Thank you for visiting nature.com. You are using a browser version with limited support for CSS. To obtain the best experience, we recommend you use a more up to date browser (or turn off compatibility mode in Internet Explorer). In the meantime, to ensure continued support, we are displaying the site without styles and JavaScript.
- View all journals
- Explore content
- About the journal
- Publish with us
- Sign up for alerts
- Published: 29 May 2014
Points of significance
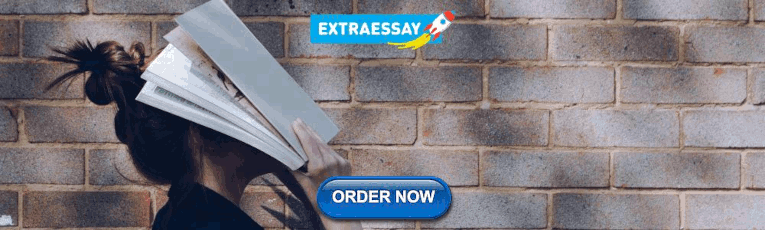
Designing comparative experiments
- Martin Krzywinski 1 &
- Naomi Altman 2
Nature Methods volume 11 , pages 597–598 ( 2014 ) Cite this article
49k Accesses
14 Citations
8 Altmetric
Metrics details
- Research data
- Statistical methods
Good experimental designs limit the impact of variability and reduce sample-size requirements.
You have full access to this article via your institution.
In a typical experiment, the effect of different conditions on a biological system is compared. Experimental design is used to identify data-collection schemes that achieve sensitivity and specificity requirements despite biological and technical variability, while keeping time and resource costs low. In the next series of columns we will use statistical concepts introduced so far and discuss design, analysis and reporting in common experimental scenarios.
In experimental design, the researcher-controlled independent variables whose effects are being studied (e.g., growth medium, drug and exposure to light) are called factors. A level is a subdivision of the factor and measures the type (if categorical) or amount (if continuous) of the factor. The goal of the design is to determine the effect and interplay of the factors on the response variable (e.g., cell size). An experiment that considers all combinations of N factors, each with n i levels, is a factorial design of type n 1 × n 2 × ... × n N . For example, a 3 × 4 design has two factors with three and four levels each and examines all 12 combinations of factor levels. We will review statistical methods in the context of a simple experiment to introduce concepts that apply to more complex designs.
Suppose that we wish to measure the cellular response to two different treatments, A and B, measured by fluorescence of an aliquot of cells. This is a single factor (treatment) design with three levels (untreated, A and B). We will assume that the fluorescence (in arbitrary units) of an aliquot of untreated cells has a normal distribution with μ = 10 and that real effect sizes of treatments A and B are d A = 0.6 and d B = 1 (A increases response by 6% to 10.6 and B by 10% to 11). To simulate variability owing to biological variation and measurement uncertainty (e.g., in the number of cells in an aliquot), we will use σ = 1 for the distributions. For all tests and calculations we use α = 0.05.
We start by assigning samples of cell aliquots to each level ( Fig. 1a ). To improve the precision (and power) in measuring the mean of the response, more than one aliquot is needed 1 . One sample will be a control (considered a level) to establish the baseline response, and capture biological and technical variability. The other two samples will be used to measure response to each treatment. Before we can carry out the experiment, we need to decide on the sample size.
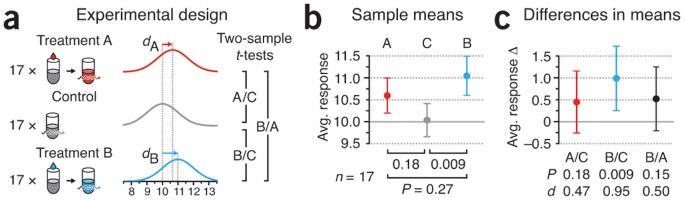
( a ) Two treated samples (A and B) with n = 17 are compared to a control (C) with n = 17 and to each other using two-sample t -tests. ( b ) Simulated means and P values for samples in a . Values are drawn from normal populations with σ = 1 and mean response of 10 (C), 10.6 (A) and 11 (B). ( c ) The preferred reporting method of results shown in b , illustrating difference in means with CIs, P values and effect size, d . All error bars show 95% CI.
We can fall back to our discussion about power 1 to suggest n . How large an effect size ( d ) do we wish to detect and at what sensitivity? Arbitrarily small effects can be detected with large enough sample size, but this makes for a very expensive experiment. We will need to balance our decision based on what we consider to be a biologically meaningful response and the resources at our disposal. If we are satisfied with an 80% chance (the lowest power we should accept) of detecting a 10% change in response, which corresponds to the real effect of treatment B ( d B = 1), the two-sample t -test requires n = 17. At this n value, the power to detect d A = 0.6 is 40%. Power calculations are easily computed with software; typically inputs are the difference in means (Δ μ ), standard deviation estimate ( σ ), α and the number of tails (we recommend always using two-tailed calculations).
Based on the design in Figure 1a , we show the simulated samples means and their 95% confidence interval (CI) in Figure 1b . The 95% CI captures the mean of the population 95% of the time; we recommend using it to report precision. Our results show a significant difference between B and control (referred to as B/C, P = 0.009) but not for A/C ( P = 0.18). Paradoxically, testing B/A does not return a significant outcome ( P = 0.15). Whenever we perform more than one test we should adjust the P values 2 . As we only have three tests, the adjusted B/C P value is still significant, P ′ = 3 P = 0.028. Although commonly used, the format used in Figure 1b is inappropriate for reporting our results: sample means, their uncertainty and P values alone do not present the full picture.
A more complete presentation of the results ( Fig. 1c ) combines the magnitude with uncertainty (as CI) in the difference in means. The effect size, d , defined as the difference in means in units of pooled standard deviation, expresses this combination of measurement and precision in a single value. Data in Figure 1c also explain better that the difference between a significant result (B/C, P = 0.009) and a nonsignificant result (A/C, P = 0.18) is not always significant (B/A, P = 0.15) 3 . Significance itself is a hard boundary at P = α , and two arbitrarily close results may straddle it. Thus, neither significance itself nor differences in significance status should ever be used to conclude anything about the magnitude of the underlying differences, which may be very small and not biologically relevant.
CIs explicitly show how close we are to making a positive inference and help assess the benefit of collecting more data. For example, the CIs of A/C and B/C closely overlap, which suggests that at our sample size we cannot reliably distinguish between the response to A and B ( Fig. 1c ). Furthermore, given that the CI of A/C just barely crosses zero, it is possible that A has a real effect that our test failed to detect. More information about our ability to detect an effect can be obtained from a post hoc power analysis, which assumes that the observed effect is the same as the real effect (normally unknown), and uses the observed difference in means and pooled variance. For A/C, the difference in means is 0.48 and the pooled s.d. ( s p ) = 1.03, which yields a post hoc power of 27%; we have little power to detect this difference. Other than increasing sample size, how could we improve our chances of detecting the effect of A?
Our ability to detect the effect of A is limited by variability in the difference between A and C, which has two random components. If we measure the same aliquot twice, we expect variability owing to technical variation inherent in our laboratory equipment and variability of the sample over time ( Fig. 2a ). This is called within-subject variation, σ wit . If we measure two different aliquots with the same factor level, we also expect biological variation, called between-subject variation, σ bet , in addition to the technical variation ( Fig. 2b ). Typically there is more biological than technical variability ( σ bet > σ wit ). In an unpaired design, the use of different aliquots adds both σ wit and σ bet to the measured difference ( Fig. 2c ). In a paired design, which uses the paired t -test 4 , the same aliquot is used and the impact of biological variation ( σ bet ) is mitigated ( Fig. 2c ). If differences in aliquots ( σ bet ) are appreciable, variance is markedly reduced (to within-subject variation) and the paired test has higher power.
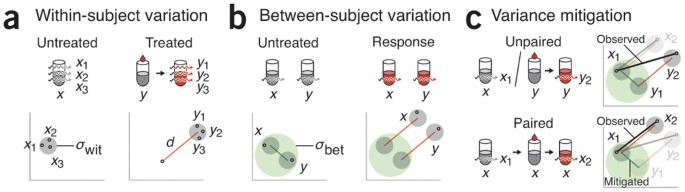
( a ) Limits of measurement and technical precision contribute to σ wit (gray circle) observed when the same aliquot is measured more than once. This variability is assumed to be the same in the untreated and treated condition, with effect d on aliquot x and y . ( b ) Biological variation gives rise to σ bet (green circle). ( c ) Paired design uses the same aliquot for both measurements, mitigating between-subject variation.
The link between σ bet and σ wit can be illustrated by an experiment to evaluate a weight-loss diet in which a control group eats normally and a treatment group follows the diet. A comparison of the mean weight after a month is confounded by the initial weights of the subjects in each group. If instead we focus on the change in weight, we remove much of the subject variability owing to the initial weight.
If we write the total variance as σ 2 = σ wit 2 + σ bet 2 , then the variance of the observed quantity in Figure 2c is 2 σ 2 for the unpaired design but 2 σ 2 (1 – ρ ) for the paired design, where ρ = σ bet 2 / σ 2 is the correlation coefficient (intraclass correlation). The relative difference is captured by ρ of two measurements on the same aliquot, which must be included because the measurements are no longer independent. If we ignore ρ in our analysis, we will overestimate the variance and obtain overly conservative P values and CIs. In the case where there is no additional variation between aliquots, there is no benefit to using the same aliquot: measurements on the same aliquot are uncorrelated ( ρ = 0) and variance of the paired test is the same as the variance of the unpaired. In contrast, if there is no variation in measurements on the same aliquot except for the treatment effect ( σ wit = 0), we have perfect correlation ( ρ = 1). Now, the difference measurement derived from the same aliquot removes all the noise; in fact, a single pair of aliquots suffices for an exact inference. Practically, both sources of variation are present, and it is their relative size—reflected in ρ —that determines the benefit of using the paired t-test.
We can see the improved sensitivity of the paired design ( Fig. 3a ) in decreased P values for the effects of A and B ( Fig. 3b versus Fig. 1b ). With the between-subject variance mitigated, we now detect an effect for A ( P = 0.013) and an even lower P value for B ( P = 0.0002) ( Fig. 3b ). Testing the difference between ΔA and ΔB requires the two-sample t -test because we are testing different aliquots, and this still does not produce a significant result ( P = 0.18). When reporting paired-test results, sample means ( Fig. 3b ) should never be shown; instead, the mean difference and confidence interval should be shown ( Fig. 3c ). The reason for this comes from our discussion above: the benefit of pairing comes from reduced variance because ρ > 0, something that cannot be gleaned from Figure 3b . We illustrate this in Figure 3c with two different sample simulations with same sample mean and variance but different correlation, achieved by changing the relative amount of σ bet 2 and σ wit 2 . When the component of biological variance is increased, ρ is increased from 0.5 to 0.8, total variance in difference in means drops and the test becomes more sensitive, reflected by the narrower CIs. We are now more certain that A has a real effect and have more reason to believe that the effects of A and B are different, evidenced by the lower P value for ΔB/ΔA from the two-sample t -test (0.06 versus 0.18; Fig. 3c ). As before, P values should be adjusted with multiple-test correction.
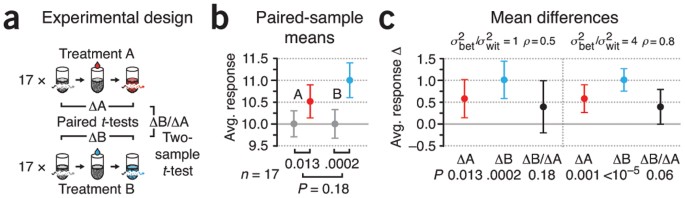
( a ) The same n = 17 sample is used to measure the difference between treatment and background (ΔA = A after − A before , ΔB = B after − B before ), analyzed with the paired t -test. Two-sample t -test is used to compare the difference between responses (ΔB versus ΔA). ( b ) Simulated sample means and P values for measurements and comparisons in a . ( c ) Mean difference, CIs and P values for two variance scenarios, σ bet 2 / σ wit 2 of 1 and 4, corresponding to ρ of 0.5 and 0.8. Total variance was fixed: σ bet 2 + σ wit 2 = 1. All error bars show 95% CI.
The paired design is a more efficient experiment. Fewer aliquots are needed: 34 instead of 51, although now 68 fluorescence measurements need to be taken instead of 51. If we assume σ wit = σ bet ( ρ = 0.5; Fig. 3c ), we can expect the paired design to have a power of 97%. This power increase is highly contingent on the value of ρ . If σ wit is appreciably larger than σ bet (i.e., ρ is small), the power of the paired test can be lower than for the two-sample variant. This is because total variance remains relatively unchanged (2 σ 2 (1 – ρ ) ≈ 2 σ 2 ) while the critical value of the test statistic can be markedly larger (particularly for small samples) because the number of degrees of freedom is now n – 1 instead of 2( n – 1). If the ratio of σ bet 2 to σ wit 2 is 1:4 ( ρ = 0.2), the paired test power drops from 97% to 86%.
To analyze experimental designs that have more than two levels, or additional factors, a method called analysis of variance is used. This generalizes the t -test for comparing three or more levels while maintaining better power than comparing all sets of two levels. Experiments with two or more levels will be our next topic.
Krzywinski, M.I. & Altman, N. Nat. Methods 10 , 1139–1140 (2013).
Article CAS Google Scholar
Krzywinski, M.I. & Altman, N. Nat. Methods 11 , 355–356 (2014).
Gelman, A. & Stern, H. Am. Stat. 60 , 328–331 (2006).
Article Google Scholar
Krzywinski, M.I. & Altman, N. Nat. Methods 11 , 215–216 (2014).
Download references
Author information
Authors and affiliations.
Martin Krzywinski is a staff scientist at Canada's Michael Smith Genome Sciences Centre.,
- Martin Krzywinski
Naomi Altman is a Professor of Statistics at The Pennsylvania State University.,
- Naomi Altman
You can also search for this author in PubMed Google Scholar
Ethics declarations
Competing interests.
The authors declare no competing financial interests.
Rights and permissions
Reprints and permissions
About this article
Cite this article.
Krzywinski, M., Altman, N. Designing comparative experiments. Nat Methods 11 , 597–598 (2014). https://doi.org/10.1038/nmeth.2974
Download citation
Published : 29 May 2014
Issue Date : June 2014
DOI : https://doi.org/10.1038/nmeth.2974
Share this article
Anyone you share the following link with will be able to read this content:
Sorry, a shareable link is not currently available for this article.
Provided by the Springer Nature SharedIt content-sharing initiative
This article is cited by
Sources of variation.
Nature Methods (2015)
ETD Outperforms CID and HCD in the Analysis of the Ubiquitylated Proteome
- Tanya R. Porras-Yakushi
- Michael J. Sweredoski
Journal of the American Society for Mass Spectrometry (2015)
Analysis of variance and blocking
Nature Methods (2014)
Nested designs
- Paul Blainey
Quick links
- Explore articles by subject
- Guide to authors
- Editorial policies
Sign up for the Nature Briefing newsletter — what matters in science, free to your inbox daily.

