoding for Unconstrained Face Detection,” authors employed handcrafted and visual features to detect human faces. Authors claimed an improvement when handcrafted and visual features are combined.
In “Data Augmentation for Handwritten Digit Recognition using Generative Adversarial Networks (GAN),” authors focused on the technique that does not require prior knowledge of the possible variabilities that exist across examples to create novel artificial examples.
In “Akin-based Orthogonal Space (AOS): A Subspace Learning Method for Face Recognition,” authors reported the use of subspace learning method is efficient for human face recognition.
In “A Kernel Machine for Hidden Object-Ranking Problems (HORPs),” authors proposed a kernel machine that allows retaining item-related ordinal information while avoiding emphasizing class-related information.
In “Verification of Genuine and Forged Offline Signatures using Siamese Neural Network (SNN),” authors reported one shot learning in SNN for signature verification.
In “Super-Resolution Quality Criterion (SRQC): A Super-Resolution Image Quality Assessment Metric,” authors reported the importance of SRQC in assessing image quality. In their experiments, authors found that the SRQC is more competent in modeling the features from curvelet transform that quantifies the quality score of the super-resolved image and it outperforms the formerly reported image quality assessment metrics.
In “Ensemble based Technique for the Assessment of Fetal Health using Cardiotocograph – A Case Study with Standard Feature Reduction Techniques,” authors reported the use of state-of-the-art feature reduction techniques to assess fetal health using cardiotocograph.
Within the scope of image processing pattern recognition, this special issue includes multiple applications domains, such as satellite imaging, biometrics, speech processing, medical imaging, and healthcare.
Author information
Authors and affiliations.
University of South Dakota, Vermillion, SD, 57069, USA
K. C. Santosh
U.S. National Library of Medicine, NIH, Bethesda, MD, 20894, USA
Sameer K. Antani
You can also search for this author in PubMed Google Scholar
Corresponding author
Correspondence to K. C. Santosh .
Additional information
Publisher’s note.
Springer Nature remains neutral with regard to jurisdictional claims in published maps and institutional affiliations.
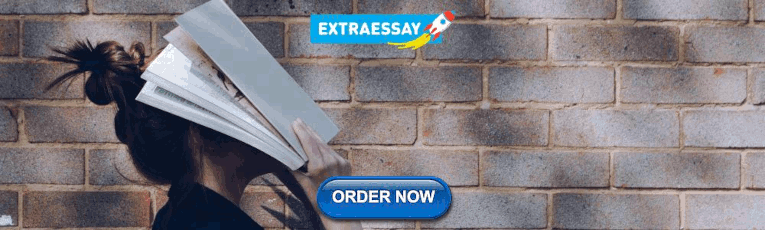
Rights and permissions
Reprints and permissions
About this article
Santosh, K.C., Antani, S.K. Recent trends in image processing and pattern recognition. Multimed Tools Appl 79 , 34697–34699 (2020). https://doi.org/10.1007/s11042-020-10093-3
Download citation
Published : 27 October 2020
Issue Date : December 2020
DOI : https://doi.org/10.1007/s11042-020-10093-3
Share this article
Anyone you share the following link with will be able to read this content:
Sorry, a shareable link is not currently available for this article.
Provided by the Springer Nature SharedIt content-sharing initiative
- Find a journal
- Publish with us
- Track your research

An official website of the United States government
The .gov means it’s official. Federal government websites often end in .gov or .mil. Before sharing sensitive information, make sure you’re on a federal government site.
The site is secure. The https:// ensures that you are connecting to the official website and that any information you provide is encrypted and transmitted securely.
- Publications
- Account settings
Preview improvements coming to the PMC website in October 2024. Learn More or Try it out now .
- Advanced Search
- Journal List
- Bentham Open Access

Viewpoints on Medical Image Processing: From Science to Application
Thomas m. deserno (né lehmann).
1 Department of Medical Informatics, Uniklinik RWTH Aachen, Germany;
Heinz Handels
2 Institute of Medical Informatics, University of Lübeck, Germany;
Klaus H. Maier-Hein (né Fritzsche)
3 Medical and Biological Informatics, German Cancer Research Center, Heidelberg, Germany;
Sven Mersmann
4 Medical and Biological Informatics, Junior Group Computer-assisted Interventions, German Cancer Research Center, Heidelberg, Germany;
Christoph Palm
5 Regensburg – Medical Image Computing (Re-MIC), Faculty of Computer Science and Mathematics, Regensburg University of Applied Sciences, Regensburg, Germany;
Thomas Tolxdorff
6 Institute of Medical Informatics, Charité - Universitätsmedizin Berlin, Germany;
Gudrun Wagenknecht
7 Electronic Systems (ZEA-2), Central Institute of Engineering, Electronics and Analytics, Forschungszentrum Jülich GmbH, Germany;
Thomas Wittenberg
8 Image Processing & Biomedical Engineering Department, Fraunhofer Institute for Integrated Circuits IIS, Erlangen, Germany
Medical image processing provides core innovation for medical imaging. This paper is focused on recent developments from science to applications analyzing the past fifteen years of history of the proceedings of the German annual meeting on medical image processing (BVM). Furthermore, some members of the program committee present their personal points of views: (i) multi-modality for imaging and diagnosis, (ii) analysis of diffusion-weighted imaging, (iii) model-based image analysis, (iv) registration of section images, (v) from images to information in digital endoscopy, and (vi) virtual reality and robotics. Medical imaging and medical image computing is seen as field of rapid development with clear trends to integrated applications in diagnostics, treatment planning and treatment.
1. INTRODUCTION
Current advances in medical imaging are made in fields such as instrumentation, diagnostics, and therapeutic applications and most of them are based on imaging technology and image processing. In fact, medical image processing has been established as a core field of innovation in modern health care [ 1 ] combining medical informatics, neuro-informatics and bioinformatics [ 2 ].
In 1984, the Society of Photo-Optical Instrumentation Engineers (SPIE) has launched a multi-track conference on medical imaging, which still is considered as the core event for innovation in the field [Methods]. Analogously in Germany, the workshop “Bildverarbeitung für die Medizin (BVM)” (Image Processing for Medicine) has recently celebrated its 20 th annual performance. The meeting has evolved over the years to a multi-track conference on international standard [ 3 , 4 , 5 , 6 , 7 , 8 , 9 ].
Nonetheless, it is hard to name the most important and innovative trends within this broad field ranging from image acquisition using novel imaging modalities to information extraction in diagnostics and treatment. Ritter et al. recently emphasized on the following aspects: (i) enhancement, (ii) segmentation, (iii) registration, (iv) quantification, (v) visualization, and (vi) computer-aided detection (CAD) [ 10 ].
Another concept of structuring is here referred to as the “from-to” approach. For instance,
- From nano to macro : Co-founded in 2002 by Michael Unser of EPFL, Switzerland, The Institute of Electrical and Electronics Engineers (IEEE) has launched an international symposium on biomedical imaging (ISBI). This conference is focused in the motto from nano to macro covering all aspects of medical imaging from sub-cellular to the organ level.
- From production to sharing : Another “from-to” migration is seen in the shift from acquisition to communication [ 11 ]. Clark et al. expected advances in the medical imaging fields along the following four axes: (i) image production and new modalities; (ii) image processing, visualization, and system simulation; (iii) image management and retrieval; and (iv) image communication and telemedicine.
- From kilobyte to terabyte : Deserno et al. identified another “from-to” migration, which is seen in the amount of data that is produced by medical imagery [ 12 ]. Today, High-resolution CT reconstructs images with 8000 x 8000 pixels per slice with 0.7 μm isotropic detail detectability, and whole body scans with this resolution reach several Gigabytes (GB) of data load. Also, microscopic whole-slide scanning systems can easily provide so-called virtual slices in the rage of 30.000 x 50.000 pixels, which equals 16.8 GB on 10 bit gray scale.
- From science to application : Finally, in this paper, we aim at analyzing recent advantages in medical imaging on another level. The focus is to identify core fields fostering transfer of algorithms into clinical use and addressing gaps still remaining to be bridged in future research.
The remainder of this review is organized as follows. In Section 3, we briefly analyze the history of the German workshop BVM. More than 15 years of proceedings are currently available and statistics is applied to identify trends in content of conference papers. Section 4 then provides personal viewpoints to challenging and pioneering fields. The results are discussed in Section 5.
2. THE GERMAN HISTORY FROM SCIENCE TO APPLICATION
Since 1994, annual proceedings of the presented contributions from the BVM workshops have been published, which are available electronically in postscript (PS) or the portable document format (PDF) from 1996. Disregarding the type of presentation (oral, poster, or software demonstration), the authors are allowed to submit papers with a length of up to five pages. In 2012 the length was increased to six pages. Both, English and German papers are allowed. The number of English contributions increased steadily over the years, and reached about 50% in 2008 [ 8 ].
In order to analyze the content of the on average 124k words long proceedings regarding the most relevant topics that were discussed on the BVM workshops, the incidence of the most frequent words has been assessed for each proceeding from 1996 until 2012. From this investigation, about 300 common words of the German and English language (e.g. and / und, etc.) have been excluded. (Fig. 1 1 ) presents a word cloud computed from the 100 most frequent terms used in the proceedings of the 2012 BVM workshop. The font sizes of the words refer to their counted frequency in the text.

Word cloud representing the most frequent 100 terms counted from the 469 page long BVM proceedings 2012 [13].
It can be seen, in 2012, “image” was the most frequent word occurring in the BVM proceedings (920 incidences), as also observed in all the other years (1996-2012: 10,123 incidences). Together with terms like “reconstruction”, “analysis”, or “processing”, medical imaging is clearly recognizable as the major subject of the BVM workshops.
Concerning the scientific direction of the BVM meeting over time, terms such as “segmentation”, “registration”, and “navigation”, which indicate image processing procedures relevant for clinical applications, have been used with increasing frequencies (Fig. 2 2 , left). The same holds for terms like “evaluation” or “experiment”, which are related to the validation of the contributions (Fig. 2 2 , middle), constituting a first step towards the transition of the scientific results into a clinical application. (Fig. 2 2 right) shows the occurrence of the words “patient” and “application” in the contributed papers of the BVM workshops between 1996 and 2012. Here, rather constant numbers of occurrences are found indicating a stringent focus on clinical applications.

Trends from BVM workshop proceedings from important terms of processing procedures (left), experimental verification (middle), and application to humans (right).
3. VIEWPOINTS FROM SCIENCE TO APPLICATION
3.1. multi-modal image processing for imaging and diagnosis.
