
An official website of the United States government
The .gov means it’s official. Federal government websites often end in .gov or .mil. Before sharing sensitive information, make sure you’re on a federal government site.
The site is secure. The https:// ensures that you are connecting to the official website and that any information you provide is encrypted and transmitted securely.
- Publications
- Account settings
- My Bibliography
- Collections
- Citation manager
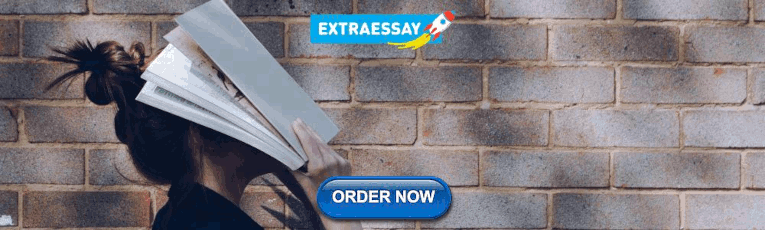
Save citation to file
Email citation, add to collections.
- Create a new collection
- Add to an existing collection
Add to My Bibliography
Your saved search, create a file for external citation management software, your rss feed.
- Search in PubMed
- Search in NLM Catalog
- Add to Search
The Role of Alcohol Dehydrogenase in Drug Metabolism: Beyond Ethanol Oxidation
Affiliations.
- 1 Pharmacokinetics, Dynamics and Metabolism, Pfizer Worldwide Research and Development, Groton, CT, 06340, USA. [email protected].
- 2 Pharmacokinetics, Dynamics and Metabolism, Pfizer Worldwide Research and Development, Groton, CT, 06340, USA.
- 3 Department of Chemistry, Stanford University, Stanford, CA, 94305, USA.
- PMID: 33415501
- DOI: 10.1208/s12248-020-00536-y
Alcohol dehydrogenases (ADHs) are most known for their roles in oxidation and elimination of ethanol. Although less known, ADHs also play a critical role in the metabolism of a number of drugs and metabolites that contain alcohol functional groups, such as abacavir (HIV/AIDS), hydroxyzine (antihistamine), and ethambutol (antituberculosis). ADHs consist of 7 gene family numbers and several genetic polymorphic forms. ADHs are cytosolic enzymes that are most abundantly found in the liver, although also present in other tissues including gastrointestinal tract and adipose. Marked species differences exist for ADHs including genes, proteins, enzymatic activity, and tissue distribution. The active site of ADHs is relatively small and cylindrical in shape. This results in somewhat narrow substrate specificity. Secondary alcohols are generally poor substrates for ADHs. In vitro-in vivo correlations for ADHs have not been established, partly due to insufficient clinical data. Fomepizole (4-methylpyrazole) is a nonspecific ADH inhibitor currently being used as an antidote for the treatment of methanol and ethylene glycol poisoning. Fomepizole also has the potential to treat intoxication of other substances of abuse by inhibiting ADHs to prevent formation of toxic metabolites. ADHs are inducible through farnesoid X receptor (FXR) and other transcription factors. Drug-drug interactions have been observed in the clinic for ADHs between ethanol and therapeutic drugs, and between fomepizole and ADH substrates. Future research in this area will provide additional insights about this class of complex, yet fascinating enzymes.
Keywords: ADH; Alcohol dehydrogenase; Drug metabolism; Drug-drug interactions; Genetic polymorphism; IVIVE; Species differences; Tissue distribution.
PubMed Disclaimer
Similar articles
- Genetic polymorphism and activities of human lung alcohol and aldehyde dehydrogenases: implications for ethanol metabolism and cytotoxicity. Yin SJ, Liao CS, Chen CM, Fan FT, Lee SC. Yin SJ, et al. Biochem Genet. 1992 Apr;30(3-4):203-15. doi: 10.1007/BF02399709. Biochem Genet. 1992. PMID: 1590750
- Oxidation of methanol, ethylene glycol, and isopropanol with human alcohol dehydrogenases and the inhibition by ethanol and 4-methylpyrazole. Lee SL, Shih HT, Chi YC, Li YP, Yin SJ. Lee SL, et al. Chem Biol Interact. 2011 May 30;191(1-3):26-31. doi: 10.1016/j.cbi.2010.12.005. Epub 2010 Dec 15. Chem Biol Interact. 2011. PMID: 21167143
- Human class I alcohol dehydrogenases catalyze the oxidation of glycols in the metabolism of norepinephrine. Mårdh G, Luehr CA, Vallee BL. Mårdh G, et al. Proc Natl Acad Sci U S A. 1985 Aug;82(15):4979-82. doi: 10.1073/pnas.82.15.4979. Proc Natl Acad Sci U S A. 1985. PMID: 3161078 Free PMC article.
- Alcohol dehydrogenases: a family of isozymes with differential functions. Holmes RS. Holmes RS. Alcohol Alcohol Suppl. 1994;2:127-30. Alcohol Alcohol Suppl. 1994. PMID: 8974326 Review.
- Natural alcohol exposure: is ethanol the main substrate for alcohol dehydrogenases in animals? Hernández-Tobías A, Julián-Sánchez A, Piña E, Riveros-Rosas H. Hernández-Tobías A, et al. Chem Biol Interact. 2011 May 30;191(1-3):14-25. doi: 10.1016/j.cbi.2011.02.008. Epub 2011 Feb 15. Chem Biol Interact. 2011. PMID: 21329681 Review.
- CNS Viral Infections-What to Consider for Improving Drug Treatment: A Plea for Using Mathematical Modeling Approaches. Sun M, Manson ML, Guo T, de Lange ECM. Sun M, et al. CNS Drugs. 2024 May;38(5):349-373. doi: 10.1007/s40263-024-01082-3. Epub 2024 Apr 5. CNS Drugs. 2024. PMID: 38580795 Free PMC article. Review.
- A phenome-wide association and Mendelian randomisation study of alcohol use variants in a diverse cohort comprising over 3 million individuals. Jennings MV, Martínez-Magaña JJ, Courchesne-Krak NS, Cupertino RB, Vilar-Ribó L, Bianchi SB, Hatoum AS, Atkinson EG, Giusti-Rodriguez P, Montalvo-Ortiz JL, Gelernter J, Artigas MS; 23andMe, Inc. Research Team; Elson SL, Edenberg HJ, Fontanillas P, Palmer AA, Sanchez-Roige S. Jennings MV, et al. EBioMedicine. 2024 May;103:105086. doi: 10.1016/j.ebiom.2024.105086. Epub 2024 Apr 4. EBioMedicine. 2024. PMID: 38580523 Free PMC article.
- Aldehyde-mediated inhibition of asparagine biosynthesis has implications for diabetes and alcoholism. John T, Saffoon N, Walsby-Tickle J, Hester SS, Dingler FA, Millington CL, McCullagh JSO, Patel KJ, Hopkinson RJ, Schofield CJ. John T, et al. Chem Sci. 2024 Jan 15;15(7):2509-2517. doi: 10.1039/d3sc06551k. eCollection 2024 Feb 14. Chem Sci. 2024. PMID: 38362406 Free PMC article.
- Preparation of Alcohol Dehydrogenase-Zinc Phosphate Hybrid Nanoflowers through Biomimetic Mineralization and Its Application in the Inhibitor Screening. Luo ML, Chen H, Chen GY, Wang S, Wang Y, Yang FQ. Luo ML, et al. Molecules. 2023 Jul 15;28(14):5429. doi: 10.3390/molecules28145429. Molecules. 2023. PMID: 37513303 Free PMC article.
- Cytosolic Enzymes Generate Cannabinoid Metabolites 7-Carboxycannabidiol and 11-Nor-9-carboxytetrahydrocannabinol. Beers JL, Authement AK, Isoherranen N, Jackson KD. Beers JL, et al. ACS Med Chem Lett. 2023 Apr 20;14(5):614-620. doi: 10.1021/acsmedchemlett.3c00017. eCollection 2023 May 11. ACS Med Chem Lett. 2023. PMID: 37197460 Free PMC article.
- Edenberg HJ, McClintick JN. Alcohol dehydrogenases, aldehyde dehydrogenases, and alcohol use disorders: a critical review. Alcohol Clin Exp Res. 2018;42(12):2281–97. https://doi.org/10.1111/acer.13904 . - DOI - PubMed - PMC
- Edenberg HJ, Bosron WF. Alcohol dehydrogenases. In: McQueen CA, editor. Comprehensive toxicology. 3nd ed. Oxford: Elsevier; 2018. p. 126–39.
- Bhatt DK, Gaedigk A, Pearce RE, Leeder JS, Prasad B. Age-dependent protein abundance of cytosolic alcohol and aldehyde dehydrogenases in human liver. Drug Metab Dispos. 2017;45(9):1044–8,S1-S34. https://doi.org/10.1124/dmd.117.076463 . - DOI - PubMed - PMC
- Parkinson A, Ogilvie BW, Buckley DB, Kazmi F, Parkinson O. Biotransformation of xenobiotics. In: Klaassen C, editor. The basic science of poisons. 9th ed. New York: McGraw-Hill Education; 2018. p. 243.
- Yang Z-N, Bosron WF, Hurley TD. Structure of human χχ alcohol dehydrogenase: a glutathione-dependent formaldehyde dehydrogenase. J Mol Biol. 1997;265(3):330–43. https://doi.org/10.1006/jmbi.1996.0731 . - DOI - PubMed
Publication types
- Search in MeSH
Related information
- Cited in Books
- PubChem Compound (MeSH Keyword)
LinkOut - more resources
Full text sources, other literature sources.
- scite Smart Citations
- MedlinePlus Health Information
- Citation Manager
NCBI Literature Resources
MeSH PMC Bookshelf Disclaimer
The PubMed wordmark and PubMed logo are registered trademarks of the U.S. Department of Health and Human Services (HHS). Unauthorized use of these marks is strictly prohibited.
Impact and relevance of alcohol dehydrogenase enantioselectivities on biotechnological applications
- Mini-Review
- Published: 15 February 2020
- Volume 104 , pages 2897–2909, ( 2020 )
Cite this article
- Afifa Ayu Koesoema ORCID: orcid.org/0000-0003-2744-4552 1 ,
- Daron M. Standley ORCID: orcid.org/0000-0003-4078-0817 2 ,
- Toshiya Senda ORCID: orcid.org/0000-0002-5153-928X 3 , 4 &
- Tomoko Matsuda ORCID: orcid.org/0000-0002-8934-8198 1
2524 Accesses
46 Citations
1 Altmetric
Explore all metrics
Alcohol dehydrogenases (ADHs) catalyze the reversible reduction of a carbonyl group to its corresponding alcohol. ADHs are widely employed for organic synthesis due to their lack of harm to the environment, broad substrate acceptance, and high enantioselectivity. This review focuses on the impact and relevance of ADH enantioselectivities on their biotechnological application. Stereoselective ADHs are beneficial to reduce challenging ketones such as ketones owning two bulky substituents or similar-sized substituents to the carbonyl carbon. Meanwhile, in cascade reactions, non-stereoselective ADHs can be utilized for the quantitative oxidation of racemic alcohol to ketone and dynamic kinetic resolution.
This is a preview of subscription content, log in via an institution to check access.
Access this article
Subscribe and save.
- Get 10 units per month
- Download Article/Chapter or eBook
- 1 Unit = 1 Article or 1 Chapter
- Cancel anytime
Price includes VAT (Russian Federation)
Instant access to the full article PDF.
Rent this article via DeepDyve
Institutional subscriptions
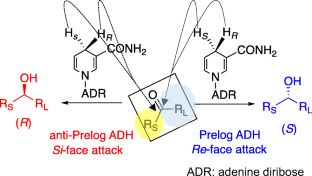
Similar content being viewed by others
Recent advances in biotechnological applications of alcohol dehydrogenases.

Efficient synthesis 1,4-cyclohexanedicarboxaldehyde by an engineered alcohol oxidase
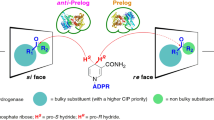
Biocatalytic characterization of an alcohol dehydrogenase variant deduced from Lactobacillus kefir in asymmetric hydrogen transfer
Abe S, Ijiri H, Negishi H, Yamanaka H, Sasaki K, Hirata K, Mori H, Ueno T (2015) Design of enzyme-encapsulated protein containers by in vivo crystal engineering. Adv Mater 27:7951–7956. https://doi.org/10.1002/adma.201503827
Article PubMed CAS Google Scholar
Agudo RR, Roiban GD, Reetz MT (2013) Induced axial chirality in biocatalytic asymmetric ketone reduction. J Am Chem Soc 135:1665–1668. https://doi.org/10.1021/ja3092517
Böhmer W, Knaus T, Mutti FG (2018) Hydrogen-borrowing alcohol bioamination with coimmobilized dehydrogenases. ChemCatChem 10:731–735. https://doi.org/10.1002/cctc.201701366
Article PubMed PubMed Central CAS Google Scholar
Bong YK, Vogel M, Collier SJ, Mitchell V, Mavinahalli J (2016) Ketoreductase-mediated stereoselective route to alpha chloroalcohols. U.S. Patent Application 2016/0160187:A1
Boukachabia M, Zeror S, Collin J, Fiaud JC, Zouioueche LA (2011) Screening method for the evaluation of asymmetric catalysts for the reduction of aliphatic ketones. Tetrahedron Lett 52:1485–1489. https://doi.org/10.1016/j.tetlet.2011.01.112
Article CAS Google Scholar
Bsharat O, Musa MM, Vieille C, Oladepo SA, Takahashi M, Hamdan SM (2017) Asymmetric reduction of substituted 2-tetralones by Thermoanaerobacter pseudoethanolicus secondary alcohol dehydrogenase. ChemCatChem 9:1487–1493. https://doi.org/10.1002/cctc.201601618
Chen C-S, Zhou B, Girdaukas G, Shieh W-R, Middlesworth F, Gopalan AS, Sih CJ (1984) Stereochemical control of yeast reductions. 2. Quantitative treatment of the kinetics of competing enzyme systems for a single substrate. Bioorg Chem 12:98–117. https://doi.org/10.1016/0045-2068(84)90021-x
Chen FF, Liu YY, Zheng GW, Xu JH (2015) Asymmetric amination of secondary alcohols by using a redox-neutral two-enzyme cascade. ChemCatChem 7:3838–3841. https://doi.org/10.1002/cctc.201500785
Chen X, Xu L, Wang A, Li H, Wang C, Pei X, Zhang P, Wu SG (2019a) Efficient synthesis of the key chiral alcohol intermediate of crizotinib using dual-enzyme@CaHPO 4 hybrid nanoflowers assembled by mimetic biomineralization. J Chem Technol Biotechnol 94:236–243. https://doi.org/10.1002/jctb.5769
Chen X, Zhang H, Maria-Solano MA, Liu W, Li J, Feng J, Liu X, Osuna S, Guo R-T, Wu Q, Zhu D, Ma Y (2019b) Efficient reductive desymmetrization of bulky 1,3-cyclodiketones enabled by structure-guided directed evolution of a carbonyl reductase. Nat Catal 2:931–941. https://doi.org/10.1038/s41929-019-0347-y
de Gonzalo G, Lavandera I, Gotor V (2011) Recent advances in biocatalysis applied to organic synthesis. Catal Methods Asymmetric Synth. 491-527. https://doi.org/10.1002/9781118087992.ch12
Chapter Google Scholar
de Gonzalo G, Alcántara AR, Domínguez de María P (2019) Cyclopentyl methyl ether (CPME): a versatile eco-friendly solvent for applications in biotechnology and biorefineries. ChemSusChem 12:2083–2097. https://doi.org/10.1002/cssc.201900079
Devalia JL, Hanotte F, Baltes E, De Vos C (2001) A randomized, double-blind, crossover comparison among cetirizine, levocetirizine, and ucb 28557 on histamine-induced cutaneous responses in healthy adult volunteers. Allergy 56:50–57. https://doi.org/10.1034/j.1398-9995.2001.00726.x
Döbber J, Gerlach T, Offermann H, Rother D, Pohl M (2018) Closing the gap for efficient immobilization of biocatalysts in continuous processes: HaloTag™ fusion enzymes for a continuous enzymatic cascade towards a vicinal chiral diol. Green Chem 20:544–552. https://doi.org/10.1039/c7gc03225k
Emmanuel MA, Greenberg NR, Oblinsky DG, Hyster TK (2016) Accessing non-natural reactivity by irradiating nicotinamide-dependent enzymes with light. Nature 540:414–417. https://doi.org/10.1038/nature20569
Encell LP (2012) Development of a dehalogenase-based protein fusion tag capable of rapid, selective and covalent attachment to customizable ligands. Curr Chem Genomics 6:55–71. https://doi.org/10.2174/1875397301206010055
Article PubMed PubMed Central Google Scholar
Erdmann V, Mackfeld U, Rother D, Jakoblinnert A (2014) Enantioselective, continuous ( R )- and ( S )-2-butanol synthesis: achieving high space-time yields with recombinant E. coli cells in a micro-aqueous, solvent-free reaction system. J Biotechnol 191:106–112. https://doi.org/10.1016/j.jbiotec.2014.06.032
Gandomkar S, Żądło-Dobrowolska A, Kroutil W (2019) Extending designed linear biocatalytic cascades for organic synthesis. ChemCatChem 11:225–243. https://doi.org/10.1002/cctc.201801063
Ge J, Lei J, Zare RN (2012) Protein-inorganic hybrid nanoflowers. Nat Nanotechnol 7:428–432. https://doi.org/10.1038/nnano.2012.80
Gotor-Fernández V, Paul CE (2019) Deep eutectic solvents for redox biocatalysis. J Biotechnol 293:24–35. https://doi.org/10.1016/j.jbiotec.2018.12.018
Gruber CC, Nestl BM, Gross J, Hildebrandt P, Bornscheuer UT, Faber K, Kroutil W (2007) Emulation of racemase activity by employing a pair of stereocomplementary biocatalysts. 8271–8276. https://doi.org/10.1002/chem.200700528
Harada T, Osawa T (1995) Enantio-differentiating hydrogenation of 2-butanone : distinction between CH 3 and C 2 H 5 with a modified nickel catalyst. In: Jannes G, Dubois V (eds) Chiral reactions in heterogeneous catalysis. Springer US, Boston, pp 83–88
Heiss C, Phillips RS (2000) Asymmetric reduction of ethynyl ketones and ethynylketoesters by secondary alcohol dehydrogenase from Thermoanaerobacter ethanolicus . J Chem Soc Perkin Trans 1(5):2821–2825. https://doi.org/10.1039/b001329n
Article Google Scholar
Hoang HN, Matsuda T (2015) Liquid carbon dioxide as an effective solvent for immobilized Candida antarctica lipase B catalyzed transesterification. Tetrahedron Lett 56:639–641. https://doi.org/10.1016/j.tetlet.2014.12.080
Hoang HN, Nagashima Y, Mori S, Kagechika H, Matsuda T (2017) CO 2 -expanded bio-based liquids as novel solvents for enantioselective biocatalysis. Tetrahedron 73:2984–2989. https://doi.org/10.1016/j.tet.2017.04.024
Karume I, Musa MM, Bsharat O, Takahashi M, Hamdan SM, El Ali B (2016) Dual enzymatic dynamic kinetic resolution by Thermoanaerobacter ethanolicus secondary alcohol dehydrogenase and Candida antarctica lipase B. RSC Adv 6:96616–96622. https://doi.org/10.1039/c6ra18895h
Keinan E, Hafeli EK, Seth KK, Lamed R (1986) Thermostable enzymes in organic synthesis. 2. Asymmetric reduction of ketones with alcohol dehydrogenase from Thermoanaerobium brockii . J Am Chem Soc 108:162–169. https://doi.org/10.1021/ja00261a026
Kizaki N, Yasohara Y, Hasegawa J, Wada M, Kataoka M, Shimizu S (2001) Synthesis of optically pure ethyl ( S )-4-chloro-3-hydroxybutanoate by Escherichia coli transformant cells coexpressing the carbonyl reductase and glucose dehydrogenase genes. Appl Microbiol Biotechnol 55:590–595. https://doi.org/10.1007/s002530100599
Koesoema AA, Sugiyama Y, Sriwong KT, Xu Z, Verina S (2019a) Reversible control of enantioselectivity by the length of ketone substituent in biocatalytic reduction. Appl Microbiol Biotechnol 103:9529–9541. https://doi.org/10.1007/s00253-019-10206-5
Koesoema AA, Sugiyama Y, Xu Z, Standley DM, Senda M, Senda T, Matsuda T (2019b) Structural basis for a highly ( S )-enantioselective reductase towards aliphatic ketones with only one carbon difference between side chain. Appl Microbiol Biotechnol 103:9543–9553. https://doi.org/10.1007/s00253-019-10093-w
Kowalski AE, Johnson LB, Dierl HK, Park S, Huber TR, Snow CD (2019) Porous protein crystals as scaffolds for enzyme immobilization. Biomater Sci 7:1898–1904. https://doi.org/10.1039/c8bm01378k
Lavandera I, Kern A, Ferreira-silva B, Glieder A, De Wildeman S, Kroutil W (2008) Stereoselective bioreduction of bulky-bulky ketones by a novel ADH from Ralstonia sp . J Org Chem 73:6003–6005. https://doi.org/10.1021/jo800849d
Li H, Zhu D, Hua L, Biehl ER (2009) Enantioselective reduction of diaryl ketones catalyzed by a carbonyl reductase from Sporobolomyces salmonicolor and its mutant enzymes. Adv Synth Catal 351:583–588. https://doi.org/10.1002/adsc.200900045
Liang J, Lalonde J, Borup B, Mitchell V, Mundorff E, Trinh N, Kochrekar DA, Cherat RN, Ganesh Pai G (2010a) Development of a biocatalytic process as an alternative to the (−)-DIP-Cl-mediated asymmetric reduction of a key intermediate of montelukast. Org Process Res Dev 14:193–198. https://doi.org/10.1021/op900272d
Liang J, Mundorff E, Voladri R, Jenne S, Gilson L, Conway A, Krebber A, Wong J, Huisman G, Truesdell S, Lalonde J (2010b) Highly enantioselective reduction of a small heterocyclic ketone : biocatalytic reduction of tetrahydrothiophene-3-one to the corresponding ( R )-alcohol. Org Process Res Dev 14:188–192. https://doi.org/10.1021/op9002714
Liang J, Jenne SJ, Mundorff E, Ching C, Gruber JM, Krebber A, Huisman GW (2013) Ketoreductase polypeptides for the reduction of acetophenones. U.S. Patent Application 2017/0067032 A1
Lingen B, Grötzinger J, Kolter D, Kula MR, Pohl M (2002) Improving the carboligase activity of benzoylformate decarboxylase from Pseudomonas putida by a combination of directed evolution and site-directed mutagenesis. Protein Eng 15:585–593. https://doi.org/10.1093/protein/15.7.585
Lingen B, Kolter-Jung D, Dünkelmann P, Feldmann R, Grötzinger J, Pohl M, Müller M (2003) Alteration of the substrate specificity of benzoylformate decarboxylase from Pseudomonas putida by directed evolution. ChemBioChem 4:721–726. https://doi.org/10.1002/cbic.200200475
Liu YC, Guo C, Liu Y, Wang HB, Wu ZL (2017) Enzymatic cascades for the stereo-complementary epimerisation of in situ generated epoxy alcohols. Org Biomol Chem 15:2562–2568. https://doi.org/10.1039/c7ob00015d
Liu JS, Kuan YC, Tsou Y, Lin TY, Hsu WH, Te Yang M, Lin JY, Wang WC (2018) Structure-guided design of Serratia marcescens short-chain dehydrogenase/reductase for stereoselective synthesis of ( R )-phenylephrine. Sci Rep 8:1–11. https://doi.org/10.1038/s41598-018-19235-y
Liu B, Qu G, Li JK, Fan W, Ma JA, Xu Y, Nie Y, Sun Z (2019) Conformational dynamics-guided loop engineering of an alcohol dehydrogenase: capture, turnover and enantioselective transformation of difficult-to-reduce ketones. Adv Synth Catal 3182-3190. https://doi.org/10.1002/adsc.201900249
López-Gallego F, Yate L (2015) Selective biomineralization of Co 3 (PO 4 ) 2 -sponges triggered by His-tagged proteins: efficient heterogeneous biocatalysts for redox processes. Chem Commun 51:8753–8756. https://doi.org/10.1039/c5cc00318k
Ma SK, Gruber J, Davis C, Newman L, Gray D, Wang A, Grate J, Huisman GW, Sheldon RA (2010) A green-by-design biocatalytic process for atorvastatin intermediate. Green Chem 12:81–86. https://doi.org/10.1039/b919115c
Man H, Kędziora K, Kulig J, Frank A, Lavandera I, Gotor-Fernández V, Rother D, Hart S, Turkenburg JP, Grogan G (2014) Structures of alcohol dehydrogenases from Ralstonia and Sphingobium spp. Reveal the molecular basis for their recognition of “bulky-bulky” ketones. Top Catal 57:356–365. https://doi.org/10.1007/s11244-013-0191-2
Maria-Solano MA, Romero-Rivera A, Osuna S (2017) Exploring the reversal of enantioselectivity on a zinc-dependent alcohol dehydrogenase. Org Biomol Chem 15:4122–4129. https://doi.org/10.1039/c7ob00482f
Matsuda T, Nakajima Y, Nakamura K (2002) Asymmetric reduction of simple aliphatic ketones with dried cells of Geotrichum candidum . Tetrahedron Asymmetry 13:971–974. https://doi.org/10.1016/S0957-4166(02)00226-4
Matsuda T, Yamanaka R, Nakamura K (2009) Recent progress in biocatalysis for asymmetric oxidation and reduction. Tetrahedron Asymmetry 20:513–557. https://doi.org/10.1016/j.tetasy.2008.12.035
Modukuru NK, Sukumaran J, Collier SJ, Chan AS, Gohel A, Huisman GW, Keledjian R, Narayanaswamy K, Novick SJ, Palanivel SM, Smith D, Wei Z, Wong B, Yeo WL, Entwistle DA (2014) Development of a practical, biocatalytic reduction for the manufacture of ( S )-licarbazepine using an evolved ketoreductase. Org Process Res Dev 18:810–815. https://doi.org/10.1021/op4003483
Montgomery SL, Mangas-Sanchez J, Thompson MP, Aleku GA, Dominguez B, Turner NJ (2017) Direct alkylation of amines with primary and secondary alcohols through biocatalytic hydrogen borrowing. Angew Chemie Int Ed 56:10491–10494. https://doi.org/10.1002/anie.201705848
Musa MM, Phillips RS (2011) Recent advances in alcohol dehydrogenase-catalyzed asymmetric production of hydrophobic alcohols. Catal Sci Technol 1:1311. https://doi.org/10.1039/c1cy00160d
Musa MM, Ziegelmann-Fjeld KI, Vieille C, Zeikus JG, Phillips RS (2007) Xerogel-encapsulated W110A secondary alcohol dehydrogenase from Thermoanaerobacter ethanolicus performs asymmetric reduction of hydrophobic ketones in organic solvents. Angew Chemie Int Ed 46:3091–3094. https://doi.org/10.1002/anie.200604615
Musa MM, Patel JM, Nealon CM, Kim CS, Phillips RS, Karume I (2015) Thermoanaerobacter ethanolicus secondary alcohol dehydrogenase mutants with improved racemization activity. J Mol Catal B Enzym 115:155–159. https://doi.org/10.1016/j.molcatb.2015.02.012
Musa MM, Bsharat O, Karume I, Vieille C, Takahashi M, Hamdan SM (2018) Expanding the substrate specificity of Thermoanaerobacter pseudoethanolicus secondary alcohol dehydrogenase by a dual site mutation. Eur J Org Chem 2018:798–805. https://doi.org/10.1002/ejoc.201701351
Mutti FG, Knaus T, Scrutton NS, Breuer M, Turner NJ (2015) Conversion of alcohols to enantiopure amines through dual-enzyme hydrogen-borrowing cascades. Science 349:1525–1529. https://doi.org/10.1126/science.aac9283
Nealon CM, Musa MM, Patel JM, Phillips RS (2015) Controlling substrate specificity and stereospecificity of alcohol dehydrogenases. ACS Catal 5:2100–2114. https://doi.org/10.1021/cs501457v
Nealon CM, Welsh TP, Kim CS, Phillips RS (2016) I86A/C295A mutant secondary alcohol dehydrogenase from Thermoanaerobacter ethanolicus has broadened substrate specificity for aryl ketones. Arch Biochem Biophys 606:151–156. https://doi.org/10.1016/j.abb.2016.08.002
Nie Y, Wang S, Xu Y, Luo S, Zhao Y-L, Xiao R, Montelione GT, Hunt JF, Szyperski T (2018) Enzyme engineering based on X-ray structures and kinetic profiling of substrate libraries: alcohol dehydrogenases for stereospecific synthesis of a broad range of chiral alcohols. ACS Catal 8:5145–5152. https://doi.org/10.1021/acscatal.8b00364
Noey EL, Tibrewal N, Jiménez-Osés G, Osuna S, Park J, Bond CM, Cascio D, Liang J, Zhang X, Huisman GW, Tang Y, Houk KN (2015) Origins of stereoselectivity in evolved ketoreductases. Proc Natl Acad Sci:E7065–E7072. https://doi.org/10.1073/pnas.1507910112
Peschke T, Skoupi M, Burgahn T, Gallus S, Ahmed I, Rabe KS, Niemeyer CM (2017) Self-immobilizing fusion enzymes for compartmentalized biocatalysis. ACS Catal 7:7866–7872. https://doi.org/10.1021/acscatal.7b02230
Popłoński J, Reiter T, Kroutil W (2018) Biocatalytic racemization employing Te SADH: substrate scope and organic solvent compatibility for dynamic kinetic resolution. ChemCatChem 10:763–768. https://doi.org/10.1002/cctc.201701395
Prelog V (1964) Specification of the stereospecificity of some oxido-reductases by diamond lattice sections. Pure Appl Chem 9:119–130. https://doi.org/10.1351/pac196409010119
Rosenthal K, Lütz S (2018) Recent developments and challenges of biocatalytic processes in the pharmaceutical industry. Curr Opin Green Sustain Chem 11:58–64. https://doi.org/10.1016/j.cogsc.2018.03.015
Sheldon RA, Brady D (2019) Broadening the scope of biocatalysis in sustainable organic synthesis. ChemSusChem 12:2859–2881. https://doi.org/10.1002/cssc.201900351
Shieh WR, Gopalan AS, Sih CJ (1985) Stereochemical control of yeast reductions. 5. Characterization of the oxidoreductases involved in the reduction of β-keto esters. J Am Chem Soc 107:2993–2994. https://doi.org/10.1021/ja00296a038
Shimoda K, Kubota N, Hamada H, Yamane S, Hirata T (2004) Asymmetric transformation of enones with Synechococcus sp. PCC 7942. Bull Chem Soc Jpn 77:2269–2272. https://doi.org/10.1246/bcsj.77.2269
Sun Z, Lonsdale R, Ilie A, Li G, Zhou J, Reetz MT (2016) Catalytic asymmetric reduction of difficult-to-reduce ketones: triple-code saturation mutagenesis of an alcohol dehydrogenase. ACS Catal 6:1598–1605. https://doi.org/10.1021/acscatal.5b02752
Thompson MP, Turner NJ (2017) Two-enzyme hydrogen-borrowing amination of alcohols enabled by a cofactor-switched alcohol dehydrogenase. ChemCatChem 9:3833–3836. https://doi.org/10.1002/cctc.201701092
Truppo MD, Pollard D, Devine P (2007) Enzyme-catalyzed enantioselective diaryl ketone reductions. Org Lett 9:335–338. https://doi.org/10.1021/ol0627909
Ueno T (2013) Porous protein crystals as reaction vessels. Chem A Eur J 19:9096–9102. https://doi.org/10.1002/chem.201300250
Velonia K, Tsigos I, Bouriotis V, Smonou I (1999) Stereospecificity of hydrogen transfer by the NAD + -linked alcohol dehydrogenase from the Antarctic psychrophile Moraxella sp. TAE123. Bioorganic Med Chem Lett 9:65–68. https://doi.org/10.1016/S0960-894X(98)00678-7
Wang S, Nie Y, Xu Y, Zhang R, Ko T-P, Huang C-H, Chan H-C, Guo R-T, Xiao R (2014) Unconserved substrate-binding sites direct the stereoselectivity of medium-chain alcohol dehydrogenase. Chem Commun 50:7770–7772. https://doi.org/10.1039/c4cc01752h
Wu K, Zheng K, Xiong L, Yang Z, Jiang Z, Meng X, Shao L (2019) Efficient synthesis of an antiviral drug intermediate using an enhanced short-chain dehydrogenase in an aqueous-organic solvent system. Appl Microbiol Biotechnol 103:4417–4427
Zhang AL, Da Yu Z, Yang LW, Yang NF (2015a) Synthesis of several polyethers derived from BINOL and their application in the asymmetric borane reduction of prochiral ketones. Tetrahedron Asymmetry 26:173–179. https://doi.org/10.1016/j.tetasy.2014.12.012
Zhang D, Chen X, Chi J, Feng J, Wu Q, Zhu D (2015b) Semi-rational engineering a carbonyl reductase for the enantioselective reduction of β-amino ketones. ACS Catal 5:2452–2457. https://doi.org/10.1021/acscatal.5b00226
Zhang R, Xu Y, Xiao R (2015c) Redesigning alcohol dehydrogenases/reductases for more efficient biosynthesis of enantiopure isomers. Biotechnol Adv 33:1671–1684. https://doi.org/10.1016/j.biotechadv.2015.08.002
Zheng Y, Yin H, Yu D, Chen X, Tang X (2017) Recent advances in biotechnological applications of alcohol dehydrogenases. Appl Microbiol Biotechnol 101:987–1001. https://doi.org/10.1007/s00253-016-8083-6
Zhou B, Gopalan AS, Van Middlesworth F, Shieh WR, Sih CJ (1983) Stereochemical control of yeast reductions. 1. Asymmetric synthesis of L-carnitine. J Am Chem Soc 105:5925–5926. https://doi.org/10.1021/ja00356a041
Zhou J, Wang Y, Xu G, Wu L, Han R, Schwaneberg U, Rao Y, Zhao YL, Zhou J, Ni Y (2018) Structural insight into enantioselective inversion of an alcohol dehydrogenase reveals a “polar gate” in stereorecognition of diaryl ketones. J Am Chem Soc 140:12645–12654. https://doi.org/10.1021/jacs.8b08640
Zhu D, Hua L (2006) Enantioselective enzymatic reductions of sterically bulky aryl alkyl ketones catalyzed by a NADPH-dependent carbonyl reductase. J Org Chem 71:9484–9486. https://doi.org/10.1021/jo061571y
Zhu D, Yang Y, Buynak JD, Hua L (2006) Stereoselective ketone reduction by a carbonyl reductase from Sporobolomyces salmonicolor . Substrate specificity, enantioselectivity and enzyme-substrate docking studies. Org Biomol Chem 4:2690–2695. https://doi.org/10.1039/b606001c
Zong C, Zhang X, Yang F, Zhou Y, Chen N, Yang Z, Ding G, Yu F, Tang Y (2019) Biotransformation of a crizotinib intermediate using a mutant alcohol dehydrogenase of Lactobacillus kefir coupled with glucose dehydrogenase. Prep Biochem Biotechnol 49:578–583. https://doi.org/10.1080/10826068.2019.1591987
Download references
This study was funded by Japan Society for the Promotion of Science under grant number JP16K05864 to Tomoko Matsuda, and Platform Project for Supporting Drug Discovery and Life Science Research (Basis for Supporting Innovative Drug Discovery and Life Science Research (BINDS)) from AMED under grant numbers 19am0101108j0003 to Daron M. Standley and 19am0101071 to Toshiya Senda.