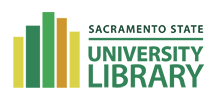
- My Library Account
- Articles, Books & More
- Course Reserves
- Site Search
- Advanced Search
- Sac State Library
- Research Guides
Research Methods Simplified
Comparative method/quasi-experimental.
- Quantitative Research
- Qualitative Research
- Primary, Seconday and Tertiary Research and Resources
- Definitions
- Sources Consulted
Comparative method or quasi-experimental ---a method used to describe similarities and differences in variables in two or more groups in a natural setting, that is, it resembles an experiment as it uses manipulation but lacks random assignment of individual subjects. Instead it uses existing groups. For examples see http://www.education.com/reference/article/quasiexperimental-research/#B
- << Previous: Qualitative Research
- Next: Primary, Seconday and Tertiary Research and Resources >>
- Last Updated: Jul 3, 2024 2:35 PM
- URL: https://csus.libguides.com/res-meth

An official website of the United States government
The .gov means it’s official. Federal government websites often end in .gov or .mil. Before sharing sensitive information, make sure you’re on a federal government site.
The site is secure. The https:// ensures that you are connecting to the official website and that any information you provide is encrypted and transmitted securely.
- Publications
- Account settings
The PMC website is updating on October 15, 2024. Learn More or Try it out now .
- Advanced Search
- Journal List
- Perspect Clin Res
- v.9(4); Oct-Dec 2018
Study designs: Part 1 – An overview and classification
Priya ranganathan.
Department of Anaesthesiology, Tata Memorial Centre, Mumbai, Maharashtra, India
Rakesh Aggarwal
1 Department of Gastroenterology, Sanjay Gandhi Postgraduate Institute of Medical Sciences, Lucknow, Uttar Pradesh, India
There are several types of research study designs, each with its inherent strengths and flaws. The study design used to answer a particular research question depends on the nature of the question and the availability of resources. In this article, which is the first part of a series on “study designs,” we provide an overview of research study designs and their classification. The subsequent articles will focus on individual designs.
INTRODUCTION
Research study design is a framework, or the set of methods and procedures used to collect and analyze data on variables specified in a particular research problem.
Research study designs are of many types, each with its advantages and limitations. The type of study design used to answer a particular research question is determined by the nature of question, the goal of research, and the availability of resources. Since the design of a study can affect the validity of its results, it is important to understand the different types of study designs and their strengths and limitations.
There are some terms that are used frequently while classifying study designs which are described in the following sections.
A variable represents a measurable attribute that varies across study units, for example, individual participants in a study, or at times even when measured in an individual person over time. Some examples of variables include age, sex, weight, height, health status, alive/dead, diseased/healthy, annual income, smoking yes/no, and treated/untreated.
Exposure (or intervention) and outcome variables
A large proportion of research studies assess the relationship between two variables. Here, the question is whether one variable is associated with or responsible for change in the value of the other variable. Exposure (or intervention) refers to the risk factor whose effect is being studied. It is also referred to as the independent or the predictor variable. The outcome (or predicted or dependent) variable develops as a consequence of the exposure (or intervention). Typically, the term “exposure” is used when the “causative” variable is naturally determined (as in observational studies – examples include age, sex, smoking, and educational status), and the term “intervention” is preferred where the researcher assigns some or all participants to receive a particular treatment for the purpose of the study (experimental studies – e.g., administration of a drug). If a drug had been started in some individuals but not in the others, before the study started, this counts as exposure, and not as intervention – since the drug was not started specifically for the study.
Observational versus interventional (or experimental) studies
Observational studies are those where the researcher is documenting a naturally occurring relationship between the exposure and the outcome that he/she is studying. The researcher does not do any active intervention in any individual, and the exposure has already been decided naturally or by some other factor. For example, looking at the incidence of lung cancer in smokers versus nonsmokers, or comparing the antenatal dietary habits of mothers with normal and low-birth babies. In these studies, the investigator did not play any role in determining the smoking or dietary habit in individuals.
For an exposure to determine the outcome, it must precede the latter. Any variable that occurs simultaneously with or following the outcome cannot be causative, and hence is not considered as an “exposure.”
Observational studies can be either descriptive (nonanalytical) or analytical (inferential) – this is discussed later in this article.
Interventional studies are experiments where the researcher actively performs an intervention in some or all members of a group of participants. This intervention could take many forms – for example, administration of a drug or vaccine, performance of a diagnostic or therapeutic procedure, and introduction of an educational tool. For example, a study could randomly assign persons to receive aspirin or placebo for a specific duration and assess the effect on the risk of developing cerebrovascular events.
Descriptive versus analytical studies
Descriptive (or nonanalytical) studies, as the name suggests, merely try to describe the data on one or more characteristics of a group of individuals. These do not try to answer questions or establish relationships between variables. Examples of descriptive studies include case reports, case series, and cross-sectional surveys (please note that cross-sectional surveys may be analytical studies as well – this will be discussed in the next article in this series). Examples of descriptive studies include a survey of dietary habits among pregnant women or a case series of patients with an unusual reaction to a drug.
Analytical studies attempt to test a hypothesis and establish causal relationships between variables. In these studies, the researcher assesses the effect of an exposure (or intervention) on an outcome. As described earlier, analytical studies can be observational (if the exposure is naturally determined) or interventional (if the researcher actively administers the intervention).
Directionality of study designs
Based on the direction of inquiry, study designs may be classified as forward-direction or backward-direction. In forward-direction studies, the researcher starts with determining the exposure to a risk factor and then assesses whether the outcome occurs at a future time point. This design is known as a cohort study. For example, a researcher can follow a group of smokers and a group of nonsmokers to determine the incidence of lung cancer in each. In backward-direction studies, the researcher begins by determining whether the outcome is present (cases vs. noncases [also called controls]) and then traces the presence of prior exposure to a risk factor. These are known as case–control studies. For example, a researcher identifies a group of normal-weight babies and a group of low-birth weight babies and then asks the mothers about their dietary habits during the index pregnancy.
Prospective versus retrospective study designs
The terms “prospective” and “retrospective” refer to the timing of the research in relation to the development of the outcome. In retrospective studies, the outcome of interest has already occurred (or not occurred – e.g., in controls) in each individual by the time s/he is enrolled, and the data are collected either from records or by asking participants to recall exposures. There is no follow-up of participants. By contrast, in prospective studies, the outcome (and sometimes even the exposure or intervention) has not occurred when the study starts and participants are followed up over a period of time to determine the occurrence of outcomes. Typically, most cohort studies are prospective studies (though there may be retrospective cohorts), whereas case–control studies are retrospective studies. An interventional study has to be, by definition, a prospective study since the investigator determines the exposure for each study participant and then follows them to observe outcomes.
The terms “prospective” versus “retrospective” studies can be confusing. Let us think of an investigator who starts a case–control study. To him/her, the process of enrolling cases and controls over a period of several months appears prospective. Hence, the use of these terms is best avoided. Or, at the very least, one must be clear that the terms relate to work flow for each individual study participant, and not to the study as a whole.
Classification of study designs
Figure 1 depicts a simple classification of research study designs. The Centre for Evidence-based Medicine has put forward a useful three-point algorithm which can help determine the design of a research study from its methods section:[ 1 ]

Classification of research study designs
- Does the study describe the characteristics of a sample or does it attempt to analyze (or draw inferences about) the relationship between two variables? – If no, then it is a descriptive study, and if yes, it is an analytical (inferential) study
- If analytical, did the investigator determine the exposure? – If no, it is an observational study, and if yes, it is an experimental study
- If observational, when was the outcome determined? – at the start of the study (case–control study), at the end of a period of follow-up (cohort study), or simultaneously (cross sectional).
In the next few pieces in the series, we will discuss various study designs in greater detail.
Financial support and sponsorship
Conflicts of interest.
There are no conflicts of interest.
Have a language expert improve your writing
Run a free plagiarism check in 10 minutes, generate accurate citations for free.
- Knowledge Base
Methodology
- Types of Research Designs Compared | Guide & Examples
Types of Research Designs Compared | Guide & Examples
Published on June 20, 2019 by Shona McCombes . Revised on June 22, 2023.
When you start planning a research project, developing research questions and creating a research design , you will have to make various decisions about the type of research you want to do.
There are many ways to categorize different types of research. The words you use to describe your research depend on your discipline and field. In general, though, the form your research design takes will be shaped by:
- The type of knowledge you aim to produce
- The type of data you will collect and analyze
- The sampling methods , timescale and location of the research
This article takes a look at some common distinctions made between different types of research and outlines the key differences between them.
Table of contents
Types of research aims, types of research data, types of sampling, timescale, and location, other interesting articles.
The first thing to consider is what kind of knowledge your research aims to contribute.
Type of research | What’s the difference? | What to consider |
---|---|---|
Basic vs. applied | Basic research aims to , while applied research aims to . | Do you want to expand scientific understanding or solve a practical problem? |
vs. | Exploratory research aims to , while explanatory research aims to . | How much is already known about your research problem? Are you conducting initial research on a newly-identified issue, or seeking precise conclusions about an established issue? |
aims to , while aims to . | Is there already some theory on your research problem that you can use to develop , or do you want to propose new theories based on your findings? |
Receive feedback on language, structure, and formatting
Professional editors proofread and edit your paper by focusing on:
- Academic style
- Vague sentences
- Style consistency
See an example
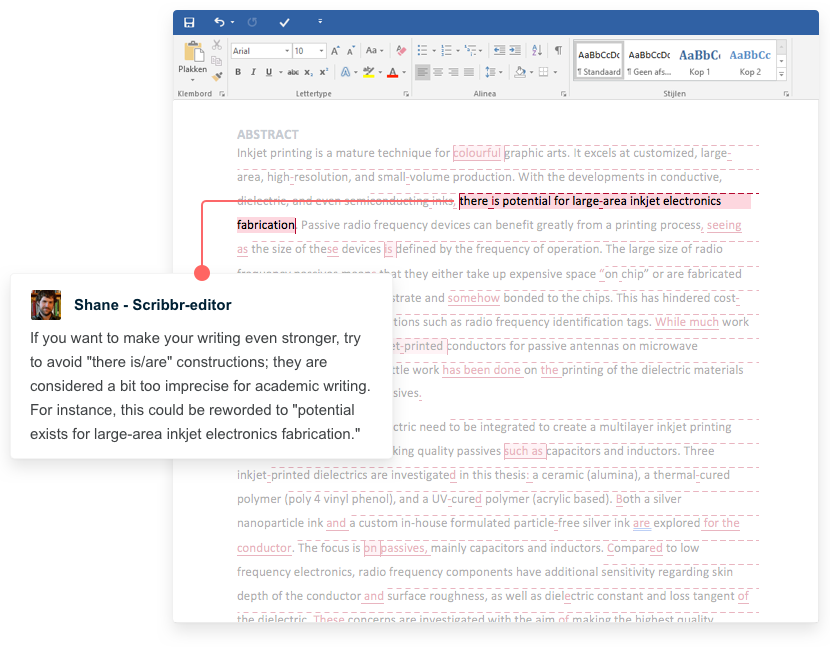
The next thing to consider is what type of data you will collect. Each kind of data is associated with a range of specific research methods and procedures.
Type of research | What’s the difference? | What to consider |
---|---|---|
Primary research vs secondary research | Primary data is (e.g., through or ), while secondary data (e.g., in government or scientific publications). | How much data is already available on your topic? Do you want to collect original data or analyze existing data (e.g., through a )? |
, while . | Is your research more concerned with measuring something or interpreting something? You can also create a research design that has elements of both. | |
vs | Descriptive research gathers data , while experimental research . | Do you want to identify characteristics, patterns and or test causal relationships between ? |
Finally, you have to consider three closely related questions: how will you select the subjects or participants of the research? When and how often will you collect data from your subjects? And where will the research take place?
Keep in mind that the methods that you choose bring with them different risk factors and types of research bias . Biases aren’t completely avoidable, but can heavily impact the validity and reliability of your findings if left unchecked.
Type of research | What’s the difference? | What to consider |
---|---|---|
allows you to , while allows you to draw conclusions . | Do you want to produce knowledge that applies to many contexts or detailed knowledge about a specific context (e.g. in a )? | |
vs | Cross-sectional studies , while longitudinal studies . | Is your research question focused on understanding the current situation or tracking changes over time? |
Field research vs laboratory research | Field research takes place in , while laboratory research takes place in . | Do you want to find out how something occurs in the real world or draw firm conclusions about cause and effect? Laboratory experiments have higher but lower . |
Fixed design vs flexible design | In a fixed research design the subjects, timescale and location are begins, while in a flexible design these aspects may . | Do you want to test hypotheses and establish generalizable facts, or explore concepts and develop understanding? For measuring, testing and making generalizations, a fixed research design has higher . |
Choosing between all these different research types is part of the process of creating your research design , which determines exactly how your research will be conducted. But the type of research is only the first step: next, you have to make more concrete decisions about your research methods and the details of the study.
Read more about creating a research design
If you want to know more about statistics , methodology , or research bias , make sure to check out some of our other articles with explanations and examples.
- Normal distribution
- Degrees of freedom
- Null hypothesis
- Discourse analysis
- Control groups
- Mixed methods research
- Non-probability sampling
- Quantitative research
- Ecological validity
Research bias
- Rosenthal effect
- Implicit bias
- Cognitive bias
- Selection bias
- Negativity bias
- Status quo bias
Cite this Scribbr article
If you want to cite this source, you can copy and paste the citation or click the “Cite this Scribbr article” button to automatically add the citation to our free Citation Generator.
McCombes, S. (2023, June 22). Types of Research Designs Compared | Guide & Examples. Scribbr. Retrieved September 23, 2024, from https://www.scribbr.com/methodology/types-of-research/
Is this article helpful?
Shona McCombes
Other students also liked, what is a research design | types, guide & examples, qualitative vs. quantitative research | differences, examples & methods, what is a research methodology | steps & tips, get unlimited documents corrected.
✔ Free APA citation check included ✔ Unlimited document corrections ✔ Specialized in correcting academic texts
Demystifying the research process: understanding a descriptive comparative research design
- Pediatric Nursing 37(4):188-9
- 37(4):188-9

- Villanova University
Discover the world's research
- 25+ million members
- 160+ million publication pages
- 2.3+ billion citations
No full-text available

To read the full-text of this research, you can request a copy directly from the author.

- Karen Joy A. Masepequeña
- Jay Ric L. Pangalaya

- Perzeus Lhey D. Villahermosa
- July M. Villaren
- Alberto Sarte
- Jennisa E Baroro

- Liezel Maravilla

- ( Corresponding
- Ali 1 Bylieva

- Rocelyn A Camino
- Rosalie N Darunday
- Honey Claire B Gallando
- Zainal Abidin

- Univers Access Inform Soc

- Kawther Sacor I. Abdulhalim

- Hong-va Leong

- Ellenie Jean Poliquit
- Jackelyn Pinlac
- Hazel Ligaray

- Lemuel Perez

- Mary Kris Maranga
- Novie Jane Maranga
- Charlene Mae Tautu-An

- Bliss Ann Marie V. Nene
- Kenn Carlo A. Delao
- , Abeguel M. Vargas

- Arlene A. Matawaran
- Elvin B. Rodriguez
- Recruit researchers
- Join for free
- Login Email Tip: Most researchers use their institutional email address as their ResearchGate login Password Forgot password? Keep me logged in Log in or Continue with Google Welcome back! Please log in. Email · Hint Tip: Most researchers use their institutional email address as their ResearchGate login Password Forgot password? Keep me logged in Log in or Continue with Google No account? Sign up