Multi-modal imaging refers to (i) different measurements at a single tomographic system (e.g., MRI and functional MRI), (ii) measurements at different tomographic systems (e.g., computed tomography (CT), positron emission tomography (PET), and single photon emission computed tomography (SPECT)), and (iii) measurements at integrated tomographic systems (PET/CT, PET/MR). Hence, multi-modal tomography has become increasingly popular in clinical and preclinical applications (Fig. 3 3 ) providing images of morphology and function (Fig. 4 4 ).

PubMed cited papers for search “multimodal AND (imaging OR tomography OR image)”.

Morphological and functional imaging in clinical and pre-clinical applications.
Multi-modal image processing for enhancing multi-modal imaging procedures primarily deals with image reconstruction and artifact reduction. Examples are the integration of additional information about tissue types from MRI as an anatomical prior to the iterative reconstruction of PET images [ 14 ] and the CT- or MR-based correction of attenuation artifacts in PET, respectively, which is an essential prerequisite for quantitative PET analysis [ 15 , 16 ]. Since these algorithms are part of the imaging workflow, only highly automated, fast, and robust algorithms providing adequate accuracy are appropriate solutions. Accordingly, the whole image in the different modalities must be considered.
This requirement differs for multi-modal diagnostic approaches. In most applications, a single organ or parts of an organ are of interest. Anatomical and particularly pathological regions often show a high variability due to structure, deformation, or movement, which is difficult to predict and is thus a great challenge for image processing. In multi-modality applications, images represent complementary information often obtained at different time-scales introducing additional complexity for algorithms. Other inequalities are introduced by the different resolutions and fields of view showing the organ of interest in different degrees of completeness. From a scientific and thus algorithmic point of view, image processing methods for multi-modal images must meet higher requirements than those applied to single-modality images.
Looking exemplarily at segmentation as one of the most complex and demanding problems in medical image processing, the modality showing anatomical and pathological structures in high resolution and contrast (e.g., MRI, CT) is typically used to segment the structure or volume of interest (VOI) to subsequently analyze other properties such as function within these target structures. Here, the different resolutions have to be regarded to correct for partial volume effects in the functional modality (e.g., PET, SPECT). Since the structures to be analyzed are dependent on the disease of the actual patient examined, automatic segmentation approaches are appropriate solutions if the anatomical structures of interest are known beforehand [ 17 ], while semi-automatic approaches are advantageous if flexibility is needed [ 18 , 19 ].
Transferring research into diagnostic application software requires a graphical user interface (GUI) to parameterize the algorithms, 2D and 3D visualization of multi-modal images and segmentation results, and tools to interact with the visualized images during the segmentation procedure. The Medical Interaction Toolkit [ 20 ] or the MevisLab [ 21 ] provide the developer with frameworks for multi-modal visualization, interaction and tools to build appropriate GUIs, yielding an interface to integrate new algorithms from science to application.
Another important aspect transferring algorithms from pure academics to clinical practice is evaluation. Phantoms can be used for evaluating specific properties of an algorithm, but not for evaluating the real situation with all its uncertainties and variability. Thus, the most important step of migrating is extensive testing of algorithms on large amounts of real clinical data, which is a great challenge particularly for multi-modal approaches, and should in future be more supported by publicly available databases.
3.2. Analysis of Diffusion Weighted Images
Due to its sensitivity to micro-structural changes in white matter, diffusion weighted imaging (DWI) is of particular interest to brain research. Stroke is the most common and well known clinical application of DWI, where the images allow the non-invasive detection of ischemia within minutes of onset and are sensitive and relatively specific in detecting changes triggered by strokes [ 22 ]. The technique has also allowed deeper insights into the pathogenesis of Alzheimer’s disease, Parkinson disease, autism spectrum disorder, schizophrenia, and many other psychiatric and non-psychiatric brain diseases. DWI is also applied in the imaging of (mild) traumatic brain injury, where conventional techniques lack sensitivity to detect the subtle changes occurring in the brain. Here, studies on sports-related traumata in the younger population have raised considerable debates in the recent past [ 23 ].
Methodologically, recent advances in the generation and analysis of large-scale networks on basis of DWI are particularly exciting and promise new dimensions in quantitative neuro-imaging via the application of the profound set of tools available in graph theory to brain image analysis [ 24 ]. DWI sheds light on the living brain network architecture, revealing the organization of fiber connections together with their development and change in disease.
Big challenges remain to be solved though: Despite many years of methodological development in DWI post-processing, the field still seems to be in its infancy. The reliable tractography-based reconstruction of known or pathological anatomy is still not solved. Current reconstruction challenges at the 2011 and 2012 annual meetings of the Medical Image Computing and Computer Assisted Intervention (MICCAI) Society have demonstrated the lack of methods that can reliably reconstruct large and well-known structures like the cortico-spinal tract in datasets of clinical quality [ 25 ]. Missing reference-based evaluation techniques hinder the well-founded demonstration of the real advantages of novel tractography algorithms over previous methods [ 26 ]. The mentioned limitations have obscured a broader application of DWI tractography, e.g. in surgical guidance. Even though the application of DWI e.g. in surgical resection has shown to facilitate the identification of risk structures [ 27 ], the widespread use of these techniques in surgical practice remains limited mainly by the lack of robust and standardized methods that can be applied multi-centered across institutions and comprehensive evaluation of these algorithms.
However, there are numerous applications of DWI in cancer imaging, which bridge imaging science and clinical application. The imaging modality has shown potential in the detection, staging and characterization of tumors (Fig. 5 5 ), the evaluation of therapy response, or even in the prediction of therapy outcome [ 28 ]. DWI was also applied in the detection and characterization of lesions in the abdomen and the pelvis, where increased cellularity of malignant tissue leads to restricted diffusion when compared to the surrounding tissue [ 29 ]. The challenge here again will be the establishment of reliable sequences and post-processing methods for the wide-spread and multi-centric application of the techniques in the future.

Depiction of fiber tracts in the vicinity of a grade IV glioblastoma. The volumetric tracking result (yellow) was overlaid on an axial T2-FLAIR image. Red and green arrows indicate the necrotic tumor core and peritumoral hyperintensity, respectively. In the frontal parts, fiber tracts are still depicted, whereas in the dorsal part, tracts seem to be either displaced or destructed by the tumor.
3.3. Model-Based Image Analysis
As already emphasized in the previous viewpoints, there is a big gap between the state of the art in current research and methods available in clinical application, especially in the field of medical image analysis [ 30 ]. Segmentation of relevant image structures (tissues, tumors, vessels etc.) is still one of the key problems in medical image computing lacking robust and automatic methods. The application of pure data-driven approaches like thresholding, region growing, edge detection, or enhanced data-driven methods like watershed algorithms, Markov random field (MRF)-based approaches, or graph cuts often leads to weak segmentations due to low contrasts between neighboring image objects, image artifacts, noise, partial volume effects etc.
Model-based segmentation integrates a-priori knowledge of the shapes and appearance of relevant structures into the segmentation process. For example, the local shape of a vessel can be characterized by the vesselness operator [ 31 ], which generates images with an enhanced representation of vessels. Using the vesselness information in combination with the original grey value image segmentation of vessels can be improved significantly and especially the segmentation of a small vessel becomes possible (e.g. [ 32 ]).
In statistical or active shape and appearance models [ 33 , 34 ], shape variability in organ distribution among individuals and characteristic gray value distributions in the neighborhood of the organ can be represented. In these approaches, a set of segmented image data is used to train active shape and active appearance models, which include information about the mean shape and shape variations as well as characteristic gray value distributions and their variation in the population represented in the training data set. Instead of direct point-to-point correspondences that are used during the generation of classical statistical shape models, Hufnagel et al. have suggested probabilistic point-to-point correspondences [ 35 ]. This approach takes into account that often inaccuracies are unavoidable by the definition of direct point correspondences between organs of different persons. In probabilistic statistical shape models, these correspondence uncertainties are respected explicitly to improve the robustness and accuracy of shape modeling and model-based segmentation. Integrated in an energy minimizing level set framework, the probabilistic statistical shape models can be used for enhanced organ segmentation [ 36 ].
In contrast thereto, atlas-based segmentation methods (e.g., [ 37 ]) realize a case-based approach and make use of the segmentation information contained in a single segmented data set, which is transferred to an unseen patient image data set. The transfer of the atlas segmentation to the patient segmentation is done by inter-individual non-linear registration methods. Multi-atlas segmentation methods using several atlases have been proposed (e.g. [ 38 ]) and show an improved accuracy and robustness in comparison to single atlas segmentation methods. Hence, multi-atlas approaches are currently in the focus of further research [ 39 , 40 ].
In future, more task-oriented systems integrated into diagnostic processes, intervention planning, therapy and follow-up are needed. In the field of image analysis, due the limited time of the physicians, automatic procedures are of special interest to segment and extract quantitative object parameters in an accurate, reproducible and robust way. Furthermore, intelligent and easy-to-use methods for fast correction of unavoidable segmentation errors are needed.
3.4. Registration of Section Images
Imaging techniques such as histology [ 41 ] or auto-radiography [ 42 ] are based on thin post-mortem sections. In comparison to in-vivo imaging, e.g. positron emission tomography (PET), magnetic resonance imaging (MRI), or DWI (as addressed in the previous viewpoint, cf. Section 4.1), several properties are considered advantageous. For instance, tissue can be processed after sectioning to enhance contrast (e.g. staining) [ 43 ], to mark specific properties like receptors [ 44 ] or to apply laser ablation studying the spatial element distribution [ 45 ]; tissue can be scanned in high-resolution [ 43 ]; and tissue is thin enough to allow optical light transmission imaging, e.g. polarized light imaging (PLI) [ 46 ]. Therefore, section imaging results in high space-resolved and high-contrasted data, which supports findings such as cytoarchitectonic boundaries [ 47 ], neuronal fiber directions [ 48 ], and receptor or element distributions [ 45 ].
Restacking of 2D sections into a 3D volume followed by the fusion of this stack with an in-vivo volume is the challenging task of medical image processing on the track from science to application. The 3D section stacks then serve as an atlas for a large variety of applications. Sections are non-linearly deformed during cutting and post-processing. Additionally, discontinuous artifacts like tears or enrolled tissue hamper the correspondence of true structure and tissue imaged.