Author information
Authors and affiliations.
Department of Life Science and Technology, School of Life Science and Technology, Tokyo Institute of Technology, 4259 Nagatsuta-cho Midori-ku, Yokohama, 226-8501, Japan
Afifa Ayu Koesoema & Tomoko Matsuda
Department of Genome Informatics, Genome Information Research Center, Research Institute of Microbial Diseases, Osaka University, 3-1 Yamadaoka, Suita, Osaka, 565-0871, Japan
Daron M. Standley
Structural Biology Research Center, Institute of Materials Structure Science, High Energy Accelerator Research Organization (KEK), 1-1 Oho Tsukuba, Ibaraki, 305-0801, Japan
Toshiya Senda
Department of Materials Structure Science, School of High Energy Accelerator Science, SOKENDAI (The Graduate University for Advanced Studies), 1-1 Oho, Tsukuba, Ibaraki, 305-0801, Japan
You can also search for this author in PubMed Google Scholar
Corresponding author
Correspondence to Tomoko Matsuda .
Ethics declarations
Conflict of interest.
The authors declare that they have no conflict of interest.
Ethical approval
This article does not contain any studies with human participants or animals performed by any of the authors.
Additional information
Publisher’s note.
Springer Nature remains neutral with regard to jurisdictional claims in published maps and institutional affiliations.
Rights and permissions
Reprints and permissions
About this article
Koesoema, A.A., Standley, D.M., Senda, T. et al. Impact and relevance of alcohol dehydrogenase enantioselectivities on biotechnological applications. Appl Microbiol Biotechnol 104 , 2897–2909 (2020). https://doi.org/10.1007/s00253-020-10440-2
Download citation
Received : 25 November 2019
Revised : 30 January 2020
Accepted : 05 February 2020
Published : 15 February 2020
Issue Date : April 2020
DOI : https://doi.org/10.1007/s00253-020-10440-2
Share this article
Anyone you share the following link with will be able to read this content:
Sorry, a shareable link is not currently available for this article.
Provided by the Springer Nature SharedIt content-sharing initiative
- Alcohol dehydrogenase
- Enantioselectivity
- Asymmetric reduction
- Enzyme engineering
- Pharmaceutical intermediate
- Find a journal
- Publish with us
- Track your research
- DOI: 10.1097/00008571-199210000-00001
- Corpus ID: 29526692
Regulation of human alcohol dehydrogenase genes.
- H. Edenberg , C. Brown
- Published in Pharmacogenetics (London) 1 October 1992
32 Citations
Regulation of the mammalian alcohol dehydrogenase genes., regulation of the human alcohol dehydrogenases genes adh1, adh2 and adh3: differences in cis-acting sequences at ctf/nf-i sites., regulation of the seven human alcohol dehydrogenase genes., regulation of human alcohol dehydrogenase gene adh7: importance of an ap-1 site., 4.06 – alcohol dehydrogenases, gata-2 and hnf-3beta regulate the human alcohol dehydrogenase 1a (adh1a) gene., tissue-specific differences in the expression of the human adh2 alcohol dehydrogenase gene and in binding of factors to cis-acting elements in its promoter., gene expression in a young multigene family: tissue-specific differences in the expression of the human alcohol dehydrogenase genes adh1, adh2, and adh3., distant hnf1 site as a master control for the human class i alcohol dehydrogenase gene expression*, a retroviral repetitive element confers tissue-specificity to the human alcohol dehydrogenase 1c (adh1c) gene., related papers.
Showing 1 through 3 of 0 Related Papers

An official website of the United States government
The .gov means it’s official. Federal government websites often end in .gov or .mil. Before sharing sensitive information, make sure you’re on a federal government site.
The site is secure. The https:// ensures that you are connecting to the official website and that any information you provide is encrypted and transmitted securely.
- Publications
- Account settings
Preview improvements coming to the PMC website in October 2024. Learn More or Try it out now .
- Advanced Search
- Journal List
- Curr Res Food Sci
- v.1; 2019 Nov
Influence of food commodities on hangover based on alcohol dehydrogenase and aldehyde dehydrogenase activities
Associated data.
Alcohol consumption often leads to hangover, a condition characterized by several symptoms, characteristically headache, nausea, fatigue and drowsiness. Hangover may be alleviated by altering the rate of alcohol metabolism and facilitating elimination of acetaldehyde by affecting the activity of alcohol dehydrogenase (ADH) and/or aldehyde dehydrogenase (ALDH) enzymes. In the present study, several food commodities like fruits, vegetables, cereals, pulses, dairy products, spices and other miscellaneous products (ascorbic acid, cocoa sample, tea, coffee, egg yolk and date samples) were investigated for their effect on the in vitro activities of the enzymes and their antioxidant properties. Of the many screened food commodities, few showed an increase in the activity of either one or both the enzymes, ADH and ALDH. Studies showed no correlation between ADH and ALDH enzyme activities and antioxidant property of the selected food commodities for anti-hangover effect. Further, an anti-hangover (AHO) product was developed using pear (65%), sweet lime (25%) and coconut water (10%) and, validated for in vitro ADH and ALDH enzyme activities. AHO product was found to enhance ADH and ALDH activities by 23.31% and 70.02%, respectively.
Graphical abstract

- • Screening of food influencing alcohol dehydrogenase (ADH) and aldehyde dehydrogenase (ALDH) activities.
- • Foods commodities enhancing ADH and/or ALDH activities can be used in AHO formulation.
- • Pear (65%), sweetlime (25%) and coconut water(10%) used in anti-hangover (AHO) formulation.
- • AHO product had increased ADH (23.3%) and ALDH (70.02%) activities.
1. Introduction
Hangover or veisalgia is the term that refers to the psychological and physiological effects following alcohol consumption. It is characterized by an unpleasant and uneasy feeling that includes, but is not limited to, headache, fatigue, drowsiness, nausea, and in some cases, vomiting ( Swift and Davidson, 1998 ). The symptoms set in when the concentration of ethanol in the blood reaches zero, implying that it is the metabolism or the metabolite that leads to this effect. The effects of hangover are attributed to several causes, the major ones being due to i) the direct effects of alcohol, ii) metabolism of alcohol, iii) other non-alcohol factors such as the presence of congeners (biologically active molecules present in alcohol, apart from ethanol, for example, methanol or ethyl formate), and iv) genetic makeup. Ethanol consumption affects specific organs or systems such as the brain, gastrointestinal tract, liver and immune system ( Swift and Davidson, 1998 ) manifesting as symptoms that are characteristic of a typical hangover.
During metabolism of ethanol, it gets broken down either through the oxidative or non-oxidative pathway ( Zakhari, 2006 ). During oxidative metabolism of alcohol to acetate, ethanol is converted to acetaldehyde by the hepatic alcohol dehydrogenase (ADH). Nicotinamide adenine dinucleotide (NAD + ) serves as the intermediate electron carrier for ADH, and it gets reduced to nicotinamide adenine dinucleotide-reduced (NADH). The increase in the NADH causes oxidative stress in the liver which also contributes to the symptoms exhibited during the phase of hangover. Acetaldehyde built-up has been attributed as major causative factor of hangover ( Zakhari, 2006 ). The alleles of the ADH and ALDH form the genetic basis for the manifestation of hangover. The genetic effect is predominantly seen among the racial populations ( Cederbaum, 2012 , Quertemont, 2004 ). The isozymes of ADH and ALDH have different affinity for their substrates, and thus the genetic makeup plays a major role in determining the rate at which an individual will eliminate ethanol from the system.
Hangover poses a considerable threat at the individual level, where along with the occurrence of unpleasantness there is also a risk of health issues that might impact everyday functioning. It also affects the economy due to a decrease in the productive work caused by sleep deprivation, loss of attentiveness and alertness ( Cederbaum, 2012 ). Several natural compounds like 6-gingerol ( Takahashi et al., 2010 ), dehydroevodiamine ( Wang et al., 2016a ), ginsenosides ( Kim et al., 2011 ), linolenic acids ( Lee et al., 2014a ) among many others, have been recommended as a cure for hangover. Researchers have investigated and identified natural components (such as polyphenols) from various food sources such as fruits, mushroom, and herbs to alleviate hangover symptoms ( Zhao et al., 2017 , Zhang et al., 2016 , Kim, 2016 , Bajpai et al., 2016 ). These natural food sources are reported to function effectively by exhibiting positive effects on the hepatic enzymes, i.e. by either enhancing the activity of ALDH and/or ADH and thereby assisting in aldehyde and alcohol clearance from the system, respectively. A herbal mixture “DTS 20” containing Viscum album L. (40%), Lycium chinense L. (30%), Inonotus obliquus (20%), and Acanthopanax senticosus H. (10%) has also been studied and found to reduce the oxidative stress and plasma alcohol concentrations ( Hong, 2015 ).
While pharmaceutics have also gained momentum in the search to find a cure for hangover, the identification of natural compounds will target the implementation of dietary modifications to ensure better results. This research work aims at studying the influence of food commodities on ADH and ALDH enzyme activities and their kinetic properties that could further help to identify specific components to formulate an effective natural AHO product. Few studies have observed that sugars like glucose and fructose do alleviate hangover by facilitating alcohol detoxification. However, these do not result through the action of the hepatic enzymes (ADH and ALDH) and are a separate entity for research scope.
2. Materials and methods
2.1. sample procurement.
The fruits, vegetables, cereals, pulses, dairy products, spices and other miscellaneous products were purchased from the local market of Mumbai city, India. The food commodities used are listed in Table 1 .
Table 1
List of food commodities screened for AHO formulation.
Fruits |
Carambola/starfruit ( ), orange ( L.), gooseberry ), black grapes ), green grapes ), pitahaya/dragonfruit , pear , Apple ), sweet lime ), mango ), kiwifruit ), pineapple ), papaya ), lemon ( L.), watermelon ( ), pomegranate ( |
Vegetables |
Garlic ( ), cucumber ( ), coriander ( ), fenugreek leaves ( ), spinach ( ), bitter gourd/bitter melon ( ), carrot ( ), Onion ( ), tomato ( ) |
Cereals |
Sorghum ( sp. bicolor), oats ( ), wheat ( ), maize ( ), peanuts ( ) millet ( ) |
Spices |
Black pepper, cassia, cinnamon, cloves, ginger, cumin (jeera), mace, nutmeg, turmeric |
Milk Products |
Butter-milk, cheese, milk, a commercial probiotic drink |
Miscellaneous |
Ascorbic acid, black tea, cocoa, coconut water, coffee, dates, egg white egg yolk, fenugreek seeds, green tea |
2.2. Chemicals and reagents
2,2 Diphenyl-1-picrylhydrazyl (DPPH), ADH and ALDH were procured from Sigma-Aldrich, Bangalore, India. Bovine serum albumin (BSA), β-mercaptoethanol, potassium chloride, gallic acid, β-nicotinamide adenine dinucleotide (NAD + ) and NADH were purchased from HiMedia Laboratories, Mumbai, India. Ethanol, methanol, and acetaldehyde were purchased from Merck Ltd, Mumbai, India.
2.3. Preparation of samples
2.3.1. fruits, vegetables, spices and cereals.
The fruits and vegetables were washed, cleaned and dried. The fruit/vegetable juices were extracted by grinding/pulping them in a mixer/grinder and filtered through Whatman filter No. 2 as samples for further experiments. Spices were ground to size ≤0.25 mm and extracted in a 1% (w/v) solution of 60% methanol using magnetic stirrer for 1 h. The extracts were filtered through Whatman No. 1 filter paper, dried and resuspended in deionized water at a concentration of 100 mg/mL ( Subbaraj et al., 2016 ). Cereals and pulses samples were prepared by following the method as per Meneses et al. (2013) with some modifications. Cereals and pulses were milled to a particle size of ≤0.25 mm and stored in refrigerator prior to sample preparation. The sample was prepared by taking 10% w/v solution of the cereals or pulses in 60% methanol and subjected to magnetic agitation for 30 min at 60 °C. The obtained extracts were then filtered, dried and resuspended in a similar fashion as the spice samples. All the fruits, vegetables, spices and cereal samples were stored at −20 °C and used for various assays within two days. Fruits and vegetables were used directly as juices since the constituents are generally homogenized and most of the bioactive compounds are available for the reaction. However, for spices the components need to be extracted using suitable solvents so as to make them available for the reaction. Similarly, dairy products were diluted in order to prevent interference from particulate matter. In the current study, extraction of the components that would also exhibit antioxidant properties were aimed at so as to test for their effect on the hepatic enzymes and also to check for their antioxidant activity.
2.3.2. Dairy products
Extracts of milk and cheddar cheese were obtained by following the protocol as per Pritchard ( Pritchard et al., 2010 ) with modifications. Briefly, Cheddar cheese was homogenized in a ratio of 1:3 w/v with deionized water and was subjected to magnetic agitation at 40 °C for 1 h. The contents were then centrifuged at 4250 g at 4 °C for 30 min. The supernatant was filtered through Whatman filter paper (No. 42) followed by membrane filtration (pore size 0.22 μm). Diluted samples (1:1 v/v) of buttermilk and commercial probiotic product were used after filtration through a membrane of pore size 0.45 μm. Samples were stored at −20 °C in deep freezer and used for assays within two days.
2.3.3. Miscellaneous
Ascorbic acid was used at the concentration of 2 mg/mL for various assays. Cocoa sample (1:100) was prepared in 80% methanol solution with stirring (1 h) followed by solvent evaporation and resuspension in deionized water. Tea and coffee (0.1 g) were brewed with 8 mL boiling deionized water for 2 min ( Floegel et al., 2011 ) and filtered through Whatman filter paper and membrane filtration (0.45 μm membrane). For egg yolk, sample preparation was done as per the method reported by Su et al. (2015) . Egg yolk was separated from egg white and beaten with addition of 95% ethanol in 1:10 ratio. The contents were then mixed in a magnetic stirrer at 65 °C for 1 h and filtered, the process was repeated twice. The ethanol fractions were pooled and ethanol was evaporated. Deionized water was added to the residue and the sample was assayed within two days. Date sample was prepared by measuring 1 g of seedless dates and mixing it in 10 mL of deionized water for 48 h under stirring conditions. The contents were then mixed in a mixer and centrifuged at 4000 rpm (Remi Centrifuge, REMI- CRP-30, Mumbai, India) at 4 °C for 20 min. The supernatant was collected further and used for assays ( Zangiabadi et al., 2011 ). All the processed samples were stored at −20 °C until the assays were performed.
2.4. Enzymatic assays for screening of food commodities
2.4.1. determination of adh activity.
The ADH activity was determined according to the protocol reported by Lee et al. (2012) with slight modifications. The following solutions were prepared: 1 M Tris HCl (adjusted to pH 7.5 with 1M HCl at 25 °C), freshly prepared 3 mM β NAD + , 12.5 mM ethanol solution, 0.5% Triton X 100, 100 mM Tris HCl buffer with 0.02% (w/v) BSA at pH 8.0, 20 U/mL ADH (prepared in buffer) and 1 mL of the test sample extract. The in vitro activity of ADH was measured as NADH formed, determined spectrophotometrically at 340 nm using an ELISA plate reader (BioTek ELx808, Mumbai, India). The reaction was started by addition of ethanol to a pre-incubated reaction mixture containing the remaining solutions and made-up to a final volume of 300 μL. The reaction was carried out at 25 °C and pH of 7.5. The control reaction was carried out in the absence of any test sample. A blank reaction (in the absence of the substrate) was also measured and values were corrected with respect to the baseline in order to eliminate the effect of any artefacts.
2.4.2. Determination of ALDH activity
The ALDH activity was also determined according to the protocol mentioned by Lee et al. (2012) with slight modifications. The following solutions were prepared: 1 M Tris HCl (adjusted to pH 8.0 with 1M HCl at 25 °C), freshly prepared 3 mM β NAD+, 100 mM acetaldehyde solution, 3 M KCl, freshly prepared 1 M β-mercaptoethanol solution, 100 mM Tris HCl buffer with 0.02% (w/v) BSA at pH 8.0, 0.1 U/mL ALDH (prepared in buffer) and 1 mL of the test sample extract. The in vitro activity of ALDH was measured as NADH formed, determined spectrophotometrically at 340 nm using an ELISA plate reader (BioTek ELx808, Mumbai, India). The reaction was started by addition of acetaldehyde to a reaction mixture of the other solutions and made-up to a final volume of 300 μL. The reaction was carried out at 25 °C and pH of 8.0. The control reaction was carried out in the absence of any test sample. A blank reaction (in the absence of the substrate) was also measured and values were corrected with respect to the baseline in order to eliminate the effect of any artefacts. The percent activity of ADH and ALDH was calculated by the formula.
2.5. Antioxidant potential
Antioxidant property of a substance was assayed by the 2,2-Diphenyl-1-picrylhydrazyl radical (DPPH•) scavenging assay ( Ramadan et al., 2008 ) using a spectrophotometer at 517 nm. Gallic acid was used as a standard. The percentage inhibition was calculated using the formula given.
The antioxidant capacities for the samples were reported as gallic acid equivalent (GAE).
2.6. Kinetic studies
The activation kinetics of the samples that positively affected the activity of ADH and ALDH were studied and the apparent K m value was calculated.
2.7. Correlation between antioxidant property and enzyme activity
The correlation between ADH activity, ALDH activity and antioxidant property of the samples were determined by plotting a scatter plot and calculating the R 2 values.
3. Theory/calculation
An AHO product was developed based on the effect of the substances on ADH activity, ALDH activity and organoleptic acceptability. D-optimal mixture design was applied to find the optimal response for any mixture of the selected substances, and to obtain the influence on the response of the combination of substances. Percentage of components; pear (A), sweet lime (B) and coconut water (C) were the three independent parameters. The dependent variables selected as the response was overall average acceptability score given by the sensory panel comprising of 15 panellists (8 females and 7 males in the age group of 22–28 years). The scoring was based on 9-point hedonic scale as follows: 9-like extremely; 8-like very much; 7-like moderately; 6-like slightly; 5-neither like nor dislike; 4-dislike slightly; 3-dislike moderately; 2-dislike very much; 1-dislike extremely.
The statistical software package Design Expert 7.0 (Stat-Ease Inc., Minneapolis, MN, USA), was used for the experimental design and data analysis. All the analyses were carried out in triplicates and the data generated has been reported in terms of mean ± standard deviation (SD). Data were assessed by ANOVA (Analysis of Variance) to determine the significance of difference observed in the samples. The significance for the mean difference for all the data generated and reported was compared using Duncan's multiple comparison test (p < 0.05).
4. Results and discussion
Food samples necessarily enhancing the ADH and/or ALDH are suitable for AHO formulation.
4.1. Effect of fruits and vegetables on alcohol dehydrogenase (ADH) activity and aldehyde dehydrogenase (ALDH) activity
Fruits and vegetables that decrease the ALDH activity are not suitable as an AHO substance. The effect of fruits and vegetables on the percent difference in activities of ADH and ALDH is shown in Table 2 . Grapes, dragon fruit and gooseberry did not significantly affect the activity of ADH while starfruit and orange significantly decreased the activity of ADH. In the present work, the high percentage activity difference between the watermelon sample reaction and control reaction indicates a positive effect of watermelon in enhancing the activity of ADH by 67.22%. Grapes did not alter the ALDH activity, while gooseberry decreased it. Studies performed by Zhang et al. (2016) also confirmed the ability of starfruit to decrease the ADH activity, though not very significantly. A commercially available AHO product “PartySmart” containing grapes and gooseberry as ingredients has been reported to have a positive effect on both ADH and ALDH ( Pordié, 2015 ). Wang et al. (2016b) observed fresh orange to decrease the ADH activity. Similarly, mango pulp has also been reported to enhance the ADH activity in rats by 129.76% ( Kim et al., 2011 ). However, Zhang et al. (2016) did not find any significant difference in the ADH activity by watermelon and lemon, Actinidia chinensis (closely related to kiwi fruit). While the difference in the reported results from that of the present study can be attributed to variations in the samples used, it could also be that in vitro and in vivo studies have yielded different results.
Table 2
Effect of fruits and vegetables on ADH and ALDH activities.