Experimental Research Design — 6 mistakes you should never make!
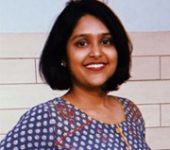
Since school days’ students perform scientific experiments that provide results that define and prove the laws and theorems in science. These experiments are laid on a strong foundation of experimental research designs.
An experimental research design helps researchers execute their research objectives with more clarity and transparency.
In this article, we will not only discuss the key aspects of experimental research designs but also the issues to avoid and problems to resolve while designing your research study.
Table of Contents
What Is Experimental Research Design?
Experimental research design is a framework of protocols and procedures created to conduct experimental research with a scientific approach using two sets of variables. Herein, the first set of variables acts as a constant, used to measure the differences of the second set. The best example of experimental research methods is quantitative research .
Experimental research helps a researcher gather the necessary data for making better research decisions and determining the facts of a research study.
When Can a Researcher Conduct Experimental Research?
A researcher can conduct experimental research in the following situations —
- When time is an important factor in establishing a relationship between the cause and effect.
- When there is an invariable or never-changing behavior between the cause and effect.
- Finally, when the researcher wishes to understand the importance of the cause and effect.
Importance of Experimental Research Design
To publish significant results, choosing a quality research design forms the foundation to build the research study. Moreover, effective research design helps establish quality decision-making procedures, structures the research to lead to easier data analysis, and addresses the main research question. Therefore, it is essential to cater undivided attention and time to create an experimental research design before beginning the practical experiment.
By creating a research design, a researcher is also giving oneself time to organize the research, set up relevant boundaries for the study, and increase the reliability of the results. Through all these efforts, one could also avoid inconclusive results. If any part of the research design is flawed, it will reflect on the quality of the results derived.
Types of Experimental Research Designs
Based on the methods used to collect data in experimental studies, the experimental research designs are of three primary types:
1. Pre-experimental Research Design
A research study could conduct pre-experimental research design when a group or many groups are under observation after implementing factors of cause and effect of the research. The pre-experimental design will help researchers understand whether further investigation is necessary for the groups under observation.
Pre-experimental research is of three types —
- One-shot Case Study Research Design
- One-group Pretest-posttest Research Design
- Static-group Comparison
2. True Experimental Research Design
A true experimental research design relies on statistical analysis to prove or disprove a researcher’s hypothesis. It is one of the most accurate forms of research because it provides specific scientific evidence. Furthermore, out of all the types of experimental designs, only a true experimental design can establish a cause-effect relationship within a group. However, in a true experiment, a researcher must satisfy these three factors —
- There is a control group that is not subjected to changes and an experimental group that will experience the changed variables
- A variable that can be manipulated by the researcher
- Random distribution of the variables
This type of experimental research is commonly observed in the physical sciences.
3. Quasi-experimental Research Design
The word “Quasi” means similarity. A quasi-experimental design is similar to a true experimental design. However, the difference between the two is the assignment of the control group. In this research design, an independent variable is manipulated, but the participants of a group are not randomly assigned. This type of research design is used in field settings where random assignment is either irrelevant or not required.
The classification of the research subjects, conditions, or groups determines the type of research design to be used.
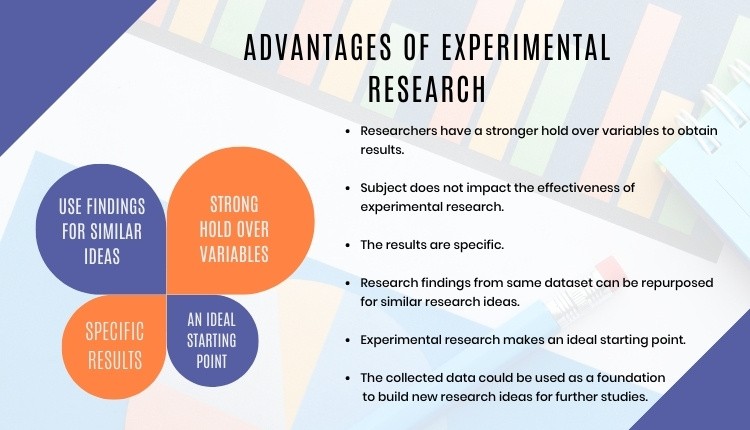
Advantages of Experimental Research
Experimental research allows you to test your idea in a controlled environment before taking the research to clinical trials. Moreover, it provides the best method to test your theory because of the following advantages:
- Researchers have firm control over variables to obtain results.
- The subject does not impact the effectiveness of experimental research. Anyone can implement it for research purposes.
- The results are specific.
- Post results analysis, research findings from the same dataset can be repurposed for similar research ideas.
- Researchers can identify the cause and effect of the hypothesis and further analyze this relationship to determine in-depth ideas.
- Experimental research makes an ideal starting point. The collected data could be used as a foundation to build new research ideas for further studies.
6 Mistakes to Avoid While Designing Your Research
There is no order to this list, and any one of these issues can seriously compromise the quality of your research. You could refer to the list as a checklist of what to avoid while designing your research.
1. Invalid Theoretical Framework
Usually, researchers miss out on checking if their hypothesis is logical to be tested. If your research design does not have basic assumptions or postulates, then it is fundamentally flawed and you need to rework on your research framework.
2. Inadequate Literature Study
Without a comprehensive research literature review , it is difficult to identify and fill the knowledge and information gaps. Furthermore, you need to clearly state how your research will contribute to the research field, either by adding value to the pertinent literature or challenging previous findings and assumptions.
3. Insufficient or Incorrect Statistical Analysis
Statistical results are one of the most trusted scientific evidence. The ultimate goal of a research experiment is to gain valid and sustainable evidence. Therefore, incorrect statistical analysis could affect the quality of any quantitative research.
4. Undefined Research Problem
This is one of the most basic aspects of research design. The research problem statement must be clear and to do that, you must set the framework for the development of research questions that address the core problems.
5. Research Limitations
Every study has some type of limitations . You should anticipate and incorporate those limitations into your conclusion, as well as the basic research design. Include a statement in your manuscript about any perceived limitations, and how you considered them while designing your experiment and drawing the conclusion.
6. Ethical Implications
The most important yet less talked about topic is the ethical issue. Your research design must include ways to minimize any risk for your participants and also address the research problem or question at hand. If you cannot manage the ethical norms along with your research study, your research objectives and validity could be questioned.
Experimental Research Design Example
In an experimental design, a researcher gathers plant samples and then randomly assigns half the samples to photosynthesize in sunlight and the other half to be kept in a dark box without sunlight, while controlling all the other variables (nutrients, water, soil, etc.)
By comparing their outcomes in biochemical tests, the researcher can confirm that the changes in the plants were due to the sunlight and not the other variables.
Experimental research is often the final form of a study conducted in the research process which is considered to provide conclusive and specific results. But it is not meant for every research. It involves a lot of resources, time, and money and is not easy to conduct, unless a foundation of research is built. Yet it is widely used in research institutes and commercial industries, for its most conclusive results in the scientific approach.
Have you worked on research designs? How was your experience creating an experimental design? What difficulties did you face? Do write to us or comment below and share your insights on experimental research designs!
Frequently Asked Questions
Randomization is important in an experimental research because it ensures unbiased results of the experiment. It also measures the cause-effect relationship on a particular group of interest.
Experimental research design lay the foundation of a research and structures the research to establish quality decision making process.
There are 3 types of experimental research designs. These are pre-experimental research design, true experimental research design, and quasi experimental research design.
The difference between an experimental and a quasi-experimental design are: 1. The assignment of the control group in quasi experimental research is non-random, unlike true experimental design, which is randomly assigned. 2. Experimental research group always has a control group; on the other hand, it may not be always present in quasi experimental research.
Experimental research establishes a cause-effect relationship by testing a theory or hypothesis using experimental groups or control variables. In contrast, descriptive research describes a study or a topic by defining the variables under it and answering the questions related to the same.

good and valuable
Very very good
Good presentation.
Rate this article Cancel Reply
Your email address will not be published.
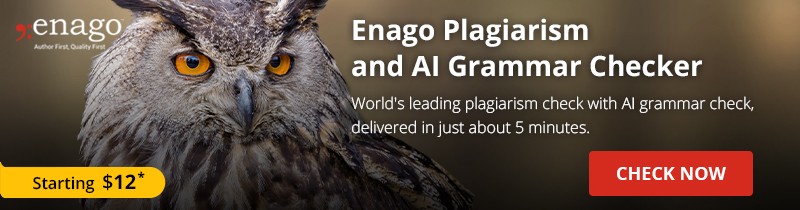
Enago Academy's Most Popular Articles
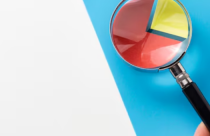
- Promoting Research
Graphical Abstracts Vs. Infographics: Best practices for using visual illustrations for increased research impact
Dr. Sarah Chen stared at her computer screen, her eyes staring at her recently published…
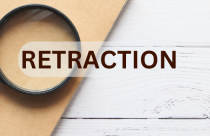
- Publishing Research
10 Tips to Prevent Research Papers From Being Retracted
Research paper retractions represent a critical event in the scientific community. When a published article…
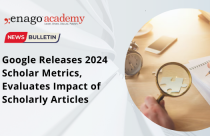
- Industry News
Google Releases 2024 Scholar Metrics, Evaluates Impact of Scholarly Articles
Google has released its 2024 Scholar Metrics, assessing scholarly articles from 2019 to 2023. This…
![comparative experimental research design What is Academic Integrity and How to Uphold it [FREE CHECKLIST]](https://www.enago.com/academy/wp-content/uploads/2024/05/FeatureImages-59-210x136.png)
Ensuring Academic Integrity and Transparency in Academic Research: A comprehensive checklist for researchers
Academic integrity is the foundation upon which the credibility and value of scientific findings are…
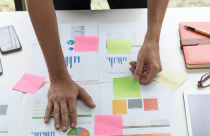
- Reporting Research
How to Optimize Your Research Process: A step-by-step guide
For researchers across disciplines, the path to uncovering novel findings and insights is often filled…
Choosing the Right Analytical Approach: Thematic analysis vs. content analysis for…
Comparing Cross Sectional and Longitudinal Studies: 5 steps for choosing the right…

Sign-up to read more
Subscribe for free to get unrestricted access to all our resources on research writing and academic publishing including:
- 2000+ blog articles
- 50+ Webinars
- 10+ Expert podcasts
- 50+ Infographics
- 10+ Checklists
- Research Guides
We hate spam too. We promise to protect your privacy and never spam you.
- AI in Academia
- Career Corner
- Diversity and Inclusion
- Infographics
- Expert Video Library
- Other Resources
- Enago Learn
- Upcoming & On-Demand Webinars
- Peer Review Week 2024
- Open Access Week 2023
- Conference Videos
- Enago Report
- Journal Finder
- Enago Plagiarism & AI Grammar Check
- Editing Services
- Publication Support Services
- Research Impact
- Translation Services
- Publication solutions
- AI-Based Solutions
- Thought Leadership
- Call for Articles
- Call for Speakers
- Author Training
- Edit Profile
I am looking for Editing/ Proofreading services for my manuscript Tentative date of next journal submission:
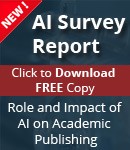
Which among these features would you prefer the most in a peer review assistant?