The so-called “problem of the digitized banana” [ 41 ] prohibits the section-by-section registration without 3D reference. Smoothness of registered stacks is not equivalent to consistency and correctness. Whereas the deformations are section-specific, the orientation of the sections in comparison to the 3D structure depends on the cutting direction and, thus, is the same for all sections. In this tangled situation the question rises, if it is better to (i) restack the sections first, register the whole stack afterwards and correct for deformations at last (volume-first approach) or (ii) to register each section individually to the 3D reference volume while correcting deformations at the same time (section-first approach). Both approaches combine
- Multi-modal registration : The need of a 3D reference and the application to correlate high-resolution section imaging findings with in-vivo imaging are sometimes solved at the same time. If possible, the 3D in-vivo modality itself is used as a reference.

Characteristic flow chart of volume-first approach and volume generation with (gray boxes) or without blockface images as intermediate reference modality (Column I). Either the in-vivo volume is post-processed to generate a pseudo-high-resolution volume with propagated section gaps (Column II) or the section volume is post-processed to get a low-resolution stack with filled gaps (Column III) [42].
Due to the variety of difficulties, missing evaluation possibilities and section specifics like post-processing, embedding, cutting procedure and tissue type there is not just one best approach to come from 2D to 3D. But careful work in this field is paid off by cutting edge applications. Not least within the European flagship, The Human Brain Project (HBP), further research in this area of medical image processing is demanded. The state-of-the-art review of HBP states in the context of human brain mapping: “What is missing to date is an integrated open source tool providing a standard application programming interface (API) for data registration and coordinate transformations and guaranteeing multi-scale and multi-modal data accuracy” [ 49 ]. Such a tool will narrow the gap from science to application.
3.5. From Images to Information in Digital Endoscopy
Basic endoscopic technologies and their routine applications (Fig. 7 7 , bottom layers) still are purely data-oriented, as the complete image analysis and interpretation is performed solely by the physician. If content of endoscopic imagery is analyzed automatically, several new application scenarios for diagnostics and intervention with increasing complexity can be identified (Fig. 7 7 , upper layers). As these new possibilities of endoscopy are inherently coupled with the use of computers, these new endoscopic methods and applications can be referred to as computer-integrated endoscopy [ 50 ]. Information, however, is referred to on the highest of the five levels of semantics (Fig. 7 7 ):

Modules to build computer-integrated endoscopy, which enables information gain from image data.
- 1. Acquisition : Advancements in diagnostic endoscopy were obtained by glass fibers for the transmission of electric light into and image information out of the body. Besides the pure wire-bound transmission of endoscopic imagery, in the past 10 years wireless broadcast came available for gastroscopic video data captured from capsule endoscopes [ 51 ].
- 2. Transportation : Based on digital technologies, essential basic processes of endoscopic still image and image sequence capturing, storage, archiving, documentation, annotation and transmission have been simplified. These developments have initially led to the possibilities for tele-diagnosis and tele-consultations in diagnostic endoscopy, where the image data is shared using local networks or the internet [ 52 ].
- 3. Enhancement : Methods and applications for image enhancement include intelligent removal of honey-comb patterns in fiberscopic recordings [ 53 ], temporal filtering for the reduction of ablation smoke and moving particles [ 54 ], image rectification for gastroscopes. Additionally, besides having an increased complexity, they have to work in real time with a maximum delay of 60 milliseconds, to be acceptable for surgeons and physicians.
- 4. Augmentation : Image processing enhances endoscopic views with additional type of information. Examples of this type are artificial working horizon, key-hole views to endoscopic panorama-images [ 55 ], 3D surfaces computed from point clouds obtained by special endoscopic imaging devices such as stereo endoscopes [ 56 ], time-of-flight endoscopes [ 57 ], or shape-from polarization approaches [ 58 ]. This level also includes the possibilities of visualization and image fusion of endoscopic views with preoperative acquired radiological imagery such as angiography or CT data [ 59 ] for better intra-operative orientation and navigation, as well as image-based tracking and navigation through tubular structures [ 60 ].
- 5. Content : Methods of content-based image analysis consider the automated segmentation, characterization and classification of diagnostic image content. Such methods describe computer-assisted detection (CADe) [ 61 ] of lesions (such as e.g. polyps) or computer-assisted diagnostics (CADx) [ 62 ], where already detected and delineated regions are characterized and classified into, for instance, benign or malign tissue areas. Furthermore, such methods automatically identify and track surgical instruments, e.g. supporting robotic surgery approaches.
On the technical side the semantics of the extracted image contents increases from the pure image recording up to the image content analysis level. This complexity also relates to the expected time axis needed to bring these methods from science to clinical applications.
From the clinical side, the most complex methods such as automated polyp detection (CADe) are considered as most important. However, it is expected that computer-integrated endoscopy systems will increasingly enter clinical applications and as such will contribute to the quality of the patient’s healthcare.
3.6. Virtual Reality and Robotics
Virtual reality (VR) and robotics are two rapidly expanding fields with growing application in surgery. VR creates three-dimensional environments increasing the capability for sensory immersion, which provides the sensation of being present in the virtual space. Applications of VR include surgical planning, case rehearsal, and case playback, which could change the paradigm of surgical training, which is especially necessary as the regulations surrounding residencies continue to change [ 63 ]. Surgeons are enabled to practice in controlled situations with preset variables to gain experience in a wide variety of surgical scenarios [ 64 ].
With the availability of inexpensive computational power and the need for cost-effective solutions in healthcare, medical technology products are being commercialized at an increasingly rapid pace. VR is already incorporated into several emerging products for medical education, radiology, surgical planning and procedures, physical rehabilitation, disability solutions, and mental health [ 65 ]. For example, VR is helping surgeons learn invasive techniques before operating, and allowing physicians to conduct real-time remote diagnosis and treatment. Other applications of VR include the modeling of molecular structures in three dimensions as well as aiding in genetic mapping and drug synthesis.
In addition, the contribution of robotics has accelerated the replacement of many open surgical treatments with more efficient minimally invasive surgical techniques using 3D visualization techniques. Robotics provides mechanical assistance with surgical tasks, contributing greater precision and accuracy and allowing automation. Robots contain features that can augment surgical performance, for instance, by steadying a surgeon’s hand or scaling the surgeon’s hand motions [ 66 ]. Current robots work in tandem with human operators to combine the advantages of human thinking with the capabilities of robots to provide data, to optimize localization on a moving subject, to operate in difficult positions, or to perform without muscle fatigue. Surgical robots require spatial orientation between the robotic manipulators and the human operator, which can be provided by VR environments that re-create the surgical space. This enables surgeons to perform with the advantage of mechanical assistance but without being alienated from the sights, sounds, and touch of surgery [ 67 ].
After many years of research and development, Japanese scientists recently presented an autonomous robot which is able to realize surgery within the human body [ 68 ]. They send a miniature robot inside the patient’s body, perceive what the robot saw and touched before conducting surgery by using the robot’s minute arms as though as it were the one’s of the surgeon.
While the possibilities – and the need – for medical VR and robotics are immense, approaches and solutions using new applications require diligent, cooperative efforts among technology developers, medical practitioners and medical consumers to establish where future requirements and demand will lie. Augmented and virtual reality substituting or enhancing the reality can be considered as multi-reality approaches [ 69 ], which are already available in commercial products for clinical applications.
4. DISCUSSION
In this paper, we have analyzed the written proceedings of the German annual meeting on Medical Imaging (BVM) and presented personal viewpoints on medical image processing focusing on the transfer from science to application. Reflecting successful clinical applications and promising technologies that have been recently developed, it turned out that medical image computing has transferred from single- to multi-images, and there are several ways to combine these images:
- Multi-modality : Figs. 2 2 and 3 3 have emphasized that medical image processing has been moved away from the simple 2D radiograph via 3D imaging modalities to multi-modal processing and analyzing. Successful applications that are transferrable into the clinics jointly process imagery from different modalities.
- Multi-resolution : Here, images with different properties from the same subject and body area need alignment and comparison. Usually, this implies a multi-resolution approach, since different modalities work on different scales of resolutions.
- Multi-scale : If data becomes large, as pointed out for digital pathology, algorithms must operate on different scales, iteratively refining the alignment from coarse-to-fine. Such algorithmic design usually is referred to as multi-scale approach.
- Multi-subject : Models have been identified as key issue for implementing applicable image computing. Such models are used for segmentation, content understanding, and intervention planning. They are generated from a reliable set of references, usually based on several subjects.
- Multi-atlas : Even more complex, the personal viewpoints have identified multi-atlas approaches that are nowadays addressed in research. For instance in segmentation, accuracy and robustness of algorithms are improved if they are based on multiple rather than a single atlas. Both, accuracy and robustness are essential requirements for transferring algorithms into the clinical use.
- Multi-semantics : Based on the example of digital endoscopy, another “multi” term is introduced. Image understanding and interpretation has been defined on several levels of semantics, and successful applications in computer-integrated endoscopy are operating on several of such levels.
- Multi-reality : Finally, our last viewpoint has addressed the augmentation of the physician’s view by means of virtual reality. Medical image computing is applied to generate and superimpose such views, which results in a multi-reality world.
Andriole, Barish, and Khorasani also have discussed issues to consider for advanced image processing in the clinical arena [ 70 ]. In completion of the collection of “multi” issues, they emphasized that radiology practices are experiencing a tremendous increase in the number of images associated with each imaging study, due to multi-slice , multi-plane and/or multi-detector 3D imaging equipment. Computer-aided detection used as a second reader or as a first-pass screener will help maintaining or perhaps improving readers' performance on such big data in terms of sensitivity and specificity.
Last not least, with all these “multies”, the computational load of algorithms again becomes an issue. Modern computers provide enormous computational power and yield a revisiting and applications of several “old” approaches, which did not find their way into the clinical use yet, just because of the processing times. However, combining many images of large sizes, processing time becomes crucial again. Scholl et al. have recently addressed this issue reviewing applications based on parallel processing and usage of graphical processors for image analysis [ 12 ]. These are seen as multi-processing methods.
In summary, medical image processing is a progressive field of research, and more and more applications are becoming part of the clinical practice. These applications are based on one or more of the “multi” concepts that we have addressed in this review. However, effects from current trends in the Medical Device Directives that increase the efforts needed for clinical trials of new medical imaging procedure, cannot be observed until today. It will hence be an interesting point to follow the trend of the translation of scientific results of future BVM workshops into clinical applications.
ACKNOWLEDGEMENTS
We would like to thank Hans-Peter Meinzer, Co-Chair of the German BVM, for his helpful suggestions and for encouraging his research fellows to contribute and hence, giving this paper a “ multi-generation ” view.
CONFLICT OF INTEREST
The author(s) confirm that this article content has no conflict of interest.