Food commodities | ADH activity (%) | ALDH activity (%) | |
---|---|---|---|
Fruits | |||
(apple) | 28.06 ± 0.95 | −76.15 ± 1.46 | |
(mango) | 31.74 ± 1.65 | −61.97 ± 1.08 | |
(watermelon) | 67.22 ± 2.81 | −57.39 ± 1.38 | |
(papaya) | 46.50 ± 3.73 | −50.55 ± 2.24 | |
(black grapes) | −1.36 ± 0.26 | −33.44 ± 2.03 | |
(gooseberry) | −2.73 ± 0.22 | −31.42 ± 2.20 | |
(pineapple) | 39.28 ± 2.98 | −30.35 ± 3.06 | |
(pomegranate) | 67.97 ± 6.95 | −22.48 ± 4.75 | |
(kiwi fruit) | 38.80 ± 6.29 | −20.09 ± 4.48 | |
(lemon) | 47.39 ± 8.76 | −19.59 ± 4.12 | |
(dragon fruit) | 4.57 ± 0.38 | −3.06 ± 1.44 | |
(green grapes) | 3.02 ± 0.16 | −0.20 ± 0.02 | |
(orange) | −8.54 ± 1.91 | 15.48 ± 5.16 | |
(starfruit) | −13.39 ± 1.18 | 22.76 ± 9.26 | |
(sweet lime) | 29.64 ± 7.36 | 33.47 ± 1.38 | |
sp. (pear) | 22.11 ± 3.21 | 90.98 ± 1.96 | |
Vegetables | |||
(garlic) | −44.11 ± 4.32 | −42.23 ± 2.72 | |
(cucumber) | −18.37 ± 2.26 | 87.25 ± 1.55 | |
(coriander) | −12.36 ± 0.70 | −58.38 ± 12.20 | |
(fenugreek leaves) | 2.62 ± 0.58 | −6.43 ± 1.53 | |
(spinach) | 6.10 ± 0.72f | −38.94 ± 2.27ef | |
(bitter gourd/bitter melon) | 14.42 ± 1.87 | −1.59 ± 0.16 | |
(carrot) | 29.33 ± 2.45 | −10.76 ± 3.55 | |
(Onion) | 48.25 ± 3.51 | 2.24 ± 0.90 | |
(tomato) | 57.25 ± 2.79 | 41.19 ± 6.37° | |
Control | 0.00 | 0.00 ± 0.00 | |
Commercial anti-hangover product | −11.64 ± 1.49 | −82.50 ± 0.57 |
Values are mean ± SD of three determinants.
Different alphabet in superscript represent that the values are significantly different (p < 0.05).
Pear showed the highest positive effect on ALDH activity at 90.98%. Orange, starfruit, and sweet lime also enhanced the ALDH activity significantly by 15.48%, 22.76% and 33.47%, respectively. The potential use of pear to alleviate hangover has been reported in previous studies based on their effect on the activity of the enzyme ( Lee et al., 2012 ). Enhanced ALDH activity of pear facilitates in faster elimination of acetaldehyde and thus it serves as a suitable AHO. Zhang et al. (2016) reported that orange and starfruit showed a decrease in the activity of ALDH (by 11.81% and 61.95%, respectively) while dragon fruit did not significantly affect the ALDH activity. Wang et al. (2016b) also reported fresh orange juice to decrease the ALDH activity. This variation could be due to the differences in the sample nature and sample preparation methods. The presence of polyphenols in the fruits has been reported to enhance the activity of ADH and ALDH ( Lee et al., 2012 ). However, the exact mechanism is yet to be elucidated.
Among the vegetable samples analysed, bitter gourd and carrot showed increase in ADH activities and decrease in ALDH activities. Tomato and cucumber samples showed an increase in the activity of ALDH (41.19% and 87.25%). Investigators have reported that heat treated cucumber had the ability to enhance the activity of ALDH and ADH ( Bajpai et al., 2016 ). Contrary to this result, our work showed a significant decrease of ADH activity by cucumber. This could be due to differences in the variety as well sample preparation methods.
4.2. Effect of dairy products, cereals, pulses, spices and other miscellaneous food products on ADH and ALDH activity
The effect of dairy products, pulses, spices, and other miscellaneous food products on ADH and ALDH activities are shown in Table 3 . Dairy products like milk showed a marginal but significant decrease in the activity of ALDH, while the other samples such as buttermilk, cheese and a commercial prebiotic drink showed an increase in the activity of the ALDH. In addition to the ability to enhance the activity of the enzyme, this effect could be attributed to the presence of microorganisms in the samples that are able to produce ALDH and thereby enhance the rate of product formation. Lactobacillus sp. fermented cream cheese has been reported as an AHO product due to its ability to increase the rate of acetaldehyde metabolism ( Konkit et al., 2016 ). Moreover, the presence of several functional peptides in these fermented products may contribute to the modified effect on the enzymes.
Table 3
Effect of dairy products, cereals, pulses, spices and other miscellaneous food products on ADH and ALDH activity.
Food commodities | ADH activity (%) | ALDH activity (%) |
---|---|---|
Dairy products | ||
Milk | −10.75 ± 2.04 | −3.93 ± 0.55° |
Buttermilk | −4.01 ± 2.15 | 17.50 ± 0.97 |
A commercial probiotic drink | −4.04 ± 1.96 | 24.23 ± 3.14 |
Cheddar cheese | 97.31 ± 6.25 | 18.63 ± 2.34 |
Cereals and pulses | ||
(oats) | −24.83 ± 1.53 | −76.81 ± 1.15 |
(peanuts) | −15.06 ± 1.44 | −66.41 ± 3.78 |
(millet) | −1.74 ± 0.58 | −47.13 ± 3.50 |
sp. (sorghum) | −26.70 ± 4.24 | −32.16 ± 3.11 |
(maize) | −14.38 ± 2.01 | −0.51 ± 0.13 |
sp. (wheat) | −17.71 ± 1.13 | 0.32 ± 0.21 |
Spices | ||
Pepper | −67.41 ± 2.95 | −34.08 ± 1.67 |
Cloves | −67.15 ± 2.06 | −60.81 ± 5.58 |
Nutmeg | −46.21 ± 2.87 | −97.89 ± 7.81 |
Cumin | −23.56 ± 0.80 | −3.54 ± 2.23° |
Cinnamon | −21.38 ± 1.88 | −61.16 ± 2.02 |
Cassia | −18.13 ± 2.01 | −21.06 ± 4.61 |
Mace | 13.26 ± 1.67 | −82.48 ± 4.09 |
Turmeric | 10.73 ± 2.28 | −23.79 ± 1.28 |
Ginger | 20.04 ± 1.41 | −35.96 ± 1.44 |
Miscellaneous | ||
Vitamin C | −88.77 ± 0.65 | −88.97 ± 3.97 |
Coffee | −42.75 ± 3.42 | −53.44 ± 2.85 |
Egg yolk | −13.15 ± 1.41 | −38.93 ± 6.78 |
Coconut water | −0.23 ± 0.01 | 13.95 ± 1.36 |
Cocoa | 0.75 ± 0.31 | −29.04 ± 2.08 |
Black tea | 2.87 ± 0.20 | 5.37 ± 0.26 |
Dates | 13.55 ± 1.15 | −26.77 ± 2.26 |
Green tea | 11.56 ± 1.46 | 2.64 ± 0.36 |
Egg white | 25.50 ± 1.41° | −3.47 ± 1.20° |
Fenugreek seeds | 86.79 ± 2.04 | −62.40 ± 2.50 |
Control | 0.00 | 0.00 ± 0.00 |
Commercial anti-hangover product | −11.64 ± 1.49 | −82.50 ± 0.57 |
All the cereal samples decreased ADH and ALDH activities. While maize and wheat had no significant effect on the activity of ALDH, the other samples analysed, showed a decrease in the activity of ALDH. This suggests that it is not advisable to include cereals and pulses in the diet post consumption of alcohol until the hangover has subsided.
Among the spice samples, mace, turmeric and ginger increased ADH activity. However, a commercial AHO product with turmeric as its principal component showed a decrease in the activity of ADH. The product also contained Vitamin C as one of its ingredients. The activity of ALDH was not significantly altered by cumin. Other spice samples showed a significant decrease in the activity of ALDH. The commercial AHO product was found to decrease the ALDH activity. So far, research work on effect of spices on ADH and ALDH have not been elaborately carried out and hence further studies (especially in vivo ) need to be undertaken to establish these results.
Among the miscellaneous samples, fenugreek seeds, egg white, green tea, black tea, and dates enhanced the activity of ADH. Cocoa and coconut water did not significantly alter the activity of ADH. Ascorbic acid significantly decreased the activity of the ADH to a very large magnitude. Most of the commercial products are marketed based on their antioxidant property and these are often rich in minerals and also contain a mixture of components such as polyphenols, flavonoids and other bioactive components. In the current study, the AHO product tested did not show any positive effect on both the hepatic enzymes (ADH and ALDH). Thus, it is possible that there exists another mechanism of action for this product. A substantial decrease in the ADH and ALDH activities due to coffee indicates that consumption of coffee post alcohol consumption could slower the rate of ethanol elimination, and hence it should not be preferred during hangover. Egg white showed enhanced ADH activity, while egg yolk showed a decrease in the ADH activity. Black tea did not significantly affect the ADH activity (2.87%) while green tea showed a marginal increase in ADH activity (11.56%). These results for green tea and black tea are in agreement with Wang et al. (2016b) . It was interesting to note that both green and black tea did not significantly affect the activity of ALDH. The difference in the results exhibited by green and black tea may be probably due to the difference in their polyphenols and mineral contents.
Fenugreek seeds increased the activity of ADH significantly to 86.79%. However, when fenugreek leaves were tested for their effect, no significant difference in the activities of ADH and ALDH was observed. Coconut water was found to enhance the activity of ALDH (13.95%), whereas egg white did not show any significant effect on both ADH and ALDH activities. Other samples decreased the activity of ALDH significantly. Coffee decreased the ALDH activity by a large magnitude; hence it is not advisable to consume coffee post alcohol intake as it might lead to acetaldehyde build-up resulting in prolonged hangover. It was observed that dates increased the activity of ADH but decreased the activity of ALDH. Samples that were able to enhance ADH and ALDH or only ALDH will be able to prevent acetaldehyde build-up, and thus may serve as an AHO.
4.3. Correlation between antioxidant property and enzyme activity
Selected food commodities were evaluated for antioxidant potential which is reported in supplementary file ( Table S1-S6 ). The correlation between ADH activity, ALDH activity and percent inhibition was determined from the correlation graphs ( Fig. 1 .). It is evident that there was no correlation between ADH, ALDH, and antioxidant activities. The correlation between the hepatic enzymes, (ADH and ALDH) and the antioxidant property was also found to be invalid. It has been reported previously that a substance known to have high antioxidant activity can serve as an AHO as it will be able to reduce the oxidative stress induced in the body upon alcohol consumption. Asparagus officinalis ( Kim et al., 2009 ) and red ginseng ( Lee et al., 2014b ) have been reported to have the ability to exert a positive effect on the activity of the hepatic enzymes due to their antioxidant activity. A herbal formulation, "DTS20" has been shown to prevent gastric mucous damage and also accelerate the metabolism of alcohol ( Hong, 2015 ). Hence, it has been widely considered that compounds having antioxidant property would have the ability to enhance the activity of ADH and ALDH. However, from the results presented in Fig. 1 (A,B,C), there were hardly any correlations between the hepatic enzymes (ADH and ALDH) as well as between their activities and the antioxidant properties of the samples. These results emphasize the need to study the effect of food commodities on ADH and ALDH activities and further use them to screen for suitable AHO ingredients rather than relying solely on the antioxidant properties of substances.

A) Correlation between alcohol dehydrogenase (ADH) and aldehyde dehydrogenase (ALDH) activity, B) radical scavenging effect and ADH activity, and C) radical scavenging effect and ALDH activity.
4.4. Effect of selected food constituents on the kinetics of ADH and ALDH
On the basis of results on enhancement of ADH and/or ALDH activities obtained in section 2.0 , pear, sweet lime and coconut were selected for their effect on K m of these enzymes. The presence of sweet lime reduced the K m value for ADH (control) and ALDH (control) from 12.52 to 6.61 mM ( Fig. 2 A) and 5.30 μM to 2.62 μM ( Fig. 2 B), respectively. In case of pear juice, the apparent K m value of ADH reduced from 12.52 mM to 6.82 mM ( Fig. 2 C), but did not alter the K m of ALDH ( Fig. 2 D), while that of the control was 12.52 mM ( Fig. 2 C&D). Coconut water showed a very marginal decrease in K m value for ADH ( Fig. 2 E) and almost no change in the K m for ALDH activity ( Fig. 2 F). The decrease in the K m value, correlated with the increase in ADH activity of pear and sweet lime but not with coconut water. Similarly, the increase in activity of ALDH by pear and coconut could not be explained by the kinetic parameters, suggesting that there could be other mechanisms that cause this effect.

Lineweaver-Burk plot of A) aldehyde dehydrogenase (ALDH) catalyzed oxidation of ethanol in the absence (control) and presence of a modifier (sweet lime), and B) alcohol dehydrogenase (ADH) catalyzed oxidation of ethanol, in the absence (control) and presence of a modifier (sweet lime) C) aldehyde dehydrogenase (ALDH) catalyzed oxidation of ethanol in the absence (control) and presence of a modifier (pear), and D) alcohol dehydrogenase (ADH) catalyzed oxidation of ethanol, in the absence (control) and presence of a modifier (pear) E) aldehyde dehydrogenase (ALDH) catalyzed oxidation of ethanol in the absence (control) and presence of a modifier (coconut), and F) alcohol dehydrogenase (ADH) catalyzed oxidation of ethanol, in the absence (control) and presence of a modifier (coconut).
Similarly, other food commodities were also tested for their effect on kinetic parameters (data not shown) and it was observed that the increase in enzyme activities could be correlated to kinetic parameters only for few cases suggesting other mechanisms through which the activity of the enzyme may have altered. A few studies have reported the inhibitory effect of certain food components on the kinetics of ALDH. Durian fruit has shown to have a mixed type of inhibitory action on yeast ALDH ( Maninang et al., 2009 ), while quercetin has been shown to be a non-competitive inhibitor for ALDH ( Bhuiya et al., 2017 ). Further studies to ascertain the role of specific molecules in the food commodities that have activated ADH and ALDH evaluated in this work need to be carried out.
4.5. Formulation of anti-hangover (AHO) beverage
Anti-hangover effect can be expected when there is an increase in the ADH and/or ALDH activities. Further, the rate of conversion of acetaldehyde to other metabolites must be higher than the rate of conversion of alcohol to acetaldehyde. The results obtained in this study provide an insight into the dietary components that can be used to prepare an AHO formulation.
A beverage formulation is suitable among the various food types. Hence preliminary efforts were made to incorporate fruit (sweet lime, pear, and coconut water) and vegetable (cucumber and tomato) juices in a beverage formulation. However, all formulations containing vegetable juices were not acceptable by the sensory panel. Hence three fruit juices, viz . sweet lime, pear, and coconut water, were selected for the AHO beverage formulation. Mango and watermelon were not selected for the beverage formulation despite their ability to enhance the activity of ADH, neither of them enhanced the activity of ALDH. This suggests that while these fruit juices can facilitate metabolism of alcohol to acetaldehyde, they would not metabolize it further to acetic acid, both of which would result in acetaldehyde build-up, and hence retention of hangover. The upper and lower limits for all three components were set according to preliminary trials (based on sensory analysis)which were set as pear (25%–65%), sweet lime (25–65%), and coconut water (10–25%). Sixteen formulations were generated with different composition of each ingredient and evaluated randomly in terms of sensory perception ( Fig. 3 .). The formulation containing comprising of pear (65% v/v), sweet lime (25% v/v), and coconut water (10% v/v) showed best acceptability, which was then compared with commercially available AHO ( Table S7 ). While the formulated AHO had a higher overall acceptability (7.8), it did not differ much in terms of appearance and aroma with other formulations in this set of experiments. The formulated AHO could enhance the activity of ADH by 23.31% and that of ALDH by 70.02%, thus validating its use as an AHO product. These findings are in accordance with the other investigators who have reported effective use of Korean pear in alleviating hangover ( Lee et al., 2013 ).

Response surface plot showing the effect of concentration of different components (pear, sweet lime, and coconut) on the overall acceptability of the anti-hangover (AHO) product.
Recently, a variety of anti-hangover (AHO) products have been launched into the market. “PartySmart” is a formulation containing grapes, gooseberry, date palm, Phyllanthus amarus , green chiretta ( Andrographis paniculata ), and chicory, which is reported to significantly reduce several of the hangover symptoms, and prevent formation of acetaldehyde adducts. As a result, rapid elimination of acetaldehyde from the blood occurs ( Ramakrishna et al., 2005 ). While the exact mechanism of the action by the product has not been elucidated, the study suggested the antioxidant property of the various ingredients in addition to the positive effect on ADH and ALDH by few ingredients in the product ( Venkataranganna et al., 2008 ). Similarly, a product named “Oh!K” containing turmeric, ginger, black pepper, green tea extracts has also been formulated. Studies have shown that this drink is an effective remedy to treat alcoholic hangover by replenishing the body with the vital nutrients that are lost as a result of hangover ( Gopi et al., 2014 ). Another product, “DotShot” containing curcumin as the principle component and other vital electrolytes, has also been reported to enhance the ALDH activity and thereby assist in the breakdown of acetaldehyde ( Harisha, 2018 ). "LIVitup" is an AHO tablet containing kalmegh ghan , a mixture of kalmegh ( Andrographis paniculata ) and neem ( Azadirachta indica ) leaves which prevents the hangover symptoms by reducing the acetaldehyde build-up in the body after alcohol consumption. The fruit juice blend developed as AHO formulation in the present study is a simple, effective, economical and ready-to-prepare alternative to these formulations with good sensory appeal.
5. Conclusion
This study analyzed the effect of certain common food commodities on hangover through in vitro studies by enhancing either alcohol dehydrogenase and aldehyde dehydrogenase activities, or at least the aldehyde dehydrogenase activity, to formulate an anti-hangover product. A beverage made from a blend of sweet lime, pear, and coconut water could be used to overcome hangover. No correlation between the antioxidant activity and the activity of alcohol dehydrogenase and aldehyde dehydrogenase were seen. This dispels the common belief that an antioxidant could serve as an anti-hangover product. The consumption of this beverage with cheese, cucumber, and tomatoes may further alleviate the hangover symptoms.
Conflict of interest
There is no conflict of interest with any individual or organization.
Acknowledgement
This work was supported by Department of Biotechnology (DBT), Government of India and Department of Science and Technology (DST), SERB (PDF/2017/001108), Government of India.
Appendix A Supplementary data to this article can be found online at https://doi.org/10.1016/j.crfs.2019.09.001 .
Appendix A. Supplementary data
The following is the Supplementary data to this article:
- Bajpai V.K., Kim N., Kim J., Kim K., Kang S.C. Protective effect of heat-treated cucumber ( Cucumis sativus L.) juice on alcohol detoxification in experimental rats. Pak. J. Pharm. Sci. 2016; 29 :1005–1009. [ PubMed ] [ Google Scholar ]
- Bhuiya S., Haque L., Pradhan A.B., Das S. Inhibitory effects of the dietary flavonoid quercetin on the enzyme activity of zinc (II)-dependent yeast alcohol dehydrogenase: spectroscopic and molecular docking studies. Int. J. Biol. Macromol. 2017; 95 :177–184. [ PubMed ] [ Google Scholar ]
- Cederbaum A.I. Alcohol metabolism. Clin. Liver Dis. 2012; 16 :667–685. [ PMC free article ] [ PubMed ] [ Google Scholar ]
- Floegel A., Kim D., Chung S., Koo S.I., Chun O.K. Comparison of ABTS/DPPH assays to measure antioxidant capacity in popular antioxidant-rich US foods. J. Food Compos. Anal. 2011; 24 :1043–1048. [ Google Scholar ]
- Gopi S., George R., Sriraam V.T., Abirami S., Thankachen R.U. Studies on the effectiveness and safety of anti hangover drink (Oh!K) in reducing cocktail (alcohol) induced hangover symptoms in adult male social drinkers. Int. J. Herb. Med. 2014; 2 :115–117. [ Google Scholar ]
- Harisha S. A study to evaluate the safety and efficacy of 'DOTSHOT' in the treatment of hangover due to alcohol intoxication. Eur. J. Pharm. Med. Res. 2018; 5 :680–689. [ Google Scholar ]
- Hong Y.H. Effects of the herb mixture, DTS20, on oxidative stress and plasma alcoholic metabolites after alcohol consumption in healthy young men. Integr. Med. Res. 2015; 5 :309–316. [ PMC free article ] [ PubMed ] [ Google Scholar ]
- Kim S. Effects of Phellinus spp . Extract on alcohol metabolic enzymes in alcohol-treated rats. Biomed. Sci. Lett. 2016; 22 :53–59. [ Google Scholar ]
- Kim B.Y., Cui Z., Lee S., Kim S., Kang H., Lee Y., Park D. Effects of Asparagus officinalis extracts on liver cell toxicity and ethanol metabolism. J. Food Sci. 2009; 74 :204–208. [ PubMed ] [ Google Scholar ]
- Kim S., Cho S.K., Min T., Kim Y., Yang S., Kim H., Hyun S., Kim H., Kim Y., Choi H. Ameliorating effects of mango ( Mangifera indica L.) fruit on plasma ethanol level in a mouse model assessed with 1H-NMR based metabolic profiling. J. Clin. Biochem. Nutr. 2011; 48 :214–221. [ PMC free article ] [ PubMed ] [ Google Scholar ]
- Konkit M., Choi W.J., Kim W. Aldehyde dehydrogenase activity in Lactococcus chungangensis : application in cream cheese to reduce aldehyde in alcohol metabolism. J. Dairy Sci. 2016; 99 :1–7. [ PubMed ] [ Google Scholar ]
- Lee H., Isse T., Kawamoto T., Woo H., Kim A.K., Park J.Y., Yang M. Effects and action mechanisms of Korean pear ( Pyrus pyrifolia cv. Shingo ) on alcohol detoxification. Phyther. Res. 2012; 26 :1753–1758. [ PubMed ] [ Google Scholar ]
- Lee H.S., Isse T., Kawamoto T., Baik H.W., Park J.Y., Yang M. Effect of Korean pear ( Pyrus pyrifolia cv. Shingo ) juice on hangover severity following alcohol consumption. Food Chem. Toxicol. 2013; 58 :101–106. [ PubMed ] [ Google Scholar ]
- Lee D.I., Kim S.T., Lee D.H., Yu J.M., Jang S.K., Joo S.S. Ginsenoside-free molecules from steam-dried ginseng berry promote ethanol metabolism: an alternative choice for an alcohol hangover. J. Food Sci. 2014; 79 :1323–1330. [ PubMed ] [ Google Scholar ]
- Lee M., Kwak J.H., Jeon G., Lee J., Seo J., Lee H., Lee J.H. Red ginseng relieves the effects of alcohol consumption and hangover symptoms in healthy men: a randomized crossover study. Food Funct. 2014; 5 :528–534. [ PubMed ] [ Google Scholar ]
- Maninang J.S., Lizada M.C.C., Gemma H. Inhibition of aldehyde dehydrogenase enzyme by durian ( Durio zibethinus Murray) fruit extract. Food Chem. 2009; 117 :352–355. [ Google Scholar ]
- Meneses N.G.T., Martins S., Teixeira J.A., Mussatto S.I. Influence of extraction solvents on the recovery of antioxidant phenolic compounds from brewer's spent grains. Separ. Purif. Technol. 2013; 108 :152–158. [ Google Scholar ]
- Pordié L. Hangover free! the social and material trajectories of PartySmart. Anthropol. Med. 2015; 22 :34–48. [ PubMed ] [ Google Scholar ]
- Pritchard S.R., Phillips M., Kailasapathy K. Identification of bioactive peptides in commercial Cheddar cheese. Food Res. Int. 2010; 43 :1545–1548. [ Google Scholar ]
- Quertemont E. Genetic polymorphism in ethanol metabolism: acetaldehyde contribution to alcohol abuse and alcoholism. Mol. Psychiatry. 2004; 9 :570–581. [ PubMed ] [ Google Scholar ]
- Ramadan M.F., Osman A.O.M., El-Akad H.M. Food ingredients total antioxidant potential of juices and beverages screening by DPPH in vitro assay. Dtsch. Lebensm.-Rundsch. 2008; 104 :235–239. [ Google Scholar ]
- Ramakrishna S., Manu M.B., Kolhapure S.A. Evaluation of the efficacy and safety of ‘PartySmart’ in the prevention of alcohol-induced hangover: a prospective, randomized, double blind, comparative, phase III clinical trial alcohol-induced hangover is a poorly understood physiological manifestation of the biochemical effects of alcoholic beverages on the body. Indian J. Clin. Pract. 2005; 15 :23–35. [ Google Scholar ]
- Su Y., Tian Y., Yan R., Wang C., Niu F., Yang Y. Study on a novel process for the separation of phospholipids, triacylglycerol and cholesterrol from egg yolk. J. Food Sci. Technol. 2015; 52 :4586–4592. [ PMC free article ] [ PubMed ] [ Google Scholar ]
- Subbaraj D.K., Baskaran A., Sureka I., Velmurugan M., Emelda E.A.J., Sathiyamurthy K. Bioactive potential of plants and spices extracts against human bacterial pathogens. IOSR J. Pharm. Biol. Sci. 2016; 11 :2278–3008. [ Google Scholar ]
- Swift R., Davidson D. Alcohol hangover: mechanisms and mediators. Alcohol Health Res. World. 1998; 22 :54–60. [ PMC free article ] [ PubMed ] [ Google Scholar ]
- Takahashi M., Li W., Koike K., Sadamoto K. Clinical effectiveness of KSS formula, a traditional folk remedy for alcohol hangover symptoms. J. Nat. Med. 2010; 64 :487–491. [ PubMed ] [ Google Scholar ]
- Venkataranganna M.V., Gopumadhavan S., Sundaram R., Peer G., Mitra S.K. Pharmacodynamics and toxicological profile of PartySmart, a herbal preparation for alcohol hangover in Wistar rats. Indian J. Med. Res. 2008; 127 :460–466. [ PubMed ] [ Google Scholar ]
- Wang F., Li Y., Zhang Y., Zhou Y., Li S., Li H. Natural products for the prevention and treatment of hangover and alcohol use disorder. Molecules. 2016; 21 :64–84. [ PMC free article ] [ PubMed ] [ Google Scholar ]
- Wang F., Zhang Y., Zhou Y., Li Y., Zhou T., Zheng J., Zhang J., Li S., Xu D., Li H. Effects of beverages on alcohol metabolism: potential health benefits and harmful impacts. Int. J. Mol. Sci. 2016; 17 :354–365. [ PMC free article ] [ PubMed ] [ Google Scholar ]
- Zakhari S. Overview: how is alcohol metabolized by the body? Alcohol Res. Health. 2006; 29 :245–254. [ PMC free article ] [ PubMed ] [ Google Scholar ]
- Zangiabadi N., Shekaari M., Sheibani V., Jafari M., Shabani M., Asadi A.R., Tajadini H., Jarahi M. Date fruit extract is a neuroprotective agent in diabetic peripheral neuropathy in streptozotocin-induced diabetic rats: a multimodal analysis. Oxid. Med. Cell Longev. 2011; 2011 :1–9. [ PMC free article ] [ PubMed ] [ Google Scholar ]
- Zhang Y., Wang F., Zhou Y., Li Y., Zhou T., Zheng J., Zhang J., Li S., Xu D., Li H. Effects of 20 selected fruits on ethanol metabolism: potential health benefits and harmful impacts. Int. J. Environ. Res. Public Health. 2016; 13 :399–411. [ PMC free article ] [ PubMed ] [ Google Scholar ]
- Zhao R.J., Huo C.Y., Qian Y., Ren D.F., Lu J. Ultra-high-pressure processing improves proteolysis and release of bioactive peptides with activation activities on alcohol metabolic enzymes in vitro from mushroom foot protein. Food Chem. 2017; 231 :25–32. [ PubMed ] [ Google Scholar ]
- Introduction
- Conclusions
- Article Information
eTable 1. Health Deficits of the Frailty Index in the UK Biobank Cohort
eTable 2. Association of Wine Preference and Drinking During Meals With Mortality in Older Drinkers From the UK Biobank Cohort
eTable 3. Association of Average Alcohol Intake Status With Mortality in Older Drinkers From the UK Biobank Cohort, Excluding Participants With Prevalent Cancer at Baseline for Cancer Mortality, or Those With Prevalent CVD at Baseline for CVD Mortality
eTable 4. Association of Wine Preference or Drinking During Meals With Mortality in Older Drinkers From the UK Biobank Cohort, Excluding Participants With Prevalent Cancer at Baseline for Cancer Mortality, or Those With Prevalent CVD at Baseline for CVD Mortality
eTable 5. Association of Wine Preference and Drinking During Meals With Mortality in Older Drinkers From the UK Biobank Cohort, Excluding Participants With Prevalent Cancer at Baseline for Cancer Mortality, or Those With Prevalent CVD at Baseline for CVD Mortality
eTable 6. Association of Average Alcohol Intake Status With Mortality in Older Drinkers From the UK Biobank Cohort, by Drinking Patterns, Excluding Participants With Prevalent Cancer at Baseline for Cancer Mortality, or Those With Prevalent CVD at Baseline for CVD Mortality
Data Sharing Statement
See More About
Sign up for emails based on your interests, select your interests.