An official website of the United States government
The .gov means it’s official. Federal government websites often end in .gov or .mil. Before sharing sensitive information, make sure you’re on a federal government site.
The site is secure. The https:// ensures that you are connecting to the official website and that any information you provide is encrypted and transmitted securely.
- Publications
- Account settings
- My Bibliography
- Collections
- Citation manager
Save citation to file
Email citation, add to collections.
- Create a new collection
- Add to an existing collection
Add to My Bibliography
Your saved search, create a file for external citation management software, your rss feed.
- Search in PubMed
- Search in NLM Catalog
- Add to Search
How to choose your study design
Affiliation.
- 1 Department of Medicine, Sydney Medical School, Faculty of Medicine and Health, University of Sydney, Sydney, New South Wales, Australia.
- PMID: 32479703
- DOI: 10.1111/jpc.14929
Research designs are broadly divided into observational studies (i.e. cross-sectional; case-control and cohort studies) and experimental studies (randomised control trials, RCTs). Each design has a specific role, and each has both advantages and disadvantages. Moreover, while the typical RCT is a parallel group design, there are now many variants to consider. It is important that both researchers and paediatricians are aware of the role of each study design, their respective pros and cons, and the inherent risk of bias with each design. While there are numerous quantitative study designs available to researchers, the final choice is dictated by two key factors. First, by the specific research question. That is, if the question is one of 'prevalence' (disease burden) then the ideal is a cross-sectional study; if it is a question of 'harm' - a case-control study; prognosis - a cohort and therapy - a RCT. Second, by what resources are available to you. This includes budget, time, feasibility re-patient numbers and research expertise. All these factors will severely limit the choice. While paediatricians would like to see more RCTs, these require a huge amount of resources, and in many situations will be unethical (e.g. potentially harmful intervention) or impractical (e.g. rare diseases). This paper gives a brief overview of the common study types, and for those embarking on such studies you will need far more comprehensive, detailed sources of information.
Keywords: experimental studies; observational studies; research method.
© 2020 Paediatrics and Child Health Division (The Royal Australasian College of Physicians).
PubMed Disclaimer
Similar articles
- Observational Studies. Hess DR. Hess DR. Respir Care. 2023 Nov;68(11):1585-1597. doi: 10.4187/respcare.11170. Epub 2023 Jun 20. Respir Care. 2023. PMID: 37339891
- Observational designs in clinical multiple sclerosis research: Particulars, practices and potentialities. Jongen PJ. Jongen PJ. Mult Scler Relat Disord. 2019 Oct;35:142-149. doi: 10.1016/j.msard.2019.07.006. Epub 2019 Jul 20. Mult Scler Relat Disord. 2019. PMID: 31394404 Review.
- Study designs in clinical research. Noordzij M, Dekker FW, Zoccali C, Jager KJ. Noordzij M, et al. Nephron Clin Pract. 2009;113(3):c218-21. doi: 10.1159/000235610. Epub 2009 Aug 18. Nephron Clin Pract. 2009. PMID: 19690439 Review.
- Study Types in Orthopaedics Research: Is My Study Design Appropriate for the Research Question? Zaniletti I, Devick KL, Larson DR, Lewallen DG, Berry DJ, Maradit Kremers H. Zaniletti I, et al. J Arthroplasty. 2022 Oct;37(10):1939-1944. doi: 10.1016/j.arth.2022.05.028. Epub 2022 Sep 6. J Arthroplasty. 2022. PMID: 36162926 Free PMC article.
- Design choices for observational studies of the effect of exposure on disease incidence. Gail MH, Altman DG, Cadarette SM, Collins G, Evans SJ, Sekula P, Williamson E, Woodward M. Gail MH, et al. BMJ Open. 2019 Dec 9;9(12):e031031. doi: 10.1136/bmjopen-2019-031031. BMJ Open. 2019. PMID: 31822541 Free PMC article.
- Effects of Electronic Serious Games on Older Adults With Alzheimer's Disease and Mild Cognitive Impairment: Systematic Review With Meta-Analysis of Randomized Controlled Trials. Zuo X, Tang Y, Chen Y, Zhou Z. Zuo X, et al. JMIR Serious Games. 2024 Jul 31;12:e55785. doi: 10.2196/55785. JMIR Serious Games. 2024. PMID: 39083796 Free PMC article. Review.
- Nurses' Adherence to the Portuguese Standard to Prevent Catheter-Associated Urinary Tract Infections (CAUTIs): An Observational Study. Paiva-Santos F, Santos-Costa P, Bastos C, Graveto J. Paiva-Santos F, et al. Nurs Rep. 2023 Oct 10;13(4):1432-1441. doi: 10.3390/nursrep13040120. Nurs Rep. 2023. PMID: 37873827 Free PMC article.
- Effects of regional anaesthesia on mortality in patients undergoing lower extremity amputation: A retrospective pooled analysis. Quak SM, Pillay N, Wong SN, Karthekeyan RB, Chan DXH, Liu CWY. Quak SM, et al. Indian J Anaesth. 2022 Jun;66(6):419-430. doi: 10.4103/ija.ija_917_21. Epub 2022 Jun 21. Indian J Anaesth. 2022. PMID: 35903599 Free PMC article.
- Peat J, Mellis CM, Williams K, Xuan W. Health Science Research: A Handbook of Quantitative Methods Chapter 2, Planning the Study. Sydney: Allen & Unwin; 2001.
- Guyatt G, Rennie D, Meade MO, Cook DJ. Users Guide to the Medical Literature: A Manual for Evidence-Based Clinical Practice, 3rd edn; Chapter 14, Harm (observational studies). New York, NY: McGraw-Hill; 2015.
- Centre for Evidence Based Medicine. Oxford EBM ‘Critical Appraisal tools’. Oxford University, UK. Available from: cebm.net [Accessed March 2020].
- Kahlert J, Bjerge Gribsholt S, Gammelager H, Dekkers OMet al. Control of confounding in the analysis phase - An overview for clinicians. Clin. Epidemiol. 2017; 9: 195-204.
- Sedgwick P. Cross sectional studies: Advantages and disadvantages. BMJ 2014; 348: g2276.
- Search in MeSH
LinkOut - more resources
Full text sources.
- Ovid Technologies, Inc.
Miscellaneous
- NCI CPTAC Assay Portal
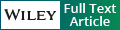
- Citation Manager
NCBI Literature Resources
MeSH PMC Bookshelf Disclaimer
The PubMed wordmark and PubMed logo are registered trademarks of the U.S. Department of Health and Human Services (HHS). Unauthorized use of these marks is strictly prohibited.
Experimental Design: Types, Examples & Methods
Saul McLeod, PhD
Editor-in-Chief for Simply Psychology
BSc (Hons) Psychology, MRes, PhD, University of Manchester
Saul McLeod, PhD., is a qualified psychology teacher with over 18 years of experience in further and higher education. He has been published in peer-reviewed journals, including the Journal of Clinical Psychology.
Learn about our Editorial Process
Olivia Guy-Evans, MSc
Associate Editor for Simply Psychology
BSc (Hons) Psychology, MSc Psychology of Education
Olivia Guy-Evans is a writer and associate editor for Simply Psychology. She has previously worked in healthcare and educational sectors.
On This Page:
Experimental design refers to how participants are allocated to different groups in an experiment. Types of design include repeated measures, independent groups, and matched pairs designs.
Probably the most common way to design an experiment in psychology is to divide the participants into two groups, the experimental group and the control group, and then introduce a change to the experimental group, not the control group.
The researcher must decide how he/she will allocate their sample to the different experimental groups. For example, if there are 10 participants, will all 10 participants participate in both groups (e.g., repeated measures), or will the participants be split in half and take part in only one group each?
Three types of experimental designs are commonly used:
1. Independent Measures
Independent measures design, also known as between-groups , is an experimental design where different participants are used in each condition of the independent variable. This means that each condition of the experiment includes a different group of participants.
This should be done by random allocation, ensuring that each participant has an equal chance of being assigned to one group.
Independent measures involve using two separate groups of participants, one in each condition. For example:
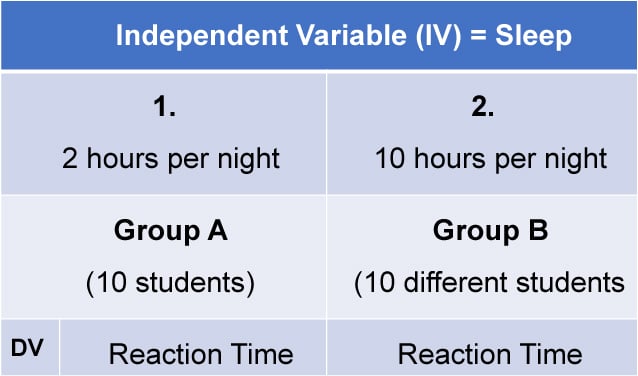
- Con : More people are needed than with the repeated measures design (i.e., more time-consuming).
- Pro : Avoids order effects (such as practice or fatigue) as people participate in one condition only. If a person is involved in several conditions, they may become bored, tired, and fed up by the time they come to the second condition or become wise to the requirements of the experiment!
- Con : Differences between participants in the groups may affect results, for example, variations in age, gender, or social background. These differences are known as participant variables (i.e., a type of extraneous variable ).
- Control : After the participants have been recruited, they should be randomly assigned to their groups. This should ensure the groups are similar, on average (reducing participant variables).
2. Repeated Measures Design
Repeated Measures design is an experimental design where the same participants participate in each independent variable condition. This means that each experiment condition includes the same group of participants.
Repeated Measures design is also known as within-groups or within-subjects design .
- Pro : As the same participants are used in each condition, participant variables (i.e., individual differences) are reduced.
- Con : There may be order effects. Order effects refer to the order of the conditions affecting the participants’ behavior. Performance in the second condition may be better because the participants know what to do (i.e., practice effect). Or their performance might be worse in the second condition because they are tired (i.e., fatigue effect). This limitation can be controlled using counterbalancing.
- Pro : Fewer people are needed as they participate in all conditions (i.e., saves time).
- Control : To combat order effects, the researcher counter-balances the order of the conditions for the participants. Alternating the order in which participants perform in different conditions of an experiment.
Counterbalancing
Suppose we used a repeated measures design in which all of the participants first learned words in “loud noise” and then learned them in “no noise.”
We expect the participants to learn better in “no noise” because of order effects, such as practice. However, a researcher can control for order effects using counterbalancing.
The sample would be split into two groups: experimental (A) and control (B). For example, group 1 does ‘A’ then ‘B,’ and group 2 does ‘B’ then ‘A.’ This is to eliminate order effects.
Although order effects occur for each participant, they balance each other out in the results because they occur equally in both groups.
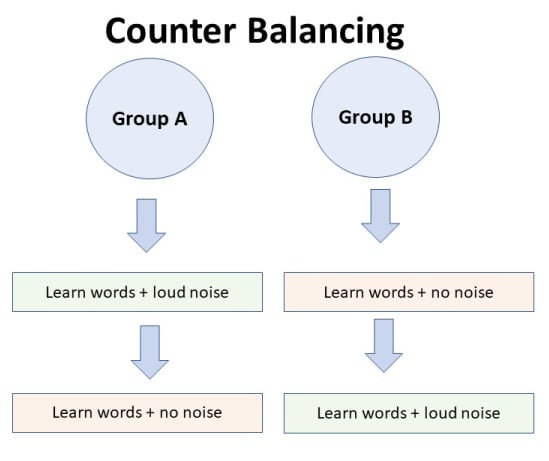
3. Matched Pairs Design
A matched pairs design is an experimental design where pairs of participants are matched in terms of key variables, such as age or socioeconomic status. One member of each pair is then placed into the experimental group and the other member into the control group .
One member of each matched pair must be randomly assigned to the experimental group and the other to the control group.
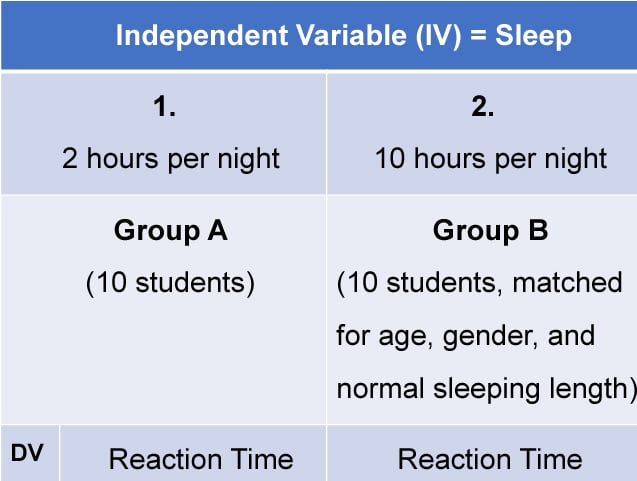
- Con : If one participant drops out, you lose 2 PPs’ data.
- Pro : Reduces participant variables because the researcher has tried to pair up the participants so that each condition has people with similar abilities and characteristics.
- Con : Very time-consuming trying to find closely matched pairs.
- Pro : It avoids order effects, so counterbalancing is not necessary.
- Con : Impossible to match people exactly unless they are identical twins!
- Control : Members of each pair should be randomly assigned to conditions. However, this does not solve all these problems.
Experimental design refers to how participants are allocated to an experiment’s different conditions (or IV levels). There are three types:
1. Independent measures / between-groups : Different participants are used in each condition of the independent variable.
2. Repeated measures /within groups : The same participants take part in each condition of the independent variable.
3. Matched pairs : Each condition uses different participants, but they are matched in terms of important characteristics, e.g., gender, age, intelligence, etc.
Learning Check
Read about each of the experiments below. For each experiment, identify (1) which experimental design was used; and (2) why the researcher might have used that design.
1 . To compare the effectiveness of two different types of therapy for depression, depressed patients were assigned to receive either cognitive therapy or behavior therapy for a 12-week period.
The researchers attempted to ensure that the patients in the two groups had similar severity of depressed symptoms by administering a standardized test of depression to each participant, then pairing them according to the severity of their symptoms.
2 . To assess the difference in reading comprehension between 7 and 9-year-olds, a researcher recruited each group from a local primary school. They were given the same passage of text to read and then asked a series of questions to assess their understanding.
3 . To assess the effectiveness of two different ways of teaching reading, a group of 5-year-olds was recruited from a primary school. Their level of reading ability was assessed, and then they were taught using scheme one for 20 weeks.
At the end of this period, their reading was reassessed, and a reading improvement score was calculated. They were then taught using scheme two for a further 20 weeks, and another reading improvement score for this period was calculated. The reading improvement scores for each child were then compared.
4 . To assess the effect of the organization on recall, a researcher randomly assigned student volunteers to two conditions.
Condition one attempted to recall a list of words that were organized into meaningful categories; condition two attempted to recall the same words, randomly grouped on the page.
Experiment Terminology
Ecological validity.
The degree to which an investigation represents real-life experiences.
Experimenter effects
These are the ways that the experimenter can accidentally influence the participant through their appearance or behavior.
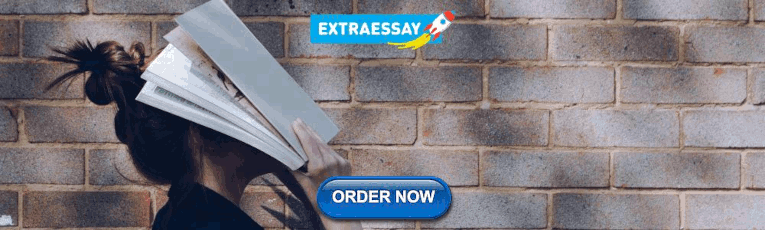
Demand characteristics
The clues in an experiment lead the participants to think they know what the researcher is looking for (e.g., the experimenter’s body language).
Independent variable (IV)
The variable the experimenter manipulates (i.e., changes) is assumed to have a direct effect on the dependent variable.
Dependent variable (DV)
Variable the experimenter measures. This is the outcome (i.e., the result) of a study.
Extraneous variables (EV)
All variables which are not independent variables but could affect the results (DV) of the experiment. Extraneous variables should be controlled where possible.
Confounding variables
Variable(s) that have affected the results (DV), apart from the IV. A confounding variable could be an extraneous variable that has not been controlled.
Random Allocation
Randomly allocating participants to independent variable conditions means that all participants should have an equal chance of taking part in each condition.
The principle of random allocation is to avoid bias in how the experiment is carried out and limit the effects of participant variables.
Order effects
Changes in participants’ performance due to their repeating the same or similar test more than once. Examples of order effects include:
(i) practice effect: an improvement in performance on a task due to repetition, for example, because of familiarity with the task;
(ii) fatigue effect: a decrease in performance of a task due to repetition, for example, because of boredom or tiredness.

Academia.edu no longer supports Internet Explorer.
To browse Academia.edu and the wider internet faster and more securely, please take a few seconds to upgrade your browser .
Enter the email address you signed up with and we'll email you a reset link.
- We're Hiring!
- Help Center
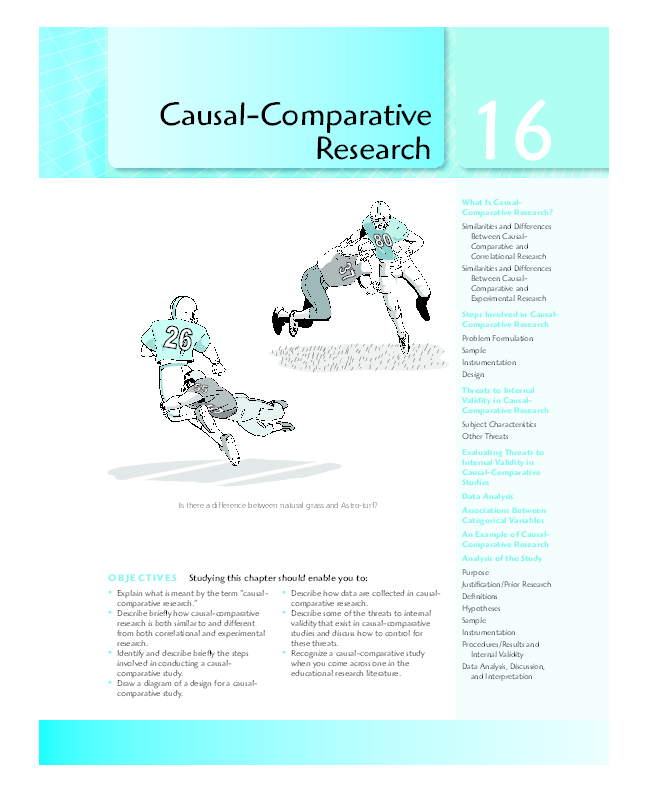
Chapter 16 Causal Comparative Research How to Design and Evaluate Research in Education 8th