Information
- Author Services
Initiatives
You are accessing a machine-readable page. In order to be human-readable, please install an RSS reader.
All articles published by MDPI are made immediately available worldwide under an open access license. No special permission is required to reuse all or part of the article published by MDPI, including figures and tables. For articles published under an open access Creative Common CC BY license, any part of the article may be reused without permission provided that the original article is clearly cited. For more information, please refer to https://www.mdpi.com/openaccess .
Feature papers represent the most advanced research with significant potential for high impact in the field. A Feature Paper should be a substantial original Article that involves several techniques or approaches, provides an outlook for future research directions and describes possible research applications.
Feature papers are submitted upon individual invitation or recommendation by the scientific editors and must receive positive feedback from the reviewers.
Editor’s Choice articles are based on recommendations by the scientific editors of MDPI journals from around the world. Editors select a small number of articles recently published in the journal that they believe will be particularly interesting to readers, or important in the respective research area. The aim is to provide a snapshot of some of the most exciting work published in the various research areas of the journal.
Original Submission Date Received: .
- Active Journals
- Find a Journal
- Proceedings Series
- For Authors
- For Reviewers
- For Editors
- For Librarians
- For Publishers
- For Societies
- For Conference Organizers
- Open Access Policy
- Institutional Open Access Program
- Special Issues Guidelines
- Editorial Process
- Research and Publication Ethics
- Article Processing Charges
- Testimonials
- Preprints.org
- SciProfiles
- Encyclopedia
Topic Information
Participating journals, topic editors.
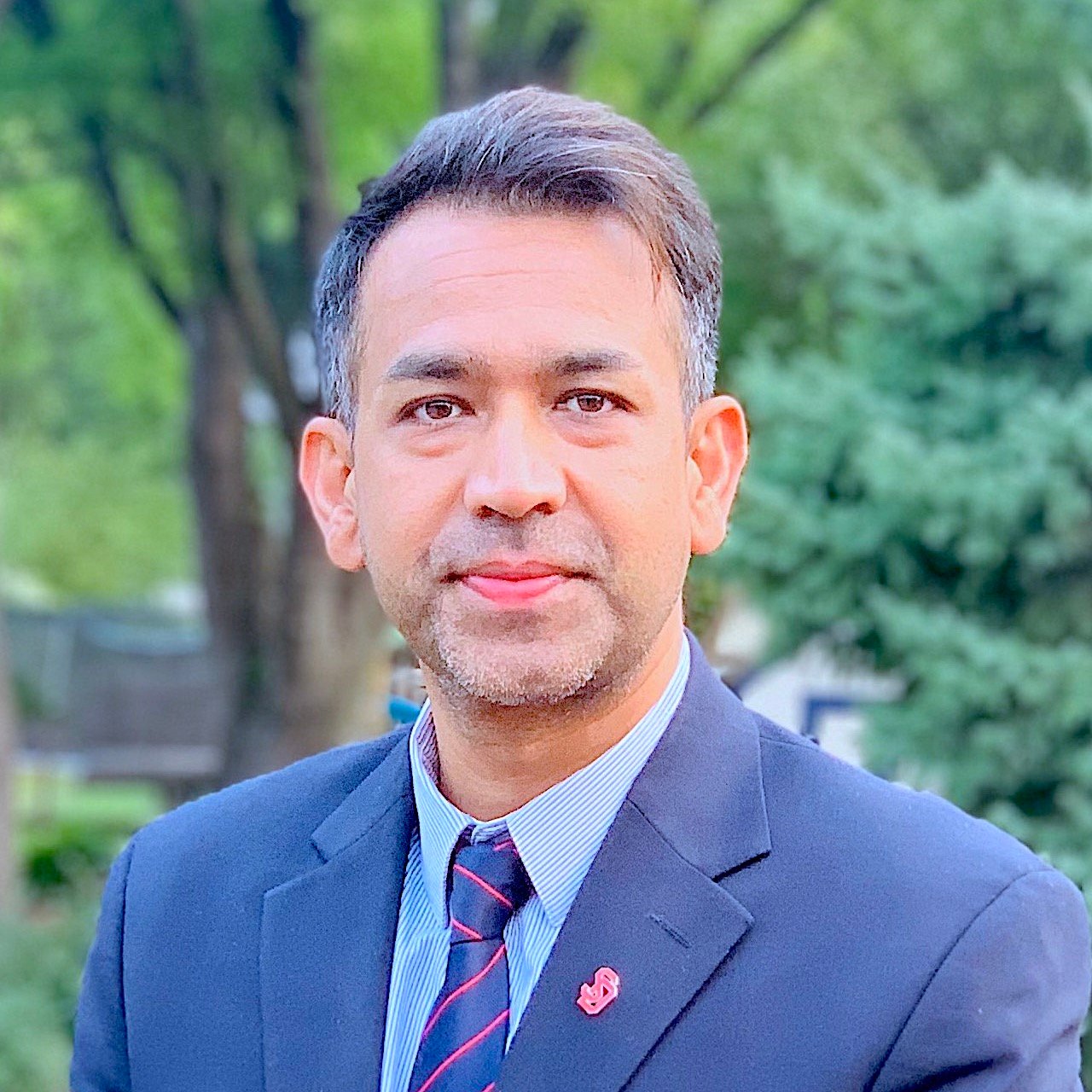
Find support for a specific problem in the support section of our website.
Please let us know what you think of our products and services.
Visit our dedicated information section to learn more about MDPI.
Recent Trends in Image Processing and Pattern Recognition
Dear Colleagues,
The 5th International Conference on Recent Trends in Image Processing and Pattern Recognition (RTIP2R) aims to attract current and/or advanced research on image processing, pattern recognition, computer vision, and machine learning. The RTIP2R will take place at the Texas A&M University—Kingsville, Texas (USA), on November 22–23, 2022, in collaboration with the 2AI Research Lab—Computer Science, University of South Dakota (USA).
Authors of selected papers from the conference will be invited to submit extended versions of their original papers and contributions under the conference topics (new papers that are closely related to the conference themes are also welcome).
We, however, are not limited to RIP2R 2022 to increase the number of submissions.
Topics of interest include, but are not limited to, the following:
- Signal and image processing .
- Computer vision and pattern recognition : object detection and/or recognition (shape, color, and texture analysis) as well as pattern recognition (statistical, structural, and syntactic methods).
- Machine learning : algorithms, clustering and classification, model selection (machine learning), feature engineering, and deep learning.
- Data analytics : data mining tools and high-performance computing in big data.
- Federated learning : applications and challenges.
- Pattern recognition and machine learning for the Internet of things (IoT).
- Information retrieval : content-based image retrieval and indexing, as well as text analytics.
- Document image analysis and understanding.
- Biometrics: face matching, iris recognition/verification, footprint verification, and audio/speech analysis as well as understanding.
- Healthcare informatics and (bio)medical imaging as well as engineering.
- Big data (from document understanding and healthcare to risk management).
- Cryptanalysis (cryptology and cryptography).
Prof. Dr. KC Santosh Dr. Ayush Goyal Dr. Djamila Aouada Dr. Aaisha Makkar Dr. Yao-Yi Chiang Dr. Satish Kumar Singh Prof. Dr. Alejandro Rodríguez-González Topic Editors
Journal Name | Impact Factor | CiteScore | Launched Year | First Decision (median) | APC |
---|---|---|---|---|---|
entropy | 1999 | 22.4 Days | CHF 2600 | ||
applsci | 2011 | 17.8 Days | CHF 2400 | ||
healthcare | 2013 | 20.5 Days | CHF 2700 | ||
jimaging | 2015 | 20.9 Days | CHF 1800 | ||
computers | 2012 | 17.2 Days | CHF 1800 | ||
BDCC | 2017 | 18 Days | CHF 1800 | ||
ai | 2020 | 17.6 Days | CHF 1600 |

- Immediately share your ideas ahead of publication and establish your research priority;
- Protect your idea from being stolen with this time-stamped preprint article;
- Enhance the exposure and impact of your research;
- Receive feedback from your peers in advance;
- Have it indexed in Web of Science (Preprint Citation Index), Google Scholar, Crossref, SHARE, PrePubMed, Scilit and Europe PMC.
Published Papers (14 papers)
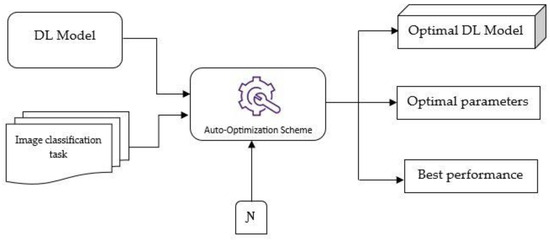
Further Information
Mdpi initiatives, follow mdpi.
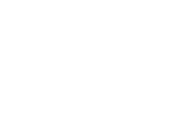
Subscribe to receive issue release notifications and newsletters from MDPI journals
Submit your Manuscript
Submit your abstract.
Digital Image Processing - Science topic

- Recruit researchers
- Join for free
- Login Email Tip: Most researchers use their institutional email address as their ResearchGate login Password Forgot password? Keep me logged in Log in or Continue with Google Welcome back! Please log in. Email · Hint Tip: Most researchers use their institutional email address as their ResearchGate login Password Forgot password? Keep me logged in Log in or Continue with Google No account? Sign up
- Open access
- Published: 05 December 2018
Application research of digital media image processing technology based on wavelet transform
- Lina Zhang 1 ,
- Lijuan Zhang 2 &
- Liduo Zhang 3
EURASIP Journal on Image and Video Processing volume 2018 , Article number: 138 ( 2018 ) Cite this article
7267 Accesses
12 Citations
Metrics details
With the development of information technology, people access information more and more rely on the network, and more than 80% of the information in the network is replaced by multimedia technology represented by images. Therefore, the research on image processing technology is very important, but most of the research on image processing technology is focused on a certain aspect. The research results of unified modeling on various aspects of image processing technology are still rare. To this end, this paper uses image denoising, watermarking, encryption and decryption, and image compression in the process of image processing technology to carry out unified modeling, using wavelet transform as a method to simulate 300 photos from life. The results show that unified modeling has achieved good results in all aspects of image processing.
1 Introduction
With the increase of computer processing power, people use computer processing objects to slowly shift from characters to images. According to statistics, today’s information, especially Internet information, transmits and stores more than 80% of the information. Compared with the information of the character type, the image information is much more complicated, so it is more complicated to process the characters on the computer than the image processing. Therefore, in order to make the use of image information safer and more convenient, it is particularly important to carry out related application research on image digital media. Digital media image processing technology mainly includes denoising, encryption, compression, storage, and many other aspects.