Customize your JAMA Network experience by selecting one or more topics from the list below.
- Academic Medicine
- Acid Base, Electrolytes, Fluids
- Allergy and Clinical Immunology
- American Indian or Alaska Natives
- Anesthesiology
- Anticoagulation
- Art and Images in Psychiatry
- Artificial Intelligence
- Assisted Reproduction
- Bleeding and Transfusion
- Caring for the Critically Ill Patient
- Challenges in Clinical Electrocardiography
- Climate and Health
- Climate Change
- Clinical Challenge
- Clinical Decision Support
- Clinical Implications of Basic Neuroscience
- Clinical Pharmacy and Pharmacology
- Complementary and Alternative Medicine
- Consensus Statements
- Coronavirus (COVID-19)
- Critical Care Medicine
- Cultural Competency
- Dental Medicine
- Dermatology
- Diabetes and Endocrinology
- Diagnostic Test Interpretation
- Drug Development
- Electronic Health Records
- Emergency Medicine
- End of Life, Hospice, Palliative Care
- Environmental Health
- Equity, Diversity, and Inclusion
- Facial Plastic Surgery
- Gastroenterology and Hepatology
- Genetics and Genomics
- Genomics and Precision Health
- Global Health
- Guide to Statistics and Methods
- Hair Disorders
- Health Care Delivery Models
- Health Care Economics, Insurance, Payment
- Health Care Quality
- Health Care Reform
- Health Care Safety
- Health Care Workforce
- Health Disparities
- Health Inequities
- Health Policy
- Health Systems Science
- History of Medicine
- Hypertension
- Images in Neurology
- Implementation Science
- Infectious Diseases
- Innovations in Health Care Delivery
- JAMA Infographic
- Law and Medicine
- Leading Change
- Less is More
- LGBTQIA Medicine
- Lifestyle Behaviors
- Medical Coding
- Medical Devices and Equipment
- Medical Education
- Medical Education and Training
- Medical Journals and Publishing
- Mobile Health and Telemedicine
- Narrative Medicine
- Neuroscience and Psychiatry
- Notable Notes
- Nutrition, Obesity, Exercise
- Obstetrics and Gynecology
- Occupational Health
- Ophthalmology
- Orthopedics
- Otolaryngology
- Pain Medicine
- Palliative Care
- Pathology and Laboratory Medicine
- Patient Care
- Patient Information
- Performance Improvement
- Performance Measures
- Perioperative Care and Consultation
- Pharmacoeconomics
- Pharmacoepidemiology
- Pharmacogenetics
- Pharmacy and Clinical Pharmacology
- Physical Medicine and Rehabilitation
- Physical Therapy
- Physician Leadership
- Population Health
- Primary Care
- Professional Well-being
- Professionalism
- Psychiatry and Behavioral Health
- Public Health
- Pulmonary Medicine
- Regulatory Agencies
- Reproductive Health
- Research, Methods, Statistics
- Resuscitation
- Rheumatology
- Risk Management
- Scientific Discovery and the Future of Medicine
- Shared Decision Making and Communication
- Sleep Medicine
- Sports Medicine
- Stem Cell Transplantation
- Substance Use and Addiction Medicine
- Surgical Innovation
- Surgical Pearls
- Teachable Moment
- Technology and Finance
- The Art of JAMA
- The Arts and Medicine
- The Rational Clinical Examination
- Tobacco and e-Cigarettes
- Translational Medicine
- Trauma and Injury
- Treatment Adherence
- Ultrasonography
- Users' Guide to the Medical Literature
- Vaccination
- Venous Thromboembolism
- Veterans Health
- Women's Health
- Workflow and Process
- Wound Care, Infection, Healing
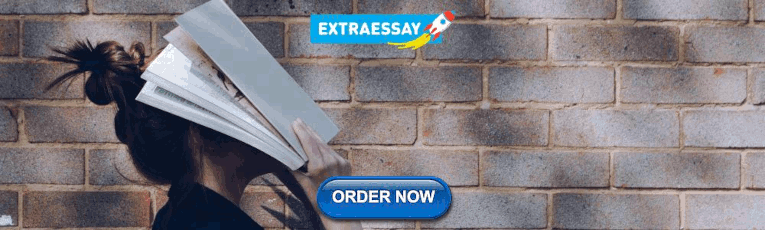
Get the latest research based on your areas of interest.
Others also liked.
- Download PDF
- X Facebook More LinkedIn
Ortolá R , Sotos-Prieto M , García-Esquinas E , Galán I , Rodríguez-Artalejo F. Alcohol Consumption Patterns and Mortality Among Older Adults With Health-Related or Socioeconomic Risk Factors. JAMA Netw Open. 2024;7(8):e2424495. doi:10.1001/jamanetworkopen.2024.24495
Manage citations:
© 2024
- Permissions
Alcohol Consumption Patterns and Mortality Among Older Adults With Health-Related or Socioeconomic Risk Factors
- 1 Department of Preventive Medicine and Public Health, Universidad Autónoma de Madrid, Madrid, Spain
- 2 Center for Biomedical Research in Epidemiology and Public Health, Madrid, Spain
- 3 Department of Environmental Health and Nutrition, Harvard T.H. Chan School of Public Health. Boston, Massachusetts
- 4 Madrid Institute for Advanced Studies Food Institute, Campus of International Excellence Universidad Autónoma de Madrid + Spanish National Research Council, Madrid, Spain
- 5 Department of Chronic Diseases, National Center for Epidemiology, Carlos III Health Institute, Madrid, Spain
Question Do health-related or socioeconomic risk factors modify the associations of alcohol consumption patterns with mortality among older drinkers?
Findings This cohort study in 135 103 older drinkers found that even low-risk drinking was associated with higher mortality among older adults with health-related or socioeconomic risk factors. Wine preference and drinking only with meals were associated with attenuating the excess mortality associated with alcohol consumption.
Meaning This cohort study identified inequalities in the detrimental health outcomes associated with alcohol that should be addressed to reduce the high disease burden of alcohol use.
Importance Alcohol consumption is a leading cause of morbidity and mortality that may be more important in older adults with socioeconomic or health-related risk factors.
Objective To examine the association of alcohol consumption patterns with 12-year mortality and its modification by health-related or socioeconomic risk factors.
Design, Setting, and Participants This prospective cohort study used data from the UK Biobank, a population-based cohort. Participants were current drinkers aged 60 years or older. Data were analyzed from September 2023 to May 2024.
Exposure According to their mean alcohol intake in grams per day, participants’ drinking patterns were classified as occasional: ≤2.86 g/d), low risk (men: >2.86-20.00 g/d; women: >2.86-10.00 g/d), moderate risk (men: >20.00-40.00 g/d; women: >10.00-20.00 g/d) and high risk (men: >40.00 g/d; women: >20.00 g/d).
Main Outcomes and Measures Health-related risk factors were assessed with the frailty index, and socioeconomic risk factors were assessed with the Townsend deprivation index. All-cause and cause-specific mortality were obtained from death certificates held by the national registries. Analyses excluded deaths in the first 2 years of follow-up and adjusted for potential confounders, including drinking patterns and preferences.
Results A total of 135 103 participants (median [IQR] age, 64.0 [62.0-67.0] years; 67 693 [50.1%] women) were included. In the total analytical sample, compared with occasional drinking, high-risk drinking was associated with higher all-cause (hazard ratio [HR], 1.33; 95% CI, 1.24-1.42), cancer (HR, 1.39; 95% CI, 1.26-1.53), and cardiovascular (HR, 1.21; 95% CI, 1.04-1.41) mortality; moderate-risk drinking was associated with higher all-cause (HR, 1.10; 95% CI, 1.03-1.18) and cancer (HR, 1.15; 95% CI, 1.05-1.27) mortality, and low-risk drinking was associated with higher cancer mortality (HR, 1.11; 95% CI, 1.01-1.22). While no associations were found for low- or moderate-risk drinking patterns vs occasional drinking among individuals without socioeconomic or health-related risk factors, low-risk drinking was associated with higher cancer mortality (HR, 1.15; 95% CI, 1.01-1.30) and moderate-risk drinking with higher all-cause (HR, 1.10; 95% CI, 1.01-1.19) and cancer (HR, 1.19; 95% CI, 1.05-1.35) mortality among those with health-related risk factors; low-risk and moderate-risk drinking patterns were associated with higher mortality from all causes (low risk: HR, 1.14; 95% CI, 1.01-1.28; moderate risk: HR, 1.17; 95% CI, 1.03-1.32) and cancer (low risk: HR, 1.25; 95% CI, 1.04-1.50; moderate risk: HR, 1.36; 95% CI, 1.13-1.63) among those with socioeconomic risk factors. Wine preference (>80% of alcohol from wine) and drinking with meals showed small protective associations with mortality, especially from cancer, but only in drinkers with socioeconomic or health-related risk factors and was associated with attenuating the excess mortality associated with high-, moderate- and even low-risk drinking.
Conclusions and Relevance In this cohort study of older drinkers from the UK, even low-risk drinking was associated with higher mortality among older adults with health-related or socioeconomic risk factors. The attenuation of mortality observed for wine preference and drinking only during meals requires further investigation, as it may mostly reflect the effect of healthier lifestyles, slower alcohol absorption, or nonalcoholic components of beverages.
Alcohol consumption is a leading cause of morbidity and mortality, accounting for approximately 5.1% of the global burden of disease and 5.3% of all deaths and being responsible for significant social and economic losses, thus representing a major public health problem. 1 Additionally, the assumed benefits of drinking low amounts of alcohol, especially on cardiovascular disease (CVD) mortality, 2 - 4 are being questioned due to selection biases, reverse causation, and residual confounding, 5 supporting health messaging that the safest level of drinking is no drinking at all or less is better. 6 , 7 Selection biases are often overlooked, but they can lead to a systematic underestimation of alcohol-related burden. That is the case of the abstainer bias, whereby the apparently lower mortality of light drinkers compared with abstainers could be explained by the higher death risk of the abstainers because they include former drinkers who quit alcohol due to poor health, as well as lifetime abstainers, 5 who often have worse lifestyle and health characteristics than regular drinkers. 8 Also, the healthy drinker/survivor bias, caused by overrepresentation of healthier drinkers who have survived the deleterious effects of alcohol, can distort comparisons, especially in older age. 5 In addition, drinking habits may influence the association between the amount of alcohol consumed and health. In this context, wine preference has been associated with lower risk of death, 9 CVD morbimortality, 10 and diabetes, 11 attributing the beneficial associations of wine to its high content in polyphenols. 12 Furthermore, drinking with meals has been associated with lower risk of all-cause, non-CVD, and cancer deaths 13 and frailty, 14 so this might be a safer option for alcohol drinkers along with moderate consumption. 15
The health impact of alcohol consumption may be greater in individuals with socioeconomic or health-related risk factors. On one hand, older adults with health-related risk factors are more susceptible to the harmful outcomes associated with alcohol due to their greater morbidity, higher use of alcohol-interacting drugs, and reduced tolerance. 16 , 17 However, some studies have observed benefits of alcohol on unhealthy aging or frailty, especially of light alcohol intake 18 , 19 and of a Mediterranean alcohol drinking pattern, defined as moderate alcohol consumption, preferably wine and accompanying meals, 14 , 20 suggesting that the protective associations of these potentially beneficial drinking patterns might be greater in individuals with ill health, although they might be due to the aforementioned methodological issues. 5 Therefore, it would be of interest to examine whether health-related risk factors modify the associations between alcohol consumption patterns and mortality.
On the other hand, there is evidence that socioeconomically disadvantaged populations have higher rates of alcohol-related harms for equivalent and even lower amounts of alcohol, probably due to the coexistence of other health challenges, including less healthy lifestyles, and lower social support or access to health care. 21 , 22 Also, the potentially beneficial associations of wine preference and drinking during meals might be more important in individuals with socioeconomic risk factors. However, to our knowledge, no previous research has examined whether socioeconomic status modifies the associations between these potentially beneficial drinking patterns and health.
Therefore, the aim of our study is to examine the associations of several potentially beneficial alcohol consumption patterns, that is, consumption of low amounts of alcohol, wine preference, and drinking only during meals, with all-cause, cancer, and CVD mortality in older adults and their modification by health-related or socioeconomic risk factors, while addressing the main methodological issues deemed to bias such associations. Thus, we restrict analyses to current drinkers and use occasional drinkers instead of abstainers as the reference group to prevent selection biases, exclude deaths in the first 2 years of follow-up to reduce reverse causation, and adjust analyses for many sociodemographic, lifestyle, and clinical variables to palliate residual confounding. We also restrict analyses to older adults because most deaths occur in this population group, which also has a high prevalence of health-related risk factors and because the protective associations of alcohol consumption have been specifically observed in older adults, 6 which is consistent with our aim to study potentially beneficial drinking patterns.
This cohort study was approved by the North West Multi-Centre Research Ethics Committee, and all participants provided written informed consent before enrollment. This study is reported following the Strengthening the Reporting of Observational Studies in Epidemiology ( STROBE ) reporting guideline.
We used data from the UK Biobank cohort, a multicenter, prospective, population-based study with more than 500 000 participants aged 40 to 69 years identified from National Health Service primary care registers and enrolled at 22 assessment sites across England, Scotland, and Wales between 2006 and 2010. At the baseline assessment visit, they completed a computer-assisted interview and a touch-screen questionnaire on sociodemographic, lifestyle, and clinical characteristics, provided biological samples, and underwent physical and medical examinations. They were followed-up for mortality through linkage to national death registries. Additional information on the UK Biobank study has been reported elsewhere. 23 , 24
At the baseline assessment visit, study participants were asked about the frequency and mean amount of the main types of alcoholic beverages that they consumed, and alcohol content was estimated by multiplying the volume ingested (in milliliters) by the volume percentage of alcohol (4.5% for beer and cider, 11.5% for white and sparkling wine, 13% for red wine, 20% for fortified wine, and 40% for spirits) and by the specific gravity of ethanol (0.789 g/mL). According to their mean alcohol intake, drinking patterns were classified into occasional (≤2.86 g/d), low risk (men: >2.86-20.00 g/d; women: >2.86-10.00 g/d), moderate risk (men: >20.00-40.00 g/d; women: >10-20.00 g/d), and high risk (men: >40.00 g/d; women: >20.00 g/d), a categorization based on the recommendations from health authorities that we have used in previous studies. 25 - 27 When more than 80% of alcohol came from a certain type of beverage, drinkers were classified as with preference for wine, with preference for other drinks, or with no preference. 27 Participants were also classified as drinkers only during meals and as drinkers either only outside of meals or at any time. Finally, participants were classified as drinkers with no wine preference nor drinking only during meals, drinkers with wine preference or drinking only during meals, and drinkers with wine preference and drinking only during meals.
Health-related risk was assessed at baseline using the frailty index (FI) developed specifically for the UK Biobank 28 based on the procedure used by Rockwood et al. 29 A total of 49 health deficits were considered, most dichotomously (1 point if present and 0 points otherwise), and a few according to severity (0 points for no deficit, 0.25-0.75 points for mild to moderate deficits, and 1 point for severe deficit). The FI score was calculated as the total sum of points assigned to each health deficit divided by the number of deficits considered and ranged from 0.00 to 0.57. The complete list of health deficits and associated scores can be found in eTable 1 in Supplement 1 . Participants were considered to have health-related risk factors if they were prefrail or frail (FI > 0.12). 28
Socioeconomic risk was assessed at baseline using the Townsend deprivation index (TDI), 30 which measures the level of an area’s socioeconomic deprivation. TDI ranges from −6.26 to 10.16, with higher score indicating greater deprivation. Participants were considered to have socioeconomic risk factors if they lived in more deprived areas (TDI > 0) and not if they lived in more affluent areas (TDI ≤ 0).
Information on mortality was obtained from death certificates held by the National Health Service (NHS) Information Centre (NHS England) up to September 30, 2021, for participants in England and Wales, and by the NHS Central Register Scotland (National Records of Scotland) up to October 31, 2021, for participants in Scotland. 31 , 32 Length of follow-up was estimated as the time from the baseline assessment visit to the date of death or administrative censoring, whichever came first. Cause-specific mortality was ascertained with the International Statistical Classification of Diseases and Related Health Problems, Tenth Revision ( ICD-10 ) classification 33 : codes C00 to C97 as primary cause of death for cancer and codes I00 to I99 for CVD.
We also used baseline information on sociodemographic, lifestyle, and clinical characteristics, including sex, age, self-reported race and ethnicity, education (college or university degree; A levels, AS levels, or equivalent; O levels, General Certificate of Secondary Education, or equivalent; Certificate of Secondary Education or equivalent; National Vocational Qualification, Higher National Diploma, Higher National Certificate, or equivalent; other professional qualifications; and no qualifications), tobacco smoking (never, former, or current), leisure-time physical activity (metabolic equivalents of task-hours per week), time spent watching television (hours per day), and prevalent morbidities (diabetes, CVD, and cancer) that could have a potential effect on the amount of alcohol consumed. In the UK Biobank, race and ethnicity are classified as Asian (Indian, Pakistani, Bangladeshi, any other Asian background), Black (Caribbean, African, any other Black background), Chinese, multiple (White and Black Caribbean, White and Black African, White and Asian, any other mixed background), White (British, Irish, any other White background), and other (any group not specified, eg, Arab).
From 217 462 participants aged at least 60 years in the UK Biobank cohort, we excluded 36 284 with incomplete information on alcohol consumption, 10 456 never drinkers, 8295 former drinkers, and 20 167 known binge drinkers (those who consumed ≥6 units of alcohol in 1 session) to avoid classifying binge drinkers with low mean alcohol intake as low-risk drinkers. We additionally excluded 1140 participants who died in the first 2 years of follow-up and 6017 participants with missing information on the FI (194 participants), the TDI (116 participants), and potential confounders (5707 participants). Thus, the analytical sample included 135 103 individuals.
The associations of alcohol consumption patterns (mean alcohol intake status, wine preference, and drinking during meals) at baseline with all-cause and cause-specific mortality were summarized with hazard ratios (HRs) and their 95% CIs obtained from Cox regression; the models included interactions between alcohol consumption patterns and health-related or socioeconomic risk factors and adjusted for baseline sociodemographic (sex, age, race and ethnicity, education, and TDI [except when stratifying by socioeconomic risk factors]), lifestyle (tobacco smoking, leisure-time physical activity, and time spent watching television), and clinical characteristics (diabetes, CVD, cancer, and FI score [except when stratifying by health-related risk factors]) of study participants. Analyses of alcohol intake were further adjusted for wine preference and drinking during meals, whereas analyses of wine preference and drinking during meals were further adjusted for mean alcohol intake and the other drinking pattern.
To characterize whether wine preference and drinking during meals modified the association of mean alcohol intake with mortality, we tested interaction terms defined as the product of the categories of mean alcohol intake by 3 categories of drinking patterns (no wine preference nor drinking only during meals, wine preference or drinking only during meals, and wine preference and drinking only during meals).
Additionally, we assessed whether sociodemographic and lifestyle variables modified the study associations by testing interaction terms defined as the product of alcohol consumption patterns by categories of such variables (except mean alcohol intake status by sex, as sex was included in the definition of alcohol intake status). Since no interactions were found, the results are presented for the total sample. Finally, we performed additional sensitivity analyses excluding participants with prevalent cancer at baseline for cancer mortality or those with prevalent CVD at baseline for CVD mortality.
Statistical significance was set at 2-sided P < .05. Analyses were performed with Stata software version 17 (StataCorp). Data were analyzed from September 2023 to May 2024.
A total of 135 103 participants (median [IQR] age, 64.0 [62.0-67.0] years; 67 693 [50.1%] women) were included. Occasional drinkers less often identified as White; were more frequently residents in England, women, and never smokers; were less physically active; had a lower educational level, a lower prevalence of CVD; and had a higher prevalence of diabetes, cancer, and health-related risk factors. Having socioeconomic risk factors was less frequent in low- and moderate-risk drinkers ( Table 1 ).
Over a median (range) follow-up of 12.4 (2.0 to 14.8) years, 15 833 deaths were recorded, including 7871 cancer deaths and 3215 CVD deaths. Compared with occasional drinking, low-risk drinking was associated with higher cancer mortality (HR, 1.11; 95% CI, 1.01-1.22); moderate-risk drinking was associated with higher all-cause (HR, 1.10; 95% CI, 1.03-1.18) and cancer (HR, 1.15; 95% CI, 1.05-1.27) mortality; and high-risk drinking was associated with higher all-cause (HR, 1.33; 95% CI, 1.24-1.42), cancer (HR, 1.39; 95% CI, 1.26-1.53), and CVD (HR, 1.21; 95% CI, 1.04-1.41) mortality ( Table 2 ). Hazards were greater in individuals with health-related or socioeconomic risk factors vs those without across categories of alcohol intake. Interestingly, while no associations with mortality were found in participants without health-related or socioeconomic risk factors among low- or moderate-risk drinkers, low-risk drinkers with health-related risk factors had higher cancer mortality (HR, 1.15; 95% CI, 1.01-1.30) and moderate-risk drinkers with health-related risk factors had higher all-cause (HR, 1.10; 95% CI, 1.01-1.19) and cancer (HR, 1.19; 95% CI, 1.05-1.35) mortality ( Table 2 ). Likewise, both low-risk and moderate-risk drinkers with socioeconomic risk factors showed higher mortality from all causes (low risk: HR, 1.14; 1.01-1.28; moderate risk: 1.17; 95% CI, 1.03-1.32) and cancer (low-risk: HR, 1.25; 95% CI, 1.04-1.50; moderate risk: HR, 1.36; 95% CI, 1.13-1.63) ( Table 2 ).
Wine preference and drinking only during meals were associated with lower all-cause mortality only in participants with health-related risk factors (wine preference: HR, 0.92; 95% CI, 0.87-0.97; drinking only during meals: HR, 0.93; 95% CI, 0.89-0.97), as well as in participants with socioeconomic risk factors (wine preference: HR, 0.84; 95% CI, 0.78-0.90; drinking only during meals: HR, 0.83; 95% CI, 0.78-0.89) ( Table 3 ). Drinking only during meals was also associated with lower cancer mortality in participants with health-related risk factors (HR, 0.92; 95% CI, 0.86-0.99) or socioeconomic risk factors (HR, 0.85; 95% CI, 0.78-0.94) ( Table 3 ). Furthermore, in individuals with socioeconomic risk factors, wine preference was associated with lower cancer mortality (HR, 0.89; 95% CI, 0.80-0.99) and drinking only during meals with lower CVD mortality (HR, 0.86; 95% CI, 0.75-1.00) ( Table 3 ). Adhering to both drinking patterns was associated with lower all-cause, cancer, and CVD mortality in drinkers with health-related or socioeconomic risk factors, and to a lesser extent, with lower all-cause death in drinkers without health-related risk factors (eTable 2 in Supplement 1 ). Importantly, wine preference and drinking during meals modified the association of mean alcohol intake with mortality: the excess risk of all-cause, cancer, and CVD death for high-risk drinkers, of all-cause and cancer death for moderate-risk drinkers, and of cancer death for low-risk drinkers vs occasional drinkers was attenuated and even lost among individuals with these drinking patterns ( Table 4 ). Analyses excluding participants with prevalent cancer at baseline for cancer mortality, or those with prevalent CVD at baseline for CVD mortality showed consistent results (eTables 3-6 in Supplement 1 ).
This cohort study in older alcohol drinkers from the UK found that compared with occasional drinkers, low-risk drinkers had higher cancer mortality, moderate-risk drinkers had higher all-cause and cancer mortality, and high-risk drinkers had higher all-cause, cancer, and CVD mortality. The excess mortality associated with alcohol consumption was higher in individuals with health-related and socioeconomic risk factors, among whom even low-risk drinkers had higher mortality, especially from cancer. Wine preference and drinking only with meals showed small protective associations with mortality, especially from cancer, among drinkers with health-related and socioeconomic risk factors, and these 2 drinking patterns attenuated the excess mortality associated with high-, moderate-, and even low-risk drinking.
In line with recent research on the associations between alcohol use and health, 6 , 34 , 35 our results corroborate the detrimental outcomes associated with heavy drinking in older adults. However, we also found higher risk for all-cause and cancer deaths in moderate-risk drinkers, unlike most previous research, which has reported protective associations of low to moderate alcohol consumption, mainly for all-cause 2 - 4 , 36 and CVD 3 , 36 , 37 mortality, ischemic heart disease, 3 , 6 , 34 and diabetes, 6 or null associations with all-cause mortality, 38 CVD, 39 and unhealthy aging. 20 This discrepancy may be due to the implementation of an important methodological improvement in our analyses, that is, using occasional drinkers as the reference group instead of lifetime abstainers, to prevent selection bias caused by misclassification of former drinkers as abstainers, and to palliate residual confounding because they are more like light drinkers than are never drinkers. 40 , 41 In fact, another analysis of the UK Biobank cohort that also avoided selection biases found an increased CVD risk in the general population for drinking up to 14 units per week. 42
To our knowledge, there are no studies examining the potential modification of health-related risk factors on the association between alcohol use and health. The stronger associations between mean alcohol intake and mortality observed in older adults with health-related risk factors make sense, since they have more morbid conditions potentially aggravated by alcohol and greater use of alcohol-interacting medications than their counterparts without health-related risk factors. 16 , 17 The fact that even low-risk drinkers with these risk factors had higher risk of cancer death is an important finding, which is consistent with the reported increased risk of several types of cancer and cancer mortality even with very low amounts of alcohol. 6 , 36 , 37 , 43
Our results also suggest that socioeconomic status acts as a modifier of the association between the amount of alcohol consumed and mortality, as mortality hazard was much greater in individuals with socioeconomic risk factors than in individuals without, in line with previous research. 21 , 22 , 44 , 45 We even found a detrimental association of low amounts of alcohol with all-cause and cancer mortality in this group, unlike the MORGAM study by DiCasetnuovo et al 44 reporting a lower mortality associated with consuming no more than 10 g/d of alcohol, which was clearer in individuals with higher vs lower education. 44 These discrepant results could again be explained by the different reference groups used: occasional drinkers in our study and never drinkers in the MORGAM study. Importantly, although older adults with socioeconomic risk factors have a higher risk of ill health and death, probably due to the coexistence of other health challenges, especially poorer lifestyles, 21 , 22 the observed associations in our study were independent of lifestyles, suggesting that other factors should account for them.