Related Papers
INTERNATIONAL JOURNAL OF MULTIPLE RESEARCH APPROACHES
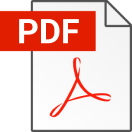
Educação & Realidade
Steven Klees
1: Comparison is the essence of science and the field of comparative and international education, like many of the social sciences, has been dominated by quantitative methodological approaches. This paper raises fundamental questions about the utility of regression analysis for causal inference. It examines three extensive literatures of applied regression analysis concerned with education policies. The paper concludes that the conditions necessary for regression analysis to yield valid causal inferences are so far from ever being met or approximated that such inferences are never valid. Alternative research methodologies are then briefly discussed.
Practising Comparison. Logics. Relations, Collaborations.
Monika Krause
Journal of educational …
Rhonda Craven
Theoretical and Methodological Approaches to Social Sciences and Knowledge Management
The Changing Academic Profession
Ulrich Teichler
Cut Eka Para Samya
Commentary on Causal Prescriptive Statements Causal prescriptive statements are necessary in the social sciences whenever there is a mission to help individuals, groups, or organizations improve. Researchers inquire whether some variable or intervention A causes an improvement in some mental, emotional, or behavioural variable B. If they are satisfied that A causes B, then they can take steps to manipulate A in the real world and thereby help people by enhancing B.
In Part 4, we begin a more detailed discussion of some of the methodologies that educational researchers use. We concentrate here on quantitative research, with a separate chapter devoted to group-comparison experimental research, single-subject experimental research, correlational research, causal-comparative research, and survey research. In each chapter, we not only discuss the method in some detail, but we also provide examples of published studies in which the researchers used one of these methods. We conclude each chapter with an analysis of a particular study's strengths and weaknesses.
Varaporn Yamtim
Causal factors and consequences of parent involvement growth: the second-order latent growth curve model
Loading Preview
Sorry, preview is currently unavailable. You can download the paper by clicking the button above.
RELATED PAPERS
Christina Nord
Emirhan Darcan PhD.
Alexis Hernandez
Prof. Marwan Dwairy
Policy Studies Journal
Herbert Asher
Facta Universitatis, Series: Teaching, Learning and Teacher Education
Marina Ciric
Roland Poellinger
Abraham Tadese
Educational Measurement: Issues and Practice
Michael Lechner
Katarzyna Steinka-Fry
J. Erola, P. Naumanen, H. Kettunen & V-M. Paasivaara (eds.). 2021. Norms, Moral and and Policy Changes: Essays in honor of Hannu Ruonavaara. Acta Universitatis Upsaliensis. pp.81-101.
Tuukka Kaidesoja
Franz-Josef Scharfenberg
Anna Kosmützky
Leadership Quarterly
Rafael Lalive
Journal of Educational Psychology
Linda Beckman
Science Park Research Organization & Counselling
The Psychological Record
Linda Hayes
Multivariate Behavioral Research
Norman Rose
Springer eBooks
Mario Coccia
The SAGE Handbook of the Philosophy of Social Sciences
Daniel Steel
International Journal of Cognitive Research in Science, Engineering and Education
Jelena Maksimović
Advances in health sciences education : theory and practice
Ineke H.a.p. Wolfhagen
RELATED TOPICS
- We're Hiring!
- Help Center
- Find new research papers in:
- Health Sciences
- Earth Sciences
- Cognitive Science
- Mathematics
- Computer Science
- Academia ©2024
Characteristics of a Comparative Research Design
Hannah richardson, 28 jun 2018.
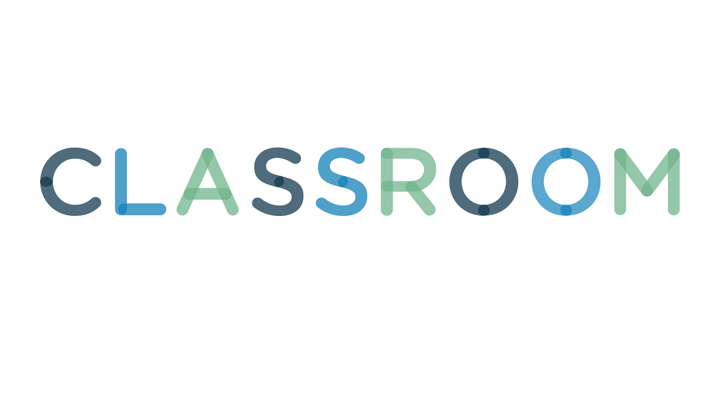
Comparative research essentially compares two groups in an attempt to draw a conclusion about them. Researchers attempt to identify and analyze similarities and differences between groups, and these studies are most often cross-national, comparing two separate people groups. Comparative studies can be used to increase understanding between cultures and societies and create a foundation for compromise and collaboration. These studies contain both quantitative and qualitative research methods.
Explore this article
- Comparative Quantitative
- Comparative Qualitative
- When to Use It
- When Not to Use It
1 Comparative Quantitative
Quantitative, or experimental, research is characterized by the manipulation of an independent variable to measure and explain its influence on a dependent variable. Because comparative research studies analyze two different groups -- which may have very different social contexts -- it is difficult to establish the parameters of research. Such studies might seek to compare, for example, large amounts of demographic or employment data from different nations that define or measure relevant research elements differently.
However, the methods for statistical analysis of data inherent in quantitative research are still helpful in establishing correlations in comparative studies. Also, the need for a specific research question in quantitative research helps comparative researchers narrow down and establish a more specific comparative research question.
2 Comparative Qualitative
Qualitative, or nonexperimental, is characterized by observation and recording outcomes without manipulation. In comparative research, data are collected primarily by observation, and the goal is to determine similarities and differences that are related to the particular situation or environment of the two groups. These similarities and differences are identified through qualitative observation methods. Additionally, some researchers have favored designing comparative studies around a variety of case studies in which individuals are observed and behaviors are recorded. The results of each case are then compared across people groups.
3 When to Use It
Comparative research studies should be used when comparing two people groups, often cross-nationally. These studies analyze the similarities and differences between these two groups in an attempt to better understand both groups. Comparisons lead to new insights and better understanding of all participants involved. These studies also require collaboration, strong teams, advanced technologies and access to international databases, making them more expensive. Use comparative research design when the necessary funding and resources are available.
4 When Not to Use It
Do not use comparative research design with little funding, limited access to necessary technology and few team members. Because of the larger scale of these studies, they should be conducted only if adequate population samples are available. Additionally, data within these studies require extensive measurement analysis; if the necessary organizational and technological resources are not available, a comparative study should not be used. Do not use a comparative design if data are not able to be measured accurately and analyzed with fidelity and validity.
- 1 San Jose State University: Selected Issues in Study Design
- 2 University of Surrey: Social Research Update 13: Comparative Research Methods
About the Author
Hannah Richardson has a Master's degree in Special Education from Vanderbilt University and a Bacheor of Arts in English. She has been a writer since 2004 and wrote regularly for the sports and features sections of "The Technician" newspaper, as well as "Coastwach" magazine. Richardson also served as the co-editor-in-chief of "Windhover," an award-winning literary and arts magazine. She is currently teaching at a middle school.
Related Articles
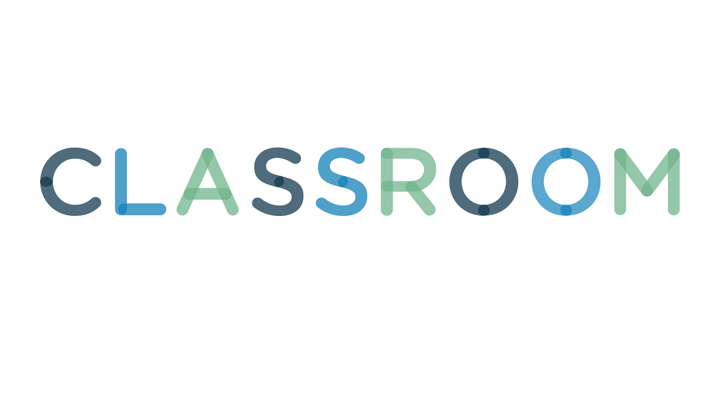
Research Study Design Types
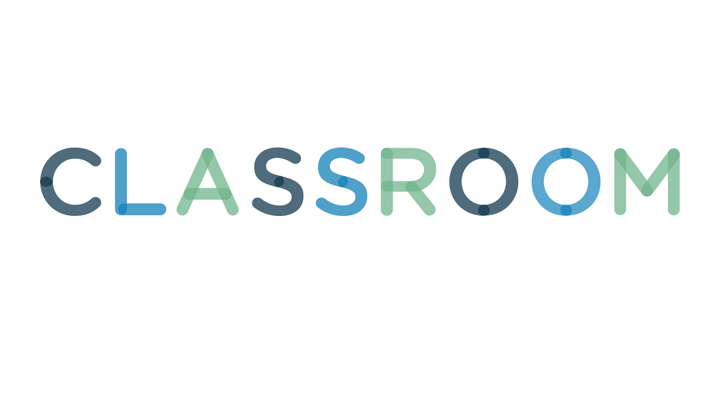
Correlational Methods vs. Experimental Methods
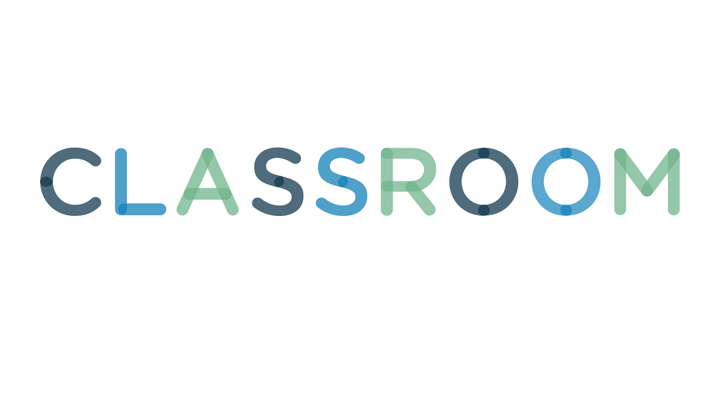
Different Types of Methodologies
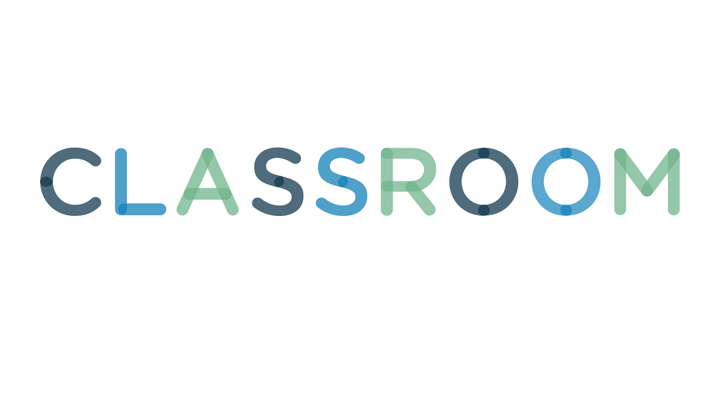
Quasi-Experiment Advantages & Disadvantages
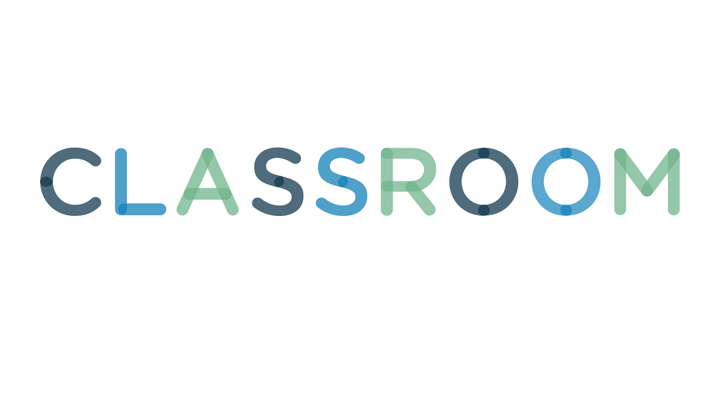
What Are the Advantages & Disadvantages of Non-Experimental...
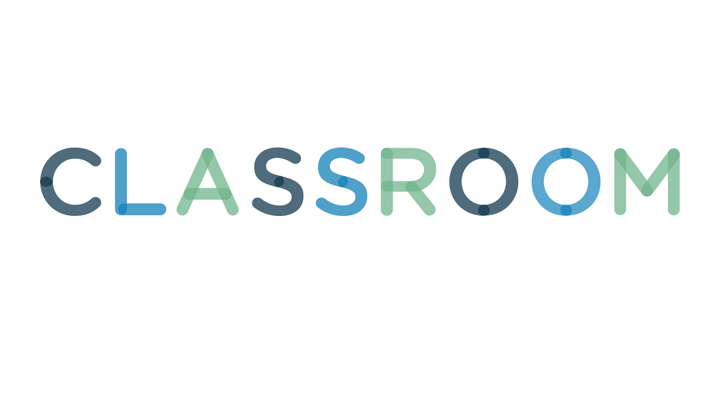
Independent vs. Dependent Variables in Sociology
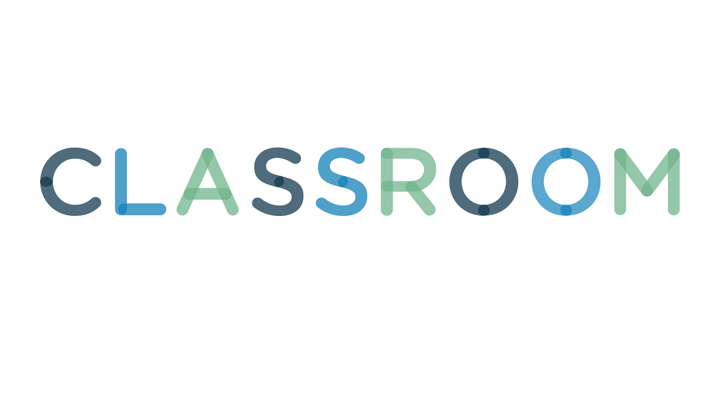
Methods of Research Design
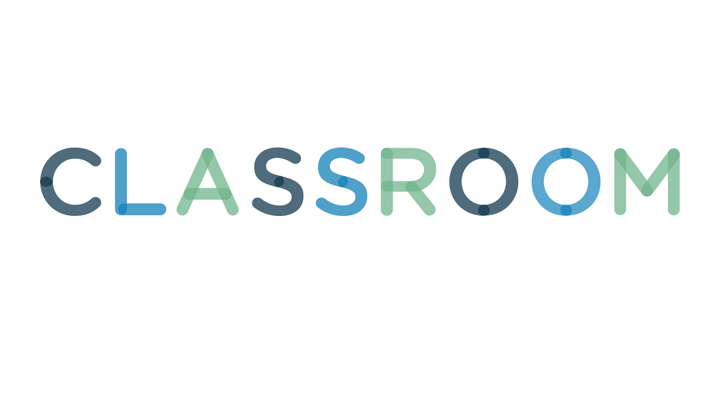
Qualitative Research Pros & Cons
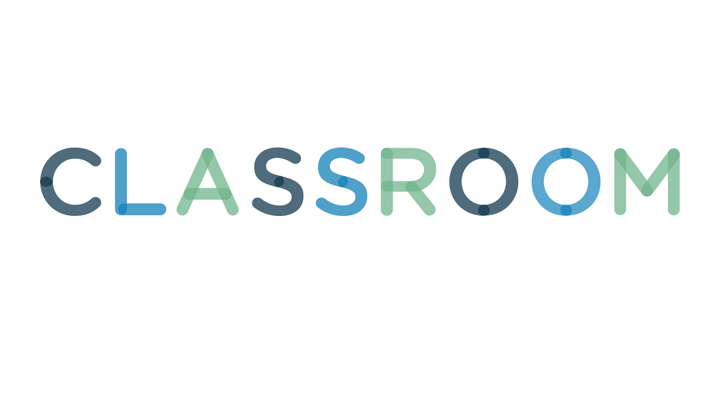
How to Form a Theoretical Study of a Dissertation
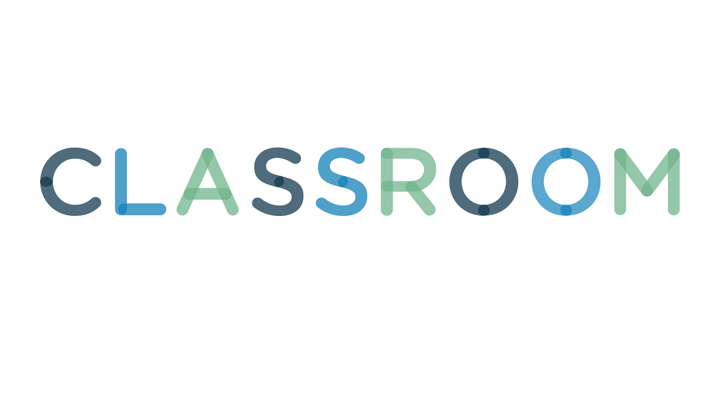
What Is the Difference Between Internal & External...
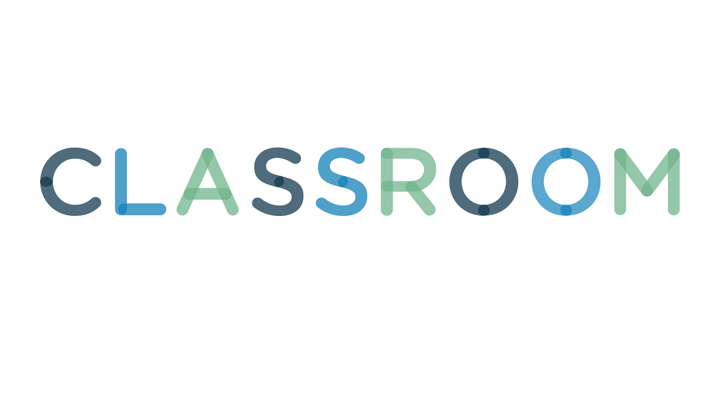
Difference Between Conceptual & Theoretical Framework
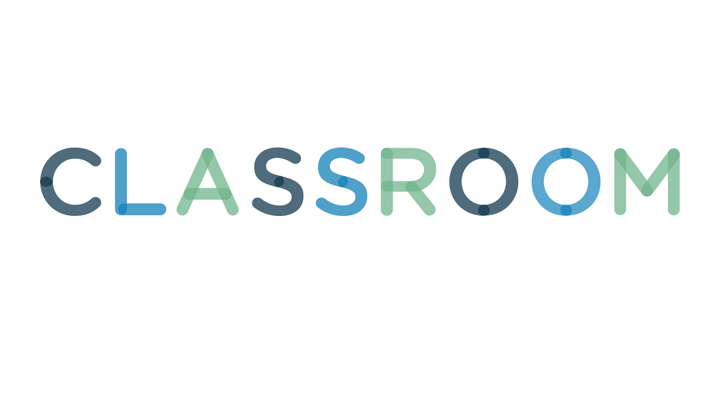
The Advantages of Exploratory Research Design
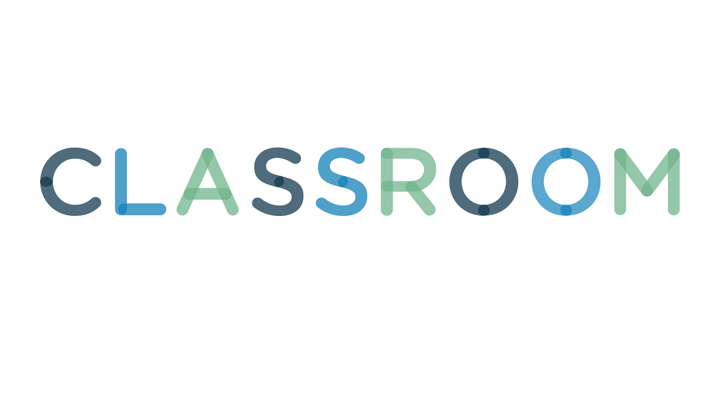
What Is Quantitative Research?
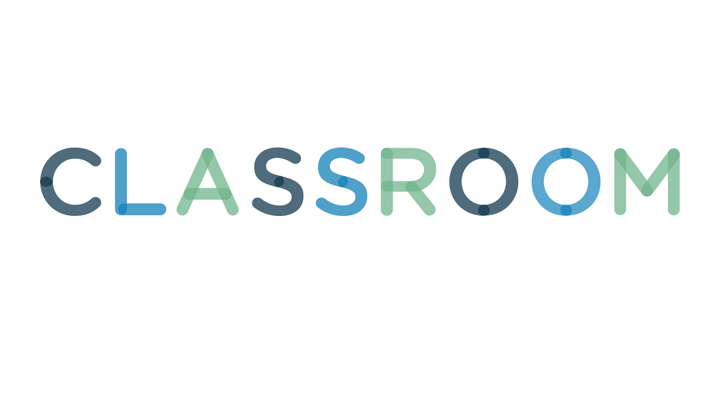
What is a Dissertation?
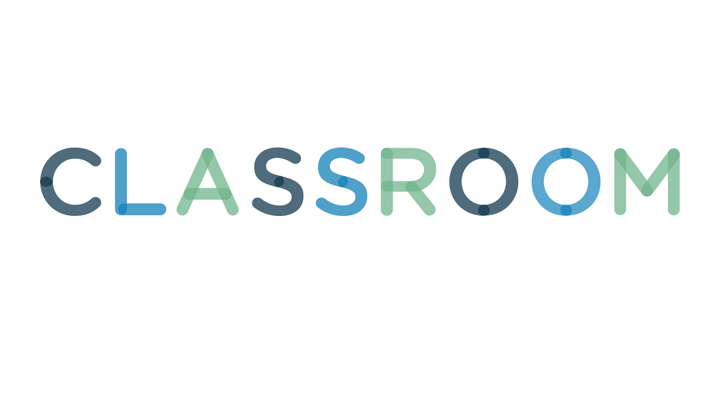
What Are the Advantages & Disadvantages of Correlation...
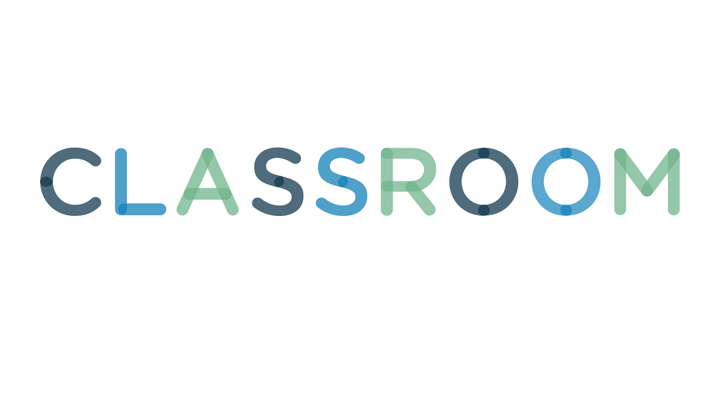
What Is the Meaning of the Descriptive Method in Research?
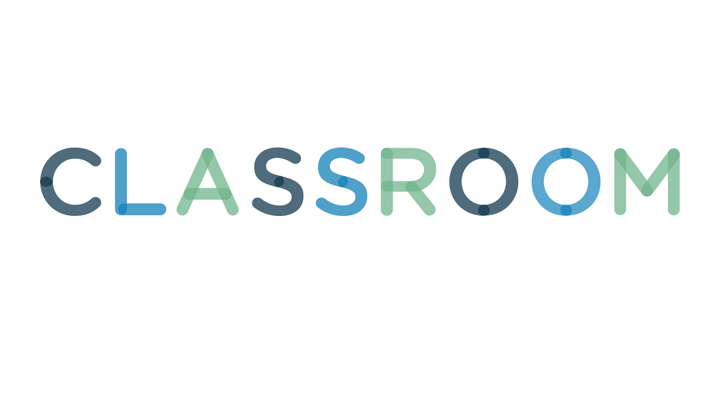
How to Use Qualitative Research Methods in a Case Study...
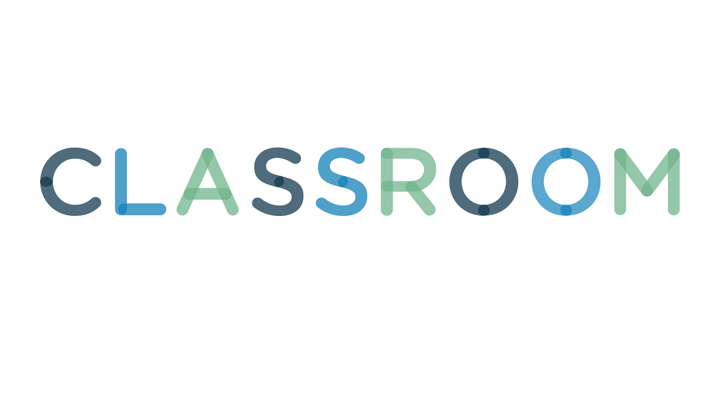
How to Tabulate Survey Results
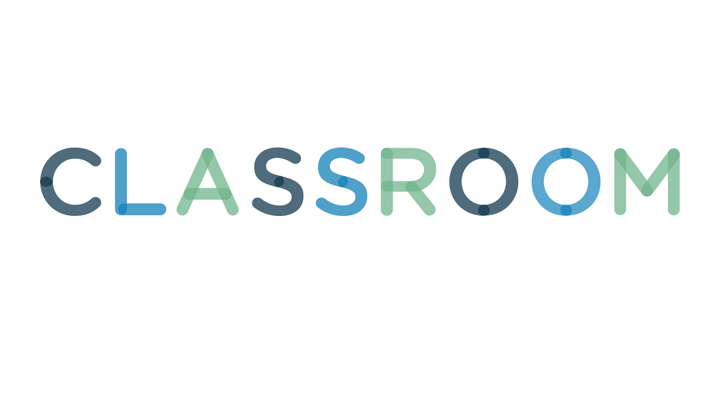
How to Cross Validate Qualitative Research Results
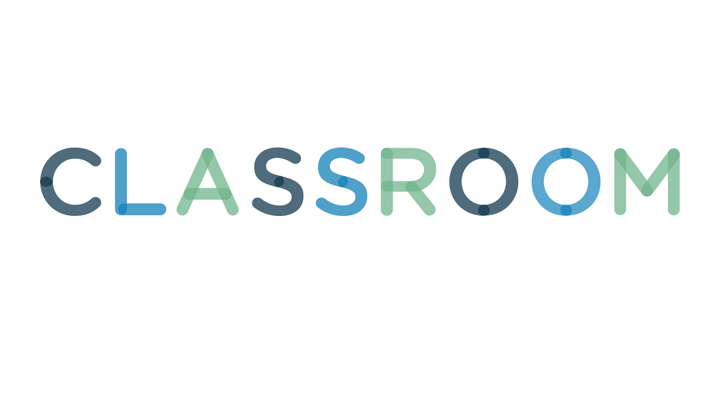
Types of Descriptive Research Methods
Regardless of how old we are, we never stop learning. Classroom is the educational resource for people of all ages. Whether you’re studying times tables or applying to college, Classroom has the answers.
- Accessibility
- Terms of Use
- Privacy Policy
- Copyright Policy
- Manage Preferences
© 2020 Leaf Group Ltd. / Leaf Group Media, All Rights Reserved. Based on the Word Net lexical database for the English Language. See disclaimer .