The purpose of image denoising is to remove the noise of the natural frequency in the image to achieve the characteristics of highlighting the meaning of the image itself. Because of the image acquisition, processing, etc., they will damage the original signal of the image. Noise is an important factor that interferes with the clarity of an image. This source of noise is varied and is mainly derived from the transmission process and the quantization process. According to the relationship between noise and signal, noise can be divided into additive noise, multiplicative noise, and quantization noise. In image noise removal, commonly used methods include a mean filter method, an adaptive Wiener filter method, a median filter, and a wavelet transform method. For example, the image denoising method performed by the neighborhood averaging method used in the literature [ 1 , 2 , 3 ] is a mean filtering method which is suitable for removing particle noise in an image obtained by scanning. The neighborhood averaging method strongly suppresses the noise and also causes the ambiguity due to the averaging. The degree of ambiguity is proportional to the radius of the field. The Wiener filter adjusts the output of the filter based on the local variance of the image. The Wiener filter has the best filtering effect on images with white noise. For example, in the literature [ 4 , 5 ], this method is used for image denoising, and good denoising results are obtained. Median filtering is a commonly used nonlinear smoothing filter that is very effective in filtering out the salt and pepper noise of an image. The median filter can both remove noise and protect the edges of the image for a satisfactory recovery. In the actual operation process, the statistical characteristics of the image are not needed, which brings a lot of convenience. For example, the literature [ 6 , 7 , 8 ] is a successful case of image denoising using median filtering. Wavelet analysis is to denoise the image by using the wavelet’s layering coefficient, so the image details can be well preserved, such as the literature [ 9 , 10 ].
Image encryption is another important application area of digital image processing technology, mainly including two aspects: digital watermarking and image encryption. Digital watermarking technology directly embeds some identification information (that is, digital watermark) into digital carriers (including multimedia, documents, software, etc.), but does not affect the use value of the original carrier, and is not easily perceived or noticed by a human perception system (such as a visual or auditory system). Through the information hidden in the carrier, it is possible to confirm the content creator, the purchaser, transmit the secret information, or determine whether the carrier has been tampered with. Digital watermarking is an important research direction of information hiding technology. For example, the literature [ 11 , 12 ] is the result of studying the image digital watermarking method. In terms of digital watermarking, some researchers have tried to use wavelet method to study. For example, AH Paquet [ 13 ] and others used wavelet packet to carry out digital watermark personal authentication in 2003, and successfully introduced wavelet theory into digital watermark research, which opened up a new idea for image-based digital watermarking technology. In order to achieve digital image secrecy, in practice, the two-dimensional image is generally converted into one-dimensional data, and then encrypted by a conventional encryption algorithm. Unlike ordinary text information, images and videos are temporal, spatial, visually perceptible, and lossy compression is also possible. These features make it possible to design more efficient and secure encryption algorithms for images. For example, Z Wen [ 14 ] and others use the key value to generate real-value chaotic sequences, and then use the image scrambling method in the space to encrypt the image. The experimental results show that the technology is effective and safe. YY Wang [ 15 ] et al. proposed a new optical image encryption method using binary Fourier transform computer generated hologram (CGH) and pixel scrambling technology. In this method, the order of pixel scrambling and the encrypted image are used as keys for decrypting the original image. Zhang X Y [ 16 ] et al. combined the mathematical principle of two-dimensional cellular automata (CA) with image encryption technology and proposed a new image encryption algorithm. The image encryption algorithm is convenient to implement, has good security, large key amount, good avalanche effect, high degree of confusion, diffusion characteristics, simple operation, low computational complexity, and high speed.
In order to realize the transmission of image information quickly, image compression is also a research direction of image application technology. The information age has brought about an “information explosion” that has led to an increase in the amount of data, so that data needs to be effectively compressed regardless of transmission or storage. For example, in remote sensing technology, space probes use compression coding technology to send huge amounts of information back to the ground. Image compression is the application of data compression technology on digital images. The purpose of image compression is to reduce redundant information in image data and store and transmit data in a more efficient format. Through the unremitting efforts of researchers, image compression technology is now maturing. For example, Lewis A S [ 17 ] hierarchically encodes the transformed coefficients, and designs a new image compression method based on the local estimation noise sensitivity of the human visual system (HVS). The algorithm can be easily mapped to 2-D orthogonal wavelet transform to decompose the image into spatial and spectral local coefficients. Devore R A [ 18 ] introduced a novel theory to analyze image compression methods based on wavelet decomposition compression. Buccigrossi R W [ 19 ] developed a probabilistic model of natural images based on empirical observations of statistical data in the wavelet transform domain. The wavelet coefficient pairs of the basis functions corresponding to adjacent spatial locations, directions, and scales are found to be non-Gaussian in their edges and joint statistical properties. They proposed a Markov model that uses linear predictors to interpret these dependencies, where amplitude is combined with multiplicative and additive uncertainty and indicates that it can interpret statistical data for various images, including photographic images, graphic images, and medical images. In order to directly prove the efficacy of the model, an image encoder called Embedded Prediction Wavelet Image Coder (EPWIC) was constructed in their research. The subband coefficients use a non-adaptive arithmetic coder to encode a bit plane at a time. The encoder uses the conditional probability calculated from the model to sort the bit plane using a greedy algorithm. The algorithm considers the MSE reduction for each coded bit. The decoder uses a statistical model to predict coefficient values based on the bits it has received. Although the model is simple, the rate-distortion performance of the encoder is roughly equivalent to the best image encoder in the literature.
From the existing research results, we find that today’s digital image-based application research has achieved fruitful results. However, this kind of results mainly focus on methods, such as deep learning [ 20 , 21 ], genetic algorithm [ 22 , 23 ], fuzzy theory, etc. [ 24 , 25 ], which also includes the method of wavelet analysis. However, the biggest problem in the existing image application research is that although the existing research on digital multimedia has achieved good research results, there is also a problem. Digital multimedia processing technology is an organic whole. From denoising, compression, storage, encryption, decryption to retrieval, it should be a whole, but the current research results basically study a certain part of this whole. Therefore, although one method is superior in one of the links, it is not necessary whether this method will be suitable for other links. Therefore, in order to solve this problem, this thesis takes digital image as the research object; realizes unified modeling by three main steps of encryption, compression, and retrieval in image processing; and studies the image processing capability of multiple steps by one method.
Wavelet transform is a commonly used digital signal processing method. Since the existing digital signals are mostly composed of multi-frequency signals, there are noise signals, secondary signals, and main signals in the signal. In the image processing, there are also many research teams using wavelet transform as a processing method, introducing their own research and achieving good results. So, can we use wavelet transform as a method to build a model suitable for a variety of image processing applications?
In this paper, the wavelet transform is used as a method to establish the denoising encryption and compression model in the image processing process, and the captured image is simulated. The results show that the same wavelet transform parameters have achieved good results for different image processing applications.
2.1 Image binarization processing method
The gray value of the point of the image ranges from 0 to 255. In the image processing, in order to facilitate the further processing of the image, the frame of the image is first highlighted by the method of binarization. The so-called binarization is to map the point gray value of the image from the value space of 0–255 to the value of 0 or 255. In the process of binarization, threshold selection is a key step. The threshold used in this paper is the maximum between-class variance method (OTSU). The so-called maximum inter-class variance method means that for an image, when the segmentation threshold of the current scene and the background is t , the pre-attraction image ratio is w0, the mean value is u0, the background point is the image ratio w1, and the mean value is u1. Then the mean of the entire image is:
The objective function can be established according to formula 1:
The OTSU algorithm makes g ( t ) take the global maximum, and the corresponding t when g ( t ) is maximum is called the optimal threshold.
2.2 Wavelet transform method
Wavelet transform (WT) is a research result of the development of Fourier transform technology, and the Fourier transform is only transformed into different frequencies. The wavelet transform not only has the local characteristics of the Fourier transform but also contains the transform frequency result. The advantage of not changing with the size of the window. Therefore, compared with the Fourier transform, the wavelet transform is more in line with the time-frequency transform. The biggest characteristic of the wavelet transform is that it can better represent the local features of certain features with frequency, and the scale of the wavelet transform can be different. The low-frequency and high-frequency division of the signal makes the feature more focused. This paper mainly uses wavelet transform to analyze the image in different frequency bands to achieve the effect of frequency analysis. The method of wavelet transform can be expressed as follows:
Where ψ ( t ) is the mother wavelet, a is the scale factor, and τ is the translation factor.
Because the image signal is a two-dimensional signal, when using wavelet transform for image analysis, it is necessary to generalize the wavelet transform to two-dimensional wavelet transform. Suppose the image signal is represented by f ( x , y ), ψ ( x , y ) represents a two-dimensional basic wavelet, and ψ a , b , c ( x , y ) represents the scale and displacement of the basic wavelet, that is, ψ a , b , c ( x , y ) can be calculated by the following formula:
According to the above definition of continuous wavelet, the two-dimensional continuous wavelet transform can be calculated by the following formula:
Where \( \overline{\psi \left(x,y\right)} \) is the conjugate of ψ ( x , y ).
2.3 Digital water mark
According to different methods of use, digital watermarking technology can be divided into the following types:
Spatial domain approach: A typical watermarking algorithm in this type of algorithm embeds information into the least significant bits (LSB) of randomly selected image points, which ensures that the embedded watermark is invisible. However, due to the use of pixel bits whose images are not important, the robustness of the algorithm is poor, and the watermark information is easily destroyed by filtering, image quantization, and geometric deformation operations. Another common method is to use the statistical characteristics of the pixels to embed the information in the luminance values of the pixels.
The method of transforming the domain: first calculate the discrete cosine transform (DCT) of the image, and then superimpose the watermark on the front k coefficient with the largest amplitude in the DCT domain (excluding the DC component), usually the low-frequency component of the image. If the first k largest components of the DCT coefficients are represented as D =, i = 1, ..., k, and the watermark is a random real sequence W =, i = 1, ..., k obeying the Gaussian distribution, then the watermark embedding algorithm is di = di(1 + awi), where the constant a is a scale factor that controls the strength of the watermark addition. The watermark image I is then obtained by inverse transforming with a new coefficient. The decoding function calculates the discrete cosine transform of the original image I and the watermark image I * , respectively, and extracts the embedded watermark W * , and then performs correlation test to determine the presence or absence of the watermark.