Regarding the potentially beneficial drinking patterns, that is, wine preference and drinking during meals, the literature is inconsistent. A 2018 pool of studies 34 reported a nondifferential association of specific types of alcoholic drinks with all-cause mortality and several CVD outcomes, whereas other studies have found protective health associations for wine but not other beverages. 15 , 46 Drinking with meals has also shown protective associations with several health outcomes. 15 In our analysis, these drinking patterns modified the association between alcohol intake and death risk. On one hand, the protective association for mortality of these patterns was only observed in individuals with socioeconomic or health-related risk factors, independently of the amount of alcohol consumed. On the other hand, the detrimental association of alcohol intake was more evident in individuals without these patterns. These findings suggest that the less detrimental associations of alcohol intake from wine or during meals are not due to alcohol itself, but to other factors, including nonalcoholic components of wine, such as antioxidants, slower absorption of alcohol ingested with meals and its consequent reduced alcoholaemia, as well as spacing drinks when drinking only with meals, or more moderate attitudes in individuals who choose to adhere to these drinking patterns.
Our study has several strengths, such as the large sample size, the long follow-up, and the methodological improvements implemented to prevent selection biases and reduce reverse causation. However, it also has some limitations. First, alcohol intake was self-reported, and therefore prone to some degree of misclassification. Also, alcohol intake was measured only at baseline and not at multiple time points over the life span, not allowing us to take into account changes in alcohol intake before the baseline assessment or to redistribute former drinkers among categories of current drinkers to reduce selection bias; this may have led to an underestimation of the true effects of alcohol consumption. 5 Second, as in any observational study, we cannot entirely rule out residual confounding, despite adjusting for many potential confounders. And third, this study was conducted in older adults in the UK with a high proportion of White participants, so our results may not be generalizable to other racial ethnic groups or populations with different lifestyles, drinking patterns, or socioeconomic development.
This cohort study among older drinkers from the UK did not find evidence of a beneficial association between low-risk alcohol consumption and mortality; however, we observed a detrimental association of even low-risk drinking in individuals with socioeconomic or health-related risk factors, especially for cancer deaths. The attenuation of the excess mortality associated with alcohol among individuals who preferred to drink wine or drink only during meals requires further investigation to elucidate the factors that may explain it. Finally, these results have important public health implications because they identify inequalities in the detrimental health outcomes associated with alcohol that should be addressed to reduce the high burden of disease of alcohol use.
Accepted for Publication: May 30, 2024.
Published: August 12, 2024. doi:10.1001/jamanetworkopen.2024.24495
Open Access: This is an open access article distributed under the terms of the CC-BY License . © 2024 Ortolá R et al. JAMA Network Open .
Corresponding Author: Rosario Ortolá, MD, PhD, Department of Preventive Medicine and Public Health, School of Medicine, Universidad Autónoma de Madrid, Calle del Arzobispo Morcillo 4, 28029 Madrid, Spain ( [email protected] ).
Author Contributions: Dr Ortolá had full access to all of the data in the study and takes responsibility for the integrity of the data and the accuracy of the data analysis.
Concept and design: Ortolá.
Acquisition, analysis, or interpretation of data: All authors.
Drafting of the manuscript: Ortolá.
Critical review of the manuscript for important intellectual content: Sotos-Prieto, García-Esquinas, Galán, Rodríguez-Artalejo.
Statistical analysis: Ortolá.
Obtained funding: Sotos-Prieto, Rodríguez-Artalejo.
Administrative, technical, or material support: Rodríguez-Artalejo.
Supervision: García-Esquinas, Galán.
Conflict of Interest Disclosures: None reported.
Funding/Support: This work was supported by the Plan Nacional sobre Drogas, Ministry of Health of Spain (grant No. 2020/17), Instituto de Salud Carlos III, State Secretary of R+D+I and Fondo Europeo de Desarrollo Regional/Fondo Social Europeo (Fondo de Investigación en Salud grants No. 19/319, 20/896, and 22/1111), Agencia Estatal de Investigación (grant No. CNS2022-135623), Carlos III Health Institute and the European Union “NextGenerationEU (grant No. PMP21/00093), and the Fundación Francisco Soria Melguizo (Papel de la Disfunción Mitocondrial en la Relación Entre Multimorbilidad Crónica y Deterioro Funcional en Ancianos project grant). Mercedes Sotos-Prieto holds a Ramón y Cajal contract (contract No. RYC-2018-025069-I) from the Ministry of Science, Innovation and Universities.
Role of the Funder/Sponsor: The funders had no role in the design and conduct of the study; collection, management, analysis, and interpretation of the data; preparation, review, or approval of the manuscript; and decision to submit the manuscript for publication.
Data Sharing Statement: See Supplement 2 .
- Register for email alerts with links to free full-text articles
- Access PDFs of free articles
- Manage your interests
- Save searches and receive search alerts
- See us on facebook
- See us on twitter
- See us on youtube
- See us on linkedin
- See us on instagram
Massive biomolecular shifts occur in our 40s and 60s, Stanford Medicine researchers find
Time marches on predictably, but biological aging is anything but constant, according to a new Stanford Medicine study.
August 14, 2024 - By Rachel Tompa

We undergo two periods of rapid change, averaging around age 44 and age 60, according to a Stanford Medicine study. Ratana21 /Shutterstock.com
If it’s ever felt like everything in your body is breaking down at once, that might not be your imagination. A new Stanford Medicine study shows that many of our molecules and microorganisms dramatically rise or fall in number during our 40s and 60s.
Researchers assessed many thousands of different molecules in people from age 25 to 75, as well as their microbiomes — the bacteria, viruses and fungi that live inside us and on our skin — and found that the abundance of most molecules and microbes do not shift in a gradual, chronological fashion. Rather, we undergo two periods of rapid change during our life span, averaging around age 44 and age 60. A paper describing these findings was published in the journal Nature Aging Aug. 14.
“We’re not just changing gradually over time; there are some really dramatic changes,” said Michael Snyder , PhD, professor of genetics and the study’s senior author. “It turns out the mid-40s is a time of dramatic change, as is the early 60s. And that’s true no matter what class of molecules you look at.”
Xiaotao Shen, PhD, a former Stanford Medicine postdoctoral scholar, was the first author of the study. Shen is now an assistant professor at Nanyang Technological University Singapore.
These big changes likely impact our health — the number of molecules related to cardiovascular disease showed significant changes at both time points, and those related to immune function changed in people in their early 60s.
Abrupt changes in number
Snyder, the Stanford W. Ascherman, MD, FACS Professor in Genetics, and his colleagues were inspired to look at the rate of molecular and microbial shifts by the observation that the risk of developing many age-linked diseases does not rise incrementally along with years. For example, risks for Alzheimer’s disease and cardiovascular disease rise sharply in older age, compared with a gradual increase in risk for those under 60.
The researchers used data from 108 people they’ve been following to better understand the biology of aging. Past insights from this same group of study volunteers include the discovery of four distinct “ ageotypes ,” showing that people’s kidneys, livers, metabolism and immune system age at different rates in different people.

Michael Snyder
The new study analyzed participants who donated blood and other biological samples every few months over the span of several years; the scientists tracked many different kinds of molecules in these samples, including RNA, proteins and metabolites, as well as shifts in the participants’ microbiomes. The researchers tracked age-related changes in more than 135,000 different molecules and microbes, for a total of nearly 250 billion distinct data points.
They found that thousands of molecules and microbes undergo shifts in their abundance, either increasing or decreasing — around 81% of all the molecules they studied showed non-linear fluctuations in number, meaning that they changed more at certain ages than other times. When they looked for clusters of molecules with the largest changes in amount, they found these transformations occurred the most in two time periods: when people were in their mid-40s, and when they were in their early 60s.
Although much research has focused on how different molecules increase or decrease as we age and how biological age may differ from chronological age, very few have looked at the rate of biological aging. That so many dramatic changes happen in the early 60s is perhaps not surprising, Snyder said, as many age-related disease risks and other age-related phenomena are known to increase at that point in life.
The large cluster of changes in the mid-40s was somewhat surprising to the scientists. At first, they assumed that menopause or perimenopause was driving large changes in the women in their study, skewing the whole group. But when they broke out the study group by sex, they found the shift was happening in men in their mid-40s, too.
“This suggests that while menopause or perimenopause may contribute to the changes observed in women in their mid-40s, there are likely other, more significant factors influencing these changes in both men and women. Identifying and studying these factors should be a priority for future research,” Shen said.
Changes may influence health and disease risk
In people in their 40s, significant changes were seen in the number of molecules related to alcohol, caffeine and lipid metabolism; cardiovascular disease; and skin and muscle. In those in their 60s, changes were related to carbohydrate and caffeine metabolism, immune regulation, kidney function, cardiovascular disease, and skin and muscle.
It’s possible some of these changes could be tied to lifestyle or behavioral factors that cluster at these age groups, rather than being driven by biological factors, Snyder said. For example, dysfunction in alcohol metabolism could result from an uptick in alcohol consumption in people’s mid-40s, often a stressful period of life.
The team plans to explore the drivers of these clusters of change. But whatever their causes, the existence of these clusters points to the need for people to pay attention to their health, especially in their 40s and 60s, the researchers said. That could look like increasing exercise to protect your heart and maintain muscle mass at both ages or decreasing alcohol consumption in your 40s as your ability to metabolize alcohol slows.
“I’m a big believer that we should try to adjust our lifestyles while we’re still healthy,” Snyder said.
The study was funded by the National Institutes of Health (grants U54DK102556, R01 DK110186-03, R01HG008164, NIH S10OD020141, UL1 TR001085 and P30DK116074) and the Stanford Data Science Initiative.
- Rachel Tompa Rachel Tompa is a freelance science writer.
About Stanford Medicine
Stanford Medicine is an integrated academic health system comprising the Stanford School of Medicine and adult and pediatric health care delivery systems. Together, they harness the full potential of biomedicine through collaborative research, education and clinical care for patients. For more information, please visit med.stanford.edu .
Hope amid crisis
Psychiatry’s new frontiers
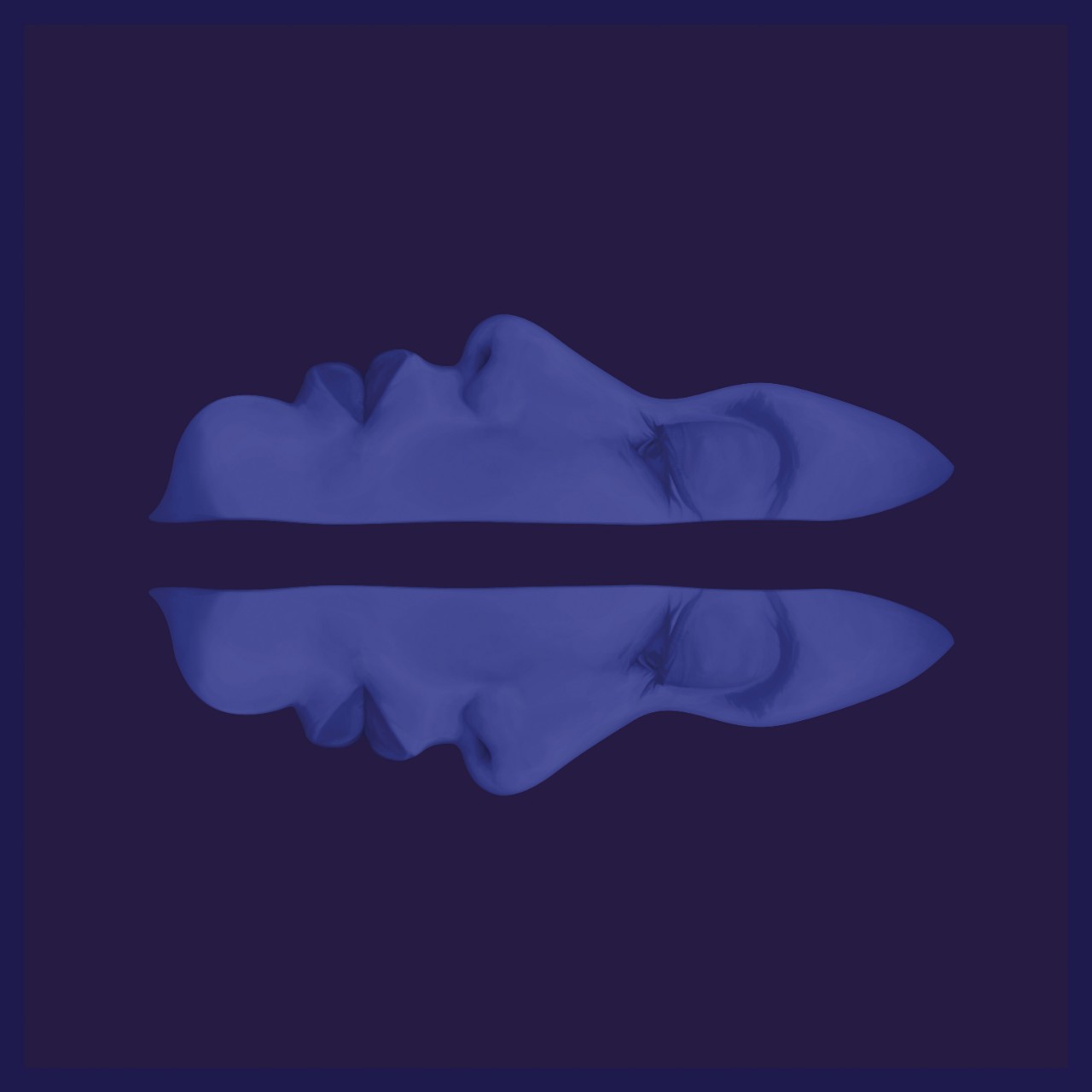
Thank you for visiting nature.com. You are using a browser version with limited support for CSS. To obtain the best experience, we recommend you use a more up to date browser (or turn off compatibility mode in Internet Explorer). In the meantime, to ensure continued support, we are displaying the site without styles and JavaScript.
- View all journals
- Explore content
- About the journal
- Publish with us
- Sign up for alerts
- Open access
- Published: 14 August 2024
Nonlinear dynamics of multi-omics profiles during human aging
- Xiaotao Shen ORCID: orcid.org/0000-0002-9608-9964 1 , 2 , 3 na1 ,
- Chuchu Wang ORCID: orcid.org/0000-0003-2015-7331 4 , 5 na1 ,
- Xin Zhou ORCID: orcid.org/0000-0001-8089-4507 1 , 6 ,
- Wenyu Zhou 1 ,
- Daniel Hornburg ORCID: orcid.org/0000-0002-6618-7774 1 ,
- Si Wu 1 &
- Michael P. Snyder ORCID: orcid.org/0000-0003-0784-7987 1 , 6
Nature Aging ( 2024 ) Cite this article
65k Accesses
2525 Altmetric
Metrics details
- Biochemistry
- Systems biology
Aging is a complex process associated with nearly all diseases. Understanding the molecular changes underlying aging and identifying therapeutic targets for aging-related diseases are crucial for increasing healthspan. Although many studies have explored linear changes during aging, the prevalence of aging-related diseases and mortality risk accelerates after specific time points, indicating the importance of studying nonlinear molecular changes. In this study, we performed comprehensive multi-omics profiling on a longitudinal human cohort of 108 participants, aged between 25 years and 75 years. The participants resided in California, United States, and were tracked for a median period of 1.7 years, with a maximum follow-up duration of 6.8 years. The analysis revealed consistent nonlinear patterns in molecular markers of aging, with substantial dysregulation occurring at two major periods occurring at approximately 44 years and 60 years of chronological age. Distinct molecules and functional pathways associated with these periods were also identified, such as immune regulation and carbohydrate metabolism that shifted during the 60-year transition and cardiovascular disease, lipid and alcohol metabolism changes at the 40-year transition. Overall, this research demonstrates that functions and risks of aging-related diseases change nonlinearly across the human lifespan and provides insights into the molecular and biological pathways involved in these changes.
Similar content being viewed by others
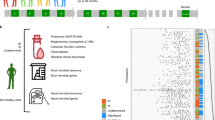
Personal aging markers and ageotypes revealed by deep longitudinal profiling
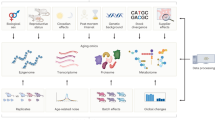
Considerations for reproducible omics in aging research
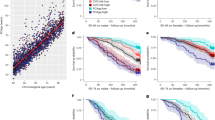
Principal component-based clinical aging clocks identify signatures of healthy aging and targets for clinical intervention
Aging is a complex and multifactorial process of physiological changes strongly associated with various human diseases, including cardiovascular diseases (CVDs), diabetes, neurodegeneration and cancer 1 . The alterations of molecules (including transcripts, proteins, metabolites and cytokines) are critically important to understand the underlying mechanism of aging and discover potential therapeutic targets for aging-related diseases. Recently, the development of high-throughput omics technologies has enabled researchers to study molecular changes at the system level 2 . A growing number of studies have comprehensively explored the molecular changes that occur during aging using omics profiling 3 , 4 , and most focus on linear changes 5 . It is widely recognized that the occurrence of aging-related diseases does not follow a proportional increase with age. Instead, the risk of these diseases accelerates at specific points throughout the human lifespan 6 . For example, in the United States, the prevalence of CVDs (encompassing atherosclerosis, stroke and myocardial infarction) is approximately 40% between the ages of 40 and 59, increases to about 75% between 60 and 79 and reaches approximately 86% in individuals older than 80 years 7 . Similarly, also in the United States, the prevalence of neurodegenerative diseases, such as Parkinson’s disease and Alzheimer’s disease, exhibits an upward trend as well as human aging progresses, with distinct turning points occurring around the ages of 40 and 65, respectively 8 , 9 , 10 . Some studies also found that brain aging followed an accelerated decline in flies 11 and chimpanzees 12 that lived past middle age and advanced age.
The observation of a nonlinear increase in the prevalence of aging-related diseases implies that the process of human aging is not a simple linear trend. Consequently, investigating the nonlinear changes in molecules will likely reveal previously unreported molecular signatures and mechanistic insights. Some studies examined the nonlinear alterations of molecules during human aging 13 . For instance, nonlinear changes in RNA and protein expression related to aging have been documented 14 , 15 , 16 . Moreover, certain DNA methylation sites have exhibited nonlinear changes in methylation intensity during aging, following a power law pattern 17 . Li et al. 18 identified the 30s and 50s as transitional periods during women’s aging. Although aging patterns are thought to reflect the underlying biological mechanisms, the comprehensive landscape of nonlinear changes of different types of molecules during aging remains largely unexplored. Remarkably, the global monitoring of nonlinear changing molecular profiles throughout human aging has yet to be fully used to extract basic insights into the biology of aging.
In the present study, we conducted a comprehensive deep multi-omics profiling on a longitudinal human cohort comprising 108 individuals aged from 25 years to 75 years. The cohort was followed over a span of several years (median, 1.7 years), with the longest monitoring period for a single participant reaching 6.8 years (2,471 days). Various types of omics data were collected from the participants’ biological samples, including transcriptomics, proteomics, metabolomics, cytokines, clinical laboratory tests, lipidomics, stool microbiome, skin microbiome, oral microbiome and nasal microbiome. The investigation explored the changes occurring across different omics profiles during human aging. Remarkably, many molecular markers and biological pathways exhibited a nonlinear pattern throughout the aging process, thereby providing valuable insight into periods of dramatic alterations during human aging.
Most of the molecules change nonlinearly during aging
We collected longitudinal biological samples from 108 participants over several years, with a median tracking period of 1.7 years and a maximum period of 6.8 years, and conducted multi-omics profiling on the samples. The participants were sampled every 3–6 months while healthy and had diverse ethnic backgrounds and ages ranging from 25 years to 75 years (median, 55.7 years). The participants’ body mass index (BMI) ranged from 19.1 kg m −2 to 40.8 kg m −2 (median, 28.2 kg m −2 ). Among the participants, 51.9% were female (Fig. 1a and Extended Data Fig. 1a–d ). For each visit, we collected blood, stool, skin swab, oral swab and nasal swab samples. In total, 5,405 biological samples (including 1,440 blood samples, 926 stool samples, 1,116 skin swab samples, 1,001 oral swab samples and 922 nasal swab samples) were collected. The biological samples were used for multi-omics data acquisition (including transcriptomics from peripheral blood mononuclear cells (PBMCs), proteomics from plasma, metabolomics from plasma, cytokines from plasma, clinical laboratory tests from plasma, lipidomics from plasma, stool microbiome, skin microbiome, oral microbiome and nasal microbiome; Methods ). In total, 135,239 biological features (including 10,346 transcripts, 302 proteins, 814 metabolites, 66 cytokines, 51 clinical laboratory tests, 846 lipids, 52,460 gut microbiome taxons, 8,947 skin microbiome taxons, 8,947 oral microbiome taxons and 52,460 nasal microbiome taxons) were acquired, resulting in 246,507,456,400 data points (Fig. 1b and Extended Data Fig. 1e,f ). The average sampling period and number of samples for each participant were 626 days and 47 samples, respectively. Notably, one participant was deeply monitored for 6.8 years (2,471 days), during which 367 samples were collected (Fig. 1c ). Overall, this extensive and longitudinal multi-omics dataset enables us to examine the molecular changes that occur during the human aging process. The detailed characteristics of all participants are provided in the Supplementary Data . For each participant, the omics data were aggregated and averaged across all healthy samples to represent the individual’s mean value, as detailed in the Methods section. Compared to cross-sectional cohorts, which have only a one-time point sample from each participant, our longitudinal dataset, which includes multiple time point samples from each participant, is more robust for detecting complex aging-related changes in molecules and functions. This is because analysis of multi-time point samples can detect participants’ baseline and robustly evaluate individuals’ longitudinal molecular changes.
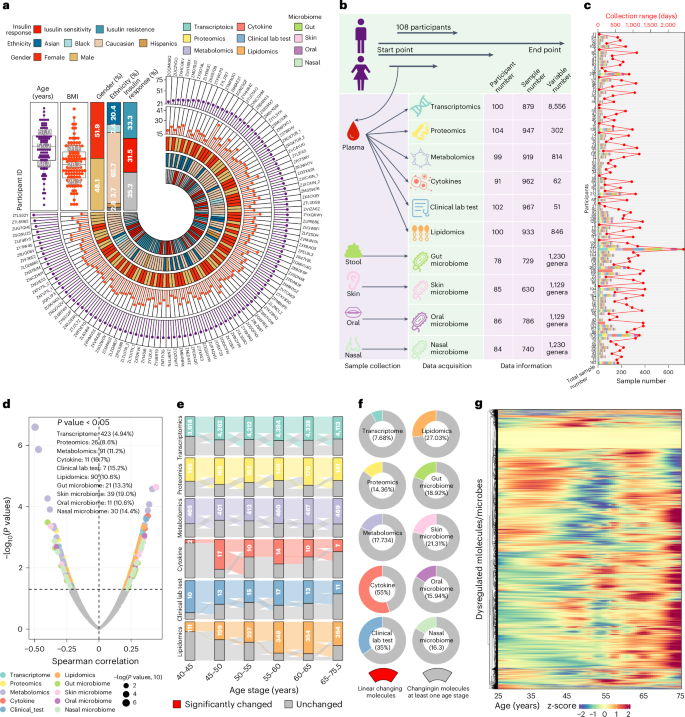
a , The demographics of the 108 participants in the study are presented. b , Sample collection and multi-omics data acquisition of the cohort. Four types of biological samples were collected, and 10 types of omics data were acquired. c , Collection time range and sample numbers for each participant. The top x axis represents the collection range for each participant (read line), and the bottom x axis represents the sample number for each participant (bar plot). Bars are color-coded by omics type. d , Significantly changed molecules and microbes during aging were detected using the Spearman correlation approach ( P < 0.05). The P values were not adjusted ( Methods ). Dots are color-coded by omics type. e , Differential expressional molecules/microbes in different age ranges compared to baseline (25–40 years old, two-sided Wilcoxon test, P < 0.05). The P values were not adjusted ( Methods ). f , The linear changing molecules comprised only a small part of dysregulated molecules in at least one age range. g , Heatmap depicting the nonlinear changing molecules and microbes during human aging.
We included samples only from healthy visits and adjusted for confounding factors (for example, BMI, sex, insulin resistance/insulin sensitivity (IRIS) and ethnicity; Extended Data Fig. 1a–d ), allowing us to discern the molecules and microbes genuinely associated with aging ( Methods ). Two common and traditional approaches, linear regression and Spearman correlation, were first used to identify the linear changing molecules during human aging 5 . The linear regression method is commonly used for linear changing molecules. As expected, both approaches have very high consistent results for each type of omics data (Supplementary Fig. 1a ). For convenience, the Spearman correlation approach was used in the analysis. Interestingly, only a small portion of all the molecules and microbes (749 out of 11,305, 6.6%; only genus level was used for microbiome data; Methods ) linearly changed during human aging (Fig. 1d and Supplementary Fig. 1b ), consistent with our previous studies 5 ( Methods ). Next, we examined nonlinear effects by categorizing all participants into distinct age stages according to their ages and investigated the dysregulated molecules within each age stage compared to the baseline (25–40 years old; Methods ). Interestingly, using this approach, 81.03% of molecules (9,106 out of 11,305) exhibited changes in at least one age stage compared to the baseline (Fig. 1e and Extended Data Fig. 2a ). Remarkably, the percentage of linear changing molecules was relatively small compared to the overall dysregulated molecules during aging (mean, 16.2%) (Fig. 1f and Extended Data Fig. 2b ). To corroborate our findings, we employed a permutation approach to calculate permutated P values, which yielded consistent results ( Methods ). The heatmap depicting all dysregulated molecules also clearly illustrates pronounced nonlinear changes (Fig. 1g ). Taken together, these findings strongly suggest that a substantial number of molecules and microbes undergo nonlinear changes throughout human aging.
Clustering reveals nonlinear multi-omics changes during aging
Next, we assessed whether the multi-omics data collected from the longitudinal cohort could serve as reliable indicators of the aging process. Our analysis revealed a substantial correlation between a significant proportion of the omics data and the ages of the participants (Fig. 2a ). Particularly noteworthy was the observation that, among all the omics data examined, metabolomics, cytokine and oral microbiome data displayed the strongest association with age (Fig. 2a and Extended Data Fig. 3a–c ). Partial least squares (PLS) regression was further used to compare the strength of the age effect across different omics data types. The results are consistent with the results presented above in Fig. 2a ( Methods ). These findings suggest the potential utility of these datasets as indicators of the aging process while acknowledging that further research is needed for validation 4 . As the omics data are not accurately matched across all the samples, we then smoothed the omics data using our previously published approach 19 ( Methods and Supplementary Fig. 2a–c ). Next, to reveal the specific patterns of molecules that change during human aging, we then grouped all the molecules with similar trajectories using an unsupervised fuzzy c-means clustering approach 19 ( Methods , Fig. 3b and Supplementary Fig. 2d,e ). We identified 11 clusters of molecular trajectories that changed during aging, which ranged in size from 638 to 1,580 molecules/microbes (Supplementary Fig. 2f and Supplementary Data ). We found that most molecular patterns exhibit nonlinear changes, indicating that aging is not a linear process (Fig. 2b ). Among the 11 identified clusters, three distinct clusters (2, 4 and 5) displayed compelling, straightforward and easily understandable patterns that spanned the entire lifespan (Fig. 2c ). Most molecules within these three clusters primarily consist of transcripts (Supplementary Fig. 2f ), which is expected because transcripts dominate the multi-omics data (8,556 out of 11,305, 75.7%). Cluster 4 exhibits a relatively stable pattern until approximately 60 years of age, after which it shows a rapid decrease (Fig. 2c ). Conversely, clusters 2 and 5 display fluctuations before 60 years of age, followed by a sharp increase and an upper inflection point at approximately 55–60 years of age (Fig. 2c ). We also attempted to observe this pattern of molecular change during aging individually. The participant with the longest follow-up period of 6.8 years (Fig. 1c ) approached the age of 60 years (range, 59.5–66.3 years; Extended Data Fig. 1g ), and it was not possible to identify obvious patterns in this short time window (Supplementary Fig. 2g ). Tracking individuals longitudinally over longer periods (decades) will be required to observe these trajectories at an individual level.