Causal Comparative Research: Methods And Examples
Ritu was in charge of marketing a new protein drink about to be launched. The client wanted a causal-comparative study…

Ritu was in charge of marketing a new protein drink about to be launched. The client wanted a causal-comparative study highlighting the drink’s benefits. They demanded that comparative analysis be made the main campaign design strategy. After carefully analyzing the project requirements, Ritu decided to follow a causal-comparative research design. She realized that causal-comparative research emphasizing physical development in different groups of people would lay a good foundation to establish the product.
What Is Causal Comparative Research?
Examples of causal comparative research variables.
Causal-comparative research is a method used to identify the cause–effect relationship between a dependent and independent variable. This relationship is usually a suggested relationship because we can’t control an independent variable completely. Unlike correlation research, this doesn’t rely on relationships. In a causal-comparative research design, the researcher compares two groups to find out whether the independent variable affected the outcome or the dependent variable.
A causal-comparative method determines whether one variable has a direct influence on the other and why. It identifies the causes of certain occurrences (or non-occurrences). It makes a study descriptive rather than experimental by scrutinizing the relationships among different variables in which the independent variable has already occurred. Variables can’t be manipulated sometimes, but a link between dependent and independent variables is established and the implications of possible causes are used to draw conclusions.
In a causal-comparative design, researchers study cause and effect in retrospect and determine consequences or causes of differences already existing among or between groups of people.
Let’s look at some characteristics of causal-comparative research:
- This method tries to identify cause and effect relationships.
- Two or more groups are included as variables.
- Individuals aren’t selected randomly.
- Independent variables can’t be manipulated.
- It helps save time and money.
The main purpose of a causal-comparative study is to explore effects, consequences and causes. There are two types of causal-comparative research design. They are:
Retrospective Causal Comparative Research
For this type of research, a researcher has to investigate a particular question after the effects have occurred. They attempt to determine whether or not a variable influences another variable.
Prospective Causal Comparative Research
The researcher initiates a study, beginning with the causes and determined to analyze the effects of a given condition. This is not as common as retrospective causal-comparative research.
Usually, it’s easier to compare a variable with the known than the unknown.
Researchers use causal-comparative research to achieve research goals by comparing two variables that represent two groups. This data can include differences in opportunities, privileges exclusive to certain groups or developments with respect to gender, race, nationality or ability.
For example, to find out the difference in wages between men and women, researchers have to make a comparative study of wages earned by both genders across various professions, hierarchies and locations. None of the variables can be influenced and cause-effect relationship has to be established with a persuasive logical argument. Some common variables investigated in this type of research are:
- Achievement and other ability variables
- Family-related variables
- Organismic variables such as age, sex and ethnicity
- Variables related to schools
- Personality variables
While raw test scores, assessments and other measures (such as grade point averages) are used as data in this research, sources, standardized tests, structured interviews and surveys are popular research tools.
However, there are drawbacks of causal-comparative research too, such as its inability to manipulate or control an independent variable and the lack of randomization. Subject-selection bias always remains a possibility and poses a threat to the internal validity of a study. Researchers can control it with statistical matching or by creating identical subgroups. Executives have to look out for loss of subjects, location influences, poor attitude of subjects and testing threats to produce a valid research study.
Harappa’s Thinking Critically program is for managers who want to learn how to think effectively before making critical decisions. Learn how leaders articulate the reasons behind and implications of their decisions. Become a growth-driven manager looking to select the right strategies to outperform targets. It’s packed with problem-solving and effective-thinking tools that are essential for skill development. What more? It offers live learning support and the opportunity to progress at your own pace. Ask for your free demo today!
Explore Harappa Diaries to learn more about topics such as Objectives Of Research Methodology , Types Of Thinking , What Is Visualisation and Effective Learning Methods to upgrade your knowledge and skills.
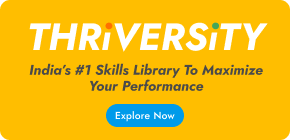
- Skip to main content
- Skip to primary sidebar
- Skip to footer
- QuestionPro

- Solutions Industries Gaming Automotive Sports and events Education Government Travel & Hospitality Financial Services Healthcare Cannabis Technology Use Case AskWhy Communities Audience Contactless surveys Mobile LivePolls Member Experience GDPR Positive People Science 360 Feedback Surveys
- Resources Blog eBooks Survey Templates Case Studies Training Help center