Compressed domain algorithm: The compressed domain digital watermarking system based on JPEG and MPEG standards not only saves a lot of complete decoding and re-encoding process but also has great practical value in digital TV broadcasting and video on demand (VOD). Correspondingly, watermark detection and extraction can also be performed directly in the compressed domain data.
The wavelet transform used in this paper is the method of transform domain. The main process is: assume that x ( m , n ) is a grayscale picture of M * N , the gray level is 2 a , where M , N and a are positive integers, and the range of values of m and n is defined as follows: 1 ≤ m ≤ M , 1 ≤ n ≤ N . For wavelet decomposition of this image, if the number of decomposition layers is L ( L is a positive integer), then 3* L high-frequency partial maps and a low-frequency approximate partial map can be obtained. Then X k , L can be used to represent the wavelet coefficients, where L is the number of decomposition layers, and K can be represented by H , V , and D , respectively, representing the horizontal, vertical, and diagonal subgraphs. Because the sub-picture distortion of the low frequency is large, the picture embedded in the watermark is removed from the picture outside the low frequency.
In order to realize the embedded digital watermark, we must first divide X K , L ( m i , n j ) into a certain size, and use B ( s , t ) to represent the coefficient block of size s * t in X K , L ( m i , n j ). Then the average value can be expressed by the following formula:
Where ∑ B ( s , t ) is the cumulative sum of the magnitudes of the coefficients within the block.
The embedding of the watermark sequence w is achieved by the quantization of AVG.
The interval of quantization is represented by Δ l according to considerations of robustness and concealment. For the low-level L th layer, since the coefficient amplitude is large, a larger interval can be set. For the other layers, starting from the L -1 layer, they are successively decremented.
According to w i = {0, 1}, AVG is quantized to the nearest singular point, even point, D ( i , j ) is used to represent the wavelet coefficients in the block, and the quantized coefficient is represented by D ( i , j ) ' , where i = 1, 2,. .., s ; j = 1,2,..., t . Suppose T = AVG /Δ l , TD = rem(| T |, 2), where || means rounding and rem means dividing by 2 to take the remainder.
According to whether TD and w i are the same, the calculation of the quantized wavelet coefficient D ( i , j ) ' can be as follows:
Using the same wavelet base, an image containing the watermark is generated by inverse wavelet transform, and the wavelet base, the wavelet decomposition layer number, the selected coefficient region, the blocking method, the quantization interval, and the parity correspondence are recorded to form a key.
The extraction of the watermark is determined by the embedded method, which is the inverse of the embedded mode. First, wavelet transform is performed on the image to be detected, and the position of the embedded watermark is determined according to the key, and the inverse operation of the scramble processing is performed on the watermark.
2.4 Evaluation method
Filter normalized mean square error.
In order to measure the effect before and after filtering, this paper chooses the normalized mean square error M description. The calculation method of M is as follows:
where N 1 and N 2 are Pixels before and after normalization.
Normalized cross-correlation function
The normalized cross-correlation function is a classic algorithm of image matching algorithm, which can be used to represent the similarity of images. The normalized cross-correlation is determined by calculating the cross-correlation metric between the reference map and the template graph, generally expressed by NC( i , j ). If the NC value is larger, it means that the similarity between the two is greater. The calculation formula for the cross-correlation metric is as follows:
where T ( m , n ) is the n th row of the template image, the m th pixel value; S ( i , j ) is the part under the template cover, and i , j is the coordinate of the lower left corner of the subgraph in the reference picture S.
Normalize the above formula NC according to the following formula:
Peak signal-to-noise ratio
Peak signal-to-noise ratio is often used as a measure of signal reconstruction quality in areas such as image compression, which is often simply defined by mean square error (MSE). Two m × n monochrome images I and K , if one is another noise approximation, then their mean square error is defined as:
Then the peak signal-to-noise ratio PSNR calculation method is:
Where Max is the maximum value of the pigment representing the image.
Information entropy
For a digital signal of an image, the frequency of occurrence of each pixel is different, so it can be considered that the image digital signal is actually an uncertainty signal. For image encryption, the higher the uncertainty of the image, the more the image tends to be random, the more difficult it is to crack. The lower the rule, the more regular it is, and the more likely it is to be cracked. For a grayscale image of 256 levels, the maximum value of information entropy is 8, so the more the calculation result tends to be 8, the better.
The calculation method of information entropy is as follows:
Correlation
Correlation is a parameter describing the relationship between two vectors. This paper describes the relationship between two images before and after image encryption by correlation. Assuming p ( x , y ) represents the correlation between pixels before and after encryption, the calculation method of p ( x , y ) can be calculated by the following formula:
3 Experiment
3.1 image parameter.
The images used in this article are all from the life photos, the shooting tool is Huawei meta 10, the picture size is 1440*1920, the picture resolution is 96 dbi, the bit depth is 24, no flash mode, there are 300 pictures as simulation pictures, all of which are life photos, and no special photos.
3.2 System environment
The computer system used in this simulation is Windows 10, and the simulation software used is MATLAB 2014B.
3.3 Wavelet transform-related parameters
For unified modeling, the wavelet decomposition used in this paper uses three layers of wavelet decomposition, and Daubechies is chosen as the wavelet base. The Daubechies wavelet is a wavelet function constructed by the world-famous wavelet analyst Ingrid Daubechies. They are generally abbreviated as dbN, where N is the order of the wavelet. The support region in the wavelet function Ψ( t ) and the scale function ϕ ( t ) is 2 N-1, and the vanishing moment of Ψ( t ) is N . The dbN wavelet has good regularity, that is, the smooth error introduced by the wavelet as a sparse basis is not easy to be detected, which makes the signal reconstruction process smoother. The characteristic of the dbN wavelet is that the order of the vanishing moment increases with the increase of the order (sequence N), wherein the higher the vanishing moment, the better the smoothness, the stronger the localization ability of the frequency domain, and the better the band division effect. However, the support of the time domain is weakened, and the amount of calculation is greatly increased, and the real-time performance is deteriorated. In addition, except for N = 1, the dbN wavelet does not have symmetry (i.e., nonlinear phase), that is, a certain phase distortion is generated when the signal is analyzed and reconstructed. N = 3 in this article.
4 Results and discussion
4.1 results 1: image filtering using wavelet transform.
In the process of image recording, transmission, storage, and processing, it is possible to pollute the image signal. The digital signal transmitted to the image will appear as noise. These noise data will often become isolated pixels. One-to-one isolated points, although they do not destroy the overall external frame of the image, but because these isolated points tend to be high in frequency, they are portable on the image as a bright spot, which greatly affects the viewing quality of the image, so to ensure the effect of image processing, the image must be denoised. The effective method of denoising is to remove the noise of a certain frequency of the image by filtering, but the denoising must ensure that the noise data can be removed without destroying the image. Figure 1 is the result of filtering the graph using the wavelet transform method. In order to test the wavelet filtering effect, this paper adds Gaussian white noise to the original image. Comparing the white noise with the frequency analysis of the original image, it can be seen that after the noise is added, the main image frequency segment of the original image is disturbed by the noise frequency, but after filtering using the wavelet transform, the frequency band of the main frame of the original image appears again. However, the filtered image does not change significantly compared to the original image. The normalized mean square error before and after filtering is calculated, and the M value before and after filtering is 0.0071. The wavelet transform is well protected to protect the image details, and the noise data is better removed (the white noise is 20%).
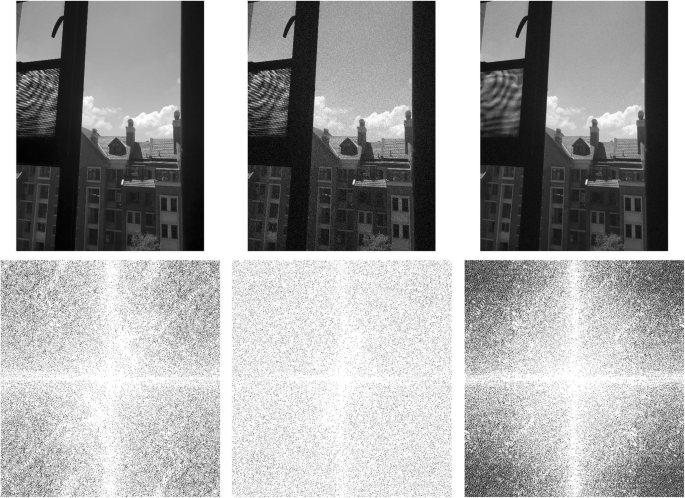
Image denoising results comparison. (The first row from left to right are the original image, plus the noise map and the filtered map. The second row from left to right are the frequency distribution of the original image, the frequency distribution of the noise plus the filtered Frequency distribution)
4.2 Results 2: digital watermark encryption based on wavelet transform
As shown in Fig. 2 , the watermark encryption process based on wavelet transform can be seen from the figure. Watermarking the image by wavelet transform does not affect the structure of the original image. The noise is 40% of the salt and pepper noise. For the original image and the noise map, the wavelet transform method can extract the watermark well.
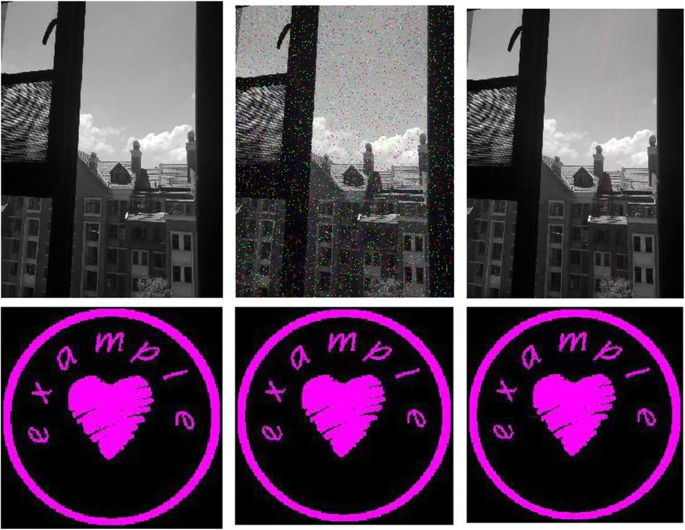
Comparison of digital watermark before and after. (The first row from left to right are the original image, plus noise and watermark, and the noise is removed; the second row are the watermark original, the watermark extracted from the noise plus watermark, and the watermark extracted after denoising)
According to the method described in this paper, the image correlation coefficient and peak-to-noise ratio of the original image after watermarking are calculated. The correlation coefficient between the original image and the watermark is 0.9871 (the first column and the third column in the first row in the figure). The watermark does not destroy the structure of the original image. The signal-to-noise ratio of the original picture is 33.5 dB, and the signal-to-noise ratio of the water-jet printing is 31.58SdB, which proves that the wavelet transform can achieve watermark hiding well. From the second row of watermarking results, the watermark extracted from the image after noise and denoising, and the original watermark correlation coefficient are (0.9745, 0.9652). This shows that the watermark signal can be well extracted after being hidden by the wavelet transform.