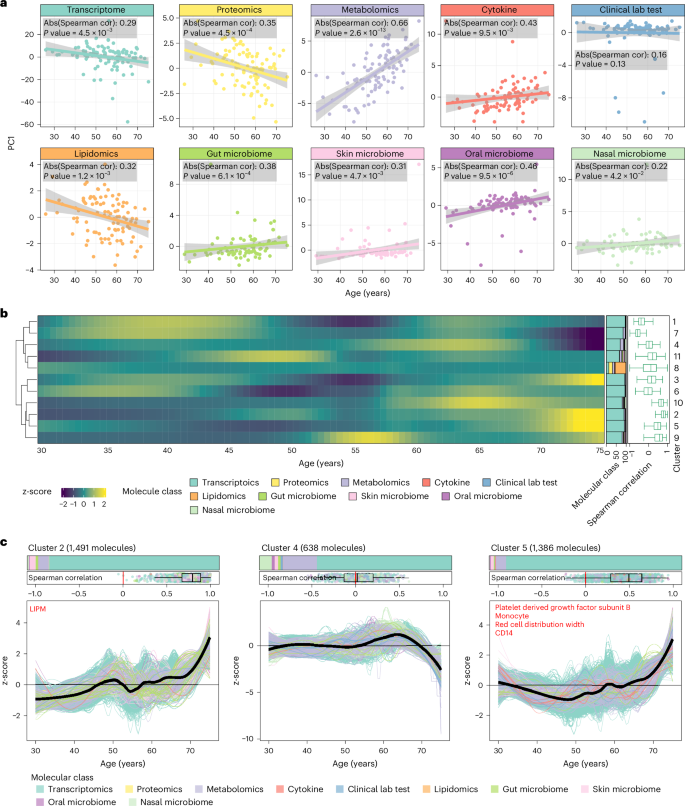
a , Spearman correlation (cor) between the first principal component and ages for each type of omics data. The shaded area around the regression line represents the 95% confidence interval. b , The heatmap shows the molecular trajectories in 11 clusters during human aging. The right stacked bar plots show the percentages of different kinds of omics data, and the right box plots show the correlation distribution between features and ages ( n = 108 participants). c , Three notable clusters of molecules that exhibit clear and straightforward nonlinear changes during human aging. The top stacked bar plots show the percentages of different kinds of omics data, and the top box plots show the correlation distribution between features and ages ( n = 108 participants). The box plot shows the median (line), interquartile range (IQR) (box) and whiskers extending to 1.5 × IQR. Bars and lines are color-coded by omics type. Abs, absolute.
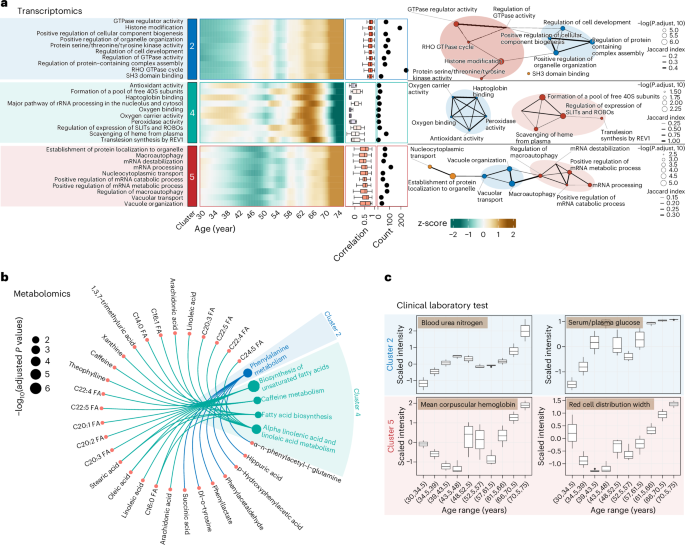
a , Pathway enrichment and module analysis for each transcriptome cluster. The left panel is the heatmap for the pathways that undergo nonlinear changes across aging. The right panel is the pathway similarity network ( Methods ) ( n = 108 participants). b , Pathway enrichment for metabolomics in each cluster. Enriched pathways and related metabolites are illustrated (Benjamini–Hochberg-adjusted P < 0.05). c , Four clinical laboratory tests that change during human aging: blood urea nitrogen, serum/plasma glucose, mean corpuscular hemoglobin and red cell distribution width ( n = 108 participants). The box plot shows the median (line), interquartile range (IQR) (box) and whiskers extending to 1.5 × IQR.
Although confounders, including sex, were corrected before analysis ( Methods ), we acknowledge that the age range for menopause in females is typically between 45 years and 55 years of age 20 , which is very close to the major transition points in all three clusters (Fig. 2c ). Therefore, we conducted further investigation into whether the menopausal status of females in the dataset contributed to the observed transition point at approximately 55 years of age (Fig. 2c ) by performing separate clustering analyses on the male and female datasets. Surprisingly, both the male and female datasets exhibited similar clusters, as illustrated in Extended Data Fig. 4a . This suggests that the transition point observed at approximately 55 years of age is not solely attributed to female menopause but, rather, represents a common phenomenon in the aging process of both sexes. This result is consistent with previous studies 14 , 15 , further supporting the notion that this transition point is a major characteristic feature of human aging. Moreover, to investigate the possibility that the transcriptomics data might skew the results toward transcriptomic changes as age-related factors, we conducted two additional clustering analyses—one focusing solely on transcriptomic data and another excluding it. Interestingly, both analyses yielded nearly identical three-cluster configurations, as observed using the complete omics dataset (Extended Data Fig. 4b ). This reinforces the robustness of the identified clusters and confirms that they are consistent across various omics platforms, not just driven by transcriptomic data.
Nonlinear changes in function and disease risk during aging
To gain further insight into the biological functions associated with the nonlinear changing molecules within the three identified clusters, we conducted separate functional analyses for transcriptomics, proteomics and metabolomics datasets for all three clusters. In brief, we constructed a similarity network using enriched pathways from various databases (Gene Ontology (GO), Kyoto Encyclopedia of Genes and Genomes (KEGG) and Reactome) and identified modules to eliminate redundant annotations. We then used all modules from different databases to reduce redundancy further using the same approach and define the final functional modules ( Methods , Extended Data Fig. 4c and Supplementary Data ). We identified some functional modules that were reported in previous studies, but we defined their more accurate patterns of change during human aging. Additionally, we also found previously unreported potential functional modules during human aging ( Supplementary Data ). For instance, in cluster 2, we identified a transcriptomic module associated with GTPase activity (adjusted P = 1.64 × 10 −6 ) and histone modification (adjusted P = 6.36 × 10 −7 ) (Fig. 3a ). Because we lack epigenomic data in this study, our findings should be validated through additional experiments in the future. GTPase activity is closely correlated with programmed cell death (apoptosis), and some previous studies showed that this activity increases during aging 21 . Additionally, histone modifications have been demonstrated to increase during human aging 22 . In cluster 4, we identified one transcriptomics module associated with oxidative stress; this module includes antioxidant activity, oxygen carrier activity, oxygen binding and peroxidase activity (adjusted P = 0.029) (Fig. 3a ). Previous studies demonstrated that oxidative stress and many reactive oxygen species (ROS) are positively associated with increased inflammation in relation to aging 23 . In cluster 5, the first transcriptomics module is associated with mRNA stability, which includes mRNA destabilization (adjusted P = 0.0032), mRNA processing (adjusted P = 3.2 × 10 −4 ), positive regulation of the mRNA catabolic process (adjusted P = 1.46 × 10 −4 ) and positive regulation of the mRNA metabolic process (adjusted P = 0.00177) (Fig. 3a ). Previous studies showed that mRNA turnover is associated with aging 24 . The second module is associated with autophagy (Fig. 3a ), which increases during human aging, as demonstrated in previous studies 25 .
In addition, we also identified certain modules in the clusters that suggest a nonlinear increase in several disease risks during human aging. For instance, in cluster 2, where components increase gradually and then rapidly after age 60, the phenylalanine metabolism pathway (adjusted P = 4.95 × 10 −4 ) was identified (Fig. 3b ). Previous studies showed that aging is associated with a progressive increase in plasma phenylalanine levels concomitant with cardiac dysfunction, and dysregulated phenylalanine catabolism is a factor that triggers deviations from healthy cardiac aging trajectories 26 . Additionally, C-X-C motif chemokine 5 (CXCL5 or ENA78) from proteomics data, which has higher concentrations in atherosclerosis 27 , is also detected in cluster 2 ( Supplementary Data ). The clinical laboratory test blood urea nitrogen, which provides important information about kidney function, is also detected in cluster 2 (Fig. 3c ). This indicates that kidney function nonlinearly decreases during aging. Furthermore, the clinical laboratory test for serum/plasma glucose, a marker of type 2 diabetes (T2D), falls within cluster 2. This is consistent with and supported by many previous studies demonstrating that aging is a major risk factor for T2D 28 . Collectively, these findings suggest a nonlinear escalation in the risk of cardiovascular and kidney diseases and T2D with advancing age, particularly after the age of 60 years (Fig. 2c ).
The identified modules in cluster 4 also indicate a nonlinear increase in disease risks. For instance, the unsaturated fatty acids biosynthesis pathway (adjusted P = 4.71 × 10 −7 ) is decreased in cluster 4. Studies have shown that unsaturated fatty acids are helpful in reducing CVD risk and maintaining brain function 29 , 30 . The pathway of alpha-linolenic acid and linolenic acid metabolism (adjusted P = 1.32 × 10 −4 ) can reduce aging-associated diseases, such as CVD 31 . We also detected the caffeine metabolism pathway (adjusted P = 7.34 × 10 −5 ) in cluster 4, which suggests that the ability to metabolize caffeine decreases during aging. Additionally, the cytokine MCP1 (chemokine (C-C motif) ligand 2 (CCL2)), a member of the CC chemokine family, plays an important immune regulatory role and is also in cluster 4 ( Supplementary Data ). These findings further support previous observations and highlight the nonlinear increase in age-related disease risk as individuals age.
Cluster 5 comprises the clinical tests of mean corpuscular hemoglobin and red cell distribution width (Fig. 3c ). These tests assess the average hemoglobin content per red blood cell and the variability in the size and volume of red blood cells, respectively. These findings align with the aforementioned transcriptomic data, which suggest a nonlinear reduction in the oxygen-carrying capacity associated with the aging process.
Aside from these three distinct clusters (Fig. 2c ), we also conducted pathway enrichment analysis across all other eight clusters, which displayed highly nonlinear trajectories, employing the same method (Fig. 2b and Supplementary Data ). Notably, cluster 11 exhibited a consistent increase up until the age of 50, followed by a decline until the age of 56, after which no substantial changes were observed up to the age of 75. A particular transcriptomics module related to DNA repair was identified, encompassing three pathways: positive regulation of double-strand break repair (adjusted P = 0.042), peptidyl−lysine acetylation (adjusted P = 1.36 × 10 −5 ) and histone acetylation (adjusted P = 3.45 × 10 −4 ) (Extended Data Fig. 4d ). These three pathways are critical in genomic stability, gene expression and metabolic balances, with their levels diminishing across the human lifespan 32 , 33 , 34 . Our findings reveal a nonlinear alteration across the human lifespan in these pathways, indicating an enhancement in DNA repair capabilities before the age of 50, a marked reduction between the ages of 50 and 56 and stabilization after that until the age of 75. The pathway enrichment results for all clusters are detailed in the Supplementary Data .
Altogether, the comprehensive functional analysis offers valuable insights into the nonlinear changes observed in molecular profiles and their correlations with biological functions and disease risks across the human lifespan. Our findings reveal that individuals aged 60 and older exhibit increased susceptibility to CVD, kidney issues and T2D. These results carry important implications for both the diagnosis and prevention of these diseases. Notably, many clinically actionable markers were identified, which have the potential for improved healthcare management and enhanced overall well-being of the aging population.
Uncovering waves of aging-related molecules during aging
Although the trajectory clustering approaches described above effectively identify nonlinear changing molecules and microbes that exhibit clear and compelling patterns throughout human aging, it may not be as effective in capturing substantial changes that occur at specific chronological aging periods. In such cases, alternative analytical approaches may be necessary to detect and characterize these dynamics. To gain a comprehensive understanding of changes in multi-omics profiling during human aging, we used a modified version of the DE-SWAN algorithm 14 , as described in the Methods section. This algorithm identifies dysregulated molecules and microbes throughout the human lifespan by analyzing molecule levels within 20-year windows and comparing two groups in 10-year parcels while sliding the window incrementally from young to old ages 14 . Using this approach and multiomics data, we detected changes at specific stages of lifespan and uncovered the sequential effects of aging. Our analysis revealed thousands of molecules exhibiting changing patterns throughout aging, forming distinct waves, as illustrated in Fig. 3a . Notably, we observed two prominent crests occurring around the ages of 45 and 65, respectively (Fig. 4a ). Notably, too, these crests were consistent with findings from a previous study that included only proteomics data 14 . Specifically, crest 2 aligns with our previous trajectory clustering result, indicating a turning point at approximately 60 years of age (Fig. 2c ).
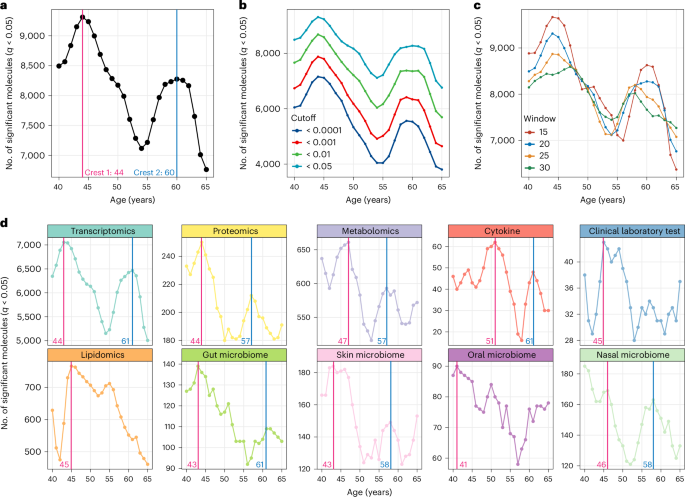
a , Number of molecules and microbes differentially expressed during aging. Two local crests at the ages of 44 years and 60 years were identified. b , c , The same waves were detected using different q value ( b ) and window ( c ) cutoffs. d , The number of molecules/microbes differentially expressed for different types of omics data during human aging.
To demonstrate the significance of the two crests, we employed different q value cutoffs and sliding window parameters, which consistently revealed the same detectable waves (Fig. 4b,c and Supplementary Fig. 4a,b ). Furthermore, when we permuted the ages of individuals, the crests disappeared (Supplementary Figs. 3a and 4c ) ( Methods ). These observations highlight the robustness of the two major waves of aging-related molecular changes across the human lifespan. Although we already accounted for confounders before our statistical analysis, we took additional steps to explore their impact. Specifically, we investigated whether confounders, such as insulin sensitivity, sex and ethnicity, differed between the two crests across various age ranges. As anticipated, these confounders did not show significant differences across other age brackets (Supplementary Fig. 4d ). This further supports our conclusion that the observed differences in the two crests are attributable to age rather than other confounding variables.
The identified crests represent notable milestones in the aging process and suggest specific age ranges where substantial molecular alterations occur. Therefore, we investigated the age-related waves for each type of omics data. Interestingly, most types of omics data exhibited two distinct crests that were highly robust (Fig. 3b and Supplementary Fig. 4 ). Notably, the proteomics data displayed two age-related crests at ages around 40 years and 60 years. Only a small overlap was observed between our dataset and the results from the previous study (1,305 proteins versus 302 proteins, with only 75 proteins overlapping). The observed pattern in our study was largely consistent with the previous findings 14 . However, our finding that many types of omics data, including transcriptomics, proteomics, metabolomics, cytokine, gut microbiome, skin microbiome and nasal microbiome, exhibit these waves, often with a similar pattern as the proteomics data (Fig. 4d ), supports the hypothesis that aging-related changes are not limited to a specific omics layer but, rather, involve a coordinated and systemic alteration across multiple molecular components. Identifying consistent crests across different omics data underscores the robustness and reliability of these molecular milestones in the aging process.
Next, we investigated the roles and functions of dysregulated molecules within two distinct crests. Notably, we found that the two crests related to aging predominantly consisted of the same molecules (Supplementary Fig. 6 ). To focus on the unique biological functions associated with each crest and eliminate commonly occurring molecules, we removed background molecules present in most stages. To explore the specific biological functions associated with each type of omics data (transcriptomics, proteomics and metabolomics) for both crests, we employed the function annotation approach described above ( Methods ). In brief, we constructed a similarity network of enriched pathways and identified modules to remove redundant annotations (Supplementary Fig. 6 and Extended Data Fig. 5a,b ). Furthermore, we applied the same approach to all modules to reduce redundancy and identify the final functional modules ( Methods and Extended Data Fig. 6a ). Our analysis revealed significant changes in multiple modules associated with the two crests (Extended Data Fig. 6b–d ). To present the results clearly, Fig. 5a displays the top 20 pathways (according to adjusted P value) for each type of omics data, and the Supplementary Data provides a comprehensive list of all identified functional modules.
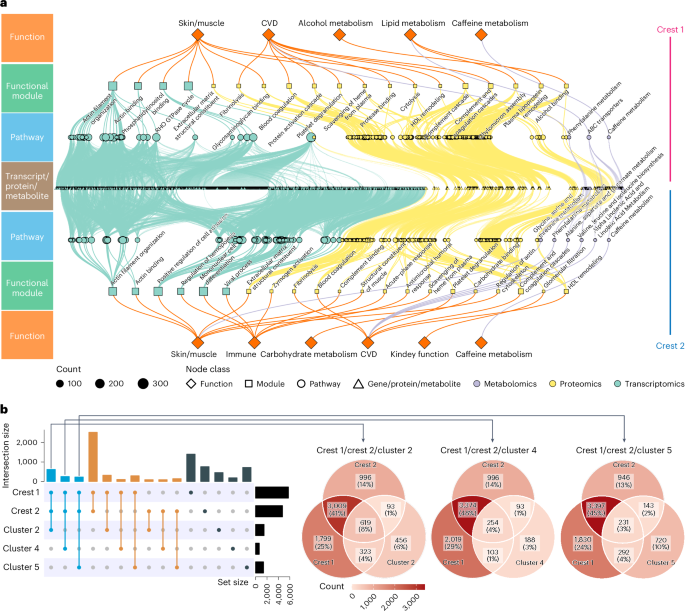
a , Pathway enrichment and biological functional module analysis for crests 1 and 2. Dots and lines are color-coded by omics type. b , The overlapping of molecules between two crests and three clusters.
Interestingly, the analysis identifies many dysregulated functional modules in crests 1 and 2, indicating a nonlinear risk for aging-related diseases. In particular, several modules associated with CVD were identified in both crest 1 and crest 2 (Fig. 5a ), which is consistent with the above results (Fig. 3b ). For instance, the dysregulation of platelet degranulation (crest 1: adjusted P = 1.77 × 10 −30 ; crest 2: adjusted P = 1.73 × 10 −26 ) 35 , 36 , complement cascade (crest 1: adjusted P = 3.84 × 10 −30 ; crest 2: adjusted P = 2.02 × 10 −28 ), complement and coagulation cascades (crest 1: adjusted P = 1.78 × 10 −46 ; crest 2: adjusted P = 2.02 × 10 −28 ) 37 , 38 , protein activation cascade (crest 1: adjusted P = 1.56 × 10 −17 ; crest 2: adjusted P = 1.61 × 10 −8 ) and protease binding (crest 1: adjusted P = 2.7 × 10 −6 ; crest 2: adjusted P = 0.0114) 39 have various effects on the cardiovascular system and can contribute to various CVDs. Furthermore, blood coagulation (crest 1: adjusted P = 1.48 × 10 −28 ; crest 2: adjusted P = 9.10 × 10 −17 ) and fibrinolysis (crest 1: adjusted P = 2.11 × 10 −15 ; crest 2: adjusted P = 1.64 × 10 −10 ) were also identified, which are essential processes for maintaining blood fluidity, and dysregulation in these modules can lead to thrombotic and cardiovascular events 40 , 41 . We also identified certain dysregulated metabolic modules associated with CVD. For example, aging has been linked to an incremental rise in plasma phenylalanine levels (crest 1: adjusted P = 9.214 × 10 −4 ; crest 2: adjusted P = 0.0453), which can contribute to the development of cardiac hypertrophy, fibrosis and dysfunction 26 . Branched-chain amino acids (BCAAs), including valine, leucine and isoleucine (crest 1: adjusted P : not significant (NS); crest 2: adjusted P = 0.0173), have also been implicated in CVD development 42 , 43 and T2D, highlighting their relevance in CVD pathophysiology. Furthermore, research suggests that alpha-linolenic acid (ALA) and linoleic acid metabolism (crest 1: adjusted P : NS; crest 2: adjusted P = 0.0217) may be protective against coronary heart disease 44 , 45 . Our investigation also identified lipid metabolism modules that are associated with CVD, including high-density lipoprotein (HDL) remodeling (crest 1: adjusted P = 1.073 × 10 −8 ; crest 2: adjusted P = 2.589 × 10 −9 ) and glycerophospholipid metabolism (crest 1: adjusted P : NS; crest 2: adjusted P = 0.0033), which influence various CVDs 46 , 47 , 48 .
In addition, the dysregulation of skin and muscle stability was found to be increased at crest 1 and crest 2, as evidenced by the identification of numerous modules associated with these processes (Fig. 5a,b ). This suggests that the aging of skin and muscle is markedly accelerated at crest 1 and crest 2. The extracellular matrix (ECM) provides structural stability, mechanical strength, elasticity and hydration to the tissues and cells, and the ECM of the skin is mainly composed of collagen, elastin and glycosaminoglycans (GAGs) 49 . Phosphatidylinositols are a class of phospholipids that have various roles in cytoskeleton organization 50 . Notably, the dysregulation of ECM structural constituent (crest 1: adjusted P = 3.32 × 10 −8 ; crest 2: adjusted P = 1.61 × 10 −8 ), GAG binding (crest 1: adjusted P = 1.805 × 10 −8 ; crest 2: adjusted P = 4.093 × 10 −6 ) and phosphatidylinositol binding (crest 1: adjusted P = 3.391 × 10 −6 ; crest 2: adjusted P = 7.832 × 10 −6 ) were identified 51 , 52 . We also identified cytolysis (crest 1: adjusted P = 2.973 × 10 −5 ; crest 2: adjusted P : NS), which can increase water loss 53 . The dysregulated actin binding (crest 1: adjusted P = 3.536 × 10 −8 ; crest 2: adjusted P = 3.435 × 10 −9 ), actin filament organization (crest 1: adjusted P = 8.406 × 10 −9 ; crest 2: adjusted P = 1.157 × 10 −9 ) and regulation of actin cytoskeleton (crest 1: adjusted P = 0.00090242; crest 2: adjusted P = 6.788 × 10 −4 ) were identified, which affect the structure and function of various tissues 54 , 55 , 56 , 57 , 58 . Additionally, cell adhesion is the attachment of a cell to another cell or to ECM via adhesion molecules 59 . We identified the positive regulation of cell adhesion (crest 1: adjusted P = 3.618 × 10 −5 ; crest 2: adjusted P = 8.272 × 10 −9 ) module, which can prevent or delay skin aging 60 , 61 . Threonine can affect sialic acid production, which is involved in cell adhesion 62 . We also identified the glycine, serine and threonine metabolism (crest 1: adjusted P : NS; crest 2: adjusted P = 0.00506) 62 . Additionally, scavenging of heme from plasma was identified (crest 1: adjusted P = 1.176 × 10 −11 ; crest 2: adjusted P = 1.694 × 10 −8 ), which can modulate skin aging as excess-free heme can damage cellular components 63 , 64 . Rho GTPases regulate a wide range of cellular responses, including changes to the cytoskeleton and cell adhesion (RHO GTPase cycle, crest 1: adjusted P = 9.956 × 10 −10 ; crest 2: adjusted P = 1.546 × 10 −5 ) 65 . In relation to muscle, previous studies demonstrated that muscle mass decreases by approximately 3–8% per decade after the age of 30, with an even higher decline rate after the age of 60, which consistently coincides with the observed second crest 66 . Interestingly, we identified dysregulation in the module associated with the structural constituent of muscle (crest 1: adjusted P = 0.00565; crest 2: adjusted P = 0.0162), consistent with previous findings 66 . Furthermore, we identified the pathway associated with caffeine metabolism (crest 1: adjusted P = 0.00378; crest 2: adjusted P = 0.0162), which is consistent with our observations above (Fig. 2b ) and implies that the capacity to metabolize caffeine undergoes a notable alteration not only around 60 years of age but also around the age of 40 years.
In crest 1, we identified specific modules associated with lipid and alcohol metabolism. Previous studies established that lipid metabolism declines with human aging 67 . Our analysis revealed several modules related to lipid metabolism, including plasma lipoprotein remodeling (crest 1: adjusted P = 2.269 × 10 −9 ), chylomicron assembly (crest 1: adjusted P = 9.065 × 10 −7 ) and ATP-binding cassette (ABC) transporters (adjusted P = 1.102 × 10 −4 ). Moreover, we discovered a module linked to alcohol metabolism (alcohol binding, adjusted P = 8.485 × 10 −7 ), suggesting a decline in alcohol metabolization efficiency with advancing age, particularly around the age of 40, when it significantly diminishes. In crest 2, we observed prominent modules related to immune dysfunction, encompassing acute-phase response (adjusted P = 2.851 × 10 −8 ), antimicrobial humoral response (adjusted P = 2.181 × 10 −5 ), zymogen activation (adjusted P = 4.367 × 10 −6 ), complement binding (adjusted P = 0.002568), mononuclear cell differentiation (adjusted P = 9.352 × 10 −8 ), viral process (adjusted P = 5.124 × 10 −7 ) and regulation of hemopoiesis (adjusted P = 3.522 × 10 −7 ) (Fig. 5a ). Age-related changes in the immune system, collectively known as immunosenescence, have been extensively documented 68 , 69 , 70 , and our results demonstrate a rapid decline at age 60. Furthermore, we also identified modules associated with kidney function (glomerular filtration, adjusted P = 0.00869) and carbohydrate metabolism (carbohydrate binding, adjusted P = 0.01045). Our previous findings indicated a decline in kidney function around the age of 60 years (Fig. 3c ), as did the present result of this observation. Previous studies indicated the influence of carbohydrates on aging, characterized by the progressive decline of physiological functions and increased susceptibility to diseases over time 71 , 72 .
In summary, our analysis identifies many dysregulated functional modules identified in both crest 1 and crest 2 that underlie the risk for various diseases and alterations of biological functions. Notably, we observed an overlap of dysregulated functional modules among clusters 2, 4 and 6 because they overlap at the molecular level, as depicted in Fig. 5b . This indicates that certain molecular components are shared among these clusters and the identified crests. However, it is important to note that numerous molecules are specific to each of the two approaches employed in our study. This suggests that these two approaches complement each other in identifying nonlinear changes in molecules and functions during human aging. By using both approaches, we were able to capture a more comprehensive understanding of the molecular alterations associated with aging and their potential implications for diseases.
Analyzing a longitudinal multi-omics dataset involving 108 participants, we successfully captured the dynamic and nonlinear molecular changes that occur during human aging. Our study’s strength lies in the comprehensive nature of the dataset, which includes multiple time point samples for each participant. This longitudinal design enhances the reliability and robustness of our findings compared to cross-sectional studies with only one time point sample for each participant. The first particularly intriguing finding from our analysis is that only a small fraction of molecules (6.6%) displayed linear changes throughout human aging (Fig. 1d ). This observation is consistent with previous research and underscores the limitations of relying solely on linear regression to understand the complexity of aging-related molecular changes 5 . Instead, our study revealed that a considerable number of molecules (81.0%) exhibited nonlinear patterns (Fig. 1e ). Notably, this nonlinear trend was observed across all types of omics data with remarkably high consistency (Fig. 1e,g ), highlighting the widespread functionally relevant nature of these dynamic changes. By unveiling the nonlinear molecular alterations associated with aging, our research contributes to a more comprehensive understanding of the aging process and its molecular underpinnings.