Home Market Research Research Tools and Apps
Causal Comparative Research: Definition, Types & Benefits
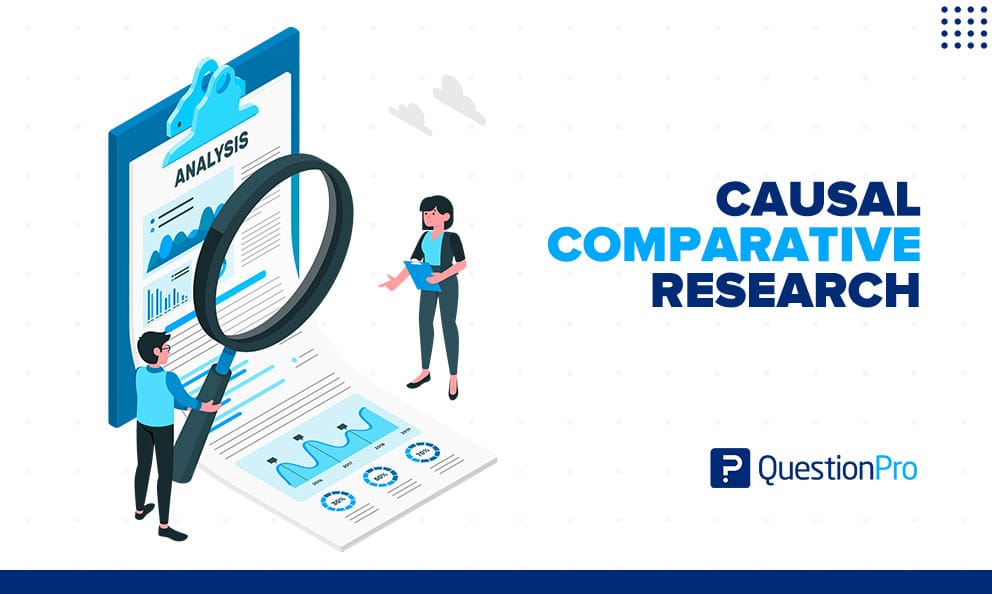
Within the field of research, there are multiple methodologies and ways to find answers to your needs, in this article we will address everything you need to know about Causal Comparative Research, a methodology with many advantages and applications.
What Is Causal Comparative Research?
Causal-comparative research is a methodology used to identify cause-effect relationships between independent and dependent variables.
Researchers can study cause and effect in retrospect. This can help determine the consequences or causes of differences already existing among or between different groups of people.
When you think of Casual Comparative Research, it will almost always consist of the following:
- A method or set of methods to identify cause/effect relationships
- A set of individuals (or entities) that are NOT selected randomly – they were intended to participate in this specific study
- Variables are represented in two or more groups (cannot be less than two, otherwise there is no differentiation between them)
- Non-manipulated independent variables – *typically, it’s a suggested relationship (since we can’t control the independent variable completely)
Types of Casual Comparative Research
Casual Comparative Research is broken down into two types:
- Retrospective Comparative Research
- Prospective Comparative Research
Retrospective Comparative Research: Involves investigating a particular question…. after the effects have occurred. As an attempt to see if a specific variable does influence another variable.
Prospective Comparative Research: This type of Casual Comparative Research is characterized by being initiated by the researcher and starting with the causes and determined to analyze the effects of a given condition. This type of investigation is much less common than the Retrospective type of investigation.
LEARN ABOUT: Quasi-experimental Research
Causal Comparative Research vs Correlation Research
The universal rule of statistics… correlation is NOT causation!
Casual Comparative Research does not rely on relationships. Instead, they’re comparing two groups to find out whether the independent variable affected the outcome of the dependent variable
When running a Causal Comparative Research, none of the variables can be influenced, and a cause-effect relationship has to be established with a persuasive, logical argument; otherwise, it’s a correlation.
Another significant difference between both methodologies is their analysis of the data collected. In the case of Causal Comparative Research, the results are usually analyzed using cross-break tables and comparing the averages obtained. At the same time, in Causal Comparative Research, Correlation Analysis typically uses scatter charts and correlation coefficients.
Advantages and Disadvantages of Causal Comparative Research
Like any research methodology, causal comparative research has a specific use and limitations to consider when considering them in your next project. Below we list some of the main advantages and disadvantages.
- It is more efficient since it allows you to save human and economic resources and to do it relatively quickly.
- Identifying causes of certain occurrences (or non-occurrences)
- Thus, descriptive analysis rather than experimental
Disadvantages
- You’re not fully able to manipulate/control an independent variable as well as the lack of randomization
- Like other methodologies, it tends to be prone to some research bias , the most common type of research is subject- selection bias , so special care must be taken to avoid it so as not to compromise the validity of this type of research.
- The loss of subjects/location influences / poor attitude of subjects/testing threats….are always a possibility
Finally, it is important to remember that the results of this type of causal research should be interpreted with caution since a common mistake is to think that although there is a relationship between the two variables analyzed, this does not necessarily guarantee that the variable influences or is the main factor to influence in the second variable.
LEARN ABOUT: ANOVA testing
QuestionPro can be your ally in your next Causal Comparative Research
QuestionPro is one of the platforms most used by the world’s leading research agencies, thanks to its diverse functions and versatility when collecting and analyzing data.
With QuestionPro you will not only be able to collect the necessary data to carry out your causal comparative research, you will also have access to a series of advanced reports and analyses to obtain valuable insights for your research project.
We invite you to learn more about our Research Suite, schedule a free demo of our main features today, and clarify all your doubts about our solutions.
LEARN MORE SIGN UP FREE
Author : John Oppenhimer
MORE LIKE THIS
Top 5 change management models to transform your organization.
Sep 20, 2024
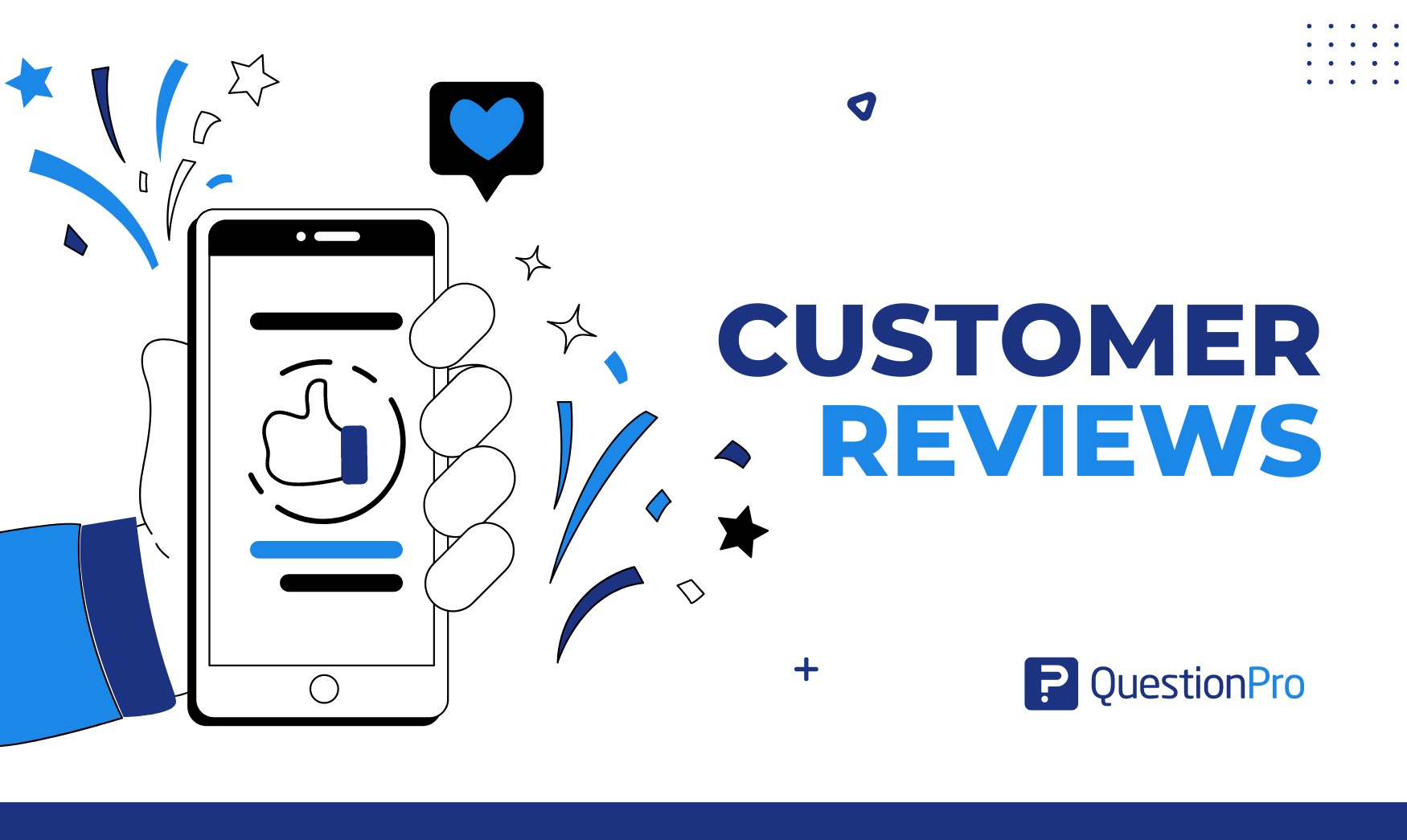
Customer Reviews: How to Ask and Easy Ways to Get Them
Sep 19, 2024
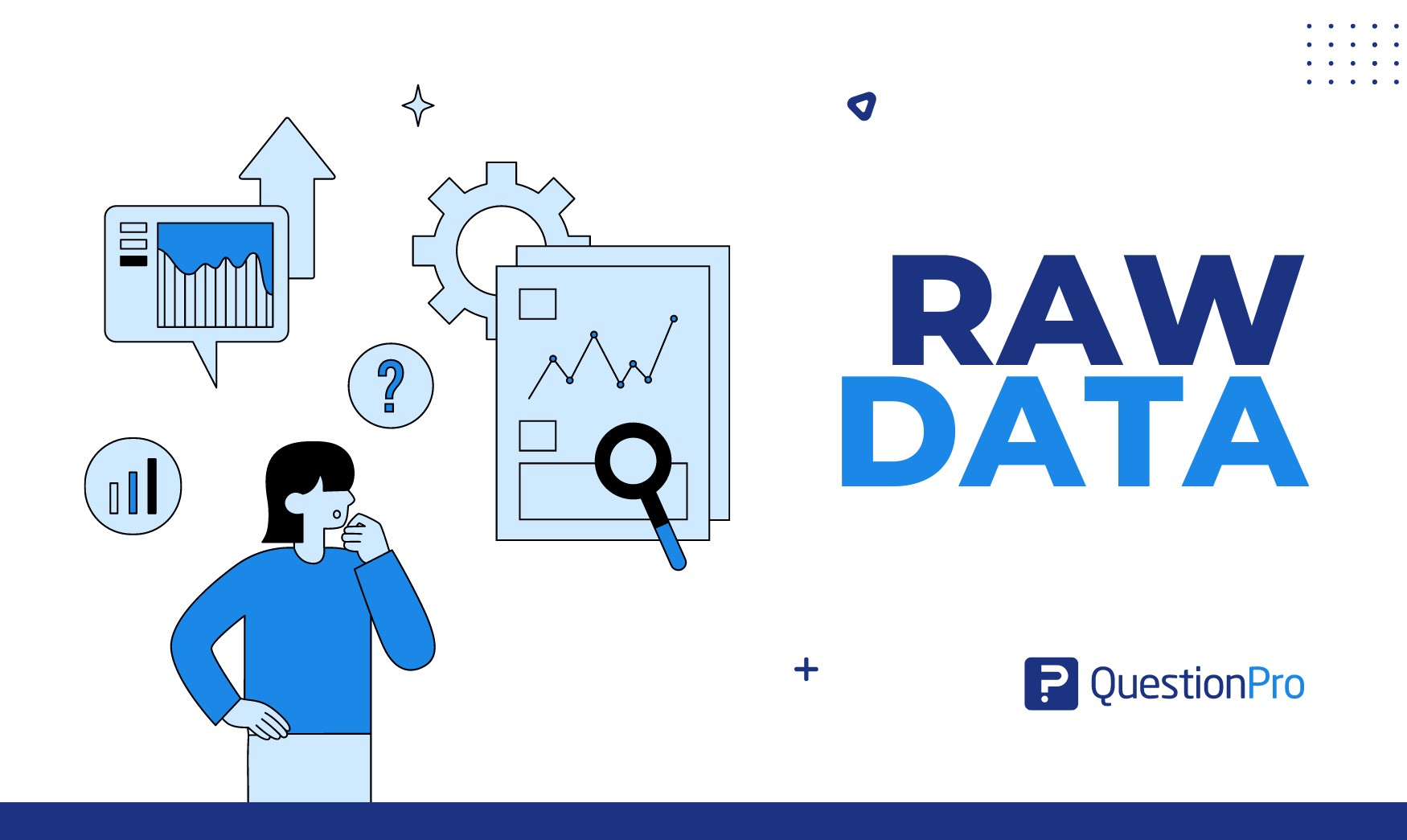
Raw Data: What it is + How to Process It
Sep 18, 2024
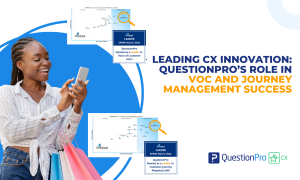
QuestionPro: Leading the Charge in Customer Journey Management and Voice of the Customer Platforms
Sep 17, 2024
Other categories
- Academic Research
- Artificial Intelligence
- Assessments
- Brand Awareness
- Case Studies
- Communities
- Consumer Insights
- Customer effort score
- Customer Engagement
- Customer Experience
- Customer Loyalty
- Customer Research
- Customer Satisfaction
- Employee Benefits
- Employee Engagement
- Employee Retention
- Friday Five
- General Data Protection Regulation
- Insights Hub
- Life@QuestionPro
- Market Research
- Mobile diaries
- Mobile Surveys
- New Features
- Online Communities
- Question Types
- Questionnaire
- QuestionPro Products
- Release Notes
- Research Tools and Apps
- Revenue at Risk
- Survey Templates
- Training Tips
- Tuesday CX Thoughts (TCXT)
- Uncategorized
- What’s Coming Up
- Workforce Intelligence
Advertisement
Comparative Analysis of Response Surface Methodology (RSM) and Taguchi Method: Optimization Hydraulic Ram Pump Performance
- Published: 21 September 2024
- Volume 5 , article number 85 , ( 2024 )
Cite this article
- Chahyani Romelin ORCID: orcid.org/0009-0008-1085-1948 1 ,
- Zahedi ORCID: orcid.org/0000-0002-5579-7024 1 &
- Badai Charamsar Nusantara ORCID: orcid.org/0009-0009-7616-0514 2
Hydraulic ram pumps offer an energy-efficient solution for water lifting, which is crucial in rural areas with limited electricity access. Comparative analysis using the Response Surface Methodology (RSM) and Taguchi method reveals distinct experimental designs and optimization outcomes. RSM entails twenty experiments, yielding optimal points at input height (3 m), input length (12 m), and vacuum tube length (120 cm). In contrast, Taguchi employs nine experiments, with optimal points at input height (3 m), input length (6 m), and vacuum tube length (120 cm). For the equation model, the RSM method shows a complex mathematical equation involving interactions between variables, while the Taguchi method provides a more straightforward equation. As for the most optimal variable, when viewed from the significant value in both methods in the ANOVA table, it is found that the input height variable is the most significant variable in optimizing the response (discharge). A better understanding of these two methods can help the selection of appropriate methods for specific situations, strengthen the knowledge of hydram pump performance, and contribute to developing more efficient and sustainable hydram pump technology.
This is a preview of subscription content, log in via an institution to check access.
Access this article
Subscribe and save.
- Get 10 units per month
- Download Article/Chapter or eBook
- 1 Unit = 1 Article or 1 Chapter
- Cancel anytime
Price includes VAT (Russian Federation)
Instant access to the full article PDF.
Rent this article via DeepDyve
Institutional subscriptions
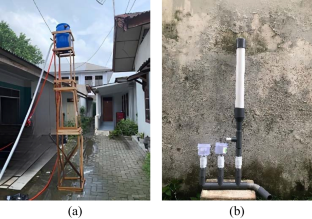
Data Availability
No datasets were generated or analysed during the current study.
Mallareddy M et al (2023) Maximizing water use efficiency in rice farming: a comprehensive review of innovative irrigation management technologies. Water (Switzerland) 15(10):1802. https://doi.org/10.3390/w15101802
Article Google Scholar
Jabbar WA et al (2024) Development of LoRaWAN-based IoT system for water quality monitoring in rural areas. Expert Syst Appl 242(December 2023):122862. https://doi.org/10.1016/j.eswa.2023.122862
Zakariazadeh A, Ahshan R, Al Abri R, Al-Abri M (2024) Renewable energy integration in sustainable water systems: a review. Clean Eng Technol 18(December 2023):100722. https://doi.org/10.1016/j.clet.2024.100722
Gopal C, Mohanraj M, Chandramohan P, Chandrasekar P (2013) Renewable energy source water pumping systems - a literature review. Renew Sustain Energy Rev 25:351–370. https://doi.org/10.1016/j.rser.2013.04.012
Liang Y et al (2024) Novel approach to remote rural heating: direct coupled photovoltaic electric heater underfloor heating system with phase change materials. Appl Therm Eng 250(13):123525. https://doi.org/10.1016/j.applthermaleng.2024.123525
Hassan Q, Algburi S, Sameen AZ, Salman HM, Jaszczur M (2023) A review of hybrid renewable energy systems: solar and wind-powered solutions: challenges, opportunities, and policy implications. Results Eng 20(November):101621. https://doi.org/10.1016/j.rineng.2023.101621
Andrade-Cedeno RJ et al (2023) A sustainable and efficient alternative for water pumping in electrically isolated rural areas of Ecuador. Energy Rep 10(August):719–733. https://doi.org/10.1016/j.egyr.2023.07.027
Rahman M, Rabha DK, Nath T (2023) The performance analysis of hydraulic ram pump: influence of specific parameters and validation with comsol-multiphysics. Int J Exp Res Rev 32:216–234. https://doi.org/10.52756/ijerr.2023.v32.019
Zeidan M, Ostfeld A (2022) Hydraulic ram pump integration into water distribution systems for energy recovery application. Water (Switzerland) 14(1):21. https://doi.org/10.3390/w14010021
Pan J, Khajepour A, Li Y, Yang J, Liu W (2021) Performance and power consumption optimization of a hydraulic variable valve actuation system. Mechatronics 73:102479. https://doi.org/10.1016/j.mechatronics.2020.102479
Nayak S, Rao M (2019) Design and development of a flexurally amplified piezoelectric actuator based piezo-hydraulic pump. Mater Today Proc 46(xxxx):9956–9965. https://doi.org/10.1016/j.matpr.2021.03.314
Fatahi-Alkouhi R, Lashkar-Ara B, Keramat A (2019) On the measurement of ram-pump power by changing in water hammer pressure wave energy. Ain Shams Eng J 10(4):681–693. https://doi.org/10.1016/j.asej.2019.05.001
Dani A, Al Quraan E (2023) Investigating research students’ perceptions about statistics and its impact on their choice of research approach. Heliyon 9(10):e20423. https://doi.org/10.1016/j.heliyon.2023.e20423
Chen WH et al (2022) A comprehensive review of thermoelectric generation optimization by statistical approach: Taguchi method, analysis of variance (ANOVA), and response surface methodology (RSM). Renew Sustain Energy Rev 169(September):112917. https://doi.org/10.1016/j.rser.2022.112917
Jamil MK et al (2024) Multi-objective RSM-based optimization of diesel-diethyl ether blends in diesel engine to achieve sustainable development goals. Case Stud Therm Eng 59(December 2023):104542. https://doi.org/10.1016/j.csite.2024.104542
Haq MZU, Sood H, Kumar R, Merta I (2024) Taguchi-optimized triple-aluminosilicate geopolymer bricks with recycled sand: a sustainable construction solution. Case Stud Constr Mater 20(December 2023):e02780. https://doi.org/10.1016/j.cscm.2023.e02780
Reza A, Chen L, Mao X (2024) Response surface methodology for process optimization in livestock wastewater treatment: a review. Heliyon 10(9):e30326. https://doi.org/10.1016/j.heliyon.2024.e30326
Muthuram N, SriramMadhav P, KeerthiVasan D, Mohan ME, Prajeeth G (2022) A review of recent literatures in poly jet printing process. Mater Today Proc 68:1906–1920. https://doi.org/10.1016/j.matpr.2022.08.090
Cetin E, TagniFossi C (2023) Experimental investigation on mechanical strength of adhesively bonded 3D-printed joints under hygrothermal conditions using Taguchi method. Int J Adhes Adhes 126(July):103472. https://doi.org/10.1016/j.ijadhadh.2023.103472
Tura AD, Isaya EO, Adizue UL, Farkas BZ, Takács M (2024) Optimization of ultra-precision CBN turning of AISI D2 using hybrid GA-RSM and Taguchi-GRA statistic tools. Heliyon 10(11):e31849. https://doi.org/10.1016/j.heliyon.2024.e31849
Bhadani K, Asbjornsson G, Hofling K, Hulthen E, Evertsson M (2023) Application of design of experiments (DoE) in evaluating crushing-screening performance for aggregates production. Miner Eng 209(July):2024. https://doi.org/10.1016/j.mineng.2024.108616
Ostovar S, Saravani H, Akbari M, Salehpour A, Sabaghi M, Rezazadeh E (2024) The effective adsorption of Ni(II) and nitrate from aquatic systems by superparamagnetic MoS2/γ-Fe2O3 nanocomposites: optimization through RSM-CCD design. Arab J Chem 17(3):105599. https://doi.org/10.1016/j.arabjc.2024.105599
Gurugubelli S, Chekuri RBR, Penmetsa RV (2022) Experimental investigation and optimization of turning process of EN8 steel using Taguchi L9 orthogonal array. Mater Today Proc 58:233–237. https://doi.org/10.1016/j.matpr.2022.01.474
Gupta RK, Singh RC (2024) Optimizing high-speed rotating shaft vibration control: experimental investigation of squeeze film dampers and a comparative analysis using Artificial Neural Networks (ANN) and Response Surface Methodology (RSM). Expert Syst Appl 249(PB):123800. https://doi.org/10.1016/j.eswa.2024.123800
Karkare YY, Rathod WR, Sathe VS, Chavan AR (2023) Combined ultrasound-microwave assisted synthesis of aripiprazole: process optimization using RSM-ANN. Chem Eng Process - Process Intensif 183(December 2022):109250. https://doi.org/10.1016/j.cep.2022.109250
Santhosh AJ, Tura AD, Jiregna IT, Gemechu WF, Ashok N, Ponnusamy M (2021) Optimization of CNC turning parameters using face centred CCD approach in RSM and ANN-genetic algorithm for AISI 4340 alloy steel. Results Eng 11:100251. https://doi.org/10.1016/j.rineng.2021.100251
Toghraie D, Tamrabad SNH, Alidoust S, Hatami H (2023) Obtaining the optimal lubrication conditions by investigating the viscosity of MWCNT (25%)-TiO2(75%)/oil SAE40 hybrid nanofluid by response surface methodology. Tribol Int 186(May):108585. https://doi.org/10.1016/j.triboint.2023.108585
Adelodun AA (2021) Modeling the operational conditions of nonthermal plasma decomposition of airborne VOCs. Environ Challenges 3(February):100063. https://doi.org/10.1016/j.envc.2021.100063
Esbo MR, Firouzjaee MR, Farahabadi HB, Alizadeh E (2024) Performance investigation of a standalone renewable energy system using response surface methodology (RSM): 4E analysis and multi-objective optimization. Energy Convers Manag 299(November 2023):117752. https://doi.org/10.1016/j.enconman.2023.117752