4.3 Results 3: image encryption based on wavelet transform
In image transmission, the most common way to protect image content is to encrypt the image. Figure 3 shows the process of encrypting and decrypting an image using wavelet transform. It can be seen from the figure that after the image is encrypted, there is no correlation with the original image at all, but the decrypted image of the encrypted image reproduces the original image.
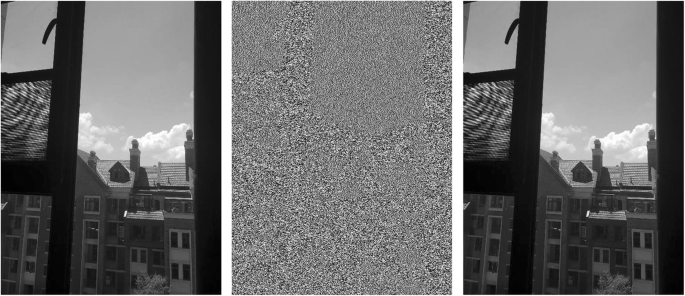
Image encryption and decryption process diagram comparison. (The left is the original image, the middle is the encrypted image, the right is the decryption map)
The information entropy of Fig. 3 is calculated. The results show that the information entropy of the original image is 3.05, the information entropy of the decrypted graph is 3.07, and the information entropy of the encrypted graph is 7.88. It can be seen from the results of information entropy that before and after encryption. The image information entropy is basically unchanged, but the information entropy of the encrypted image becomes 7.88, indicating that the encrypted image is close to a random signal and has good confidentiality.
4.4 Result 4: image compression
Image data can be compressed because of the redundancy in the data. The redundancy of image data mainly manifests as spatial redundancy caused by correlation between adjacent pixels in an image; time redundancy due to correlation between different frames in an image sequence; spectral redundancy due to correlation of different color planes or spectral bands. The purpose of data compression is to reduce the number of bits required to represent the data by removing these data redundancy. Since the amount of image data is huge, it is very difficult to store, transfer, and process, so the compression of image data is very important. Figure 4 shows the result of two compressions of the original image. It can be seen from the figure that although the image is compressed, the main frame of the image does not change, but the image sharpness is significantly reduced. The Table 1 shows the compressed image properties.
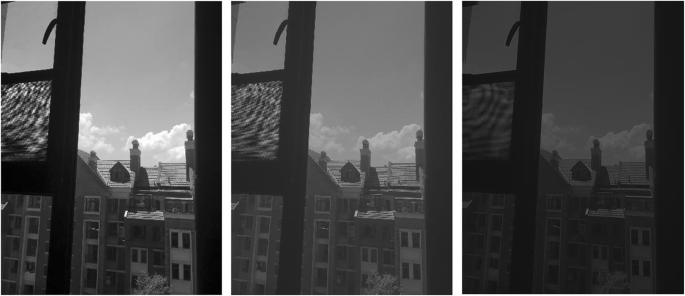
Image comparison before and after compression. (left is the original image, the middle is the first compression, the right is the second compression)
It can be seen from the results in Table 1 that after multiple compressions, the size of the image is significantly reduced and the image is getting smaller and smaller. The original image needs 2,764,800 bytes, which is reduced to 703,009 after a compression, which is reduced by 74.5%. After the second compression, only 182,161 is left, which is 74.1% lower. It can be seen that the wavelet transform can achieve image compression well.
5 Conclusion
With the development of informatization, today’s era is an era full of information. As the visual basis of human perception of the world, image is an important means for humans to obtain information, express information, and transmit information. Digital image processing, that is, processing images with a computer, has a long history of development. Digital image processing technology originated in the 1920s, when a photo was transmitted from London, England to New York, via a submarine cable, using digital compression technology. First of all, digital image processing technology can help people understand the world more objectively and accurately. The human visual system can help humans get more than 3/4 of the information from the outside world, and images and graphics are the carriers of all visual information, despite the identification of the human eye. It is very powerful and can recognize thousands of colors, but in many cases, the image is blurred or even invisible to the human eye. Image enhancement technology can make the blurred or even invisible image clear and bright. There are also some relevant research results on this aspect of research, which proves that relevant research is feasible [ 26 , 27 ].
It is precisely because of the importance of image processing technology that many researchers have begun research on image processing technology and achieved fruitful results. However, with the deepening of image processing technology research, today’s research has a tendency to develop in depth, and this depth is an in-depth aspect of image processing technology. However, the application of image processing technology is a system engineering. In addition to the deep requirements, there are also systematic requirements. Therefore, if the unified model research on multiple aspects of image application will undoubtedly promote the application of image processing technology. Wavelet transform has been successfully applied in many fields of image processing technology. Therefore, this paper uses wavelet transform as a method to establish a unified model based on wavelet transform. Simulation research is carried out by filtering, watermark hiding, encryption and decryption, and image compression of image processing technology. The results show that the model has achieved good results.
Abbreviations
Cellular automata
Computer generated hologram
Discrete cosine transform
Embedded Prediction Wavelet Image Coder
Human visual system
Least significant bits
Video on demand
Wavelet transform
H.W. Zhang, The research and implementation of image Denoising method based on Matlab[J]. Journal of Daqing Normal University 36 (3), 1-4 (2016)
J.H. Hou, J.W. Tian, J. Liu, Analysis of the errors in locally adaptive wavelet domain wiener filter and image Denoising[J]. Acta Photonica Sinica 36 (1), 188–191 (2007)
Google Scholar
M. Lebrun, An analysis and implementation of the BM3D image Denoising method[J]. Image Processing on Line 2 (25), 175–213 (2012)
Article Google Scholar
A. Fathi, A.R. Naghsh-Nilchi, Efficient image Denoising method based on a new adaptive wavelet packet thresholding function[J]. IEEE transactions on image processing a publication of the IEEE signal processing. Society 21 (9), 3981 (2012)
MATH Google Scholar
X. Zhang, X. Feng, W. Wang, et al., Gradient-based wiener filter for image denoising [J]. Comput. Electr. Eng. 39 (3), 934–944 (2013)
T. Chen, K.K. Ma, L.H. Chen, Tri-state median filter for image denoising.[J]. IEEE Transactions on Image Processing A Publication of the IEEE Signal Processing Society 8 (12), 1834 (1999)
S.M.M. Rahman, M.K. Hasan, Wavelet-domain iterative center weighted median filter for image denoising[J]. Signal Process. 83 (5), 1001–1012 (2003)
Article MATH Google Scholar
H.L. Eng, K.K. Ma, Noise adaptive soft-switching median filter for image denoising[C]// IEEE International Conference on Acoustics, Speech, and Signal Processing, 2000. ICASSP '00. Proceedings. IEEE 4 , 2175–2178 (2000)
S.G. Chang, B. Yu, M. Vetterli, Adaptive wavelet thresholding for image denoising and compression[J]. IEEE transactions on image processing a publication of the IEEE signal processing. Society 9 (9), 1532 (2000)
M. Kivanc Mihcak, I. Kozintsev, K. Ramchandran, et al., Low-complexity image Denoising based on statistical modeling of wavelet Coecients[J]. IEEE Signal Processing Letters 6 (12), 300–303 (1999)
J.H. Wu, F.Z. Lin, Image authentication based on digital watermarking[J]. Chinese Journal of Computers 9 , 1153–1161 (2004)
MathSciNet Google Scholar
A. Wakatani, Digital watermarking for ROI medical images by using compressed signature image[C]// Hawaii international conference on system sciences. IEEE (2002), pp. 2043–2048
A.H. Paquet, R.K. Ward, I. Pitas, Wavelet packets-based digital watermarking for image verification and authentication [J]. Signal Process. 83 (10), 2117–2132 (2003)
Z. Wen, L.I. Taoshen, Z. Zhang, An image encryption technology based on chaotic sequences[J]. Comput. Eng. 31 (10), 130–132 (2005)
Y.Y. Wang, Y.R. Wang, Y. Wang, et al., Optical image encryption based on binary Fourier transform computer-generated hologram and pixel scrambling technology[J]. Optics & Lasers in Engineering 45 (7), 761–765 (2007)
X.Y. Zhang, C. Wang, S.M. Li, et al., Image encryption technology on two-dimensional cellular automata[J]. Journal of Optoelectronics Laser 19 (2), 242–245 (2008)
A.S. Lewis, G. Knowles, Image compression using the 2-D wavelet transform[J]. IEEE Trans. Image Process. 1 (2), 244–250 (2002)
R.A. Devore, B. Jawerth, B.J. Lucier, Image compression through wavelet transform coding[J]. IEEE Trans.inf.theory 38 (2), 719–746 (1992)
Article MathSciNet MATH Google Scholar
R.W. Buccigrossi, E.P. Simoncelli, Image compression via joint statistical characterization in the wavelet domain[J]. IEEE transactions on image processing a publication of the IEEE signal processing. Society 8 (12), 1688–1701 (1999)
A.A. Cruzroa, J.E. Arevalo Ovalle, A. Madabhushi, et al., A deep learning architecture for image representation, visual interpretability and automated basal-cell carcinoma cancer detection. Med Image Comput Comput Assist Interv. 16 , 403–410 (2013)
S.P. Mohanty, D.P. Hughes, M. Salathé, Using deep learning for image-based plant disease detection[J]. Front. Plant Sci. 7 , 1419 (2016)
B. Sahiner, H. Chan, D. Wei, et al., Image feature selection by a genetic algorithm: application to classification of mass and normal breast tissue[J]. Med. Phys. 23 (10), 1671 (1996)
B. Bhanu, S. Lee, J. Ming, Adaptive image segmentation using a genetic algorithm[J]. IEEE Transactions on Systems Man & Cybernetics 25 (12), 1543–1567 (2002)
Y. Egusa, H. Akahori, A. Morimura, et al., An application of fuzzy set theory for an electronic video camera image stabilizer[J]. IEEE Trans. Fuzzy Syst. 3 (3), 351–356 (1995)
K. Hasikin, N.A.M. Isa, Enhancement of the low contrast image using fuzzy set theory[C]// Uksim, international conference on computer modelling and simulation. IEEE (2012), pp. 371–376
P. Yang, Q. Li, Wavelet transform-based feature extraction for ultrasonic flaw signal classification. Neural Comput. & Applic. 24 (3–4), 817–826 (2014)
R.K. Lama, M.-R. Choi, G.-R. Kwon, Image interpolation for high-resolution display based on the complex dual-tree wavelet transform and hidden Markov model. Multimedia Tools Appl. 75 (23), 16487–16498 (2016)
Download references
Acknowledgements
The authors thank the editor and anonymous reviewers for their helpful comments and valuable suggestions.