To further investigate the nonlinear changing molecules observed in our study, we employed a trajectory clustering approach to group molecules with similar temporal patterns. This analysis revealed the presence of three distinct clusters (Fig. 2c ) that exhibited clear and compelling patterns across the human lifespan. These clusters suggest that there are specific age ranges, such as around 60 years old, where distinct and extensive molecular changes occur (Fig. 2c ). Functional analysis revealed several modules that exhibited nonlinear changes during human aging. For example, we identified a module associated with oxidative stress, which is consistent with previous studies linking oxidative stress to the aging process 23 (Fig. 3a ). Our analysis indicates that this pathway increases significantly after the age of 60 years. In cluster 5, we identified a transcriptomics module associated with mRNA stabilization and autophagy (Fig. 3a ). Both of these processes have been implicated in the aging process and are involved in maintaining cellular homeostasis and removing damaged components. Furthermore, our analysis uncovered nonlinear changes in disease risk across aging. In cluster 2, we identified the phenylalanine metabolism pathway (Fig. 3b ), which has been associated with cardiac dysfunction during aging 26 . Additionally, we found that the clinical laboratory tests blood urea nitrogen and serum/plasma glucose increase significantly with age (cluster 2; Fig. 3c ), indicating a nonlinear decline in kidney function and an increased risk of T2D with age, with a critical threshold occurring approximately at the age of 60 years. In cluster 4, we identified pathways related to cardiovascular health, such as the biosynthesis of unsaturated fatty acids and caffeine metabolism (Fig. 3b ). Overall, our study provides compelling evidence for the existence of nonlinear changes in molecular profiles during human aging. By elucidating the specific functional modules and disease-related pathways that exhibit such nonlinear changes, we contribute to a better understanding of the complex molecular dynamics underlying the aging process and its implications for disease risk.
Although the trajectory clustering approach proves effective in identifying molecules that undergo nonlinear changes, it may not be as proficient in capturing substantial alterations that occur at specific time points without exhibiting a consistent pattern in other stages. We then employed a modified version of the DE-SWAN algorithm 14 to comprehensively investigate changes in multi-omics profiling throughout human aging. This approach enabled us to identify waves of dysregulated molecules and microbes across the human lifespan. Our analysis revealed two prominent crests occurring around the ages of 40 years and 60 years, which were consistent across various omics data types, suggesting their universal nature (Fig. 4a,e ). Notably, in the proteomics data, we observed crests around the ages of 40 years and 60 years, which aligns approximately with a previous study (which reported crests at ages 34 years, 60 years and 78 years) 14 . Due to the age range of our cohort being 25–75 years, we did not detect the third peak. Furthermore, the differences in proteomics data acquisition platforms (mass spectrometry versus SomaScan) 14 , 73 resulted in different identified proteins, with only a small overlap (1,305 proteins versus 302 proteins, of which only 75 were shared). This discrepancy may explain the age variation of the first crest identified in the two studies (approximately 10 years). However, despite the differences in the two proteomics datasets, the wave patterns observed in both studies were highly similar 14 (Fig. 4a ). Remarkably, by considering multiple omics data types, we consistently identified similar crests for each type, indicating the universality of these waves of change across plasma molecules and microbes from various body sites (Fig. 4e and Supplementary Fig. 3 ).
The analysis of molecular functionality in the two distinct crests revealed the presence of several modules, indicating a nonlinear increase in the risks of various diseases (Fig. 5a ). Both crest 1 and crest 2 exhibit the identification of multiple modules associated with CVD, which aligns with the aforementioned findings (Fig. 3b ). Moreover, we observed an escalated dysregulation in skin and muscle functioning in both crest 1 and crest 2. Additionally, we identified a pathway linked to caffeine metabolism, indicating a noticeable alteration in caffeine metabolization not only around the age of 60 but also around the age of 40. This shift may be due to either a metabolic shift or a change in caffeine consumption. In crest 1, we also identified specific modules associated with lipid and alcohol metabolism, whereas crest 2 demonstrated prominent modules related to immune dysfunction. Furthermore, we also detected modules associated with kidney function and carbohydrate metabolism, which is consistent with our above results. These findings reinforce our previous observations regarding a decline in kidney function around the age of 60 years (Fig. 3c ) while shedding light on the impact of dysregulated functional modules in both crest 1 and crest 2, suggesting nonlinear changes in disease risk and functional dysregulation. Notably, we identified an overlap of dysregulated functional modules among clusters 2, 4 and 6, indicating molecular-level similarities between these clusters and the identified crests (Fig. 5b ). This suggests the presence of shared molecular components among these clusters and crests. However, it is crucial to note that there are also numerous molecules specific to each of the two approaches employed in our study, indicating that these approaches complement each other in identifying nonlinear changes in molecules and functions during human aging.
The present research is subject to certain constraints. We accounted for many basic characteristics (confounders) of participants in the cohort; but because this study primarily reflects between-individual differences, there may be additional confounders due to the different age distributions of the participants. For example, we identified a notable decrease in oxygen carrier activity around age 60 (Figs. 2c and 3a ) and marked variations in alcohol and caffeine metabolism around ages 40 and 60 (Fig. 3a ). However, these findings might be shaped by participants’ lifestyle—that is, physical activity and their alcohol and caffeine intake. Regrettably, we do not have such detailed behavioral data for the entire group, necessitating validation in upcoming research. Although initial BMI and insulin sensitivity measurements were available at cohort entry, subsequent metrics during the observation span were absent, marking a study limitation.
A further constraint is our cohort’s modest size, encompassing merely 108 individuals (eight individuals between 25 years and 40 years of age), which hampers the full utilization of deep learning and may affect the robustness of the identification of nonlinear changing features in Fig. 1e . Although advanced computational techniques, including deep learning, are pivotal for probing nonlinear patterns, our sample size poses restrictions. Expanding the cohort size in subsequent research would be instrumental in harnessing the full potential of machine learning tools. Another limitation of our study is that the recruitment of participants was within the community around Stanford University, driven by rigorous sample collection procedures and the substantial expenses associated with setting up a longitudinal cohort. Although our participants exhibited a considerable degree of ethnic age and biological sex diversity (Fig. 1a and Supplementary Data ), it is important to acknowledge that our cohort may not fully represent the diversity of the broader population. The selectivity of our cohort limits the generalizability of our findings. Future studies should aim to include a more diverse cohort to enhance the external validity and applicability of the results.
In addition, the mean observation span for participants was 626 days, which is insufficient for detailed inflection point analyses. Our cohort’s age range of 25–70 years lacks individuals who lie outside of this range. The molecular nonlinearity detected might be subject to inherent variations or oscillations, a factor to consider during interpretation. Our analysis has not delved into the nuances of the dynamical systems theory, which provides a robust mathematical framework for understanding observed behaviors. Delving into this theory in future endeavors may yield enhanced clarity and interpretation of the data.
Moreover, it should be noted that, in our study, the observed nonlinear molecular changes occurred across individuals of varying ages rather than within the same individuals. This is attributed to the fact that, despite our longitudinal study, the follow-up period for our participants was relatively brief for following aging patterns (median, 1.7 years; Extended Data Fig. 1g ). Such a timeframe is inadequate for detecting nonlinear molecular changes that unfold over decades throughout the human lifespan. Addressing this limitation in future research is essential.
Lastly, our study’s molecular data are derived exclusively from blood samples, casting doubt on its direct relevance to specific tissues, such as the skin or muscles. We propose that blood gene expression variations might hint at overarching physiological alterations, potentially impacting the ECM in tissues, including skin and muscle. Notably, some blood-based biomarkers and transcripts have demonstrated correlations with tissue modifications, inflammation and other elements influencing the ECM across diverse tissues 74 , 75 .
In our future endeavors, the definitive confirmation of our findings hinges on determining if nonlinear molecular patterns align with nonlinear changes in functional capacities, disease occurrences and mortality hazards. For a holistic grasp of this, amalgamating multifaceted data from long-term cohort studies covering several decades becomes crucial. Such data should encompass molecular markers, comprehensive medical records, functional assessments and mortality data. Moreover, employing cutting-edge statistical techniques is vital to intricately decipher the ties between these nonlinear molecular paths and health-centric results.
In summary, the unique contribution of our study lies not merely in reaffirming the nonlinear nature of aging but also in the depth and breadth of the multi-omics data that we analyzed. Our study goes beyond stating that aging is nonlinear by identifying specific patterns, inflection points and potential waves in aging across multiple layers of biological data during human aging. Identifying specific clusters with distinct patterns, functional implications and disease risks enhances our understanding of the aging process. By considering the nonlinear dynamics of aging-related changes, we can gain insights into specific periods of significant changes (around age 40 and age 60) and the molecular mechanisms underlying age-related diseases, which could lead to the development of early diagnosis and prevention strategies. These comprehensive multi-omics data and the approach allow for a more nuanced understanding of the complexities involved in the aging process, which we think adds value to the existing body of research. However, further research is needed to validate and expand upon these findings, potentially incorporating larger cohorts to capture the full complexity of aging.
The participant recruitment, sample collection, data acquisition and data processing were documented in previous studies conducted by Zhou et al. 76 , Ahadi et al. 5 , Schüssler-Fiorenza Rose et al. 77 , Hornburg et al. 78 and Zhou et al. 79 .
Participant recruitment
Participants provided informed written consent for the study under research protocol 23602, which was approved by the Stanford University institutional review board. This study adheres to all relevant ethical regulations, ensuring informed consents were obtained from all participants. All participants consented to publication of potentially identifiable information. The cohort comprised 108 participants who underwent follow-up assessments. Exclusion criteria encompassed conditions such as anemia, kidney disease, a history of CVD, cancer, chronic inflammation or psychiatric illnesses as well as any prior bariatric surgery or liposuction. Each participant who met the eligibility criteria and provided informed consent underwent a one-time modified insulin suppression test to quantify insulin-mediated glucose uptake at the beginning of the enrollment 76 . The steady-state plasma glucose (SSPG) levels served as a direct indicator of each individual’s insulin sensitivity in processing a glucose load. We categorized individuals with SSPG levels below 150 mg dl −1 as insulin sensitive and those with levels of 150 mg dl −1 or higher as insulin resistant 80 , 81 . Thirty-eight participants were missing SSPG values, rendering their insulin resistance or sensitivity status undetermined. We also collected fasting plasma glucose (FPG) data for 69 participants at enrollment. Based on the FPG levels, two participants were identified as having diabetes at enrollment, with FPG levels exceeding 126 mg dl −1 ( Supplementary Data ). Additionally, we measured hemoglobin A1C (HbA1C) levels during each visit, using it as a marker for average glucose levels over the past 3 months: 6.5% or higher indicates diabetes. Accordingly, four participants developed diabetes during the study period. At the beginning of the enrollment, BMI was also measured for each participant. Participants received no compensation.
Comprehensive sample collection was conducted during the follow-up period, and multi-omics data were acquired (Fig. 1b ). For each visit, the participants self-reported as healthy or non-healthy 76 . To ensure accuracy and minimize the impact of confounding factors, only samples from individuals classified as healthy were selected for subsequent analysis.
Transcriptomics
Transcriptomic profiling was conducted on flash-frozen PBMCs. RNA isolation was performed using a QIAGEN All Prep kit. Subsequently, RNA libraries were assembled using an input of 500 ng of total RNA. In brief, ribosomal RNA (rRNA) was selectively eliminated from the total RNA pool, followed by purification and fragmentation. Reverse transcription was carried out using a random primer outfitted with an Illumina-specific adaptor to yield a cDNA library. A terminal tagging procedure was used to incorporate a second adaptor sequence. The final cDNA library underwent amplification. RNA sequencing libraries underwent sequencing on an Illumina HiSeq 2000 platform. Library quantification was performed via an Agilent Bioanalyzer and Qubit fluorometric quantification (Thermo Fisher Scientific) using a high-sensitivity dsDNA kit. After normalization, barcoded libraries were pooled at equimolar ratios into a multiplexed sequencing library. An average of 5–6 libraries were processed per HiSeq 2000 lane. Standard Illumina pipelines were employed for image analysis and base calling. Read alignment to the hg19 reference genome and personal exomes was achieved using the TopHat package, followed by transcript assembly and expression quantification via HTseq and DESeq2. In the realm of data pre-processing, genes with an average read count across all samples lower than 0.5 were excluded. Samples exhibiting an average read count lower than 0.5 across all remaining genes were likewise removed. For subsequent global variance and correlation assessments, genes with an average read count of less than 1 were eliminated.
Plasma sample tryptic peptides were fractionated using a NanoLC 425 System (SCIEX) operating at a flow rate of 5 μl min −1 under a trap-elute configuration with a 0.5 × 10 mm ChromXP column (SCIEX). The liquid chromatography gradient was programmed for a 43-min run, transitioning from 4% to 32% of mobile phase B, with an overall run time of 1 h. Mobile phase A consisted of water with 0.1% formic acid, and mobile phase B was formulated with 100% acetonitrile and 0.1% formic acid. An 8-μg aliquot of non-depleted plasma was loaded onto a 15-cm ChromXP column. Mass spectrometry analysis was executed employing SWATH acquisition on a TripleTOF 6600 system. A set of 100 variable Q1 window SWATH acquisition methods was designed in high-sensitivity tandem mass spectrometry (MS/MS) mode. Subsequent data analysis included statistical scoring of peak groups from individual runs via pyProphet 82 , followed by multi-run alignment through TRIC60, ultimately generating a finalized data matrix with a false discovery rate (FDR) of 1% at the peptide level and 10% at the protein level. Protein quantitation was based on the sum of the three most abundant peptide signals for each protein. Batch effect normalization was achieved by subtracting principal components that primarily exhibited batch-associated variation, using Perseus software v.1.4.2.40.
Untargeted metabolomics
A ternary solvent system of acetone, acetonitrile and methanol in a 1:1:1 ratio was used for metabolite extraction. The extracted metabolites were dried under a nitrogen atmosphere and reconstituted in a 1:1 methanol:water mixture before analysis. Metabolite profiles were generated using both hydrophilic interaction chromatography (HILIC) and reverse-phase liquid chromatography (RPLC) under positive and negative ion modes. Thermo Q Exactive Plus mass spectrometers were employed for HILIC and RPLC analyses, respectively, in full MS scan mode. MS/MS data were acquired using quality control (QC) samples. For the HILIC separations, a ZIC-HILIC column was used with mobile phase solutions of 10 mM ammonium acetate in 50:50 and 95:5 acetonitrile:water ratios. In the case of RPLC, a Zorbax SBaq column was used, and the mobile phase consisted of 0.06% acetic acid in water and methanol. Metabolic feature detection was performed using Progenesis QI software. Features from blanks and those lacking sufficient linearity upon dilution were excluded. Only features appearing in more than 33% of the samples were retained for subsequent analyses, and any missing values were imputed using the k -nearest neighbors approach. We employed locally estimated scatterplot smoothing (LOESS) normalization 83 to correct the metabolite-specific signal drift over time. The metid package 84 was used for metabolite annotation.
Cytokine data
A panel of 62 human cytokines, chemokines and growth factors was analyzed in EDTA-anticoagulated plasma samples using Luminex-based multiplex assays with conjugated antibodies (Affymetrix). Raw fluorescence measurements were standardized to median fluorescence intensity values and subsequently subjected to variance-stabilizing transformation to account for batch-related variations. As previously reported 76 , data points characterized by background noise, termed CHEX, that deviate beyond five standard deviations from the mean (mean ± 5 × s.d.) were excluded from the analyses.
Clinical laboratory test
The tests encompassed a comprehensive metabolic panel, a full blood count, glucose and HbA1C levels, insulin assays, high-sensitivity C-reactive protein (hsCRP), immunoglobulin M (IgM) and lipid, kidney and liver panels.
Lipid extraction and quantification procedures were executed in accordance with established protocols 78 . In summary, complex lipids were isolated from 40 μl of EDTA plasma using a solvent mixture comprising methyl tertiary-butyl ether, methanol and water, followed by a biphasic separation. Subsequent lipid analysis was conducted on the Lipidyzer platform, incorporating a differential mobility spectrometry device (SelexION Technology) and a QTRAP 5500 mass spectrometer (SCIEX).
Immediately after arrival, samples were stored at −80 °C. Stool and nasal samples were processed and sequenced in-house at the Jackson Laboratory for Genomic Medicine, whereas oral and skin samples were outsourced to uBiome for additional processing. Skin and oral samples underwent 30 min of beads-beating lysis, followed by a silica-guanidinium thiocyanate-based nucleic acid isolation protocol. The V4 region of the 16S rRNA gene was amplified using specific primers, after which the DNA was barcoded and sequenced on an Illumina NextSeq 500 platform via a 2 × 150-bp paired-end protocol. Similarly, stool and nasal samples were processed for 16S rRNA V1–V3 region amplification using a different set of primers and sequenced on an Illumina MiSeq platform. For data processing, the raw sequencing data were demultiplexed using BCL2FASTQ software and subsequently filtered for quality. Reads with a Q-score lower than 30 were excluded. The DADA2 R package was used for further sequence data processing, which included filtering out reads with ambiguous bases and errors, removing chimeras and aligning sequences against a validated 16S rRNA gene database. Relative abundance calculations for amplicon sequence variants (ASVs) were performed, and samples with inadequate sequencing depth (<1,000 reads) were excluded. Local outlier factor (LOF) was calculated for each point on a depth-richness plot, and samples with abnormal LOF were removed. In summary, rigorous procedures were followed in both the collection and processing stages, leveraging automated systems and specialized software to ensure the quality and integrity of the microbiome data across multiple body sites.
Statistics and reproducibility
For all data processing, statistical analysis and data visualization tasks, RStudio, along with R language (v.4.2.1), was employed. A comprehensive list of the packages used can be found in the Supplementary Note . The Benjamini–Hochberg method was employed to account for multiple comparisons. Spearman correlation coefficients were calculated using the R functions ‘cor’ and ‘cor.test’. Principal-component analysis (PCA) was conducted using the R function ‘princomp’. Before all the analyses, the confounders, such as BMI, sex, IRIS and ethnicity, were adjusted using the previously published method 19 . In brief, we used the intensity of each feature as the dependent variable (Y) and the confounding factors as the independent variables (X) to build a linear regression model. The residuals from this model were then used as the adjusted values for that specific feature.
All the omics data were acquired randomly. No statistical methods were used to predetermine the sample size, but our sample sizes are similar to those reported in previous publications 5 , 76 , 77 , 78 , 79 , and no data were excluded from the analyses. Additionally, the investigators were blinded to allocation during experiments and outcome assessment to the conditions of the experiments. Data distribution was assumed to be normal, but this was not formally tested.
The icons used in figures are from iconfont.cn, which can be used for non-commercial purposes under the MIT license ( https://pub.dev/packages/iconfont/license ).
Cross-sectional dataset generation
The ‘cross-sectional’ dataset was created by briefly extracting information from the longitudinal dataset. The mean value was calculated to represent each molecule’s intensity for each participant. Similarly, the age of each participant was determined by calculating the mean value of ages across all sample collection time points.
Linear changing molecule detection
We detected linear changing molecules during human aging using Spearman correlation and linear regression modeling. The confounders, such as BMI, sex, IRIS and ethnicity, were adjusted using the previously published method 19 . Our analysis revealed a high correlation between these two approaches in identifying such molecules. Based on these findings, we used the Spearman correlation approach to showcase the linear changing molecules during human aging. The permutation test was also used to get the permutated P values for each feature. In brief, each feature was subjected to sample label shuffling followed by a recalculation of the Spearman correlation. This process was reiterated 10,000 times, yielding 10,000 permuted Spearman correlations. The original Spearman correlation was then compared against these permuted values to obtain the permuted P values.
Dysregulated molecules compared to baseline during human aging
To depict the dysregulated molecules during human aging compared to the baseline, we categorized the participants into different age stages based on their ages. The baseline stage was defined as individuals aged 25–40 years. For each age stage group, we employed the Wilcoxon test to identify dysregulated molecules in comparison to the baseline, considering a significance threshold of P < 0.05. Before the statistical analysis, all the confounders were corrected. Subsequently, we visualized the resulting dysregulated molecules at different age stages using a Sankey plot. The permutation test was also used to get the permutated P values for each feature. In brief, we shuffled the sample labels and recalculated the absolute mean difference between the two groups, against which the actual absolute mean difference was benchmarked to derive the permuted P values. To identify the molecules and microbes that exhibited significant changes at any given age stage, we adjusted the P values for each feature by multiplying them by 6. This adjustment adheres to the Bonferroni correction method, ensuring a rigorous evaluation of statistical significance.
Evaluation of the age reflected by different types of omics data
To assess whether each type of omics data accurately reflects the ages of individuals in our dataset, we conducted a PCA. Subsequently, we computed the Spearman correlation coefficient between the ages of participants and the first principal component (PC1). The absolute value of this coefficient was used to evaluate the degree to which the omics data reflect the ages (Fig. 2a ). PLS regression was also used to compare the strength of the age effect to the different omics data types. In brief, the ‘pls’ function from the R package mixOmics was used to construct the regression model between omics data and ages. Then, the ‘perf’ function was used to assess the performance of all the modules with sevenfold cross-validation. The R 2 was extracted to assess the strength of the age effect on the different omics data types.
To accommodate the varying time points of biological and omics data, we employed the LOESS approach. This approach allowed us to smooth and predict the multi-omics data at specific time points (that is, every half year) 14 , 85 . In brief, for each molecule, we fitted a LOESS regression model. During the fitting process, the LOESS argument ‘span’ was optimized through cross-validation. This ensured that the LOESS model provided an accurate and non-overfitting fit to the data (Supplementary Fig. 2a,b ). Once we obtained the LOESS prediction model, we applied it to predict the intensity of each molecule at every half-year time point.
Trajectory clustering analysis
To conduct trajectory clustering analysis, we employed the fuzzy c-means clustering approach available in the R package ‘Mfuzz.’ This approach was previously described in our publication 19 . The analysis proceeded in several steps. First, the omics data were auto-scaled to ensure comparable ranges. Next, we computed the minimum centroid distances for a range of cluster numbers, specifically from 2 to 22, in step 1. These minimum centroid distances served as a cluster validity index, helping us determine the optimal cluster number. Based on predefined rules, we selected the optimal cluster number. To refine the accuracy of this selection, we merged clusters with center expression data correlations greater than 0.8 into a single cluster. This step aimed to capture similar patterns within the data. The resulting optimal cluster number was then used for the fuzzy c-means clustering. Only molecules with memberships above 0.5 were retained within each cluster for further analysis. This threshold ensured that the molecules exhibited a strong association with their assigned cluster and contributed considerably to the cluster’s characteristics.
Pathway enrichment analysis and functional module identification
Transcriptomics and proteomics pathway enrichment.
Pathway enrichment analysis was conducted using the ‘clusterProfiler’ R package 86 . The GO, KEGG and Reactome databases were used. The P values were adjusted using the Benjamini–Hochberg method, with a significance threshold set at <0.05. To minimize redundant enriched pathways and GO terms, we employed a series of analyses. First, for enriched GO terms, we used the ‘Wang’ algorithm from the R package ‘simplifyEnrichment’ to calculate the similarity between GO terms. Only connections with a similarity score greater than 0.7 were retained to construct the GO term similarity network. Subsequently, community analysis was performed using the ‘igraph’ R package to partition the network into distinct modules. The GO term with the smallest enrichment adjusted P value was chosen as the representative within each module. The same approach was applied to the enriched KEGG and Reactome pathways, with one slight modification. In this case, the ‘jaccard’ algorithm was used to calculate the similarity between pathways, and a similarity cutoff of 0.5 was employed for the Jaccard index. After removing redundant enriched pathways, we combined all the remaining GO terms and pathways. Subsequently, we calculated the similarity between these merged entities using the Jaccard index. This similarity analysis aimed to capture the overlap and relationships between the different GO terms and pathways. Using the same approach as before, we performed community analysis to identify distinct biological functional modules based on the merged GO terms and pathways.
Identification of functional modules
First, we used the ‘Wang’ algorithm for the GO database and the ‘jaccard’ algorithm for the KEGG and Reactome databases to calculate the similarity between pathways. The enriched pathways served as nodes in a similarity network, with edges representing the similarity between two nodes. Next, we employed the R package ‘igraph’ to identify modules within the network based on edge betweenness. By gradually removing edges with the highest edge betweenness scores, we constructed a hierarchical map known as a dendrogram, representing a rooted tree of the graph. The leaf nodes correspond to individual pathways, and the root node represents the entire graph 87 . We then merged pathways within each module, selecting the pathway with the smallest adjusted P value to represent the module. After this step, we merged pathways from all three databases into modules. Subsequently, we repeated the process by calculating the similarity between modules from all three databases using the ‘jaccard’ algorithm. Once again, we employed the same approach described above to identify the functional modules.
Metabolomics pathway enrichment
To perform pathway enrichment analysis for metabolomics data, we used the human KEGG pathway database. This database was obtained from KEGG using the R package ‘massDatabase’ 88 . For pathway enrichment analysis, we employed the hypergeometric distribution test from the ‘TidyMass’ project 89 . This statistical test allowed us to assess the enrichment of metabolites within each pathway. To account for multiple tests, P values were adjusted using the Benjamini–Hochberg method. We considered pathways with Benjamini–Hochberg-adjusted P values lower than 0.05 as significantly enriched.
Modified DE-SWAN
The DE-SWAN algorithm 14 was used. To begin, a unique age is selected as the center of a 20-year window. Molecule levels in individuals younger than and older than that age are compared using the Wilcoxon test to assess differential expression. P values are calculated for each molecule, indicating the significance of the observed differences. To ensure sufficient sample sizes for statistical analysis in each time window, the initial window ranges from ages 25 to 50. The left half of this window covers ages 25–40, whereas the right half spans ages 41–50. The window then moves in one-year steps; this is why Fig. 4 displays an age range of 40–65 years. To account for multiple comparisons, these P values are adjusted using Benjamini–Hochberg correction. To evaluate the robustness and relevance of the DE-SWAN results, the algorithm is tested with various parcel widths, including 15 years, 20 years, 25 years and 30 years. Additionally, different q value thresholds, such as <0.0001, <0.001, <0.01 and <0.05, are applied. By comparing the results obtained with these different parameters to results obtained by chance, we can assess the significance of the findings. To generate random results for comparison, the phenotypes of the individuals are randomly permuted, and the modified DE-SWAN algorithm is applied to the permuted dataset. This allows us to determine whether the observed results obtained with DE-SWAN are statistically significant and not merely a result of chance.
Reporting summary
Further information on research design is available in the Nature Portfolio Reporting Summary linked to this article.
Data availability
The raw data used in this study can be accessed without any restrictions on the National Institutes of Health Human Microbiome 2 project site ( https://portal.hmpdacc.org ). Both the raw and processed data are also available on the Stanford iPOP site ( http://med.stanford.edu/ipop.html ). Researchers and interested individuals can visit these websites to access the data. For further details and inquiries about the study, we recommend contacting the corresponding author, who can provide additional information and address any specific questions related to the research.
Code availability
The statistical analysis and data processing in this study were performed using R v.4.2.1, along with various base packages and additional packages. Detailed information about the specific packages used can be found in the Supplementary Note , which accompanies the manuscript. Furthermore, all the custom scripts developed for this study have been made openly accessible and can be found on the GitHub repository at https://github.com/jaspershen-lab/ipop_aging . By visiting this repository, researchers and interested individuals can access and use the custom scripts for their own analyses or to replicate the study’s findings.
Hou, Y. et al. Ageing as a risk factor for neurodegenerative disease. Nat. Rev. Neurol. 15 , 565–581 (2019).
Article PubMed Google Scholar
Chen, R. et al. Personal omics profiling reveals dynamic molecular and medical phenotypes. Cell 148 , 1293–1307 (2012).
Article CAS PubMed PubMed Central Google Scholar
Valdes, A. M., Glass, D. & Spector, T. D. Omics technologies and the study of human ageing. Nat. Rev. Genet. 14 , 601–607 (2013).
Rutledge, J., Oh, H. & Wyss-Coray, T. Measuring biological age using omics data. Nat. Rev. Genet. 23 , 715–727 (2022).