Liu S et al (2024) Effects of nitrogen and carbon source addition on biomass and protein production by Rhodopseudomonas via the RSM-CCD approach. Desalin Water Treat 319:100438. https://doi.org/10.1016/j.dwt.2024.100438
Onu CE, Nwabanne JT, Ohale PE, Asadu CO (2021) Comparative analysis of RSM, ANN and ANFIS and the mechanistic modeling in eriochrome black-T dye adsorption using modified clay. South African J Chem Eng 36(January 2021):24–42. https://doi.org/10.1016/j.sajce.2020.12.003
Mushtaq RT et al (2024) Multi-objective optimization of laser polishing parameters for enhanced mechanical properties, sustainability, and surface finish of 3D-printed industrial ABS polymers using response surface methodology (RSM). J Mater Res Technol 29(023):3168–3184. https://doi.org/10.1016/j.jmrt.2024.02.023
Govindasamy R, Subramani S, Kumar PD, Rao G LN (2024) Optimization of design and fuel parameters of a DI CI engine through desirability function and RSM for lower emissions and clean environment. J Energy Inst 114(x):101607. https://doi.org/10.1016/j.joei.2024.101607
Manikandan P, Chandran NS, Vasugi V, Narendra S (2024) Application of Taguchi approach to optimize the waste glass powder in developing eco-friendly ternary blended aluminosilicate matrix. Case Stud Constr Mater 21(June):e03398. https://doi.org/10.1016/j.cscm.2024.e03398
Yin X, Muhieldeen MW, Razman R, Yong Chung Ee J (2023) Multi-objective optimization of window configuration and furniture arrangement for the natural ventilation of office buildings using Taguchi-based grey relational analysis. Energy Build 296(July):113385. https://doi.org/10.1016/j.enbuild.2023.113385
Patil S et al (2024) Evaluation and optimization of mechanical properties of laterized concrete containing fly ash and steel fiber using Taguchi robust design method. Alexandria Eng J 87(December 2023):682–706. https://doi.org/10.1016/j.aej.2023.12.009
Yadav R, Lee HH, Meena A, Sharma YK (2022) Effect of alumina particulate and E-glass fiber reinforced epoxy composite on erosion wear behavior using Taguchi orthogonal array. Tribol Int 175(July):107860. https://doi.org/10.1016/j.triboint.2022.107860
Abdullah MS, Ismail F (2024) Optimization of Savonius rotor blade performance using Taguchi method: experimental and 3D-CFD approach. Energy 303(December 2023):131801. https://doi.org/10.1016/j.energy.2024.131801
Gökler SH (2023) Prediction of Covid-19 confirmed cases and deaths using hybrid support vector machine-Taguchi method. Comput Ind Eng 191(December):2024. https://doi.org/10.1016/j.cie.2024.110103
Xie S, Kan Y, Li Y, Li Y, Jiang S, Song M (2024) Responses analysis and structural optimization of a semisubmersible platform based on the Taguchi experiment method. Ocean Eng 306(April):118074. https://doi.org/10.1016/j.oceaneng.2024.118074
Chen WH, Wang YS, Chang MH, Show PL, Hoang AT (2024) Operation parameter interaction and optimization of vertical axis wind turbine analyzed by Taguchi method with modified additive model and ANOVA. Energy Rep 11(April):5189–5200. https://doi.org/10.1016/j.egyr.2024.04.070
Kantasiri T, Kasemsiri P, Pongsa U, Posi P, Chindaprasirt P (2024) Optimization of concrete containing waste crumb rubber mix design for thermal insulating applications using Taguchi method. Constr Build Mater 434(September 2023):136636. https://doi.org/10.1016/j.conbuildmat.2024.136636
Xie F, Liang Z, Lai K, Liu Y, Wang Z, Li X (2024) Influence of operating parameters on hydrogen DISI engine at injection pressure-drop by experimental investigation and Taguchi method. Fuel 362(December 2023):130840. https://doi.org/10.1016/j.fuel.2023.130840
Download references
Acknowledgements
I would like to express my deepest gratitude to all those who have supported me throughout the preparation of this journal. My heartfelt thanks go to Winona, Sonyia, and Kania for their invaluable assistance as discussion partners during the writing process. I am also profoundly grateful to Nabila, Rahel, Fidelia, Agnes, and Dita for their help in gathering research data, as well as for their unwavering moral support and insightful advice throughout the research process. Thank you all for your contributions and encouragement.
Author information
Authors and affiliations.
Department of Mathematics, Faculty of Mathematics and Natural Sciences, Universitas Sumatera Utara, Jl. Bioteknologi No.1 USU Campus Padang Bulan, Medan, 20155, Indonesia
Chahyani Romelin & Zahedi
Department of Mechanical Engineering, Faculty of Engineering, Universitas Sumatera Utara, Jl. Almamater Kampus USU, Medan, 20155, Indonesia
Badai Charamsar Nusantara
You can also search for this author in PubMed Google Scholar
Contributions
1. Contribution of Author 1:
Author 1 acts as the lead author who initiated the research idea. Author 1 is responsible for developing the research concept, drafting the theoretical framework, and writing the main parts of the manuscript, including the background, literature review, and methodology.
2. Author 2:
Author 2 takes primary responsibility for the overall research. Author 2 critically evaluates the writing, ensuring the accuracy and scientific consistency of the entire manuscript. In addition, author 2 is also the main drafter in the application of scientific writing rules, ensuring the research meets applicable methodological and ethical standards.
3. Author 3:
Author 3 is responsible for the development of the experimental concept for the hydraulic ram pump research and analysis of the response results. Author 3 designed the experimental methodology, conducted data collection and analysis, and interpreted the experimental results. The findings were synthesised and integrated into the results and discussion section of the manuscript.
In addition, all authors were supportive of each other’s responsibilities, working together to ensure the success of the research and the quality of the manuscript. This collaboration included constructive discussions, sharing insights, and providing valuable feedback at every stage of the research and writing.
Corresponding author
Correspondence to Zahedi .
Ethics declarations
Competing interests.
The authors declare no competing interests.
Additional information
Publisher's note.
Springer Nature remains neutral with regard to jurisdictional claims in published maps and institutional affiliations.
Rights and permissions
Springer Nature or its licensor (e.g. a society or other partner) holds exclusive rights to this article under a publishing agreement with the author(s) or other rightsholder(s); author self-archiving of the accepted manuscript version of this article is solely governed by the terms of such publishing agreement and applicable law.
Reprints and permissions
About this article
Romelin, C., Zahedi & Nusantara, B.C. Comparative Analysis of Response Surface Methodology (RSM) and Taguchi Method: Optimization Hydraulic Ram Pump Performance. Oper. Res. Forum 5 , 85 (2024). https://doi.org/10.1007/s43069-024-00359-z
Download citation
Received : 07 July 2024
Accepted : 24 August 2024
Published : 21 September 2024
DOI : https://doi.org/10.1007/s43069-024-00359-z
Share this article
Anyone you share the following link with will be able to read this content:
Sorry, a shareable link is not currently available for this article.
Provided by the Springer Nature SharedIt content-sharing initiative
- Hydraulic ram pump
- Irrigation technology
- Optimization
- Find a journal
- Publish with us
- Track your research
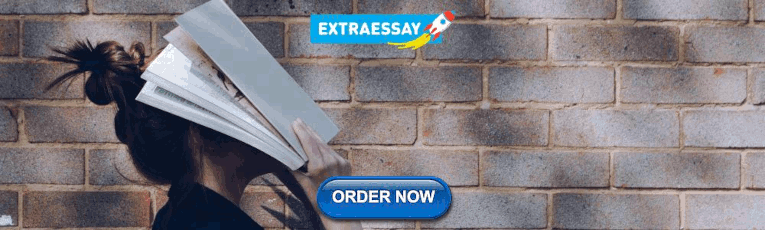
IMAGES
VIDEO
COMMENTS
Figure 1: Design and reporting of a single-factor experiment with three levels using a two-sample t-test. (a) Two treated samples (A and B) with n = 17 are compared to a control (C) with n = 17 ...
For comparative studies, the design options are experimental versus observational and prospective versus retrospective. The quality of eHealth comparative studies depends on such aspects of methodological design as the choice of variables, sample size, sources of bias, confounders, and adherence to quality and reporting guidelines.
Comparative method or quasi-experimental---a method used to describe similarities and differences in variables in two or more groups in a natural setting, that is, it resembles an experiment as it uses manipulation but lacks random assignment of individual subjects. Instead it uses existing groups.
Comparative research in communication and media studies is conventionally understood as the contrast among different macro-level units, such as world regions, countries, sub-national regions, social milieus, language areas and cultural thickenings, at one point or more points in time. ... Such quasi-experimental research designs often forbid a ...
In contrast to the chapters on survey research, experimentation, or content analysis that described a distinct set of skills, in this chapter, a variety of comparative research techniques are discussed. What makes a study comparative is not the particular techniques employed but the theoretical orientation and the sources of data.
A comparative design involves studying variation by comparing a limited number of cases without using statistical probability analyses. Such designs are particularly useful for knowledge development when we lack the conditions for control through variable-centred, quasi-experimental designs. Comparative designs often combine different research ...
Design of Comparative Experiments ... to probability theory, operations research, optimization, and mathematical programming. The books contain clear presentations of new developments in the field and also of the state of ... 8.1 Experimental units bigger than observational units 131
Learn how to use comparative designs to study variation by comparing a limited number of cases without statistical methods. Explore the different strategies for case-centred and variable-centred comparisons, and how they can be combined with case analysis.
Comparative is a concept that derives from the verb "to compare" (the etymology is Latin comparare, derivation of par = equal, with prefix com-, it is a systematic comparison).Comparative studies are investigations to analyze and evaluate, with quantitative and qualitative methods, a phenomenon and/or facts among different areas, subjects, and/or objects to detect similarities and/or ...
Research goals. Comparative communication research is a combination of substance (specific objects of investigation studied in diferent macro-level contexts) and method (identification of diferences and similarities following established rules and using equivalent concepts).
Food Research International, Vol. 43, Issue. 2, p. 526. ... 'This is 'the beauty and joy of experimental design': a mathematically beautiful and eloquently written treatise by the master!' ... It treats the design of comparative experiments with a unique approach not seen in other books …A must-read for anyone designing experiments or ...
The study design used to answer a particular research question depends on the nature of the question and the availability of resources. In this article, which is the first part of a series on "study designs," we provide an overview of research study designs and their classification. The subsequent articles will focus on individual designs.
Learn how to choose the right type of research design for your project based on the research aims, data, sampling, timescale, and location. Compare different types of research designs with examples and tips.
A comparative study is a kind of method that analyzes phenomena and then put them together. to find the points of differentiation and similarity (MokhtarianPour, 2016). A comparative perspective ...
• Descriptive Comparatives: is a non-experimental, quantitative research design. It is a casual comparative research and pre-experimental research (Cantrell, 2011) • Experiments: testing ...
Pre-experimental research is of three types —. One-shot Case Study Research Design. One-group Pretest-posttest Research Design. Static-group Comparison. 2. True Experimental Research Design. A true experimental research design relies on statistical analysis to prove or disprove a researcher's hypothesis.
Research designs are broadly divided into observational studies (i.e. cross-sectional; case-control and cohort studies) and experimental studies (randomised control trials, RCTs). Each design has a specific role, and each has both advantages and disadvantages. Moreover, while the typical RCT is a pa …
This is thanks in large part to your strategic research design. As you prepare for your quantitative dissertation research, you'll need to think about structuring your research design. There are several types of quantitative research designs, such as the experimental, comparative or predictive correlational designs.
Three types of experimental designs are commonly used: 1. Independent Measures. Independent measures design, also known as between-groups, is an experimental design where different participants are used in each condition of the independent variable. This means that each condition of the experiment includes a different group of participants.
In Part 4, we begin a more detailed discussion of some of the methodologies that educational researchers use. We concentrate here on quantitative research, with a separate chapter devoted to group-comparison experimental research, single-subject experimental research, correlational research, causal-comparative research, and survey research.
Experimental design is a discipline within statistics concerned with the analysis and design of experiments. Design is intended to help research create experiments such that cause and effect can be established from tests of the hypothesis. We introduced elements of experimental design in Chapter 2.4. Here, we expand our discussion of ...
Learn what comparative research design is, how it compares two groups across different contexts, and what methods and challenges it involves. Find out when to use and when not to use this design for your study.
In a causal-comparative research design, the researcher compares two groups to find out whether the independent variable affected the outcome or the dependent variable. A causal-comparative method determines whether one variable has a direct influence on the other and why. It identifies the causes of certain occurrences (or non-occurrences).
Causal-comparative research is a methodology used to identify cause-effect relationships between independent and dependent variables. Researchers can study cause and effect in retrospect. This can help determine the consequences or causes of differences already existing among or between different groups of people.
Hydraulic ram pumps offer an energy-efficient solution for water lifting, which is crucial in rural areas with limited electricity access. Comparative analysis using the Response Surface Methodology (RSM) and Taguchi method reveals distinct experimental designs and optimization outcomes. RSM entails twenty experiments, yielding optimal points at input height (3 m), input length (12 m), and ...