This work was supported by
Shandong social science planning research project in 2018
Topic: The Application of Shandong Folk Culture in Animation in The View of Digital Media (No. 18CCYJ14).
Shandong education science 12th five-year plan 2015
Topic: Innovative Research on Stop-motion Animation in The Digital Media Age (No. YB15068).
Shandong education science 13th five-year plan 2016–2017
Approval of “Ports and Arts Education Special Fund”: BCA2017017.
Topic: Reform of Teaching Methods of Hand Drawn Presentation Techniques (No. BCA2017017).
National Research Youth Project of state ethnic affairs commission in 2018
Topic: Protection and Development of Villages with Ethnic Characteristics Under the Background of Rural Revitalization Strategy (No. 2018-GMC-020).
Availability of data and materials
Authors can provide the data.
About the authors
Zaozhuang University, No. 1 Beian Road., Shizhong District, Zaozhuang City, Shandong, P.R. China.
Lina, Zhang was born in Jining, Shandong, P.R. China, in 1983. She received a Master degree from Bohai University, P.R. China. Now she works in School of Media, Zaozhuang University, P.R. China. Her research interests include animation and Digital media art.
Lijuan, Zhang was born in Jining, Shandong, P.R. China, in 1983. She received a Master degree from Jingdezhen Ceramic Institute, P.R. China. Now she works in School of Fine Arts and Design, Zaozhuang University, P.R. China. Her research interests include Interior design and Digital media art.
Liduo, Zhang was born in Zaozhuang, Shandong, P.R. China, in 1982. He received a Master degree from Monash University, Australia. Now he works in School of economics and management, Zaozhuang University. His research interests include Internet finance and digital media.
Author information
Authors and affiliations.
School of Media, Zaozhuang University, Zaozhuang, Shandong, China
School of Fine Arts and Design, Zaozhuang University, Zaozhuang, Shandong, China
Lijuan Zhang
School of Economics and Management, Zaozhuang University, Zaozhuang, Shandong, China
Liduo Zhang
You can also search for this author in PubMed Google Scholar
Contributions
All authors take part in the discussion of the work described in this paper. The author LZ wrote the first version of the paper. The author LZ and LZ did part experiments of the paper, LZ revised the paper in different version of the paper, respectively. All authors read and approved the final manuscript.
Corresponding author
Correspondence to Lijuan Zhang .
Ethics declarations
Competing interests.
The authors declare that they have no competing interests.
Publisher’s Note
Springer Nature remains neutral with regard to jurisdictional claims in published maps and institutional affiliations.
Rights and permissions
Open Access This article is distributed under the terms of the Creative Commons Attribution 4.0 International License ( http://creativecommons.org/licenses/by/4.0/ ), which permits unrestricted use, distribution, and reproduction in any medium, provided you give appropriate credit to the original author(s) and the source, provide a link to the Creative Commons license, and indicate if changes were made.
Reprints and permissions
About this article
Cite this article.
Zhang, L., Zhang, L. & Zhang, L. Application research of digital media image processing technology based on wavelet transform. J Image Video Proc. 2018 , 138 (2018). https://doi.org/10.1186/s13640-018-0383-6
Download citation
Received : 28 September 2018
Accepted : 23 November 2018
Published : 05 December 2018
DOI : https://doi.org/10.1186/s13640-018-0383-6
Share this article
Anyone you share the following link with will be able to read this content:
Sorry, a shareable link is not currently available for this article.
Provided by the Springer Nature SharedIt content-sharing initiative
- Image processing
- Digital watermark
- Image denoising
- Image encryption
- Image compression
IEEE Account
- Change Username/Password
- Update Address
Purchase Details
- Payment Options
- Order History
- View Purchased Documents
Profile Information
- Communications Preferences
- Profession and Education
- Technical Interests
- US & Canada: +1 800 678 4333
- Worldwide: +1 732 981 0060
- Contact & Support
- About IEEE Xplore
- Accessibility
- Terms of Use
- Nondiscrimination Policy
- Privacy & Opting Out of Cookies
A not-for-profit organization, IEEE is the world's largest technical professional organization dedicated to advancing technology for the benefit of humanity. © Copyright 2024 IEEE - All rights reserved. Use of this web site signifies your agreement to the terms and conditions.
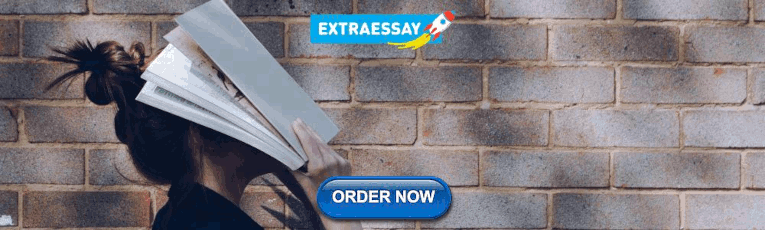
IMAGES
COMMENTS
Machine learning is a relatively new field. With the deepening of people's research in this field, the application of machine learning is increasingly extensive. On the other hand, with the advancement of science and technology, graphics have been an indispensable medium of information transmission, and image processing technology is also booming. However, the traditional image processing ...
Image processing is manipulation of an image that has been digitised and uploaded into a computer. Software programs modify the image to make it more useful, and can for example be used to enable ...
Image Processing: Research O pportunities and Challenges. Ravindra S. Hegadi. Department of Computer Science. Karnatak University, Dharwad-580003. ravindrahegadi@rediffmail. Abstract. Interest in ...
Abstract. Image Processing includes changing the nature of an image in order to improve its pictorial information for human interpretation, for autonomous machine perception. Digital image ...
Within the domain of image processing, a wide array of methodologies is dedicated to tasks including denoising, enhancement, segmentation, feature extraction, and classification. These techniques collectively address the challenges and opportunities posed by different aspects of image analysis and manipulation, enabling applications across various fields. Each of these methodologies ...
All kinds of image processing approaches. | Explore the latest full-text research PDFs, articles, conference papers, preprints and more on IMAGE PROCESSING. ... Publications related to Image ...
Image processing based modeling for Rosa roxburghii fruits mass and volume estimation. Zhiping Xie. , Junhao Wang. & Manyu Sun. Article. 05 July 2024 | Open Access.
With the recent advances in digital technology, there is an eminent integration of ML and image processing to help resolve complex problems. In this special issue, we received six interesting papers covering the following topics: image prediction, image segmentation, clustering, compressed sensing, variational learning, and dynamic light coding.
Section 4—Image Processing Developments: describes related work and different state-of-the-art approaches used by researchers to solve modern-day challenges. ... When we consider the volume of research developed, there is a clear increase in published research papers targeting image processing and DL, over the last decades. ...
Deep learning is a powerful multi-layer architecture that has important applications in image processing and text classification. This paper first introduces the development of deep learning and two important algorithms of deep learning: convolutional neural networks and recurrent neural networks. The paper then introduces three applications of deep learning for image recognition, image ...
This paper comprehensively overviews image and signal processing, including their fundamentals, advanced techniques, and applications. Image processing involves analyzing and manipulating digital images, while signal processing focuses on analyzing and interpreting signals in various domains. The fundamentals encompass digital signal representation, Fourier analysis, wavelet transforms ...
Technological advancements in computing multiple opportunities in a wide variety of fields that range from document analysis (Santosh, 2018), biomedical and healthcare informatics (Santosh et al., 2019; Santosh et al., 2021; Santosh and Gaur, 2021; Santosh and Joshi, 2021), and biometrics to intelligent language processing.These applications primarily leverage AI tools and/or techniques, where ...
The Call for Papers of the special issue was initially sent out to the participants of the 2018 conference (2nd International Conference on Recent Trends in Image Processing and Pattern Recognition). To attract high quality research articles, we also accepted papers for review from outside the conference event.
This Special Issue presents a forum for the publication of articles describing the use of classical and modern artificial intelligence methods in image processing applications. The main aim of this Special Issue is to capture recent contributions of high-quality papers focusing on advanced image processing and analysis applications, including ...
In this Special Issue, we invite authors to submit original research papers, reviews, and viewpoint articles that are related to recent advances at all levels of the applications and technologies of imaging and signal analysis. ... The Special Issue on "Digital Image Processing: Advanced Technologies and Applications" covers rising trends ...
Papers. TL;DR: The origins, challenges and solutions of NIH Image and ImageJ software are discussed, and how their history can serve to advise and inform other software projects. Abstract: For the past 25 years NIH Image and ImageJ software have been pioneers as open tools for the analysis of scientific images.
The image classification is a classical problem of image processing, computer vision and machine learning fields. In this paper we study the image classification using deep learning.
Abstract. Medical image processing provides core innovation for medical imaging. This paper is focused on recent developments from science to applications analyzing the past fifteen years of history of the proceedings of the German annual meeting on medical image processing (BVM). Furthermore, some members of the program committee present their ...
Dear Colleagues, The 5th International Conference on Recent Trends in Image Processing and Pattern Recognition (RTIP2R) aims to attract current and/or advanced research on image processing, pattern recognition, computer vision, and machine learning. The RTIP2R will take place at the Texas A&M University—Kingsville, Texas (USA), on November 22 ...
Explore the latest full-text research PDFs, articles, conference papers, preprints and more on DIGITAL IMAGE PROCESSING. Find methods information, sources, references or conduct a literature ...
With the development of information technology, people access information more and more rely on the network, and more than 80% of the information in the network is replaced by multimedia technology represented by images. Therefore, the research on image processing technology is very important, but most of the research on image processing technology is focused on a certain aspect. The research ...
In this paper we give a tutorial overview of the field of digital image processing. Following a brief discussion of some basic concepts in this area, image processing algorithms are presented with emphasis on fundamental techniques which are broadly applicable to a number of applications. In addition to several real-world examples of such techniques, we also discuss the applicability of ...
With the gradual improvement of artificial intelligence technology, image processing has become a common technology and is widely used in various fields to provide people with high-quality services. Starting from computer vision algorithms and image processing technologies, the computer vision display system is designed, and image distortion correction algorithms are explored for reference.