Ahadi, S. et al. Personal aging markers and ageotypes revealed by deep longitudinal profiling. Nat. Med. 26 , 83–90 (2020).
Ram, U. et al. Age-specific and sex-specific adult mortality risk in India in 2014: analysis of 0.27 million nationally surveyed deaths and demographic estimates from 597 districts. Lancet Glob. Health 3 , e767–e775 (2015).
Rodgers, J. L. et al. Cardiovascular risks associated with gender and aging. J. Cardiovasc. Dev. Dis. 6 , 19 (2019).
CAS PubMed PubMed Central Google Scholar
Poewe, W. et al. Parkinson disease. Nat. Rev. Dis. Primers 3 , 17013 (2017).
Hy, L. X. & Keller, D. M. Prevalence of AD among whites: a summary by levels of severity. Neurology 55 , 198–204 (2000).
Article CAS PubMed Google Scholar
Nussbaum, R. L. & Ellis, C. E. Alzheimer’s disease and Parkinson’s disease. N. Engl. J. Med. 348 , 1356–1364 (2003).
Xiong, Y. et al. Vimar/RAP1GDS1 promotes acceleration of brain aging after flies and mice reach middle age. Commun. Biol. 6 , 420 (2023).
Sherwood, C. C. et al. Aging of the cerebral cortex differs between humans and chimpanzees. Proc. Natl Acad. Sci. USA 108 , 13029–13034 (2011).
Márquez, E. J. et al. Sexual-dimorphism in human immune system aging. Nat. Commun. 11 , 751 (2020).
Article PubMed PubMed Central Google Scholar
Lehallier, B. et al. Undulating changes in human plasma proteome profiles across the lifespan. Nat. Med. 25 , 1843–1850 (2019).
Fehlmann, T. et al. Common diseases alter the physiological age-related blood microRNA profile. Nat. Commun. 11 , 5958 (2020).
Shavlakadze, T. et al. Age-related gene expression signature in rats demonstrate early, late, and linear transcriptional changes from multiple tissues. Cell Rep. 28 , 3263–3273 (2019).
Vershinina, O., Bacalini, M. G., Zaikin, A., Franceschi, C. & Ivanchenko, M. Disentangling age-dependent DNA methylation: deterministic, stochastic, and nonlinear. Sci Rep. 11 , 9201 (2021).
Li, J. et al. Determining a multimodal aging clock in a cohort of Chinese women. Med 4 , 825–848 (2023).
Shen, X. Multi-omics microsampling for the profiling of lifestyle-associated changes in health. Nat. Biomed. Eng . 8 , 11–29 (2024).
Takahashi, T. A. & Johnson, K. M. Menopause. Med. Clin. North Am. 99 , 521–534 (2015).
Umbayev, B. et al. Role of a small GTPase Cdc42 in aging and age-related diseases. Biogerontology 24 , 27–46 (2023).
Yi, S.-J. & Kim, K. New insights into the role of histone changes in aging. Int. J. Mol. Sci. 21 , 8241 (2020).
Liguori, I. et al. Oxidative stress, aging, and diseases. Clin. Interv. Aging 13 , 757–772 (2018).
Borbolis, F. & Syntichaki, P. Cytoplasmic mRNA turnover and ageing. Mech. Ageing Dev. 152 , 32–42 (2015).
Kaushik, S. et al. Autophagy and the hallmarks of aging. Ageing Res. Rev. 72 , 101468 (2021).
Czibik, G. et al. Dysregulated phenylalanine catabolism plays a key role in the trajectory of cardiac aging. Circulation 144 , 559–574 (2021).
Rousselle, A. et al. CXCL5 limits macrophage foam cell formation in atherosclerosis. J. Clin. Invest. 123 , 1343–1347 (2013).
Fazeli, P. K., Lee, H. & Steinhauser, M. L. Aging is a powerful risk factor for type 2 diabetes mellitus independent of body mass index. Gerontology 66 , 209–210 (2019).
Allayee, H., Roth, N. & Hodis, H. N. Polyunsaturated fatty acids and cardiovascular disease: implications for nutrigenetics. J. Nutrigenet. Nutrigenomics 2 , 140–148 (2009).
Sacks, F. M. et al. Dietary fats and cardiovascular disease: a presidential advisory from the American Heart Association. Circulation 136 , e1–e23 (2017).
Qi, W. et al. The ω-3 fatty acid α-linolenic acid extends Caenorhabditis elegans lifespan via NHR-49/PPARα and oxidation to oxylipins. Aging Cell 16 , 1125–1135 (2017).
Bird, A. W. et al. Acetylation of histone H4 by Esa1 is required for DNA double-strand break repair. Nature 419 , 411–415 (2002).
Sivanand, S. et al. Nuclear acetyl-CoA production by ACLY promotes homologous recombination. Mol. Cell 67 , 252–265 (2017).
Zhao, S. et al. Regulation of cellular metabolism by protein lysine acetylation. Science 327 , 1000–1004 (2010).
Vericel, E. et al. Platelets and aging I.—Aggregation, arachidonate metabolism and antioxidant status. Thromb. Res. 49 , 331–342 (1988).
Gu, S. X. & Dayal, S. Redox mechanisms of platelet activation in aging. Antioxidants (Basel) 11 , 995 (2022).
Oikonomopoulou, K., Ricklin, D., Ward, P. A. & Lambris, J. D. Interactions between coagulation and complement–their role in inflammation. Semin. Immunopathol. 34 , 151–165 (2012).
Wasiak, S. et al. Downregulation of the complement cascade in vitro, in mice and in patients with cardiovascular disease by the bet protein inhibitor apabetalone (RVX-208). J. Cardiovasc. Transl. 10 , 337–347 (2017).
Article Google Scholar
Slack, M. A. & Gordon, S. M. Protease activity in vascular disease. Arterioscl. Thromb. Vasc. Biol. 39 , e210–e218 (2019).
Mari, D. et al. Hemostasis and ageing. Immun. Ageing 5 , 12 (2008).
Lowe, G. & Rumley, A. The relevance of coagulation in cardiovascular disease: what do the biomarkers tell us? Thromb. Haemostasis 112 , 860–867 (2014).
Li, Y. et al. Branched chain amino acids exacerbate myocardial ischemia/reperfusion vulnerability via enhancing GCN2/ATF6/PPAR-α pathway-dependent fatty acid oxidation. Theranostics 10 , 5623–5640 (2020).
McGarrah, R. W. & White, P. J. Branched-chain amino acids in cardiovascular disease. Nat. Rev. Cardiol. 20 , 77–89 (2023).
Arsenian, M. Potential cardiovascular applications of glutamate, aspartate, and other amino acids. Clin. Cardiol. 21 , 620–624 (1998).
Grajeda-Iglesias, C. & Aviram, M. Specific amino acids affect cardiovascular diseases and atherogenesis via protection against macrophage foam cell formation: review article. Rambam Maimonides Med. J. 9 , e0022 (2018).
Chen, H. et al. Comprehensive metabolomics identified the prominent role of glycerophospholipid metabolism in coronary artery disease progression. Front. Mol. Biosci. 8 , 632950 (2021).
Giammanco, A. et al. Hyperalphalipoproteinemia and beyond: the role of HDL in cardiovascular diseases. Life (Basel) 11 , 581 (2021).
CAS PubMed Google Scholar
Zhu, Q. et al. Comprehensive metabolic profiling of inflammation indicated key roles of glycerophospholipid and arginine metabolism in coronary artery disease. Front. Immunol. 13 , 829425 (2022).
Yue, B. Biology of the extracellular matrix: an overview. J. Glaucoma 23 , S20–S23 (2014).
Zambrzycka, A. Aging decreases phosphatidylinositol-4,5-bisphosphate level but has no effect on activities of phosphoinositide kinases. Pol. J. Pharmacol. 56 , 651–654 (2004).
Lee, D. H., Oh, J.-H. & Chung, J. H. Glycosaminoglycan and proteoglycan in skin aging. J. Dermatol. Sci. 83 , 174–181 (2016).
Khan, A. U., Qu, R., Fan, T., Ouyang, J. & Dai, J. A glance on the role of actin in osteogenic and adipogenic differentiation of mesenchymal stem cells. Stem Cell Res. Ther. 11 , 283 (2020).
Lago, J. C. & Puzzi, M. B. The effect of aging in primary human dermal fibroblasts. PLoS ONE 14 , e0219165 (2019).
Pollard, T. D. Actin and actin-binding proteins. Cold Spring Harb. Perspect. Biol. 8 , a018226 (2016).
Lai, W.-F. & Wong, W.-T. Roles of the actin cytoskeleton in aging and age-associated diseases. Ageing Res. Rev. 58 , 101021 (2020).
Garcia, G., Homentcovschi, S., Kelet, N. & Higuchi-Sanabria, R. Imaging of actin cytoskeletal integrity during aging in C. elegans . Methods Mol. Biol. 2364 , 101–137 (2022).
Kim, Y. J. et al. Links of cytoskeletal integrity with disease and aging. Cells 11 , 2896 (2022).
Oosterheert, W., Klink, B. U., Belyy, A., Pospich, S. & Raunser, S. Structural basis of actin filament assembly and aging. Nature 611 , 374–379 (2022).
Bruzzone, A. et al. Dosage-dependent regulation of cell proliferation and adhesion through dual β2-adrenergic receptor/cAMP signals. FASEB J. 28 , 1342–1354 (2014).
McEver, R. P. & Luscinskas, F. W. Cell adhesion. In Hematology 7th edn (eds Hoffman, R. et al.) 127–134 (Elsevier, 2018).
Persa, O. D., Koester, J. & Niessen, C. M. Regulation of cell polarity and tissue architecture in epidermal aging and cancer. J. Invest. Dermatol. 141 , 1017–1023 (2021).
Canfield, C.-A. & Bradshaw, P. C. Amino acids in the regulation of aging and aging-related diseases. Transl. Med. Aging 3 , 70–89 (2019).
Chiabrando, D., Vinchi, F., Fiorito, V., Mercurio, S. & Tolosano, E. Heme in pathophysiology: a matter of scavenging, metabolism and trafficking across cell membranes. Front. Pharmacol. 5 , 61 (2014).
Aggarwal, S. et al. Heme scavenging reduces pulmonary endoplasmic reticulum stress, fibrosis, and emphysema. JCI Insight 3 , e120694 (2018).
Hodge, R. G. & Ridley, A. J. Regulating Rho GTPases and their regulators. Nat. Rev. Mol. Cell Biol. 17 , 496–510 (2016).
Siparsky, P. N., Kirkendall, D. T. & Garrett, W. E. Muscle changes in aging. Sports Health 6 , 36–40 (2014).
Johnson, A. A. & Stolzing, A. The role of lipid metabolism in aging, lifespan regulation, and age-related disease. Aging Cell 18 , e13048 (2019).
Paganelli, R., Scala, E., Quinti, I. & Ansotegui, I. J. Humoral immunity in aging. Aging Clin. Exp. Res. 6 , 143–150 (1994).
Article CAS Google Scholar
Goronzy, J. J. & Weyand, C. M. Understanding immunosenescence to improve responses to vaccines. Nat. Immunol. 14 , 428–436 (2013).
Cunha, L. L., Perazzio, S. F., Azzi, J., Cravedi, P. & Riella, L. V. Remodeling of the immune response with aging: immunosenescence and its potential impact on COVID-19 immune response. Front. Immunol. 11 , 1748 (2020).
Lee, D., Son, H. G., Jung, Y. & Lee, S.-J. V. The role of dietary carbohydrates in organismal aging. Cell. Mol. Life Sci. 74 , 1793–1803 (2017).
Franco-Juárez, B. et al. Effects of high dietary carbohydrate and lipid intake on the lifespan of C. elegans . Cells 10 , 2359 (2021).
Gold, L. et al. Aptamer-based multiplexed proteomic technology for biomarker discovery. PLoS ONE 5 , e15004 (2010).
Galliera, E., Tacchini, L. & Corsi Romanelli, M. M. Matrix metalloproteinases as biomarkers of disease: updates and new insights. Clin. Chem. Lab. Med. 53 , 349–355 (2015).
Golusda, L., Kühl, A. A., Siegmund, B. & Paclik, D. Extracellular matrix components as diagnostic tools in inflammatory bowel disease. Biology (Basel) 10 , 1024 (2021).
Zhou, W. et al. Longitudinal multi-omics of host–microbe dynamics in prediabetes. Nature 569 , 663–671 (2019).
Schüssler-Fiorenza Rose, et al. A longitudinal big data approach for precision health. Nat. Med. 25 , 792–804 (2019).
Hornburg, D. et al. Dynamic lipidome alterations associated with human health, disease and ageing. Nat. Metab. 5 , 1578–1594 (2023).
Zhou, X. et al. Longitudinal profiling of the microbiome at four body sites reveals core stability and individualized dynamics during health and disease. Cell Host Microbe 32 , 506–526 (2024).
Contreras, P. H., Serrano, F. G., Salgado, A. M. & Vigil, P. Insulin sensitivity and testicular function in a cohort of adult males suspected of being insulin-resistant. Front. Med. (Lausanne) 5 , 190 (2018).
Evans, D. J., Murray, R. & Kissebah, A. H. Relationship between skeletal muscle insulin resistance, insulin-mediated glucose disposal, and insulin binding. Effects of obesity and body fat topography. J. Clin. Invest. 74 , 1515–1525 (1984).
Röst, H. L., Schmitt, U., Aebersold, R. & Malmström, L. pyOpenMS: a Python-based interface to the OpenMS mass-spectrometry algorithm library. Proteomics 14 , 74–77 (2014).
Shen, X. et al. Normalization and integration of large-scale metabolomics data using support vector regression. Metabolomics 12 , 89 (2016).
Shen, X. et al. metID: an R package for automatable compound annotation for LC−MS-based data. Bioinformatics 38 , 568–569 (2022).
Marabita, F. et al. Multiomics and digital monitoring during lifestyle changes reveal independent dimensions of human biology and health. Cell Syst. 13 , 241–255 (2022).
Wu, T. et al. clusterProfiler 4.0: a universal enrichment tool for interpreting omics data. Innovation (Camb.) 2 , 100141 (2021).
Newman, M. E. J. & Girvan, M. Finding and evaluating community structure in networks. Phys. Rev. E 69 , 026113 (2004).
Shen, X., Wang, C. & Snyder, M. P. massDatabase: utilities for the operation of the public compound and pathway database. Bioinformatics 38 , 4650–4651 (2022).
Shen, X. et al. TidyMass an object-oriented reproducible analysis framework for LC–MS data. Nat. Commun. 13 , 4365 (2022).
Download references
Acknowledgements
We sincerely thank all the research participants for their dedicated involvement in this study. We also thank A. Chen and L. Stainton for their valuable administrative assistance. Additionally, we are deeply grateful to A.T. Brunger’s support for this work. This work was supported by National Institutes of Health (NIH) grants U54DK102556 (M.P.S.), R01 DK110186-03 (M.P.S.), R01HG008164 (M.P.S.), NIH S10OD020141 (M.P.S.), UL1 TR001085 (M.P.S.) and P30DK116074 (M.P.S.) and by the Stanford Data Science Initiative (M.P.S.). The funders had no role in study design, data collection and analysis, decision to publish or preparation of the manuscript.
Author information
These authors contributed equally: Xiaotao Shen, Chuchu Wang.
Authors and Affiliations
Department of Genetics, Stanford University School of Medicine, Stanford, CA, USA
Xiaotao Shen, Xin Zhou, Wenyu Zhou, Daniel Hornburg, Si Wu & Michael P. Snyder
Lee Kong Chian School of Medicine, Nanyang Technological University, Singapore, Singapore
Xiaotao Shen
School of Chemistry, Chemical Engineering and Biotechnology, Singapore, Singapore
Howard Hughes Medical Institute, Stanford University, Stanford, CA, USA
Chuchu Wang
Department of Molecular and Cellular Physiology, Stanford University, Stanford, CA, USA
Stanford Center for Genomics and Personalized Medicine, Stanford, CA, USA
Xin Zhou & Michael P. Snyder
You can also search for this author in PubMed Google Scholar
Contributions
X.S. and M.P.S. conceptualized and designed the study. X.Z. and W.Z. prepared the microbiome data. D.H. and W.S. prepared the lipidomics data. X.S. and C.W. conducted the data analysis. X.S. and C.W. prepared the figures. X.S., C.W. and M.P.S. contributed to the writing and revision of the manuscript, with input from other authors. M.S. and X.S. supervised the overall study.
Corresponding author
Correspondence to Michael P. Snyder .
Ethics declarations
Competing interests.
M.P.S. is a co-founder of Personalis, SensOmics, Qbio, January AI, Filtricine, Protos and NiMo and is on the scientific advisory boards of Personalis, SensOmics, Qbio, January AI, Filtricine, Protos, NiMo and Genapsys. D.H. has a financial interest in PrognomIQ and Seer. All other authors have no competing interests.
Peer review
Peer review information.
Nature Aging thanks Daniel Belsky and the other, anonymous, reviewer(s) for their contribution to the peer review of this work.
Additional information
Publisher’s note Springer Nature remains neutral with regard to jurisdictional claims in published maps and institutional affiliations.
Extended data
Extended data fig. 1 demographic data of all the participants in the study..
a , The ages positively correlate with BMI. The shaded area around the regression line represents the 95% confidence interval. b , Gender with age. c , Ethnicity with age. d , Insulin response with age. e , biological sample collection for all the participants. f , Overlap of the different kinds of omics data. g , The age range for each participant in this study.
Extended Data Fig. 2 Most of the molecules change nonlinearly during human aging.
a , Differential expressional microbes in different age ranges compared to baselines (25 – 40 years old, two-sided Wilcoxon test, p -value < 0.05). b , Most of the linear changing molecules and microbiota are also included in the molecules/microbes that significantly dysregulated at least one age range.
Extended Data Fig. 3 Omics data can represent aging.
PCA score plot of metabolomics data ( a ), cytokine ( b ), and oral microbiome ( c ).
Extended Data Fig. 4 Functional analysis of molecules in different clusters.
a , The Jaccard index between clusters from different datasets. b , The overlap between clusters using different types of omics data. c , Functional module detection and identification. d , Functional analysis of nonlinear changing molecules for all clusters.
Extended Data Fig. 5 Function annotation for significantly dysregulated molecules in crest 1 and 2.
a , Transcriptomics data. b , Proteomics data. c , Metabolomics data.
Extended Data Fig. 6 Pathways enrichment results for crest 1 and 2.
a , The final functional modules identified for Crest 1 and 2. b , The pathway enrichment analysis results for transcriptomics data. c , The pathway enrichment analysis results for proteomics data. d , The pathway enrichment results for metabolomics data.
Supplementary information
Supplementary figs. 1–6, reporting summary, supplementary data analysis results of the study., rights and permissions.
Open Access This article is licensed under a Creative Commons Attribution 4.0 International License, which permits use, sharing, adaptation, distribution and reproduction in any medium or format, as long as you give appropriate credit to the original author(s) and the source, provide a link to the Creative Commons licence, and indicate if changes were made. The images or other third party material in this article are included in the article’s Creative Commons licence, unless indicated otherwise in a credit line to the material. If material is not included in the article’s Creative Commons licence and your intended use is not permitted by statutory regulation or exceeds the permitted use, you will need to obtain permission directly from the copyright holder. To view a copy of this licence, visit http://creativecommons.org/licenses/by/4.0/ .
Reprints and permissions
About this article
Cite this article.
Shen, X., Wang, C., Zhou, X. et al. Nonlinear dynamics of multi-omics profiles during human aging. Nat Aging (2024). https://doi.org/10.1038/s43587-024-00692-2
Download citation
Received : 09 December 2023
Accepted : 22 July 2024
Published : 14 August 2024
DOI : https://doi.org/10.1038/s43587-024-00692-2
Share this article
Anyone you share the following link with will be able to read this content:
Sorry, a shareable link is not currently available for this article.
Provided by the Springer Nature SharedIt content-sharing initiative
Quick links
- Explore articles by subject
- Guide to authors
- Editorial policies
Sign up for the Nature Briefing newsletter — what matters in science, free to your inbox daily.

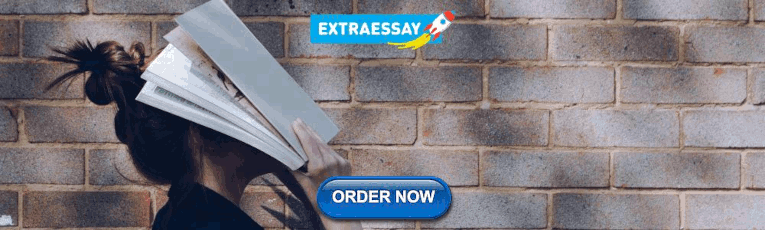
IMAGES
COMMENTS
Alcohol dehydrogenases. There are 6 closely related ADHs whose structure and enzymology have been studied; a seventh (ADH6) has not been found as a protein in vivo (Table 1) (Bosron et al., 1993, Hurley et al., 2002, Edenberg and Bosron, 2018).Their pattern of expression in tissues differs (Figure 2).ADH1A, ADH1B, and ADH1C are called class I ADHs; they are more than 90% identical in amino ...
The primary enzymes involved in alcohol metabolism are alcohol dehydrogenase (ADH) and aldehyde dehydrogenase (ALDH). Both enzymes occur in several forms that are encoded by different genes; moreover, there are variants (i.e., alleles) of some of these genes that encode enzymes with different characteristics and which have different ethnic distributions.
Abstract. Alcohol is eliminated from the body by various metabolic mechanisms. The primary enzymes involved are aldehyde dehydrogenase (ALDH), alcohol dehydrogenase (ADH), cytochrome P450 (CYP2E1), and catalase. Variations in the genes for these enzymes have been found to influence alcohol consumption, alcohol-related tissue damage, and alcohol ...
Alcohol Dehydrogenase. abacavir. Alcohol dehydrogenases (ADHs) are most known for their roles in oxidation and elimination of ethanol. Although less known, ADHs also play a critical role in the metabolism of a number of drugs and metabolites that contain alcohol functional groups, such as abacavir (HIV/AIDS), hydroxyzine (antihista ….
Alcohol dehydrogenases (ADHs) are most known for their roles in oxidation and elimination of ethanol. Although less known, ADHs also play a critical role in the metabolism of a number of drugs and metabolites that contain alcohol functional groups, such as abacavir (HIV/AIDS), hydroxyzine (antihistamine), and ethambutol (antituberculosis). ADHs consist of 7 gene family numbers and several ...
The genes that have the largest impact on alcohol consumption and Alcohol Use Disorders are alcohol dehydrogenase 1B (ADH1B) and aldehyde dehydrogenase 2 (ALDH2); both work by altering the rate of ethanol metabolism to at least transiently elevate acetaldehyde.Different functional variations are important in different populations.
A functional variant in alcohol dehydrogenase 1B (ADH1B) is protective in people of European and Asian descent, and a different functional variant in the same gene is protective in those of African descent. A strongly protective variant in aldehyde dehydrogenase 2 (ALDH2) is essentially only found in Asians. This highlights the need to study a ...
Yeast alcohol dehydrogenase (yADH) serves as an excellent model system for enzyme-catalyzed H transfer because unlike many other enzymes, the chemical step, oxidation of a primary alcohol to an aldehyde by NAD+, is rate-limiting with aromatic substrates (7). Furthermore, the thermodynamics of the reaction allow for examination of both the ...
Relative sizes and positions of the seven human alcohol dehydrogenase (ADH) genes on the long arm of chromosome 4 (i.e., chromosome 4q). They are shown in the direction in which the genes are ...
Summary. Alcohol dehydrogenases constitute a complex system of enzymes, classes, isozymes, and allelic variants. The zinc containing, well-known liver enzyme is a class I medium-chain alcohol dehydrogenase. Other classes of this family include the class II protein, the glutathione-dependent formaldehyde dehydrogenase (the class III enzyme), the ...
Alcohol dehydrogenases (ADHs) catalyze the reversible reduction of a carbonyl group to its corresponding alcohol. ADHs are widely employed for organic synthesis due to their lack of harm to the environment, broad substrate acceptance, and high enantioselectivity. This review focuses on the impact and relevance of ADH enantioselectivities on their biotechnological application. Stereoselective ...
It is becoming clear that noncoding variants in both ADH and ALDH genes also may influence alcohol metabolism and, consequently, alcoholism risk; the specific nature and effects of these variants still need further study. The primary enzymes involved in alcohol metabolism are alcohol dehydrogenase (ADH) and aldehyde dehydrogenase (ALDH). Both enzymes occur in several forms that are encoded by ...
A highly instructive, wide-ranging laboratory project in which students study the effects of various parameters on the enzymatic activity of alcohol dehydrogenase has been adapted for the upper-division biochemistry and physical biochemistry laboratory. Our two main goals were to provide enhanced data analysis, featuring nonlinear regression, and also to give students experience in ...
The alcohol dehydrogenase 1 (EC 1.1.1.1) genes encode oxidoreductases that catalyze the interconversion between alcohols and aldehydes with the reduction of NAD + to NADH. ADHs are broadly distributed in both eukaryotic and prokaryotic kingdoms and play important roles in the metabolism of steroids, xenobiotic alcohols and aldehydes [1] , [2 ...
This review focuses upon the regulation of the three human class I alcohol dehydrogenase genes, ADH1,ADH2 and ADH3, which are expressed at high levels in liver, and at different levels in other tissues. This review focuses upon the regulation of the three human class I alcohol dehydrogenase genes, ADH1, ADH2 and ADH3. These closely related genes are expressed at high levels in liver, and at ...
Yeast (Saccharomyces cerevisiae) alcohol dehydrogenase I (ADH1) is the constitutive enzyme that reduces acetaldehyde to ethanol during the fermentation of glucose. ADH1 is a homotetramer of subunits with 347 amino acid residues. A structure for ADH1 was determined by X-ray crystallography at 2.4 Å resolution. The asymmetric unit contains four different subunits, arranged as similar dimers ...
In the present paper, we review current data on the role of acetaldehyde and ethanol metabolism in alcohol consumption and abuse. ... Whereas alcohol dehydrogenase metabolizes the bulk of ethanol ...
The serum level ADH I in ICP patients was 2.79 mU/l. While the serum level of ADH I in the normal control group was 1.72 mU/l. Compared with the control group, ADH I were remarkablely (about 62% ...
In fact, drugs such as disulfiram which inhibit aldehyde dehydrogenase (ALDH), and cause acetaldehyde accumulation if alcohol is consumed, are used as a treatment for alcoholism by causing ...
Yeast alcohol dehydrogenase (EC1.1.1.1) is able to catalyze the oxidation of acetaldehyde by NAD+ with a concomitant formation of ethanol, at pH8.8 and pH7.1; the stoichiometry of aldehyde ...
1. Introduction. Hangover or veisalgia is the term that refers to the psychological and physiological effects following alcohol consumption. It is characterized by an unpleasant and uneasy feeling that includes, but is not limited to, headache, fatigue, drowsiness, nausea, and in some cases, vomiting (Swift and Davidson, 1998).The symptoms set in when the concentration of ethanol in the blood ...
However, we also found higher risk for all-cause and cancer deaths in moderate-risk drinkers, unlike most previous research, which has reported protective associations of low to moderate alcohol consumption, mainly for all-cause 2-4,36 and CVD 3,36,37 mortality, ischemic heart disease, 3,6,34 and diabetes, 6 or null associations with all-cause ...
Identifying and studying these factors should be a priority for future research," Shen said. Changes may influence health and disease risk. In people in their 40s, significant changes were seen in the number of molecules related to alcohol, caffeine and lipid metabolism; cardiovascular disease; and skin and muscle.
This study analyzed the effect of certain common food commodities on hangover through in vitro studies by enhancing either alcohol dehydrogenase and aldehyde dehydrogenase activities, or at least the aldehyde dehydrogenase activity, to formulate an anti-hangover product. A beverage made from a blend of sweet lime, pear, and coconut water could ...
Overall, this research demonstrates that functions and risks of aging-related diseases change nonlinearly across the human lifespan and provides insights into the molecular and biological pathways ...